Detailed comparison between R language and python
Comparing the r language and python, both have their own merits. Which one you want to learn can be made according to your actual needs. Of course, it is best to learn both.
Related recommendations: "python video"
##01 Development purpose
R The language R was developed by statisticians. It was born with the important mission of statistical analysis, drawing, and data mining. Therefore, there are a lot of statistical principles and knowledge in the R language system. If you have some statistical background, R will make it more enjoyable and refreshing for you to use various models and complex formulas, because you can always find the corresponding package and you can call it with just a few lines of code.02 Applicable people
03 Learning Curve
This is one of the questions that beginners are most concerned about before getting started. Which one is more difficult to learn? In fact, because we don’t understand everyone’s knowledge background and learning costs, this question cannot give a black-and-white absolute answer. This is why users of R and Python on various forums always have different opinions on how difficult it is to get started.04 Industry Selection & Development Direction
There are a lot of data comparing the popularity of R and Python on the Internet. Overall, Python ranks higher, mainly The reason is that R is only used in the context of data science, while Python, as a general-purpose language, is widely used.
05 Comparative analysis of advantages and disadvantages
data visualization
Words are not as good as tables, tables are not as good as pictures. R and visualization are a perfect match. Some essential visualization software packages are such as ggplot2, ggvis, googleVis and rCharts. Due to the complete statistical model and exquisite detailed design, in R you can quickly complete a beautiful and grand layout using one or a few lines of code. 100% data graph, you can clearly see the characteristics and trends of the data.
Python also has some good visualization libraries, such as Matplotlib, Seaborn, Bokeh and Pygal. It can also complete data graphs as beautiful as R, but you need to write your own code to express and define them, such as Line graphs, bar graphs, distance and proportion of horizontal and vertical coordinates, color selection, etc.
Data Analysis
R contains more built-in functions for data analysis. You can directly use the summary built-in function. Dataframe is built-in to R. structure.
Python needs to rely on third-party software packages, such as statsmodels and pandas packages, to provide powerful data analysis functions.
Data structure
The data structure in R is very simple, mainly including vectors (one-dimensional), multi-dimensional arrays (two-dimensional) matrix), list (unstructured data), data frame (structured data). The variable type of R is relatively simple, and the variable type is the same in different packages.
Python contains richer data structures to achieve more precise access to data and memory control, multi-dimensional arrays (read-write, ordered), tuples (read-only, ordered), sets (Repetitive, disordered), dictionary (Key-Value), etc. In different packages, there are different expressions to define variables. For example, series is used to express lists in the pandas package, while array is used to express lists in the numpy package.
In comparison, Python's richer data structure will increase the learning cost, but it runs more accurately and faster.
Running speed
The running speed of R is relatively slow. In the regression of large samples, insufficient memory will occur if used improperly. Usually, big data needs to be converted into small data through the database (through groupby) before it can be handed over to R for analysis. Or combined with other big data processing tools, such as spark.
Although Python is not as fast as C, it is still very advantageous compared to R. It can directly process G data and is more accurate in very large data operations. a little better.
Help documentation and self-study costs
Compared with Python, which has a wider range of users, R’s help documentation is relatively undetailed and incomplete. The accompanying chestnut is also relatively concise, with some general explanations and usage.
However, Python's code statements, example displays, parameter analysis and other details are relatively complete. People who write help documents will more often provide a complete demo, so it is more friendly for self-taught people.
In addition, Python is a universal language. You can share notebooks with friends without them having to install anything. More importantly, you can bring people from different backgrounds together to be flexible. It has strong flexibility, good scalability, multi-functional work, and is very likely to generate more sparks of thinking.
Example
Text information mining is a common data processing and analysis usage scenario, such as e-commerce online shopping evaluation, social networking Website tags, sentiment analysis in news, etc.
When using R for sentiment analysis, you need to preprocess the data, remove useless symbols, and do word segmentation. Then build a word-document-label data set to create a document-term matrix, and then use various packages to perform machine learning algorithms.
Since the text of sentiment analysis is usually a very large-scale data, the processing speed in R is relatively slow, and multiple packages need to be used to collaborate.
When using Python for sentiment analysis, you first need to decompose the sentence into words, then perform feature extraction and remove stop words; then reduce the dimension, and then perform classification algorithm model training and model evaluation
Python's packages are highly integrated, especially for the problem of text mining sentiment analysis, which can make this operation faster and easier.
Time series analysis is a theory and method of establishing mathematical models through curve fitting and parameter estimation based on time series data obtained from system observations. It can be used in the financial field, weather forecasting, market analysis, etc.
When using R language for time series analysis, R has many packages that can be used to process regular and irregular time series, which is very advantageous, such as library(xts), library(timeSeires), library(zoo) —Time basic package, library (FinTS)--call the autoregressive test function, etc., and the results produced are also very intuitive and clear.
When using Python for timing analysis, there is no particularly complete timing analysis package, and there are no equations written specifically for prediction. Just like visualization, the operator needs to write more code by himself. Commonly used, statsmodels module, this module can be used for time series difference, modeling and model testing.
Have you felt it through the above two chestnuts!
There is no distinction between good and bad tools, it depends on the specific problem you want to solve.
The above is the detailed content of Detailed comparison between R language and python. For more information, please follow other related articles on the PHP Chinese website!

Hot AI Tools

Undresser.AI Undress
AI-powered app for creating realistic nude photos

AI Clothes Remover
Online AI tool for removing clothes from photos.

Undress AI Tool
Undress images for free

Clothoff.io
AI clothes remover

Video Face Swap
Swap faces in any video effortlessly with our completely free AI face swap tool!

Hot Article

Hot Tools

Notepad++7.3.1
Easy-to-use and free code editor

SublimeText3 Chinese version
Chinese version, very easy to use

Zend Studio 13.0.1
Powerful PHP integrated development environment

Dreamweaver CS6
Visual web development tools

SublimeText3 Mac version
God-level code editing software (SublimeText3)

Hot Topics


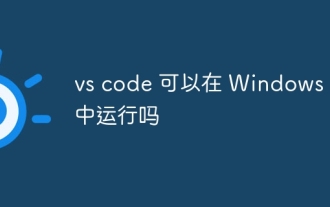
VS Code can run on Windows 8, but the experience may not be great. First make sure the system has been updated to the latest patch, then download the VS Code installation package that matches the system architecture and install it as prompted. After installation, be aware that some extensions may be incompatible with Windows 8 and need to look for alternative extensions or use newer Windows systems in a virtual machine. Install the necessary extensions to check whether they work properly. Although VS Code is feasible on Windows 8, it is recommended to upgrade to a newer Windows system for a better development experience and security.
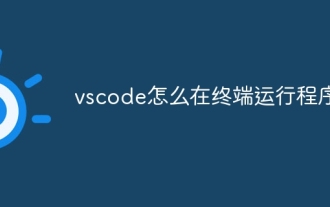
In VS Code, you can run the program in the terminal through the following steps: Prepare the code and open the integrated terminal to ensure that the code directory is consistent with the terminal working directory. Select the run command according to the programming language (such as Python's python your_file_name.py) to check whether it runs successfully and resolve errors. Use the debugger to improve debugging efficiency.
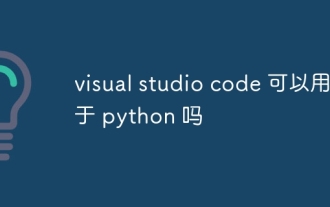
VS Code can be used to write Python and provides many features that make it an ideal tool for developing Python applications. It allows users to: install Python extensions to get functions such as code completion, syntax highlighting, and debugging. Use the debugger to track code step by step, find and fix errors. Integrate Git for version control. Use code formatting tools to maintain code consistency. Use the Linting tool to spot potential problems ahead of time.
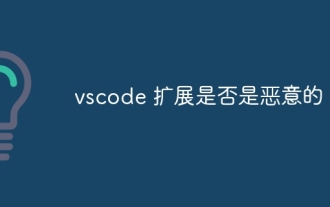
VS Code extensions pose malicious risks, such as hiding malicious code, exploiting vulnerabilities, and masturbating as legitimate extensions. Methods to identify malicious extensions include: checking publishers, reading comments, checking code, and installing with caution. Security measures also include: security awareness, good habits, regular updates and antivirus software.
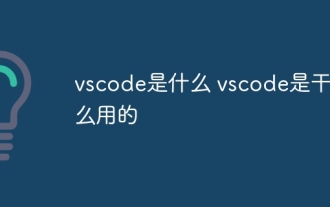
VS Code is the full name Visual Studio Code, which is a free and open source cross-platform code editor and development environment developed by Microsoft. It supports a wide range of programming languages and provides syntax highlighting, code automatic completion, code snippets and smart prompts to improve development efficiency. Through a rich extension ecosystem, users can add extensions to specific needs and languages, such as debuggers, code formatting tools, and Git integrations. VS Code also includes an intuitive debugger that helps quickly find and resolve bugs in your code.
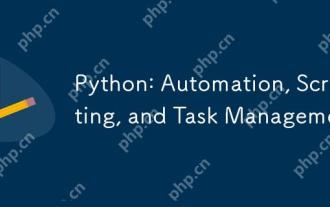
Python excels in automation, scripting, and task management. 1) Automation: File backup is realized through standard libraries such as os and shutil. 2) Script writing: Use the psutil library to monitor system resources. 3) Task management: Use the schedule library to schedule tasks. Python's ease of use and rich library support makes it the preferred tool in these areas.
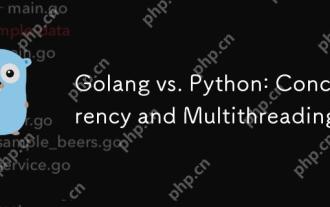
Golang is more suitable for high concurrency tasks, while Python has more advantages in flexibility. 1.Golang efficiently handles concurrency through goroutine and channel. 2. Python relies on threading and asyncio, which is affected by GIL, but provides multiple concurrency methods. The choice should be based on specific needs.
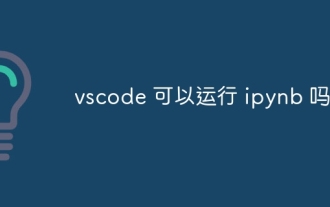
The key to running Jupyter Notebook in VS Code is to ensure that the Python environment is properly configured, understand that the code execution order is consistent with the cell order, and be aware of large files or external libraries that may affect performance. The code completion and debugging functions provided by VS Code can greatly improve coding efficiency and reduce errors.
