Responsible machine learning – the 'glass box” approach
Translator | Cui Hao
Reviewer | Sun Shujuan
Opening
Machine learning is not a profound technology. Just as multi-parameter and hyperparameter methods in complex deep neural networks are just a form of cognitive computing, they don't seem that profound.
There are other types of machine learning (some involving deep neural networks). The model results of this type of machine learning, the determination of the model, and the complexity that affects the model are all Be very transparent.
All of this depends on how well the organization understands the sources of its data.
In other words, you need to understand everything from model training data to production data model. It is also integral to interpreting, refining and improving its results. In this way, organizations can greatly increase the business value of their models.
More importantly, it further improves the fairness, accountability and transparency of this technology, making it more reliable and complete for the entire society.
Joel Minnick, vice president of marketing at Databricks, admitted: “This is why you need granular understanding of the upstream and downstream of the data to be able to do machine learning responsibly.”
for data Lineage compilation catalog
Data training and data generation for models will involve multiple technologies such as data sources, data conversion, and data integration. In a mature data catalog solution, real-time data capture can be achieved, so the progress can be monitored at any time to understand the execution progress of the model. "It gives me a clear understanding of the context in which the data is being used in the model. Also, you know, where did this data come from? What other data did we get from it? When was it generated? So I can To better understand how I should use this data," said data scientist Minnick.
"Data lineage" (recording data source, movement, and processing) consists of metadata, and the data directory is used to store relevant data sets. Catalogs also enable users to include tags and other descriptors as additional metadata, which can help trace data provenance and establish trust in the data. "Data lineage," as Minnick describes it, can generate "API-driven services" that connect a range of platforms (including data scientist platforms, data engineer platforms, and end-user platforms).
Data Governance: Born for Data Science
The improved traceability of data training and data operations will affect the results of machine learning models, and the model results are in turn related to data governance in the field of data science. closely related. Therefore, data governance is inextricably linked to the data science platform that creates and deploys models. “Skills manage spreadsheets and files, manage notebooks, and manage dashboards at the same time. It’s the modern way to manage production and consumption data,” commented Minnick. This statement rings true for data scientists who build models in their notebooks and monitor output through dashboards.
Clear and Transparent
Nonetheless, simply connecting to a data science tool platform through an API to obtain "data lineage" is only one aspect of transparently leveraging machine learning. In order to achieve the purpose of improving the output of the model, the output model also needs to be calibrated with the content determined in the data lineage. For example, how to traceability model data so that data scientists "can understand if something goes wrong with some data, they can isolate that part of the data," Minnick noted.
Logically, this knowledge can be used to understand why there are problems with specific data types, and thus correct them or improve the accuracy of the model by removing them entirely. According to Minnick, more and more organizations are realizing the benefits of applying “data lineage” to model results, “due in part to the rise of machine learning and artificial intelligence in various industries today. It’s becoming more and more common. Last year, when we launched our AutoML product, we used a "glass box" to represent transparency into data sources."
Regulatory consequences and others
Some organizations also use "data lineage" to provide The ability of adaptive cognitive computing models to enhance their regulatory compliance capabilities. Industries such as finance and healthcare are highly regulated, requiring companies to clearly explain how they make decisions for their customers. Data traceability creates a roadmap for building machine learning models and understanding model results—invaluable for regulatory compliance.
This information also assists internal audits, allowing companies to understand where they are failing in regulatory areas so that issues can be corrected to prevent breaches. “Being able to present very granular data lineage information to regulators, not just across tables but where that data can be used anywhere across a broad organization, is really important,” Minnick asserts. When this advantage coincides with the idea that data sources improve model accuracy, this approach is likely to become a best practice for deploying this technology.
Translator Introduction
Cui Hao, 51CTO community editor and senior architect, has 18 years of software development and architecture experience and 10 years of distributed architecture experience. Formerly a technical expert at HP. He is willing to share and has written many popular technical articles with more than 600,000 reads. Author of "Principles and Practice of Distributed Architecture".
Original title: A “Glass Box” Approach to Responsible Machine Learning, author: Jelani Harper
The above is the detailed content of Responsible machine learning – the 'glass box” approach. For more information, please follow other related articles on the PHP Chinese website!

Hot AI Tools

Undresser.AI Undress
AI-powered app for creating realistic nude photos

AI Clothes Remover
Online AI tool for removing clothes from photos.

Undress AI Tool
Undress images for free

Clothoff.io
AI clothes remover

Video Face Swap
Swap faces in any video effortlessly with our completely free AI face swap tool!

Hot Article

Hot Tools

Notepad++7.3.1
Easy-to-use and free code editor

SublimeText3 Chinese version
Chinese version, very easy to use

Zend Studio 13.0.1
Powerful PHP integrated development environment

Dreamweaver CS6
Visual web development tools

SublimeText3 Mac version
God-level code editing software (SublimeText3)

Hot Topics




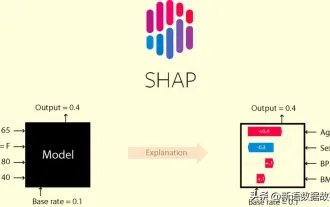
In the fields of machine learning and data science, model interpretability has always been a focus of researchers and practitioners. With the widespread application of complex models such as deep learning and ensemble methods, understanding the model's decision-making process has become particularly important. Explainable AI|XAI helps build trust and confidence in machine learning models by increasing the transparency of the model. Improving model transparency can be achieved through methods such as the widespread use of multiple complex models, as well as the decision-making processes used to explain the models. These methods include feature importance analysis, model prediction interval estimation, local interpretability algorithms, etc. Feature importance analysis can explain the decision-making process of a model by evaluating the degree of influence of the model on the input features. Model prediction interval estimate
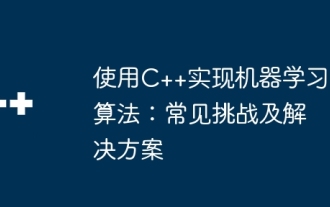
Common challenges faced by machine learning algorithms in C++ include memory management, multi-threading, performance optimization, and maintainability. Solutions include using smart pointers, modern threading libraries, SIMD instructions and third-party libraries, as well as following coding style guidelines and using automation tools. Practical cases show how to use the Eigen library to implement linear regression algorithms, effectively manage memory and use high-performance matrix operations.
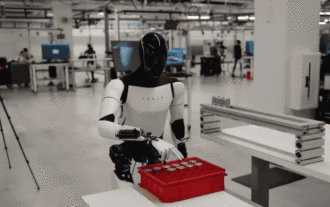
The latest video of Tesla's robot Optimus is released, and it can already work in the factory. At normal speed, it sorts batteries (Tesla's 4680 batteries) like this: The official also released what it looks like at 20x speed - on a small "workstation", picking and picking and picking: This time it is released One of the highlights of the video is that Optimus completes this work in the factory, completely autonomously, without human intervention throughout the process. And from the perspective of Optimus, it can also pick up and place the crooked battery, focusing on automatic error correction: Regarding Optimus's hand, NVIDIA scientist Jim Fan gave a high evaluation: Optimus's hand is the world's five-fingered robot. One of the most dexterous. Its hands are not only tactile
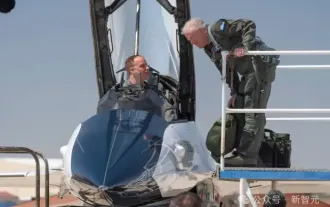
Recently, the military circle has been overwhelmed by the news: US military fighter jets can now complete fully automatic air combat using AI. Yes, just recently, the US military’s AI fighter jet was made public for the first time and the mystery was unveiled. The full name of this fighter is the Variable Stability Simulator Test Aircraft (VISTA). It was personally flown by the Secretary of the US Air Force to simulate a one-on-one air battle. On May 2, U.S. Air Force Secretary Frank Kendall took off in an X-62AVISTA at Edwards Air Force Base. Note that during the one-hour flight, all flight actions were completed autonomously by AI! Kendall said - "For the past few decades, we have been thinking about the unlimited potential of autonomous air-to-air combat, but it has always seemed out of reach." However now,
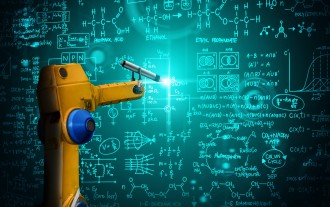
Machine learning is an important branch of artificial intelligence that gives computers the ability to learn from data and improve their capabilities without being explicitly programmed. Machine learning has a wide range of applications in various fields, from image recognition and natural language processing to recommendation systems and fraud detection, and it is changing the way we live. There are many different methods and theories in the field of machine learning, among which the five most influential methods are called the "Five Schools of Machine Learning". The five major schools are the symbolic school, the connectionist school, the evolutionary school, the Bayesian school and the analogy school. 1. Symbolism, also known as symbolism, emphasizes the use of symbols for logical reasoning and expression of knowledge. This school of thought believes that learning is a process of reverse deduction, through existing
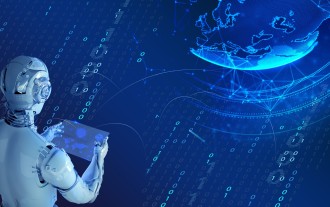
Translator | Reviewed by Li Rui | Chonglou Artificial intelligence (AI) and machine learning (ML) models are becoming increasingly complex today, and the output produced by these models is a black box – unable to be explained to stakeholders. Explainable AI (XAI) aims to solve this problem by enabling stakeholders to understand how these models work, ensuring they understand how these models actually make decisions, and ensuring transparency in AI systems, Trust and accountability to address this issue. This article explores various explainable artificial intelligence (XAI) techniques to illustrate their underlying principles. Several reasons why explainable AI is crucial Trust and transparency: For AI systems to be widely accepted and trusted, users need to understand how decisions are made
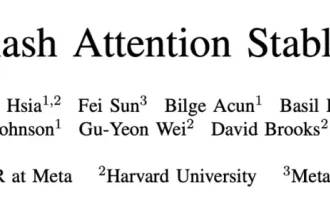
MetaFAIR teamed up with Harvard to provide a new research framework for optimizing the data bias generated when large-scale machine learning is performed. It is known that the training of large language models often takes months and uses hundreds or even thousands of GPUs. Taking the LLaMA270B model as an example, its training requires a total of 1,720,320 GPU hours. Training large models presents unique systemic challenges due to the scale and complexity of these workloads. Recently, many institutions have reported instability in the training process when training SOTA generative AI models. They usually appear in the form of loss spikes. For example, Google's PaLM model experienced up to 20 loss spikes during the training process. Numerical bias is the root cause of this training inaccuracy,
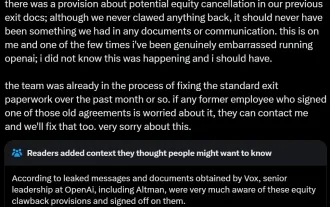
Last week, amid the internal wave of resignations and external criticism, OpenAI was plagued by internal and external troubles: - The infringement of the widow sister sparked global heated discussions - Employees signing "overlord clauses" were exposed one after another - Netizens listed Ultraman's "seven deadly sins" Rumors refuting: According to leaked information and documents obtained by Vox, OpenAI’s senior leadership, including Altman, was well aware of these equity recovery provisions and signed off on them. In addition, there is a serious and urgent issue facing OpenAI - AI safety. The recent departures of five security-related employees, including two of its most prominent employees, and the dissolution of the "Super Alignment" team have once again put OpenAI's security issues in the spotlight. Fortune magazine reported that OpenA
