


Is ChatGPT going to kill the data annotation industry? 20 times cheaper than humans and more accurate
Unexpectedly, the first group of people eliminated after the evolution of AI are the people who help train AI.
Many NLP applications require manual annotation of large amounts of data for a variety of tasks, especially training classifiers or evaluating the performance of unsupervised models. Depending on the scale and complexity, these tasks may be performed by crowdsourced workers on platforms such as MTurk as well as trained annotators such as research assistants.
We know that language large models (LLM) can "emerge" after reaching a certain scale - that is, they can acquire new capabilities that were previously unforeseen. As a large model that promotes a new outbreak of AI, ChatGPT’s capabilities in many tasks have exceeded people’s expectations, including labeling data sets and training yourself.
Recently, researchers from the University of Zurich have demonstrated that ChatGPT outperforms crowdsourcing work platforms and human work on multiple annotation tasks, including relevance, stance, topic and frame detection. assistant.
Additionally, the researchers did the math: ChatGPT costs less than $0.003 per annotation — roughly 20 times cheaper than MTurk. These results show the potential of large language models to greatly improve the efficiency of text classification.
Paper link: https://arxiv.org/abs/2303.15056
Research Details
Many NLP applications require high-quality annotated data, especially for training classifiers or evaluating the performance of unsupervised models. For example, researchers sometimes need to filter noisy social media data for relevance, assign texts to different topic or conceptual categories, or measure their emotional stance. Regardless of the specific method used for these tasks (supervised, semi-supervised, or unsupervised learning), accurately labeled data is required to build a training set or use it as a gold standard to evaluate performance.
The usual way people deal with this is to recruit research assistants or use crowdsourcing platforms like MTurk. When OpenAI built ChatGPT, it also subcontracted the problem of negative content to a Kenyan data annotation agency, and conducted a lot of annotation training before it was officially launched.
This report submitted by the University of Zurich in Switzerland explores the potential of large language models (LLM) in text annotation tasks, focusing on ChatGPT, released in November 2022. It proves that zero-shot (i.e. without any additional training) ChatGPT outperforms MTurk annotation on classification tasks at only a few tenths of the cost of manual labor.
The researchers used a sample of 2,382 tweets collected in a previous study. The tweets were labeled by trained annotators (research assistants) for five different tasks: relevance, stance, topic, and two frame detection. In the experiment, the researcher submitted the task to ChatGPT as a zero-shot classification and simultaneously to the crowdsourcing workers on MTurk, and then evaluated the performance of ChatGPT based on two benchmarks: relative to the accuracy of human workers on the crowdsourcing platform, and accuracy relative to research assistant annotators.
It was found that on four out of five tasks, ChatGPT had a higher zero-sample accuracy than MTurk. ChatGPT's encoder agreement exceeds that of MTurk and trained annotators for all tasks. Furthermore, in terms of cost, ChatGPT is much cheaper than MTurk: five classification tasks cost about $68 on ChatGPT (25264 annotations) and about $657 on MTurk (12632 annotations).
That puts ChatGPT’s cost per annotation at about $0.003, or one-third of a cent — about 20 times cheaper than MTurk, and with higher quality. Given this, it is now possible to annotate more samples or create large training sets for supervised learning. Based on existing tests, 100,000 annotations cost approximately $300.
The researchers say that while further research is needed to better understand how ChatGPT and other LLMs function in a broader context, these results suggest they have the potential to change the way researchers conduct The way data is annotated, and disrupts part of the business model of platforms like MTurk.
Experimental Process
The researchers used a dataset of 2382 tweets that were manually annotated from previous studies on tasks related to content moderation. Specifically, trained annotators (research assistants) constructed gold standards for five conceptual categories with varying numbers of categories: relevance of tweets to content moderation questions (relevant/irrelevant); regarding Article 230 ( position as part of the U.S. Communications Decency Act of 1996), a key part of U.S. Internet legislation; topic identification (six categories); Group 1 frameworks (content moderation as problem, solution, or neutral); and Section 1 Two sets of frameworks (fourteen categories).
The researchers then performed these exact same classifications using ChatGPT and crowdsourced workers recruited on MTurk. Four sets of annotations were made for ChatGPT. To explore the impact of the ChatGPT temperature parameter that controls the degree of randomness in the output, it is annotated here with the default values of 1 and 0.2, which imply less randomness. For each temperature value, the researchers performed two sets of annotations to calculate ChatGPT's encoder agreement.
For the experts, the study found two political science graduate students annotating tweets for all five tasks. For each task, coders were given the same set of instructions and were asked to independently annotate tweets on a task-by-task basis. To calculate the accuracy of ChatGPT and MTurk, the comparison only considered tweets that both trained annotators agreed upon.
For MTurk, the goal of the research is to select the best group of workers, specifically through screening those who are classified by Amazon as "MTurk Masters", have more than 90% positive reviews, and work in the United States By.
This study uses the "gpt-3.5-turbo" version of the ChatGPT API to classify tweets. Annotation took place between March 9 and March 20, 2023. For each annotation task, the researchers intentionally avoided adding any ChatGPT-specific prompts such as “let’s think step by step” to ensure comparability between ChatGPT and MTurk crowdworkers.
After testing several variations, people decided to feed tweets to ChatGPT one by one with a prompt like this: "This is the tweet I selected, please mark it for [task-specific instructions (e.g., one of the topics in the instructions)]. Additionally, four ChatGPT responses were collected per tweet in this study, and a new chat session was also created for each tweet to ensure ChatGPT results Not affected by annotation history.
Figure 1. ChatGPT zero compared to high-scoring annotators on MTurk -shot's text annotation capabilities. ChatGPT has better accuracy than MTurk in four of the five tasks.
In the above figure, ChatGPT has the advantage Among the four tasks, ChatGPT has a slight advantage in one case (relevance), but its performance is very similar to MTurk. In the other three cases (frams I, frams II, and Stance), ChatGPT outperforms MTurk by 2.2 to 3.4 times. Furthermore, considering the difficulty of the task, the number of classes, and the fact that the annotations are zero-sample, the accuracy of ChatGPT is generally more than adequate.
For correlation, there are two For categories (relevant/irrelevant), ChatGPT has an accuracy of 72.8%, while for stance, there are three categories (positive/negative/neutral) with an accuracy of 78.7%. As the number of categories increases, the accuracy decreases, Although the inherent difficulty of the task also has an impact. Regarding the encoder protocol, Figure 1 shows that the performance of ChatGPT is very high, exceeding 95% for all tasks when the temperature parameter is set to 0.2. These values are higher than any human, including those trained on annotator. Even using the default temperature value of 1 (which means more randomness), the inter-coder agreement is always over 84%. The relationship between inter-coder agreement and accuracy is positive but weak ( Pearson correlation coefficient: 0.17). Although the correlation is based on only five data points, it suggests that lower temperature values may be more suitable for the annotation task, as it appears to improve the consistency of the results without significantly reducing accuracy.
It must be emphasized that testing ChatGPT is very difficult. Content moderation is a complex topic that requires significant resources. In addition to positions, researchers have developed concepts for specific research purposes categories. In addition, some tasks involve a large number of categories, yet ChatGPT still achieves high accuracy.
Using models to annotate data is nothing new. In computer science research using large-scale data sets, people often label a small number of samples and then amplify them with machine learning. However, after outperforming humans, we may be able to trust the judgments from ChatGPT more in the future.
The above is the detailed content of Is ChatGPT going to kill the data annotation industry? 20 times cheaper than humans and more accurate. For more information, please follow other related articles on the PHP Chinese website!

Hot AI Tools

Undresser.AI Undress
AI-powered app for creating realistic nude photos

AI Clothes Remover
Online AI tool for removing clothes from photos.

Undress AI Tool
Undress images for free

Clothoff.io
AI clothes remover

AI Hentai Generator
Generate AI Hentai for free.

Hot Article

Hot Tools

Notepad++7.3.1
Easy-to-use and free code editor

SublimeText3 Chinese version
Chinese version, very easy to use

Zend Studio 13.0.1
Powerful PHP integrated development environment

Dreamweaver CS6
Visual web development tools

SublimeText3 Mac version
God-level code editing software (SublimeText3)

Hot Topics


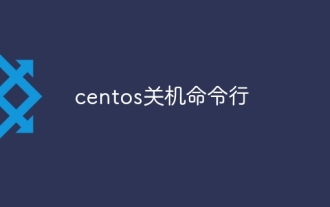
The CentOS shutdown command is shutdown, and the syntax is shutdown [Options] Time [Information]. Options include: -h Stop the system immediately; -P Turn off the power after shutdown; -r restart; -t Waiting time. Times can be specified as immediate (now), minutes ( minutes), or a specific time (hh:mm). Added information can be displayed in system messages.
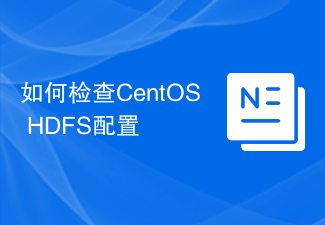
Complete Guide to Checking HDFS Configuration in CentOS Systems This article will guide you how to effectively check the configuration and running status of HDFS on CentOS systems. The following steps will help you fully understand the setup and operation of HDFS. Verify Hadoop environment variable: First, make sure the Hadoop environment variable is set correctly. In the terminal, execute the following command to verify that Hadoop is installed and configured correctly: hadoopversion Check HDFS configuration file: The core configuration file of HDFS is located in the /etc/hadoop/conf/ directory, where core-site.xml and hdfs-site.xml are crucial. use
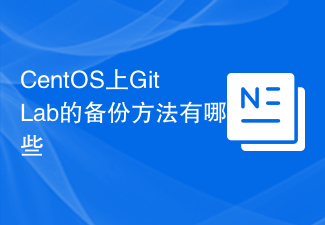
Backup and Recovery Policy of GitLab under CentOS System In order to ensure data security and recoverability, GitLab on CentOS provides a variety of backup methods. This article will introduce several common backup methods, configuration parameters and recovery processes in detail to help you establish a complete GitLab backup and recovery strategy. 1. Manual backup Use the gitlab-rakegitlab:backup:create command to execute manual backup. This command backs up key information such as GitLab repository, database, users, user groups, keys, and permissions. The default backup file is stored in the /var/opt/gitlab/backups directory. You can modify /etc/gitlab
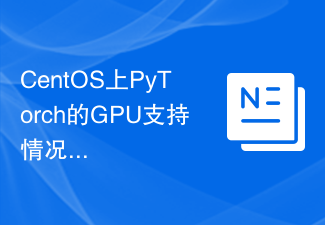
Enable PyTorch GPU acceleration on CentOS system requires the installation of CUDA, cuDNN and GPU versions of PyTorch. The following steps will guide you through the process: CUDA and cuDNN installation determine CUDA version compatibility: Use the nvidia-smi command to view the CUDA version supported by your NVIDIA graphics card. For example, your MX450 graphics card may support CUDA11.1 or higher. Download and install CUDAToolkit: Visit the official website of NVIDIACUDAToolkit and download and install the corresponding version according to the highest CUDA version supported by your graphics card. Install cuDNN library:
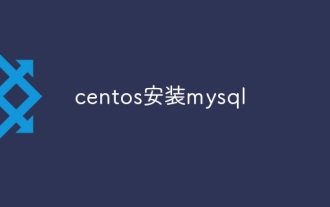
Installing MySQL on CentOS involves the following steps: Adding the appropriate MySQL yum source. Execute the yum install mysql-server command to install the MySQL server. Use the mysql_secure_installation command to make security settings, such as setting the root user password. Customize the MySQL configuration file as needed. Tune MySQL parameters and optimize databases for performance.
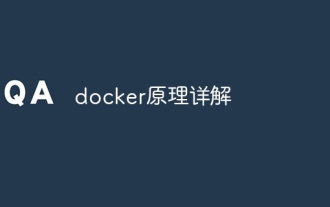
Docker uses Linux kernel features to provide an efficient and isolated application running environment. Its working principle is as follows: 1. The mirror is used as a read-only template, which contains everything you need to run the application; 2. The Union File System (UnionFS) stacks multiple file systems, only storing the differences, saving space and speeding up; 3. The daemon manages the mirrors and containers, and the client uses them for interaction; 4. Namespaces and cgroups implement container isolation and resource limitations; 5. Multiple network modes support container interconnection. Only by understanding these core concepts can you better utilize Docker.
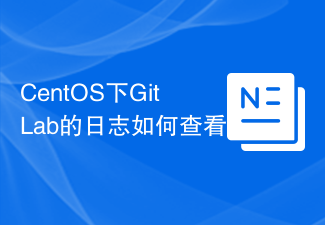
A complete guide to viewing GitLab logs under CentOS system This article will guide you how to view various GitLab logs in CentOS system, including main logs, exception logs, and other related logs. Please note that the log file path may vary depending on the GitLab version and installation method. If the following path does not exist, please check the GitLab installation directory and configuration files. 1. View the main GitLab log Use the following command to view the main log file of the GitLabRails application: Command: sudocat/var/log/gitlab/gitlab-rails/production.log This command will display product
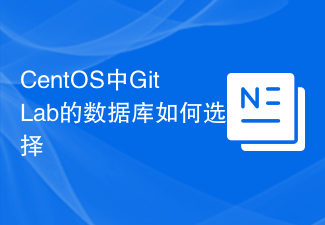
When installing and configuring GitLab on a CentOS system, the choice of database is crucial. GitLab is compatible with multiple databases, but PostgreSQL and MySQL (or MariaDB) are most commonly used. This article analyzes database selection factors and provides detailed installation and configuration steps. Database Selection Guide When choosing a database, you need to consider the following factors: PostgreSQL: GitLab's default database is powerful, has high scalability, supports complex queries and transaction processing, and is suitable for large application scenarios. MySQL/MariaDB: a popular relational database widely used in Web applications, with stable and reliable performance. MongoDB:NoSQL database, specializes in
