Using Python to process the KNN classification algorithm
【相关推荐:Python3视频教程 】
KNN分类算法的介绍
KNN分类算法(K-Nearest-Neighbors Classification),又叫K近邻算法,是一个概念极其简单,而分类效果又很优秀的分类算法。
他的核心思想就是,要确定测试样本属于哪一类,就寻找所有训练样本中与该测试样本“距离”最近的前K个样本,然后看这K个样本大部分属于哪一类,那么就认为这个测试样本也属于哪一类。简单的说就是让最相似的K个样本来投票决定。
这里所说的距离,一般最常用的就是多维空间的欧式距离。这里的维度指特征维度,即样本有几个特征就属于几维。
KNN示意图如下所示。(图片来源:百度百科)
上图中要确定测试样本绿色属于蓝色还是红色。
显然,当K=3时,将以1:2的投票结果分类于红色;而K=5时,将以3:2的投票结果分类于蓝色。
KNN算法简单有效,但没有优化的暴力法效率容易达到瓶颈。如样本个数为N,特征维度为D的时候,该算法时间复杂度呈O(DN)增长。
所以通常KNN的实现会把训练数据构建成K-D Tree(K-dimensional tree),构建过程很快,甚至不用计算D维欧氏距离,而搜索速度高达O(D*log(N))。
不过当D维度过高,会产生所谓的”维度灾难“,最终效率会降低到与暴力法一样。
因此通常D>20以后,最好使用更高效率的Ball-Tree,其时间复杂度为O(D*log(N))。
人们经过长期的实践发现KNN算法虽然简单,但能处理大规模的数据分类,尤其适用于样本分类边界不规则的情况。最重要的是该算法是很多高级机器学习算法的基础。
当然,KNN算法也存在一切问题。比如如果训练数据大部分都属于某一类,投票算法就有很大问题了。这时候就需要考虑设计每个投票者票的权重了。
测试数据
测试数据的格式仍然和前面使用的身高体重数据一致。不过数据稍微增加了一些
1.5 40 thin 1.5 50 fat 1.5 60 fat 1.6 40 thin 1.6 50 thin 1.6 60 fat 1.6 70 fat 1.7 50 thin 1.7 60 thin 1.7 70 fat 1.7 80 fat 1.8 60 thin 1.8 70 thin 1.8 80 fat 1.8 90 fat 1.9 80 thin 1.9 90 fat
Python代码实现
scikit-learn提供了优秀的KNN算法支持。
import numpy as np from sklearn import neighbors from sklearn.metrics import precision_recall_curve from sklearn.metrics import classification_report from sklearn.cross_validation import train_test_split import matplotlib.pyplot as plt ''' 数据读入 ''' data = [] labels = [] with open("data\\1.txt") as ifile: for line in ifile: tokens = line.strip().split(' ') data.append([float(tk) for tk in tokens[:-1]]) labels.append(tokens[-1]) x = np.array(data) labels = np.array(labels) y = np.zeros(labels.shape) ''' 标签转换为0/1 ''' y[labels=='fat']=1 ''' 拆分训练数据与测试数据 ''' x_train, x_test, y_train, y_test = train_test_split(x, y, test_size = 0.2) ''' 创建网格以方便绘制 ''' h = .01 x_min, x_max = x[:, 0].min() - 0.1, x[:, 0].max() + 0.1 y_min, y_max = x[:, 1].min() - 1, x[:, 1].max() + 1 xx, yy = np.meshgrid(np.arange(x_min, x_max, h), np.arange(y_min, y_max, h)) ''' 训练KNN分类器 ''' clf = neighbors.KNeighborsClassifier(algorithm='kd_tree') clf.fit(x_train, y_train) '''测试结果的打印''' answer = clf.predict(x) print(x) print(answer) print(y) print(np.mean( answer == y)) '''准确率与召回率''' precision, recall, thresholds = precision_recall_curve(y_train, clf.predict(x_train)) answer = clf.predict_proba(x)[:,1] print(classification_report(y, answer, target_names = ['thin', 'fat'])) ''' 将整个测试空间的分类结果用不同颜色区分开''' answer = clf.predict_proba(np.c_[xx.ravel(), yy.ravel()])[:,1] z = answer.reshape(xx.shape) plt.contourf(xx, yy, z, cmap=plt.cm.Paired, alpha=0.8) ''' 绘制训练样本 ''' plt.scatter(x_train[:, 0], x_train[:, 1], c=y_train, cmap=plt.cm.Paired) plt.xlabel(u'身高') plt.ylabel(u'体重') plt.show()
结果分析
输出结果:
[ 0. 0. 1. 0. 0. 1. 1. 0. 0. 1. 1. 0. 0. 1. 1. 0. 1.]
[ 0. 1. 1. 0. 0. 1. 1. 0. 0. 1. 1. 0. 0. 1. 1. 0. 1.]
准确率=0.94, score=0.94
precision recall f1-score support
thin 0.89 1.00 0.94 8
fat 1.00 0.89 0.94 9
avg / total 0.95 0.94 0.94 17
KNN分类器在众多分类算法中属于最简单的之一,需要注意的地方不多。有这几点要说明:
1、KNeighborsClassifier可以设置3种算法:‘brute',‘kd_tree',‘ball_tree'。如果不知道用哪个好,设置‘auto'让KNeighborsClassifier自己根据输入去决定。
2、注意统计准确率时,分类器的score返回的是计算正确的比例,而不是R2。R2一般应用于回归问题。
3、本例先根据样本中身高体重的最大最小值,生成了一个密集网格(步长h=0.01),然后将网格中的每一个点都当成测试样本去测试,最后使用contourf函数,使用不同的颜色标注出了胖、廋两类。
容易看到,本例的分类边界,属于相对复杂,但却又与距离呈现明显规则的锯齿形。
This kind of boundary linear function is difficult to handle. The KNN algorithm has inherent advantages in dealing with such boundary problems. We will see in the subsequent series that the accuracy rate = 0.94 for this data set is considered an excellent result.
【Related recommendations: Python3 video tutorial】
The above is the detailed content of Using Python to process the KNN classification algorithm. For more information, please follow other related articles on the PHP Chinese website!

Hot AI Tools

Undresser.AI Undress
AI-powered app for creating realistic nude photos

AI Clothes Remover
Online AI tool for removing clothes from photos.

Undress AI Tool
Undress images for free

Clothoff.io
AI clothes remover

Video Face Swap
Swap faces in any video effortlessly with our completely free AI face swap tool!

Hot Article

Hot Tools

Notepad++7.3.1
Easy-to-use and free code editor

SublimeText3 Chinese version
Chinese version, very easy to use

Zend Studio 13.0.1
Powerful PHP integrated development environment

Dreamweaver CS6
Visual web development tools

SublimeText3 Mac version
God-level code editing software (SublimeText3)

Hot Topics


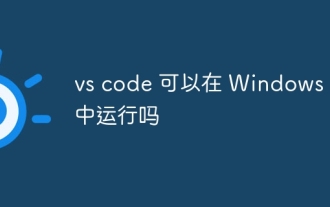
VS Code can run on Windows 8, but the experience may not be great. First make sure the system has been updated to the latest patch, then download the VS Code installation package that matches the system architecture and install it as prompted. After installation, be aware that some extensions may be incompatible with Windows 8 and need to look for alternative extensions or use newer Windows systems in a virtual machine. Install the necessary extensions to check whether they work properly. Although VS Code is feasible on Windows 8, it is recommended to upgrade to a newer Windows system for a better development experience and security.
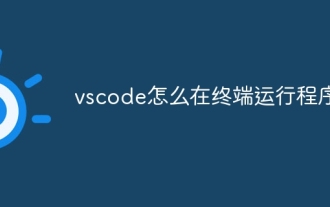
In VS Code, you can run the program in the terminal through the following steps: Prepare the code and open the integrated terminal to ensure that the code directory is consistent with the terminal working directory. Select the run command according to the programming language (such as Python's python your_file_name.py) to check whether it runs successfully and resolve errors. Use the debugger to improve debugging efficiency.
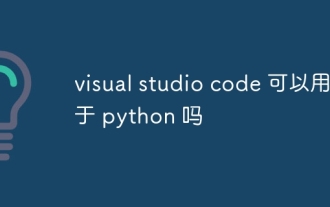
VS Code can be used to write Python and provides many features that make it an ideal tool for developing Python applications. It allows users to: install Python extensions to get functions such as code completion, syntax highlighting, and debugging. Use the debugger to track code step by step, find and fix errors. Integrate Git for version control. Use code formatting tools to maintain code consistency. Use the Linting tool to spot potential problems ahead of time.
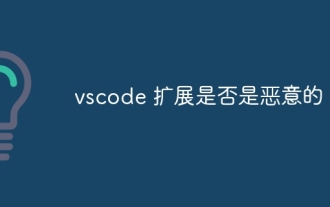
VS Code extensions pose malicious risks, such as hiding malicious code, exploiting vulnerabilities, and masturbating as legitimate extensions. Methods to identify malicious extensions include: checking publishers, reading comments, checking code, and installing with caution. Security measures also include: security awareness, good habits, regular updates and antivirus software.
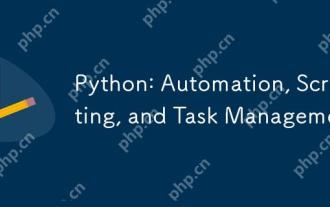
Python excels in automation, scripting, and task management. 1) Automation: File backup is realized through standard libraries such as os and shutil. 2) Script writing: Use the psutil library to monitor system resources. 3) Task management: Use the schedule library to schedule tasks. Python's ease of use and rich library support makes it the preferred tool in these areas.
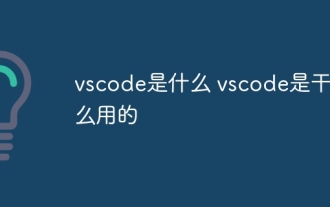
VS Code is the full name Visual Studio Code, which is a free and open source cross-platform code editor and development environment developed by Microsoft. It supports a wide range of programming languages and provides syntax highlighting, code automatic completion, code snippets and smart prompts to improve development efficiency. Through a rich extension ecosystem, users can add extensions to specific needs and languages, such as debuggers, code formatting tools, and Git integrations. VS Code also includes an intuitive debugger that helps quickly find and resolve bugs in your code.
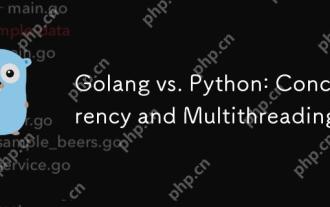
Golang is more suitable for high concurrency tasks, while Python has more advantages in flexibility. 1.Golang efficiently handles concurrency through goroutine and channel. 2. Python relies on threading and asyncio, which is affected by GIL, but provides multiple concurrency methods. The choice should be based on specific needs.
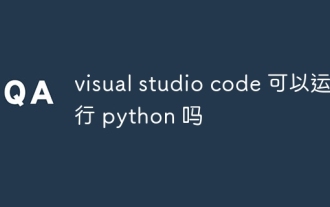
VS Code not only can run Python, but also provides powerful functions, including: automatically identifying Python files after installing Python extensions, providing functions such as code completion, syntax highlighting, and debugging. Relying on the installed Python environment, extensions act as bridge connection editing and Python environment. The debugging functions include setting breakpoints, step-by-step debugging, viewing variable values, and improving debugging efficiency. The integrated terminal supports running complex commands such as unit testing and package management. Supports extended configuration and enhances features such as code formatting, analysis and version control.
