


Jeff Dean's large-scale multi-task learning SOTA was criticized, and it would cost US$60,000 to reproduce it
In October 2021, Jeff Dean personally wrote an article introducing a new machine learning architecture-Pathways.
The purpose is very simple, which is to enable an AI to span tens of thousands of tasks, understand different types of data, and achieve it with extremely high efficiency at the same time:
More than half a year later, in March 2022, Jeff Dean finally released the Pathways paper.
Paper link: https://arxiv.org/abs/2203.12533
, which adds a lot of technical information Details, such as the most basic system architecture, etc.
In April 2022, Google used Pathways’ PaLM language model to emerge, successively breaking the SOTA of multiple natural language processing tasks. This Transformer language has 540 billion parameters. The model once again proves that “power can work miracles”.
In addition to using the powerful Pathways system, the paper introduces that the training of PaLM uses 6144 TPU v4, using a high-quality data set of 780 billion tokens, and a certain proportion of them non-English multilingual corpus.
Paper address: https://arxiv.org/abs/2204.02311
Recently, a new work by Jeff Dean caused another stir Everyone’s guesses about Pathways.
Another piece of the Pathways puzzle has been put together?
There are only two authors of this paper: the famous Jeff Dean and the Italian engineer Andrea Gesmundo.
What’s interesting is that not only is Gesmundo very low-key, but Jeff Dean, who just blew up his Imagen two days ago, didn’t mention it at all on Twitter.
After reading it, some netizens speculated that this may be a component of the next generation AI architecture Pathways.
Paper address: https://arxiv.org/abs/2205.12755
The idea of this article is as follows:
By dynamically incorporating new tasks into a large-scale running system, fragments of sparse multi-task machine learning models can be used to improve the quality of new tasks and can be automatically shared among related tasks. Fragments of the model.
This approach can improve the quality of each task and improve model efficiency in terms of convergence time, number of training instances, energy consumption, etc. The machine learning problem framework proposed in this article can be regarded as a generalization and synthesis of standard multi-task and continuous learning formalizations.
Under this framework, no matter how large the task set is, it can be jointly solved.
Moreover, over time, the task set can be expanded by adding a continuous stream of new tasks. The distinction between pre-training tasks and downstream tasks also disappears.
Because, as new tasks are added, the system will look for how to combine existing knowledge and representations with new model capabilities to achieve the high quality level of each new task. . The knowledge and representations learned while solving a new task can also be used in any future tasks, or to continue learning on existing tasks.
This method is called "Mutation Multitasking Network" or µ2Net. (μ=Mutation)
Two types of mutation models for large-scale continuous learning experiments
To put it simply, it is to generate a large-scale multi-task network to jointly solve multiple tasks. Not only is the quality and efficiency of each task improved, but the model can also be expanded by dynamically adding new tasks.
The more knowledge is accumulated into the system through learning from previous tasks, the higher the quality of the solutions to subsequent tasks.
In addition, in terms of reducing the newly added parameters of each task, the efficiency of solving new tasks can be continuously improved. The generated multi-task model is sparsely activated, and the model integrates a task-based routing mechanism. As the model expands, the increase in computational cost of each task is guaranteed to be bounded.
The activated and increased parameters of each task account for the percentage of the total number of multi-task system parameters
Learned from each task The knowledge is divided into parts that can be reused by multiple tasks. Experiments have shown that this chunking technique avoids common problems of multi-task and continuous learning models, such as catastrophic forgetting, gradient interference and negative transfer.
The exploration of the task route space and the identification of the most relevant subset of prior knowledge for each task is guided by an evolutionary algorithm designed to dynamically adjust the exploration/ Exploit balance without the need to manually adjust meta-parameters. The same evolutionary logic is used to dynamically adjust hyperparameter multi-task model components.
Since it is called "mutation network", how do you explain this mutation?
Deep neural networks are often defined by architecture and hyperparameters. The architecture in this article is composed of a series of neural network layers. Each layer maps an input vector to a variable-dimensional output vector, and details of network instantiation, such as the configuration of the optimizer or data preprocessing, are determined by hyperparameters.
So the mutations mentioned here are also divided into two categories, layer cloning mutations and hyperparameter mutations.
The layer clone mutation creates a copy of any parent model layer that can be trained by the child model. If a layer of the parent model is not selected for cloning, the current state is frozen and shared with the child model to ensure the invariance of the pre-existing model.
Hyperparameter mutation is used to modify the configuration inherited by the child layer from the parent layer. The new value for each hyperparameter can be drawn from a set of valid values. For numeric hyperparameters, the set of valid values is sorted as a list, and sampling is limited to adjacent values to apply an incremental change constraint.
Let’s take a look at the actual effect:
On the three data sets of ImageNet 2012, cifar100, and cifar10, µ2Net is in 5 The performance after task iteration and 10 task iterations exceeded the current most versatile and best-performing ViT pre-trained fine-tuning model.
In terms of task expansion, after adding VTAB-full and VDD continuous learning tasks, the performance of µ2Net has been further improved. The VDD continuous learning task performance on the cifar10 data set reached 99.43 % of the best results.
On the multi-task character classification benchmark task, after two task iterations, µ2Net refreshed the SOTA level on most data sets, and the data set size ranged from 2.5k to 240k sample sizes vary.
Simply put, under this architecture, the more tasks the model learns, the more knowledge the system learns, and the easier it is to solve new tasks.
For example, a ViT-L architecture (307 million parameters) can evolve into a multi-task system with 130.87 billion parameters and solve 69 tasks.
Additionally, as the system grows, the sparsity of parameter activations keeps the computational effort and memory usage of each task constant. Experiments show that the average number of added parameters for each task is reduced by 38%, while the multi-task system only activates 2.3% of the total parameters for each task.
Of course, at this point, it's just an architecture and preliminary experiment.
Netizen: The paper is very good, but...
Although the paper is great, some people don’t seem to buy it.
Some netizens who love to expose the emperor's new clothes posted on reddit, saying that they no longer believe in love... Oh no, AI papers produced by "top laboratories/research institutions" .
The netizen with the ID "Mr. Acurite" said that he naturally believed in the data and model operation results in these papers.
But, take this paper by teacher Jeff Dean as an example. The 18-page paper talks about a particularly complex evolutionary convolution and multi-task learning algorithm. It is powerful and eye-catching. Very thumbs up.
However, there are two points that have to be made:
First, what Jeff Dean proposed in the paper proves that he is better than the competition. The running score result is that the CIFAR-10 benchmark test accuracy is 99.43, which is better than the current SOTA's 99.40... wording to describe.
#Second, at the end of the paper, there is a time consumption table using the TPU running algorithm to obtain the final results, totaling 17810 hours.Assuming someone who is not working at Google wants to reproduce the results of the paper and rents a TPU at the market price of US$3.22 per hour to run it again, the cost will be US$57,348.
What does it mean? Do you need to set a competency threshold even for daily papers?
Of course, this approach is now a trend in the industry, including but not limited to big players such as Google and OpenAI. Everyone pours into the model some ideas to improve the status quo, and a lot of preprocessed data and benchmarks.
Then, as long as the running result is numerically higher than that of the opponent by even two decimal places after a percentage point, the researcher can confidently add a new line of thesis title to the resume!
What real impetus will be given to academia and industry by doing this? Ordinary graduate students cannot afford to spend money to verify your conclusions, and ordinary companies cannot use such boring benchmarks in projects.
The same sentence, what does it mean?
Is this the acceptable comfort zone in the AI world? A small group of big companies, and occasionally top schools, show off every day that I have money and can do whatever I want, but you have no money and have to lag behind?
If we continue like this, we might as well open another computer science journal and collect papers whose results can be reproduced in eight hours on a consumer-grade stand-alone graphics card.
In the thread, graduate students with thesis assignments complained one after another.
A netizen whose ID is "Support Vector Machine" said that he is a practitioner in a small laboratory. Because of this momentum, he has almost completely lost the motivation to continue to engage in deep learning. .
Because with the budget of my own laboratory, there is no way to compare with these giants, and I cannot produce benchmark results that show bottom-line performance.
Even if you have a new theoretical idea, it is difficult to write a paper that can pass the review. This is because the current paper reviewers have developed a "beautiful picture bias" due to the ability of major manufacturers. The images used for testing in the paper are not good-looking, and everything is in vain.
This is not to say that the giant manufacturers are useless. Projects such as GPT and DALL-E are really groundbreaking. But if my own machine can't run, why should I be excited?
Another netizen who is a doctoral student showed up and commented on the post to support the "support vector machine".
The doctoral student submitted a paper on the flow model two years ago, which mainly focused on discovering the potential space of data that can be sampled, and had no impact on the quality of the model's image generation.
As a result, the critical comment given by the grader of the paper was: "The generated images do not look as good as those generated with GAN."
Another graduate student with the ID name "Uday" also said that the grader's critical comment on the paper he submitted for conferences in 2021 was: "The data is not fancy enough."
It seems that manpower is not as good as money's ability. This is really a worldwide trend in which the psychology of the East and the West is the same, and the Taoism of China and foreign countries is not broken.But in thirty years in Hedong and thirty years in Hexi, maybe the grass-roots implementation of algorithms and universal capitalization will bring about the second miracle of garage startups defeating IBM.
The above is the detailed content of Jeff Dean's large-scale multi-task learning SOTA was criticized, and it would cost US$60,000 to reproduce it. For more information, please follow other related articles on the PHP Chinese website!

Hot AI Tools

Undresser.AI Undress
AI-powered app for creating realistic nude photos

AI Clothes Remover
Online AI tool for removing clothes from photos.

Undress AI Tool
Undress images for free

Clothoff.io
AI clothes remover

AI Hentai Generator
Generate AI Hentai for free.

Hot Article

Hot Tools

Notepad++7.3.1
Easy-to-use and free code editor

SublimeText3 Chinese version
Chinese version, very easy to use

Zend Studio 13.0.1
Powerful PHP integrated development environment

Dreamweaver CS6
Visual web development tools

SublimeText3 Mac version
God-level code editing software (SublimeText3)

Hot Topics
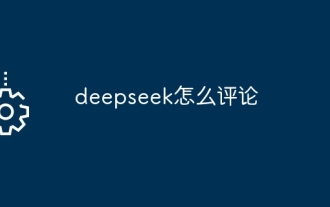
DeepSeek is a powerful information retrieval tool. Its advantage is that it can deeply mine information, but its disadvantages are that it is slow, the result presentation method is simple, and the database coverage is limited. It needs to be weighed according to specific needs.
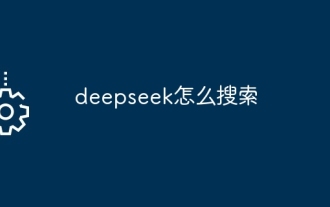
DeepSeek is a proprietary search engine that only searches in a specific database or system, faster and more accurate. When using it, users are advised to read the document, try different search strategies, seek help and feedback on the user experience in order to make the most of their advantages.
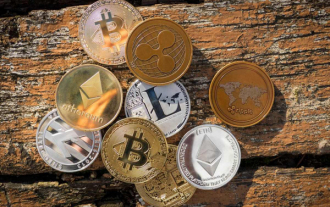
This article introduces the registration process of the Sesame Open Exchange (Gate.io) web version and the Gate trading app in detail. Whether it is web registration or app registration, you need to visit the official website or app store to download the genuine app, then fill in the user name, password, email, mobile phone number and other information, and complete email or mobile phone verification.
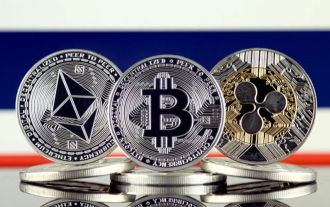
It is crucial to choose a formal channel to download the app and ensure the safety of your account.
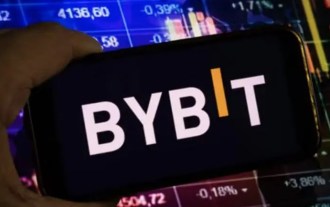
Why can’t the Bybit exchange link be directly downloaded and installed? Bybit is a cryptocurrency exchange that provides trading services to users. The exchange's mobile apps cannot be downloaded directly through AppStore or GooglePlay for the following reasons: 1. App Store policy restricts Apple and Google from having strict requirements on the types of applications allowed in the app store. Cryptocurrency exchange applications often do not meet these requirements because they involve financial services and require specific regulations and security standards. 2. Laws and regulations Compliance In many countries, activities related to cryptocurrency transactions are regulated or restricted. To comply with these regulations, Bybit Application can only be used through official websites or other authorized channels
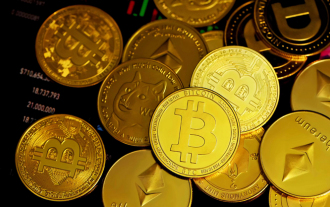
Gate.io is a leading cryptocurrency exchange that offers a wide range of crypto assets and trading pairs. Registering Gate.io is very simple. You just need to visit its official website or download the app, click "Register", fill in the registration form, verify your email, and set up two-factor verification (2FA), and you can complete the registration. With Gate.io, users can enjoy a safe and convenient cryptocurrency trading experience.
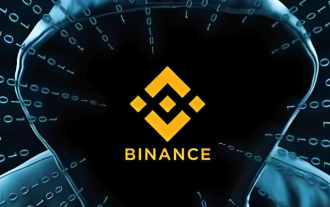
To access the latest version of Binance website login portal, just follow these simple steps. Go to the official website and click the "Login" button in the upper right corner. Select your existing login method. If you are a new user, please "Register". Enter your registered mobile number or email and password and complete authentication (such as mobile verification code or Google Authenticator). After successful verification, you can access the latest version of Binance official website login portal.
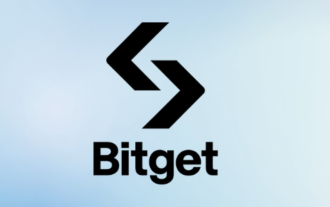
This guide provides detailed download and installation steps for the official Bitget Exchange app, suitable for Android and iOS systems. The guide integrates information from multiple authoritative sources, including the official website, the App Store, and Google Play, and emphasizes considerations during download and account management. Users can download the app from official channels, including app store, official website APK download and official website jump, and complete registration, identity verification and security settings. In addition, the guide covers frequently asked questions and considerations, such as
