


Smart agriculture: Computer vision and robotics are being used to improve efficiency
Artificial intelligence in agriculture helps detect pests, plant diseases and malnutrition in farms. AI sensors can identify and target weeds before deciding which herbicide to use in the area. Precision agriculture, often referred to as artificial intelligence systems, is helping to improve the overall quality and accuracy of harvests.
The role of computer vision
We need a lot of land to feed billions of people. Today, manual cultivation is no longer possible. Meanwhile, crop failures are often caused by insect pests and plant diseases. Given the scale of modern agricultural operations, detecting and stopping this pest invasion is challenging.
This adds a new application to computer vision technology. Farmers can use aerial photography to identify early indicators of plant disease or pests at the macro level and to identify crop diseases at the micro level using close-up photos of leaves and plants. A commonly used computer vision method in these studies is convolutional neural networks. Today, more applications of artificial intelligence in agriculture are being developed.
It’s worth noting that we use the term “computer vision” very broadly here. Images are generally not the most reliable source of information. Many important aspects of plant life are best studied using other methods. Specialized sensors are often used to collect hyperspectral images or 3D laser scans are used to better understand plant health. In the field of agronomy, this type of technology is increasingly used due to the application of artificial intelligence in agriculture.
This data type is typically high-resolution and more similar to photos than medical imaging. AgMRI is an on-site monitoring system. Although specialized models are required to process this data, convolutional neural networks in particular can be used due to the spatial organization of the data.
Multi-million dollar investment in plant phenotyping and imaging research. The main task now is to collect sizable datasets of crop plants, usually in the form of pictures or three-dimensional images, and compare phenotypic information with plant genotypes. Research results and information can be used to advance agricultural technology worldwide. Agriculture is not the only field using smart AI systems, AI is also a hot topic in recruitment.
How Robots Are Used in Agriculture
Many autonomous agricultural robots are capable of digging holes and planting seeds in the ground while adhering to established basic patterns and taking into account the unique characteristics of the area. The robots are also able to manage the plant's growth process and interact with each plant individually. When the crops are ripe, the robots will harvest them, again treating each plant the way it should be treated.
Drones can automatically spray crops. Small, nimble drones can deliver hazardous materials with greater precision than larger aircraft. Additionally, aerial photography captured using spray drones can be used to collect data for the computer vision algorithms described at the beginning of this article.
Robots specifically designed for harvesting are increasingly being created and deployed. Combine harvesters have been used for a long time, while individual weeds can be identified and mechanically removed by robots. This is another remarkable achievement of contemporary robotics and computer vision, because before this, it was impossible to differentiate between weeds and beneficial plants, or to use robotic hands to interact with small plants.
Artificial intelligence improves efficiency in agriculture
While many agricultural robots are still prototypes or only tested on a small scale, the application of ML, AI and robotics in agriculture is already evident . It is believed that in the near future, more and more agricultural activities will adopt mechanization.
Today, more applications of artificial intelligence in agriculture are being developed. For example, a pilot project of one company applies computer vision to animal husbandry, but this area has not yet attracted widespread interest from deep learning companies.
Machine Learning and Artificial Intelligence in Agriculture
Of course, there are already some initiatives to use livestock tracking data for machine learning. For example, a Pakistani company has launched a collar that can wirelessly monitor the activity and body temperature of cows. And French researchers are working on facial recognition technology for cows.
Additionally, there are plans to apply artificial intelligence to pig farming, a hitherto underutilized industry with a market value of hundreds of billions of dollars. On modern farms, pigs are housed in relatively small groups and the most comparable animals are selected. Food is the main expense in pig production and, therefore, the main goal of contemporary pig production is to maximize the fattening process.
If farmers had a comprehensive understanding of pig weight gain, they could solve this problem. Animals are usually weighed only twice in their lives, when they are born and when they are sold. If experts knew how each piglet gained weight, they could design a unique fattening program for each pig, or even a unique combination of food additives. This will significantly increase production.
Although getting animals onto the scale is not particularly difficult, it can put a lot of stress on them, and stressed pigs will lose weight. This new artificial intelligence research aims to create a new, non-invasive method of weighing animals. Use computer vision models to infer pig weight from photo and video data. These estimates will be incorporated into existing traditional analytical machine learning models to enhance the fattening process.
What is the future of artificial intelligence in agriculture
Farming and livestock farming are sometimes viewed as outdated professions. Today, however, artificial intelligence in agriculture is becoming a common tool on many farms. The main reason for this phenomenon is the large number of jobs that are carried out simultaneously in agriculture.
They are so tedious that deep learning and contemporary artificial intelligence must be used to automate them. Although the cultivated plants and pigs are identical, they do not come from the same assembly line. Every tomato bush and every pig requires a unique approach, so human intervention is absolutely necessary.
We can leverage current developments in artificial intelligence to solve challenges while also automating technologies that interact with animals and plants and take into account their unique characteristics. Weighing a pig is easier than learning how to pass the Turing test, and operating a tractor in a wide field is easier than operating a car in heavy traffic.
Since agriculture remains one of the largest and most important industries in the world, even small improvements in efficiency can lead to significant gains. This is why many companies are prioritizing artificial intelligence in agriculture.
The above is the detailed content of Smart agriculture: Computer vision and robotics are being used to improve efficiency. For more information, please follow other related articles on the PHP Chinese website!

Hot AI Tools

Undresser.AI Undress
AI-powered app for creating realistic nude photos

AI Clothes Remover
Online AI tool for removing clothes from photos.

Undress AI Tool
Undress images for free

Clothoff.io
AI clothes remover

Video Face Swap
Swap faces in any video effortlessly with our completely free AI face swap tool!

Hot Article

Hot Tools

Notepad++7.3.1
Easy-to-use and free code editor

SublimeText3 Chinese version
Chinese version, very easy to use

Zend Studio 13.0.1
Powerful PHP integrated development environment

Dreamweaver CS6
Visual web development tools

SublimeText3 Mac version
God-level code editing software (SublimeText3)

Hot Topics


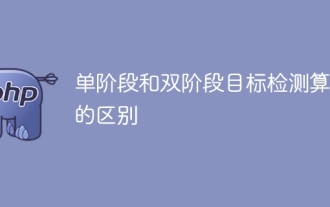
Object detection is an important task in the field of computer vision, used to identify objects in images or videos and locate their locations. This task is usually divided into two categories of algorithms, single-stage and two-stage, which differ in terms of accuracy and robustness. Single-stage target detection algorithm The single-stage target detection algorithm converts target detection into a classification problem. Its advantage is that it is fast and can complete the detection in just one step. However, due to oversimplification, the accuracy is usually not as good as the two-stage object detection algorithm. Common single-stage target detection algorithms include YOLO, SSD and FasterR-CNN. These algorithms generally take the entire image as input and run a classifier to identify the target object. Unlike traditional two-stage target detection algorithms, they do not need to define areas in advance, but directly predict
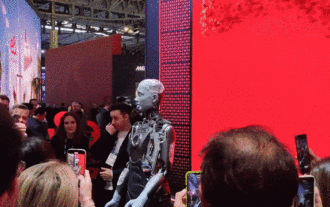
The humanoid robot Ameca has been upgraded to the second generation! Recently, at the World Mobile Communications Conference MWC2024, the world's most advanced robot Ameca appeared again. Around the venue, Ameca attracted a large number of spectators. With the blessing of GPT-4, Ameca can respond to various problems in real time. "Let's have a dance." When asked if she had emotions, Ameca responded with a series of facial expressions that looked very lifelike. Just a few days ago, EngineeredArts, the British robotics company behind Ameca, just demonstrated the team’s latest development results. In the video, the robot Ameca has visual capabilities and can see and describe the entire room and specific objects. The most amazing thing is that she can also
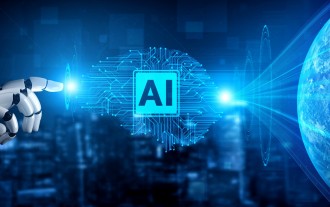
In the field of industrial automation technology, there are two recent hot spots that are difficult to ignore: artificial intelligence (AI) and Nvidia. Don’t change the meaning of the original content, fine-tune the content, rewrite the content, don’t continue: “Not only that, the two are closely related, because Nvidia is expanding beyond just its original graphics processing units (GPUs). The technology extends to the field of digital twins and is closely connected to emerging AI technologies. "Recently, NVIDIA has reached cooperation with many industrial companies, including leading industrial automation companies such as Aveva, Rockwell Automation, Siemens and Schneider Electric, as well as Teradyne Robotics and its MiR and Universal Robots companies. Recently,Nvidiahascoll
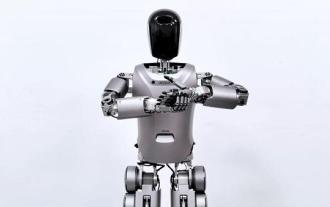
Editor of Machine Power Report: Wu Xin The domestic version of the humanoid robot + large model team completed the operation task of complex flexible materials such as folding clothes for the first time. With the unveiling of Figure01, which integrates OpenAI's multi-modal large model, the related progress of domestic peers has been attracting attention. Just yesterday, UBTECH, China's "number one humanoid robot stock", released the first demo of the humanoid robot WalkerS that is deeply integrated with Baidu Wenxin's large model, showing some interesting new features. Now, WalkerS, blessed by Baidu Wenxin’s large model capabilities, looks like this. Like Figure01, WalkerS does not move around, but stands behind a desk to complete a series of tasks. It can follow human commands and fold clothes
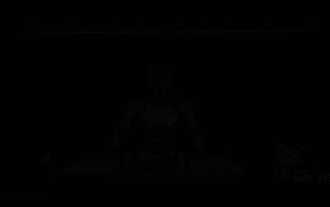
This week, FigureAI, a robotics company invested by OpenAI, Microsoft, Bezos, and Nvidia, announced that it has received nearly $700 million in financing and plans to develop a humanoid robot that can walk independently within the next year. And Tesla’s Optimus Prime has repeatedly received good news. No one doubts that this year will be the year when humanoid robots explode. SanctuaryAI, a Canadian-based robotics company, recently released a new humanoid robot, Phoenix. Officials claim that it can complete many tasks autonomously at the same speed as humans. Pheonix, the world's first robot that can autonomously complete tasks at human speeds, can gently grab, move and elegantly place each object to its left and right sides. It can autonomously identify objects
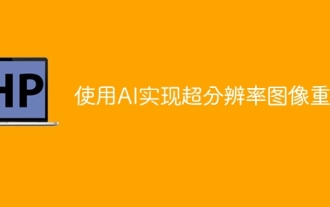
Super-resolution image reconstruction is the process of generating high-resolution images from low-resolution images using deep learning techniques, such as convolutional neural networks (CNN) and generative adversarial networks (GAN). The goal of this method is to improve the quality and detail of images by converting low-resolution images into high-resolution images. This technology has wide applications in many fields, such as medical imaging, surveillance cameras, satellite images, etc. Through super-resolution image reconstruction, we can obtain clearer and more detailed images, which helps to more accurately analyze and identify targets and features in images. Reconstruction methods Super-resolution image reconstruction methods can generally be divided into two categories: interpolation-based methods and deep learning-based methods. 1) Interpolation-based method Super-resolution image reconstruction based on interpolation
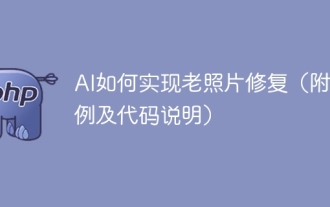
Old photo restoration is a method of using artificial intelligence technology to repair, enhance and improve old photos. Using computer vision and machine learning algorithms, the technology can automatically identify and repair damage and flaws in old photos, making them look clearer, more natural and more realistic. The technical principles of old photo restoration mainly include the following aspects: 1. Image denoising and enhancement. When restoring old photos, they need to be denoised and enhanced first. Image processing algorithms and filters, such as mean filtering, Gaussian filtering, bilateral filtering, etc., can be used to solve noise and color spots problems, thereby improving the quality of photos. 2. Image restoration and repair In old photos, there may be some defects and damage, such as scratches, cracks, fading, etc. These problems can be solved by image restoration and repair algorithms
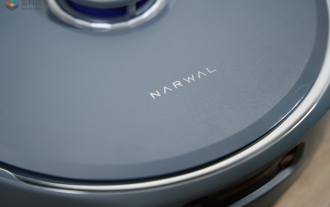
Sweeping and mopping robots are one of the most popular smart home appliances among consumers in recent years. The convenience of operation it brings, or even the need for no operation, allows lazy people to free their hands, allowing consumers to "liberate" from daily housework and spend more time on the things they like. Improved quality of life in disguised form. Riding on this craze, almost all home appliance brands on the market are making their own sweeping and mopping robots, making the entire sweeping and mopping robot market very lively. However, the rapid expansion of the market will inevitably bring about a hidden danger: many manufacturers will use the tactics of sea of machines to quickly occupy more market share, resulting in many new products without any upgrade points. It is also said that they are "matryoshka" models. Not an exaggeration. However, not all sweeping and mopping robots are
