Using machine learning to reconstruct faces in videos
Translator|Cui Hao
Reviser|Sun Shujuan
Opening Chapter
The workflow of the new system will take into account occlusion situations, such as when an object moves away from the line of sight. This is also one of the biggest challenges for deepfake software, as FAN landmarks can barely account for these situations and their translation quality tends to degrade as faces are avoided or occluded.
The new system avoids the above problems by defining "contour energy" that matches the boundaries of 3D faces (3DMM) and 2D faces (defined by FAN landmarks).
Optimization
The application scenario of this system is real-time deformation, such as real-time transformation of face shape in a video chat filter. Currently, frameworks cannot achieve this, so providing the necessary computing resources to enable "real-time" deformation becomes a significant challenge.
According to the assumptions of the paper, the latency of each frame operation of the 24fps video relative to the material per second in the pipeline is 16.344 seconds. At the same time, for feature estimation and 3D facial deformation, it is also accompanied by one hit (respectively 321 ms and 160 ms).
As a result, optimization has made key progress in reducing latency. Since joint optimization across all frames would significantly increase system overhead, and optimization of the initialization style (assuming consistent speaker characteristics throughout) may lead to anomalies, the authors adopted a sparse mode to calculate coefficients at realistic intervals of sampled frames.
Joint optimization is then performed on this subset of frames, resulting in a leaner reconstruction process.
Facial Surface
The morphing technology used in this project is an adaptation of the author’s 2020 work Deep Shapely Portraits (DSP).
Deep Shapely Portraits, 2020 submission to ACM Multimedia. The paper was led by researchers from the Zhejiang University-Tencent Joint Laboratory for Game and Intelligent Graphics Innovation Technology
The authors observed that “we extend this method from reshaping a single image to reshaping an entire image sequence.”
Testing
The paper points out that there is no comparable historical data to evaluate the new method. Therefore, the authors compared their curved video output frames with static DSP output.
Testing the new system against static images from Deep Shapely Portraits
The author pointed out that due to the use of sparse mapping, the DSP method will have traces of artificial modification— —The new framework solves this problem through dense mapping. Furthermore, the paper argues that videos produced by DSP lack smoothness and visual coherence.
The authors pointed out:
“The results show that our method can stably and coherently generate reshaped portrait videos, while image-based methods can easily lead to obvious flickering artifacts (artificial Traces of modification)."
Translator introduction
Cui Hao, 51CTO community editor, senior architect, has 18 years of software development and architecture experience, and 10 years of distributed architecture experience. Formerly a technical expert at HP. He is willing to share and has written many popular technical articles with more than 600,000 reads. Author of "Principles and Practice of Distributed Architecture".
Original title: Restructuring Faces in Videos With Machine Learning, author: Martin Anderson
The above is the detailed content of Using machine learning to reconstruct faces in videos. For more information, please follow other related articles on the PHP Chinese website!

Hot AI Tools

Undresser.AI Undress
AI-powered app for creating realistic nude photos

AI Clothes Remover
Online AI tool for removing clothes from photos.

Undress AI Tool
Undress images for free

Clothoff.io
AI clothes remover

Video Face Swap
Swap faces in any video effortlessly with our completely free AI face swap tool!

Hot Article

Hot Tools

Notepad++7.3.1
Easy-to-use and free code editor

SublimeText3 Chinese version
Chinese version, very easy to use

Zend Studio 13.0.1
Powerful PHP integrated development environment

Dreamweaver CS6
Visual web development tools

SublimeText3 Mac version
God-level code editing software (SublimeText3)

Hot Topics










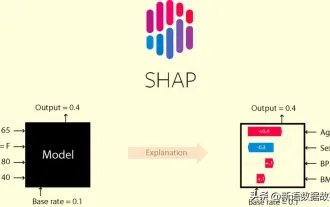
In the fields of machine learning and data science, model interpretability has always been a focus of researchers and practitioners. With the widespread application of complex models such as deep learning and ensemble methods, understanding the model's decision-making process has become particularly important. Explainable AI|XAI helps build trust and confidence in machine learning models by increasing the transparency of the model. Improving model transparency can be achieved through methods such as the widespread use of multiple complex models, as well as the decision-making processes used to explain the models. These methods include feature importance analysis, model prediction interval estimation, local interpretability algorithms, etc. Feature importance analysis can explain the decision-making process of a model by evaluating the degree of influence of the model on the input features. Model prediction interval estimate
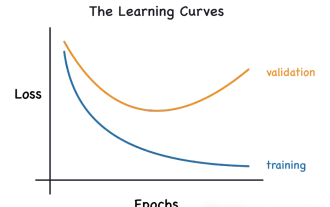
This article will introduce how to effectively identify overfitting and underfitting in machine learning models through learning curves. Underfitting and overfitting 1. Overfitting If a model is overtrained on the data so that it learns noise from it, then the model is said to be overfitting. An overfitted model learns every example so perfectly that it will misclassify an unseen/new example. For an overfitted model, we will get a perfect/near-perfect training set score and a terrible validation set/test score. Slightly modified: "Cause of overfitting: Use a complex model to solve a simple problem and extract noise from the data. Because a small data set as a training set may not represent the correct representation of all data." 2. Underfitting Heru
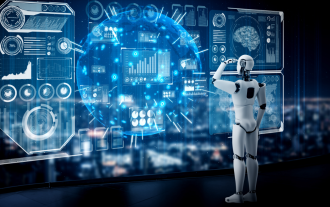
In the 1950s, artificial intelligence (AI) was born. That's when researchers discovered that machines could perform human-like tasks, such as thinking. Later, in the 1960s, the U.S. Department of Defense funded artificial intelligence and established laboratories for further development. Researchers are finding applications for artificial intelligence in many areas, such as space exploration and survival in extreme environments. Space exploration is the study of the universe, which covers the entire universe beyond the earth. Space is classified as an extreme environment because its conditions are different from those on Earth. To survive in space, many factors must be considered and precautions must be taken. Scientists and researchers believe that exploring space and understanding the current state of everything can help understand how the universe works and prepare for potential environmental crises
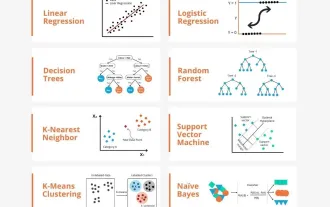
In layman’s terms, a machine learning model is a mathematical function that maps input data to a predicted output. More specifically, a machine learning model is a mathematical function that adjusts model parameters by learning from training data to minimize the error between the predicted output and the true label. There are many models in machine learning, such as logistic regression models, decision tree models, support vector machine models, etc. Each model has its applicable data types and problem types. At the same time, there are many commonalities between different models, or there is a hidden path for model evolution. Taking the connectionist perceptron as an example, by increasing the number of hidden layers of the perceptron, we can transform it into a deep neural network. If a kernel function is added to the perceptron, it can be converted into an SVM. this one
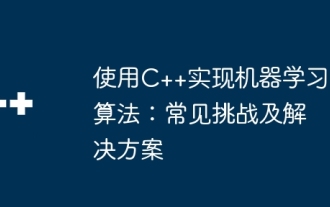
Common challenges faced by machine learning algorithms in C++ include memory management, multi-threading, performance optimization, and maintainability. Solutions include using smart pointers, modern threading libraries, SIMD instructions and third-party libraries, as well as following coding style guidelines and using automation tools. Practical cases show how to use the Eigen library to implement linear regression algorithms, effectively manage memory and use high-performance matrix operations.
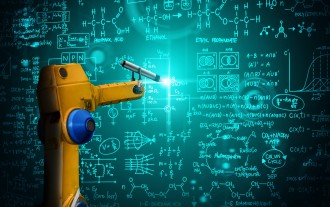
Machine learning is an important branch of artificial intelligence that gives computers the ability to learn from data and improve their capabilities without being explicitly programmed. Machine learning has a wide range of applications in various fields, from image recognition and natural language processing to recommendation systems and fraud detection, and it is changing the way we live. There are many different methods and theories in the field of machine learning, among which the five most influential methods are called the "Five Schools of Machine Learning". The five major schools are the symbolic school, the connectionist school, the evolutionary school, the Bayesian school and the analogy school. 1. Symbolism, also known as symbolism, emphasizes the use of symbols for logical reasoning and expression of knowledge. This school of thought believes that learning is a process of reverse deduction, through existing
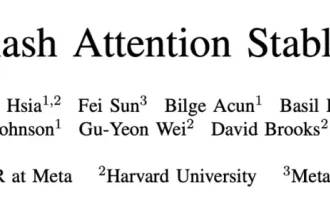
MetaFAIR teamed up with Harvard to provide a new research framework for optimizing the data bias generated when large-scale machine learning is performed. It is known that the training of large language models often takes months and uses hundreds or even thousands of GPUs. Taking the LLaMA270B model as an example, its training requires a total of 1,720,320 GPU hours. Training large models presents unique systemic challenges due to the scale and complexity of these workloads. Recently, many institutions have reported instability in the training process when training SOTA generative AI models. They usually appear in the form of loss spikes. For example, Google's PaLM model experienced up to 20 loss spikes during the training process. Numerical bias is the root cause of this training inaccuracy,
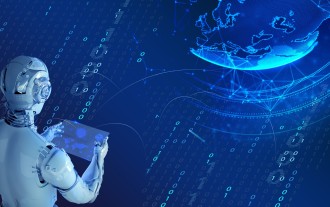
Translator | Reviewed by Li Rui | Chonglou Artificial intelligence (AI) and machine learning (ML) models are becoming increasingly complex today, and the output produced by these models is a black box – unable to be explained to stakeholders. Explainable AI (XAI) aims to solve this problem by enabling stakeholders to understand how these models work, ensuring they understand how these models actually make decisions, and ensuring transparency in AI systems, Trust and accountability to address this issue. This article explores various explainable artificial intelligence (XAI) techniques to illustrate their underlying principles. Several reasons why explainable AI is crucial Trust and transparency: For AI systems to be widely accepted and trusted, users need to understand how decisions are made
