


OpenMLDB R&D leader Lu Mian, Fourth Paradigm system architect: Open source machine learning database OpenMLDB: a production-level feature platform that is consistent online and offline
Guest: Lu Mian
Organization: Mo Se
The speech content is now organized as follows, hoping to inspire you. Data and Feature Challenges for the Implementation of Artificial Intelligence Engineering Today, according to statistics, 95% of the time in the implementation of artificial intelligence is spent on data. Although there are various data tools such as MySQL on the market, they are far from solving the problem of artificial intelligence implementation. So, let’s first look at the data issues. If you have participated in some machine learning application development, you should be deeply impressed by MLOps, as shown in the following figure:For example, as shown in the figure below, a real-time recommendation system that meets the production-level online requirements is built. User Xiao Li performs a search with the keyword "washing machine". He needs to put the original request data as well as users, products, and transactions in the system. The information data are combined for real-time feature calculation, and then some more meaningful features are generated, which is the so-called feature engineering, the process of generating features. For example, the system will generate "the top three best-selling washing machines purchased by customers of a certain age group in the past three months." This type of feature does not require strong timeliness and is calculated based on longer historical data. However, the system may also need some highly time-sensitive data, such as "browsing records within the past hour/half hour", etc. After the system obtains the newly calculated features, it will provide the model for inference. There are two main requirements for such a system feature platform. One is correctness, that is, the consistency of online and offline feature calculations; the other is efficiency, that is, real-time feature calculation, delay
Full life cycle from feature calculation development to launch
Before the OpenMLDB methodology, we mainly used the process shown in the figure below for feature calculation development.
#First we need to create a scenario where data scientists will use Python/SparkSQL tools for offline feature extraction. The KPI of data scientists is to build a business requirement model that meets the accuracy. When the model quality reaches the standard, the task is completed. The engineering challenges faced by feature scripts after they go online, such as low latency, high concurrency, and high availability, are not within the jurisdiction of scientists.
##In order to put the Python script written by the data scientist online, the engineering team needs to intervene. All they have to do is to The offline scripts created by scientists were reconstructed and optimized, and C/Database was used for real-time feature extraction services. This meets a series of engineering requirements for low latency, high concurrency, and high availability, allowing feature scripts to truly go online for online services.
#This process is very expensive and requires the intervention of two sets of skill teams, and they use different tools. After the two sets of processes are completed, the consistency of the calculation logic needs to be checked. That is, the calculation logic of the feature script developed by the data scientist must be completely consistent with the logic of the final real-time feature extraction. This requirement seems clear and simple, but it will introduce a lot of communication costs, testing costs, and iterative development costs during the consistency verification process. According to past experience, the larger the project, the longer the consistency verification will take and the cost will be very high.
Generally speaking, the main reason for the inconsistency between online and offline during the consistency verification process is that the development tools are inconsistent. For example, scientists use Python, and engineering teams A database is used, and differences in tool capabilities may lead to functional compromises and inconsistencies; there are also gaps in the definition of data, algorithms, and cognition.
#In short, the cost of development based on the traditional two sets of processes is very high, requiring two sets of developers from different skill stations and the development and operation of two sets of systems. It is also necessary to add stacked verification, verification, etc.
#And OpenMLDB provides a low-cost open source solution.
OpenMLDB: A production-level feature calculation platform that is consistent online and offline
In June last year, OpenMLDB was officially open sourced and is a young player in the open source community. project, but has been implemented in more than 100 scenarios, covering more than 300 nodes.
OpenMLDB is an open source machine learning database. Its main function is to provide a consistent online and offline feature platform. So how does OpenMLDB meet the needs of high performance and correctness?
As shown in the figure above, first of all, the only programming language used by OpenMLDB is SQL. There are no longer two sets of tool chains. Both data scientists and developers use SQL to express features.
Secondly, two sets of engines are separated within OpenMLDB. One is the "batch SQL engine", which performs source code level optimization based on Spark, provides a higher-performance computing method, and makes syntax expansion. ; The other set is the "real-time SQL engine", which is a resource time series database self-developed by our team. The default is a time series database based on a memory storage engine. Based on the "real-time SQL engine", we can achieve online efficient millisecond-level real-time calculations, while also ensuring high availability, low latency, and high concurrency.
There is also an important "consistency execution plan generator" between these two engines, which aims to ensure the consistency of online and offline execution plan logic. With it, online and offline consistency can be naturally guaranteed without the need for manual proofreading.
In short, based on this architecture, our ultimate goal is to achieve the optimization goal of "development and online", which mainly includes three steps: offline SQL feature script development; one-click deployment and online ; Access real-time request data stream.
It can be seen that compared with the previous two sets of processes, two sets of tool chains, and two sets of developer investment, the biggest advantage of this set of engines is that it saves a lot of engineering costs. , that is, as long as data scientists use SQL to develop feature scripts, they no longer need the engineering team to do a second round of optimization, and they can go online directly. There is no need for intermediate manual operations of online and offline consistency verification, which saves a lot of time. and cost.
The following figure shows the complete process of OpenMLDB from offline development to online service:
Overall, OpenMLDB solves a core problem - online and offline consistency of machine learning; and provides a core feature - millisecond-level real-time feature calculation. These two points are the core values provided by OpenMLDB.
Because OpenMLDB has two sets of engines, online and offline, the application methods are also different. The following figure shows our recommended method for reference:
Next, we will introduce some core components in OpenMLDB Or features:
Feature 1, online and offline consistent execution engine, based on a unified underlying computing function, adaptive adjustment of online and offline execution modes from logical plan to physical plan, thus making Online and offline consistency is naturally guaranteed.
Feature two, high-performance online feature calculation engine, including high-performance double-layer jump table memory index data structure; real-time computing pre-aggregation technology hybrid optimization strategy; provides both memory/disk Storage engines to meet different performance and cost requirements.
Feature three, optimized offline computing engine for feature calculation, including multi-window parallel computing optimization; data skew calculation optimization; SQL syntax extension; Spark distribution optimized for feature calculation, etc. . These all result in a significant improvement in performance compared to the community version.
Feature 4, SQL extension for feature engineering. As mentioned before, we use SQL for feature definition, but in fact SQL is not designed for feature calculation. Therefore, after studying a large number of cases and accumulating usage experience, we found that it is necessary to make some extensions to the SQL syntax to make it better handle feature calculation. Scenes. There are two important extensions here, one is LAST JOIN and the other is the more commonly used WINDOW UNION, as shown in the following figure:
Feature five, enterprise-level feature support. As a distributed database, OpenMLDB has the characteristics of high availability, seamless expansion and contraction, and smooth upgrade, and has been implemented in many enterprise cases.
Feature 6: Development and management with SQL as the core. OpenMLDB is also a database management. It is similar to traditional databases. For example, if a CLI is provided, then OpenMLDB can be used in the entire CLI. The entire process is implemented in it, from offline feature calculation, SQL solution online to online request, etc., which can provide a full-process development experience based on SQL and CLI.
In addition, OpenMLDB is now open source, and the expansion of its upstream and downstream ecology is as shown in the figure below:
OpenMLDB v0.5.0: Performance, cost, and ease of use enhancements
Next, let’s introduce## A new version of #OpenMLDB v0.5, we have made some enhancements in three aspects.
First, let’s take a look at the development history of OpenMLDB. In June 2021, OpenMLDB was open sourced. In fact, it already had many customers before that, and it had started developing the first line of code in 2017. It has been four or five years of technology accumulation.
In the first anniversary after open source, we iterated about five versions. Compared with previous versions, v0.5.0 has the following significant features:
Performance upgrade, aggregation technology can significantly improve long window performance. Pre-aggregation optimization improves performance by two orders of magnitude in terms of both latency and throughput under long window queries.
Cost reduction, starting from version v0.5.0, the online engine provides two engine options based on memory and external memory. Based on memory, low latency and high concurrency; providing millisecond-level latency response at higher usage costs. Based on external memory, it is less sensitive to performance; the cost can be reduced by 75% under low-cost use and typical configuration based on SSD. The upper-layer business codes of the two engines are imperceptible and can be switched at zero cost.
Enhanced ease of use. We introduced user-defined functions (UDF) in version v0.5.0, which means that if SQL cannot meet your feature extraction logical expression, user-defined functions, such as C/C UDF, UDF dynamic registration, etc., are supported to facilitate users. Expand computing logic and improve application coverage.
Finally, thank you to all OpenMLDB developers. Since the beginning of open source, nearly 100 contributors have made code contributions in our community. At the same time, we also welcome more developers to join. Community, contribute your own strength and do more meaningful things together.
The conference speech replay and PPT are now online, enter the official websiteView exciting content.
The above is the detailed content of OpenMLDB R&D leader Lu Mian, Fourth Paradigm system architect: Open source machine learning database OpenMLDB: a production-level feature platform that is consistent online and offline. For more information, please follow other related articles on the PHP Chinese website!

Hot AI Tools

Undresser.AI Undress
AI-powered app for creating realistic nude photos

AI Clothes Remover
Online AI tool for removing clothes from photos.

Undress AI Tool
Undress images for free

Clothoff.io
AI clothes remover

Video Face Swap
Swap faces in any video effortlessly with our completely free AI face swap tool!

Hot Article

Hot Tools

Notepad++7.3.1
Easy-to-use and free code editor

SublimeText3 Chinese version
Chinese version, very easy to use

Zend Studio 13.0.1
Powerful PHP integrated development environment

Dreamweaver CS6
Visual web development tools

SublimeText3 Mac version
God-level code editing software (SublimeText3)

Hot Topics


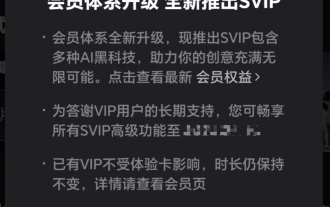
This site reported on June 27 that Jianying is a video editing software developed by FaceMeng Technology, a subsidiary of ByteDance. It relies on the Douyin platform and basically produces short video content for users of the platform. It is compatible with iOS, Android, and Windows. , MacOS and other operating systems. Jianying officially announced the upgrade of its membership system and launched a new SVIP, which includes a variety of AI black technologies, such as intelligent translation, intelligent highlighting, intelligent packaging, digital human synthesis, etc. In terms of price, the monthly fee for clipping SVIP is 79 yuan, the annual fee is 599 yuan (note on this site: equivalent to 49.9 yuan per month), the continuous monthly subscription is 59 yuan per month, and the continuous annual subscription is 499 yuan per year (equivalent to 41.6 yuan per month) . In addition, the cut official also stated that in order to improve the user experience, those who have subscribed to the original VIP
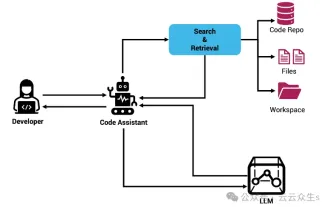
Improve developer productivity, efficiency, and accuracy by incorporating retrieval-enhanced generation and semantic memory into AI coding assistants. Translated from EnhancingAICodingAssistantswithContextUsingRAGandSEM-RAG, author JanakiramMSV. While basic AI programming assistants are naturally helpful, they often fail to provide the most relevant and correct code suggestions because they rely on a general understanding of the software language and the most common patterns of writing software. The code generated by these coding assistants is suitable for solving the problems they are responsible for solving, but often does not conform to the coding standards, conventions and styles of the individual teams. This often results in suggestions that need to be modified or refined in order for the code to be accepted into the application
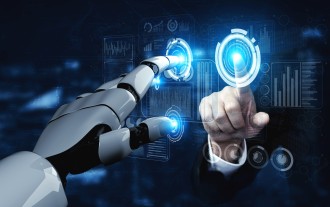
Large Language Models (LLMs) are trained on huge text databases, where they acquire large amounts of real-world knowledge. This knowledge is embedded into their parameters and can then be used when needed. The knowledge of these models is "reified" at the end of training. At the end of pre-training, the model actually stops learning. Align or fine-tune the model to learn how to leverage this knowledge and respond more naturally to user questions. But sometimes model knowledge is not enough, and although the model can access external content through RAG, it is considered beneficial to adapt the model to new domains through fine-tuning. This fine-tuning is performed using input from human annotators or other LLM creations, where the model encounters additional real-world knowledge and integrates it

To learn more about AIGC, please visit: 51CTOAI.x Community https://www.51cto.com/aigc/Translator|Jingyan Reviewer|Chonglou is different from the traditional question bank that can be seen everywhere on the Internet. These questions It requires thinking outside the box. Large Language Models (LLMs) are increasingly important in the fields of data science, generative artificial intelligence (GenAI), and artificial intelligence. These complex algorithms enhance human skills and drive efficiency and innovation in many industries, becoming the key for companies to remain competitive. LLM has a wide range of applications. It can be used in fields such as natural language processing, text generation, speech recognition and recommendation systems. By learning from large amounts of data, LLM is able to generate text
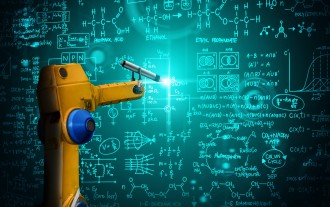
Machine learning is an important branch of artificial intelligence that gives computers the ability to learn from data and improve their capabilities without being explicitly programmed. Machine learning has a wide range of applications in various fields, from image recognition and natural language processing to recommendation systems and fraud detection, and it is changing the way we live. There are many different methods and theories in the field of machine learning, among which the five most influential methods are called the "Five Schools of Machine Learning". The five major schools are the symbolic school, the connectionist school, the evolutionary school, the Bayesian school and the analogy school. 1. Symbolism, also known as symbolism, emphasizes the use of symbols for logical reasoning and expression of knowledge. This school of thought believes that learning is a process of reverse deduction, through existing
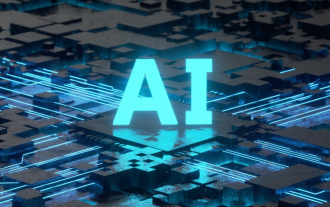
Editor |ScienceAI Question Answering (QA) data set plays a vital role in promoting natural language processing (NLP) research. High-quality QA data sets can not only be used to fine-tune models, but also effectively evaluate the capabilities of large language models (LLM), especially the ability to understand and reason about scientific knowledge. Although there are currently many scientific QA data sets covering medicine, chemistry, biology and other fields, these data sets still have some shortcomings. First, the data form is relatively simple, most of which are multiple-choice questions. They are easy to evaluate, but limit the model's answer selection range and cannot fully test the model's ability to answer scientific questions. In contrast, open-ended Q&A
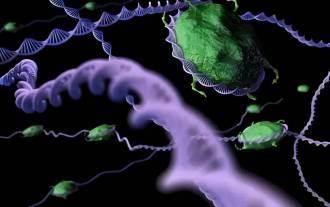
Editor | KX In the field of drug research and development, accurately and effectively predicting the binding affinity of proteins and ligands is crucial for drug screening and optimization. However, current studies do not take into account the important role of molecular surface information in protein-ligand interactions. Based on this, researchers from Xiamen University proposed a novel multi-modal feature extraction (MFE) framework, which for the first time combines information on protein surface, 3D structure and sequence, and uses a cross-attention mechanism to compare different modalities. feature alignment. Experimental results demonstrate that this method achieves state-of-the-art performance in predicting protein-ligand binding affinities. Furthermore, ablation studies demonstrate the effectiveness and necessity of protein surface information and multimodal feature alignment within this framework. Related research begins with "S
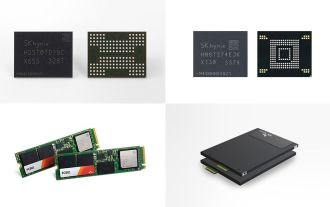
According to news from this site on August 1, SK Hynix released a blog post today (August 1), announcing that it will attend the Global Semiconductor Memory Summit FMS2024 to be held in Santa Clara, California, USA from August 6 to 8, showcasing many new technologies. generation product. Introduction to the Future Memory and Storage Summit (FutureMemoryandStorage), formerly the Flash Memory Summit (FlashMemorySummit) mainly for NAND suppliers, in the context of increasing attention to artificial intelligence technology, this year was renamed the Future Memory and Storage Summit (FutureMemoryandStorage) to invite DRAM and storage vendors and many more players. New product SK hynix launched last year
