The environmental costs and promises of artificial intelligence
Artificial intelligence (AI) often appears in two extreme forms in popular culture and political analysis. It could either represent the key to a futuristic utopia where human intelligence meets technological prowess, or it could be the first step toward a dystopian rise of machines. Academics, entrepreneurs, and even activists are employing the same binary thinking when applying artificial intelligence to combat climate change.
The tech industry’s singular focus on the role of AI in creating a new technological utopia obscures the ways in which AI may exacerbate environmental degradation, often directly Ways to hurt marginalized people. To make the most of AI technology in the fight against climate change, while acknowledging its massive energy consumption, technology companies leading the AI trend need to explore solutions to AI’s environmental impact.
Artificial intelligence can be a powerful tool in the fight against climate change. For example, AI self-driving cars could reduce emissions by 50% by 2050 by determining the most efficient routes. The use of AI in agriculture results in higher yields; groundnut farmers in India achieved a 30% increase in income by using AI technology.
In addition, artificial intelligence can provide faster and more accurate analysis of satellite images, identifying disaster-hit areas that require assistance or rainforest damage. AI-driven data analytics can also help predict dangerous weather patterns and improve accountability by accurately monitoring whether governments and companies are complying with their emissions targets.
However, artificial intelligence and the wider internet and communications industries are increasingly under fire for using too much energy. Take data processing as an example. The supercomputers used to run cutting-edge artificial intelligence programs are powered by the public grid and backed by backup diesel generators. Training a single AI system can emit more than 250,000 pounds of carbon dioxide.
In fact, the use of AI technology across all sectors produces as much CO2 emissions as the aviation industry. These additional emissions disproportionately affect historically marginalized communities, which tend to live in highly polluted areas and are more vulnerable to the direct impacts of pollution’s health hazards.
Recently, AI scientists and engineers have responded to these criticisms and are considering new sources to power data farms. Yet even new, ostensibly more sustainable energy sources, such as rechargeable batteries, can exacerbate climate change and harm communities. Most rechargeable batteries are made using lithium, a rare earth metal whose extraction can negatively impact marginalized communities. Due to the growing demand for clean energy, lithium extraction requires large amounts of water, consuming 500,000 gallons of water for each ton of lithium extracted.
In Chile, the world’s second-largest lithium producer, indigenous communities such as the Copiapó in the north often clash with mining companies over land and water rights. These mining activities are very water-intensive, with the Energy Institute reporting that in the Salar de Atacama they consume 65 percent of the region’s water. This water loss damages and permanently depletes wetlands and water sources, drives native plant and animal species to the brink of extinction, and impacts local populations. Describing lithium as a “clean” energy source simply because it causes less harm to the environment than diesel or coal is a false dichotomy that discourages stakeholders from pursuing newer, greener energy sources.
The development of artificial intelligence technology is a symbol of incredible progress; however, progress is not one-size-fits-all, and the companies developing these technologies have a responsibility to ensure that marginalized communities do not bear the brunt of the negative impacts of the artificial intelligence revolution.
Some data farms have switched entirely to clean energy. For example, data farms in Iceland run largely on clean energy provided by the island’s hydroelectric and geothermal resources, and the country has become a popular location for new data centers. These data centers also don’t need energy-intensive fans or air conditioners to cool them—Iceland’s cold climate will do just fine. However, Iceland is uniquely suited to host a data processing center and most countries cannot replicate this unique environmental condition.
Big data companies can avoid the pitfalls of lithium batteries by using physical batteries. Made from concrete, these batteries store gravitational potential energy in elevated concrete blocks, which can then be harnessed at any time. It's not a far-fetched idea - two 35-ton concrete blocks are suspended by a 246-foot tower in a Swiss valley. These are early prototypes of what physical batteries will look like, and together they hold enough energy to power two thousand homes (2 megawatts). Physical batteries are a potential alternative to lithium batteries, are less costly to the environment and marginalized communities, and can be made from commonly used materials such as concrete.
The U.S. government, through the Department of Energy and the Defense Advanced Research Projects Agency (DARPA), has invested billions of dollars in improving lithium batteries, specifically by making solid-state lithium-ion batteries that are much more durable than traditional lithium-ion batteries. Batteries can offer better safety, energy density and longevity. Some private companies have pledged to expand the use of lithium-ion technology in their facilities, including Google, which created a pilot program to phase out diesel generators in some data centers and replace them with lithium-ion batteries. These investments are insufficient, especially as electric carmakers and the U.S. government invest billions in new batteries. Tech companies need to do more to help solve the energy use and storage issues posed by AI.
Artificial intelligence offers many advantages for solving the current climate crisis, but the potential environmental side effects cannot be ignored. Tech companies are often praised for their creativity and ingenuity, the skills they need to apply to solve problems related to artificial intelligence.
The above is the detailed content of The environmental costs and promises of artificial intelligence. For more information, please follow other related articles on the PHP Chinese website!

Hot AI Tools

Undresser.AI Undress
AI-powered app for creating realistic nude photos

AI Clothes Remover
Online AI tool for removing clothes from photos.

Undress AI Tool
Undress images for free

Clothoff.io
AI clothes remover

AI Hentai Generator
Generate AI Hentai for free.

Hot Article

Hot Tools

Notepad++7.3.1
Easy-to-use and free code editor

SublimeText3 Chinese version
Chinese version, very easy to use

Zend Studio 13.0.1
Powerful PHP integrated development environment

Dreamweaver CS6
Visual web development tools

SublimeText3 Mac version
God-level code editing software (SublimeText3)

Hot Topics


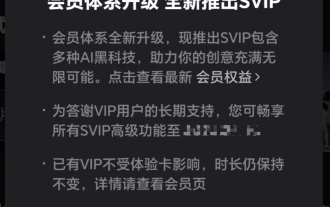
This site reported on June 27 that Jianying is a video editing software developed by FaceMeng Technology, a subsidiary of ByteDance. It relies on the Douyin platform and basically produces short video content for users of the platform. It is compatible with iOS, Android, and Windows. , MacOS and other operating systems. Jianying officially announced the upgrade of its membership system and launched a new SVIP, which includes a variety of AI black technologies, such as intelligent translation, intelligent highlighting, intelligent packaging, digital human synthesis, etc. In terms of price, the monthly fee for clipping SVIP is 79 yuan, the annual fee is 599 yuan (note on this site: equivalent to 49.9 yuan per month), the continuous monthly subscription is 59 yuan per month, and the continuous annual subscription is 499 yuan per year (equivalent to 41.6 yuan per month) . In addition, the cut official also stated that in order to improve the user experience, those who have subscribed to the original VIP
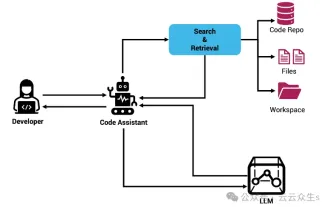
Improve developer productivity, efficiency, and accuracy by incorporating retrieval-enhanced generation and semantic memory into AI coding assistants. Translated from EnhancingAICodingAssistantswithContextUsingRAGandSEM-RAG, author JanakiramMSV. While basic AI programming assistants are naturally helpful, they often fail to provide the most relevant and correct code suggestions because they rely on a general understanding of the software language and the most common patterns of writing software. The code generated by these coding assistants is suitable for solving the problems they are responsible for solving, but often does not conform to the coding standards, conventions and styles of the individual teams. This often results in suggestions that need to be modified or refined in order for the code to be accepted into the application
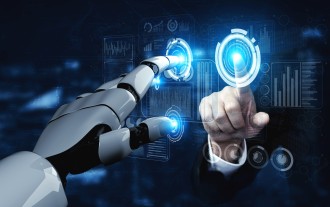
Large Language Models (LLMs) are trained on huge text databases, where they acquire large amounts of real-world knowledge. This knowledge is embedded into their parameters and can then be used when needed. The knowledge of these models is "reified" at the end of training. At the end of pre-training, the model actually stops learning. Align or fine-tune the model to learn how to leverage this knowledge and respond more naturally to user questions. But sometimes model knowledge is not enough, and although the model can access external content through RAG, it is considered beneficial to adapt the model to new domains through fine-tuning. This fine-tuning is performed using input from human annotators or other LLM creations, where the model encounters additional real-world knowledge and integrates it

To learn more about AIGC, please visit: 51CTOAI.x Community https://www.51cto.com/aigc/Translator|Jingyan Reviewer|Chonglou is different from the traditional question bank that can be seen everywhere on the Internet. These questions It requires thinking outside the box. Large Language Models (LLMs) are increasingly important in the fields of data science, generative artificial intelligence (GenAI), and artificial intelligence. These complex algorithms enhance human skills and drive efficiency and innovation in many industries, becoming the key for companies to remain competitive. LLM has a wide range of applications. It can be used in fields such as natural language processing, text generation, speech recognition and recommendation systems. By learning from large amounts of data, LLM is able to generate text
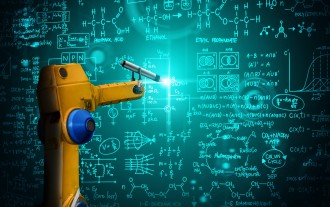
Machine learning is an important branch of artificial intelligence that gives computers the ability to learn from data and improve their capabilities without being explicitly programmed. Machine learning has a wide range of applications in various fields, from image recognition and natural language processing to recommendation systems and fraud detection, and it is changing the way we live. There are many different methods and theories in the field of machine learning, among which the five most influential methods are called the "Five Schools of Machine Learning". The five major schools are the symbolic school, the connectionist school, the evolutionary school, the Bayesian school and the analogy school. 1. Symbolism, also known as symbolism, emphasizes the use of symbols for logical reasoning and expression of knowledge. This school of thought believes that learning is a process of reverse deduction, through existing
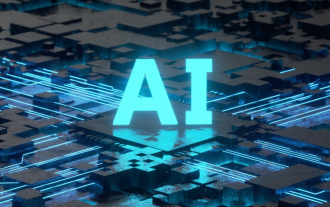
Editor |ScienceAI Question Answering (QA) data set plays a vital role in promoting natural language processing (NLP) research. High-quality QA data sets can not only be used to fine-tune models, but also effectively evaluate the capabilities of large language models (LLM), especially the ability to understand and reason about scientific knowledge. Although there are currently many scientific QA data sets covering medicine, chemistry, biology and other fields, these data sets still have some shortcomings. First, the data form is relatively simple, most of which are multiple-choice questions. They are easy to evaluate, but limit the model's answer selection range and cannot fully test the model's ability to answer scientific questions. In contrast, open-ended Q&A
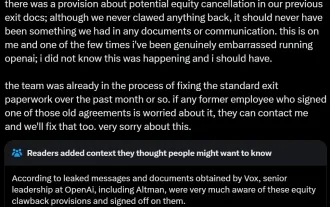
Last week, amid the internal wave of resignations and external criticism, OpenAI was plagued by internal and external troubles: - The infringement of the widow sister sparked global heated discussions - Employees signing "overlord clauses" were exposed one after another - Netizens listed Ultraman's "seven deadly sins" Rumors refuting: According to leaked information and documents obtained by Vox, OpenAI’s senior leadership, including Altman, was well aware of these equity recovery provisions and signed off on them. In addition, there is a serious and urgent issue facing OpenAI - AI safety. The recent departures of five security-related employees, including two of its most prominent employees, and the dissolution of the "Super Alignment" team have once again put OpenAI's security issues in the spotlight. Fortune magazine reported that OpenA
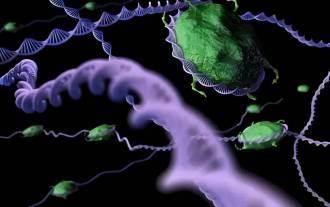
Editor | KX In the field of drug research and development, accurately and effectively predicting the binding affinity of proteins and ligands is crucial for drug screening and optimization. However, current studies do not take into account the important role of molecular surface information in protein-ligand interactions. Based on this, researchers from Xiamen University proposed a novel multi-modal feature extraction (MFE) framework, which for the first time combines information on protein surface, 3D structure and sequence, and uses a cross-attention mechanism to compare different modalities. feature alignment. Experimental results demonstrate that this method achieves state-of-the-art performance in predicting protein-ligand binding affinities. Furthermore, ablation studies demonstrate the effectiveness and necessity of protein surface information and multimodal feature alignment within this framework. Related research begins with "S
