


An article discussing the application of SLAM technology in autonomous driving
Positioning occupies an irreplaceable position in autonomous driving, and there is promising development in the future. Currently, positioning in autonomous driving relies on RTK and high-precision maps, which adds a lot of cost and difficulty to the implementation of autonomous driving. Just imagine that when humans drive, they do not need to know their own global high-precision positioning and the detailed surrounding environment. It is enough to have a global navigation path and match the vehicle's position on the path. What is involved here is the SLAM field. key technologies.
What is SLAM
SLAM (Simultaneous Localization and Mapping), also known as CML (Concurrent Mapping and Localization), real-time positioning and map Construction, or concurrent mapping and positioning. The problem can be described as: Put a robot into an unknown location in an unknown environment. Is there a way for the robot to gradually draw a complete map of the environment while deciding in which direction the robot should move? For example, a sweeping robot is a very typical SLAM problem. The so-called complete map (a consistent map) means that it can travel to every accessible corner of the room without obstacles.
SLAM was first proposed by Smith, Self and Cheeseman in 1988. Due to its important theoretical and application value, it is considered by many scholars to be the key to realizing a truly fully autonomous mobile robot.
When simulated humans come to a strange environment, in order to quickly familiarize themselves with the environment and complete their tasks (such as finding a restaurant, finding a hotel), they should do the following in order:
a. Use your eyes to observe surrounding landmarks such as buildings, big trees, flower beds, etc., and remember their features (feature extraction)
b. In one's own mind, based on the information obtained by the binoculars, the characteristic landmarks are reconstructed in the three-dimensional map (three-dimensional reconstruction)
c. When walking, constantly acquire new Feature landmarks, and correct the map model in your mind (bundle adjustment or EKF)
d. Determine your position (trajectory) based on the feature landmarks you obtained from walking some time ago
e. When you walk a long way unintentionally, match it with the previous landmarks in your mind to see if you have returned to the original path (loop-closure detection). In reality, this step is optional. The above five steps are performed simultaneously, so they are Simultaneous Localization and Mapping.
Laser SLAM and visual SLAM
The sensors currently used in SLAM are mainly divided into two categories, lidar and camera. LiDAR can be divided into single-line and multi-line types, with different angular resolution and accuracy.
VSLAM is mainly implemented using cameras. There are many types of cameras, mainly divided into monocular, binocular, monocular structured light, and binocular. Structured light and ToF are several categories. Their core is to obtain RGB and depth map (depth information). Due to the impact of manufacturing costs, visual SLAM has become more and more popular in recent years. Real-time mapping and positioning through low-cost cameras is also very technically difficult. Take ToF (Time of Flight), a promising depth acquisition method, as an example.
The sensor emits modulated near-infrared light, which is reflected when it encounters an object. The sensor calculates the time difference or phase difference between light emission and reflection to convert the distance of the photographed scene to generate depth information. . Similar to radar, or imagine a bat, softkinetic's DS325 uses a ToF solution (designed by TI). However, its receiver microstructure is relatively special, with two or more shutters, and can measure ps-level time differences. However, its unit pixel size is usually 100um, so the current resolution is not high.
After the depth map is obtained, the SLAM algorithm begins to work. Due to different Sensors and requirements, the presentation form of SLAM is slightly different. . It can be roughly divided into laser SLAM (also divided into 2D and 3D) and visual SLAM (also divided into Sparse, semiDense, Dense), but the main ideas are similar.
Elements of SLAM algorithm implementation
SLAM technology is very practical, but also quite difficult. In the field of autonomous driving that requires precise positioning at all times, you want to complete the implementation of SLAM It is also full of difficulties. Generally speaking, the SLAM algorithm mainly considers the following four aspects when implementing it:
1. Map representation issues, such as dense and sparse are different expressions of it. This needs to be based on Actual scene needs to make a choice;
2. Information perception problem, you need to consider how to comprehensively perceive the environment. RGBD camera FOV is usually smaller, but lidar is larger;
3. Data association issues. Different sensors have different data types, timestamps, and coordinate system expressions, which need to be handled uniformly;
4. Positioning and composition problems refer to how to achieve pose estimation and modeling, which involves many mathematical problems, physical model establishment, state estimation and optimization; others include loop detection problems, exploration problems (exploration), and kidnapping problems (kidnapping).
The currently popular visual SLAM framework mainly includes front-end and back-end:
Front-end
#The front-end is equivalent to VO (visual odometry), which studies the transformation relationship between frames.
First extract the feature points of each frame image, use adjacent frame images to match the feature points, then use RANSAC to remove large noise, and then perform matching to obtain a pose information (position and attitude ), and at the same time, the attitude information provided by the IMU (Inertial Measurement Unit) can be used for filtering and fusion. The back-end is mainly to optimize the front-end results, using filtering theory (EKF, UKF, PF), or optimization theory TORO, G2O Perform tree or graph optimization. Finally, the optimal pose estimate is obtained.
Backend
The backend has more difficulties and involves more mathematical knowledge. Generally speaking, everyone has slowly abandoned the traditional filtering theory and moved towards graph optimization.
Because based on the filtering theory, the filter stability increases too fast, which puts a lot of pressure on the PF for EKF (Extended Kalman Filter) that requires frequent inversion.
Graph-based SLAM is usually based on keyframes to establish multiple nodes and relative transformation relationships between nodes, such as affine transformation matrices, and continuously Maintenance of key nodes is carried out to ensure the capacity of the graph and reduce the amount of calculation while ensuring accuracy.
The future application of SLAM in the field of autonomous driving
Slam technology has achieved good implementation effects and achievements in many fields. Including indoor mobile robots, AR scenes, drones, etc. In the field of autonomous driving, SLAM technology has not received much attention. On the one hand, because most of the current autonomous driving industry is solved through RTK, too many resources will not be invested in in-depth research. On the other hand, SLAM technology has not received much attention. On the one hand, it is also because the current technology is not yet mature. In a life-related field such as autonomous driving, any new technology must pass the test of time before it can be accepted.
In the future, as sensor accuracy gradually improves, SLAM will also show its talents in the field of autonomous driving, with its low cost and high performance. Robustness will bring revolutionary changes to autonomous driving. As SLAM technology becomes more and more popular, more and more positioning talents will flood into the field of autonomous driving, injecting fresh blood into autonomous driving and bringing new technical directions and research areas.
The above is the detailed content of An article discussing the application of SLAM technology in autonomous driving. For more information, please follow other related articles on the PHP Chinese website!

Hot AI Tools

Undresser.AI Undress
AI-powered app for creating realistic nude photos

AI Clothes Remover
Online AI tool for removing clothes from photos.

Undress AI Tool
Undress images for free

Clothoff.io
AI clothes remover

AI Hentai Generator
Generate AI Hentai for free.

Hot Article

Hot Tools

Notepad++7.3.1
Easy-to-use and free code editor

SublimeText3 Chinese version
Chinese version, very easy to use

Zend Studio 13.0.1
Powerful PHP integrated development environment

Dreamweaver CS6
Visual web development tools

SublimeText3 Mac version
God-level code editing software (SublimeText3)

Hot Topics


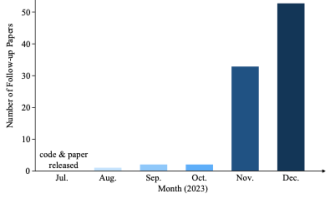
Written above & the author’s personal understanding Three-dimensional Gaussiansplatting (3DGS) is a transformative technology that has emerged in the fields of explicit radiation fields and computer graphics in recent years. This innovative method is characterized by the use of millions of 3D Gaussians, which is very different from the neural radiation field (NeRF) method, which mainly uses an implicit coordinate-based model to map spatial coordinates to pixel values. With its explicit scene representation and differentiable rendering algorithms, 3DGS not only guarantees real-time rendering capabilities, but also introduces an unprecedented level of control and scene editing. This positions 3DGS as a potential game-changer for next-generation 3D reconstruction and representation. To this end, we provide a systematic overview of the latest developments and concerns in the field of 3DGS for the first time.
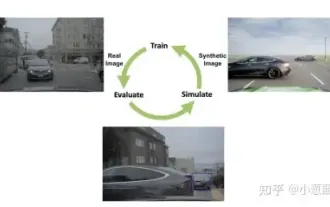
Yesterday during the interview, I was asked whether I had done any long-tail related questions, so I thought I would give a brief summary. The long-tail problem of autonomous driving refers to edge cases in autonomous vehicles, that is, possible scenarios with a low probability of occurrence. The perceived long-tail problem is one of the main reasons currently limiting the operational design domain of single-vehicle intelligent autonomous vehicles. The underlying architecture and most technical issues of autonomous driving have been solved, and the remaining 5% of long-tail problems have gradually become the key to restricting the development of autonomous driving. These problems include a variety of fragmented scenarios, extreme situations, and unpredictable human behavior. The "long tail" of edge scenarios in autonomous driving refers to edge cases in autonomous vehicles (AVs). Edge cases are possible scenarios with a low probability of occurrence. these rare events
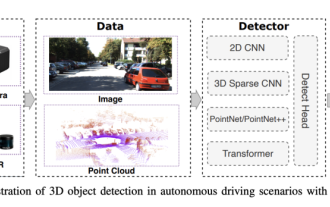
0.Written in front&& Personal understanding that autonomous driving systems rely on advanced perception, decision-making and control technologies, by using various sensors (such as cameras, lidar, radar, etc.) to perceive the surrounding environment, and using algorithms and models for real-time analysis and decision-making. This enables vehicles to recognize road signs, detect and track other vehicles, predict pedestrian behavior, etc., thereby safely operating and adapting to complex traffic environments. This technology is currently attracting widespread attention and is considered an important development area in the future of transportation. one. But what makes autonomous driving difficult is figuring out how to make the car understand what's going on around it. This requires that the three-dimensional object detection algorithm in the autonomous driving system can accurately perceive and describe objects in the surrounding environment, including their locations,
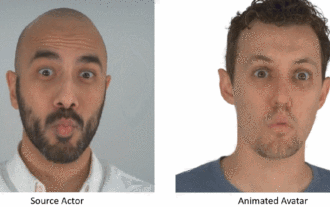
With such a powerful AI imitation ability, it is really impossible to prevent it. It is completely impossible to prevent it. Has the development of AI reached this level now? Your front foot makes your facial features fly, and on your back foot, the exact same expression is reproduced. Staring, raising eyebrows, pouting, no matter how exaggerated the expression is, it is all imitated perfectly. Increase the difficulty, raise the eyebrows higher, open the eyes wider, and even the mouth shape is crooked, and the virtual character avatar can perfectly reproduce the expression. When you adjust the parameters on the left, the virtual avatar on the right will also change its movements accordingly to give a close-up of the mouth and eyes. The imitation cannot be said to be exactly the same, but the expression is exactly the same (far right). The research comes from institutions such as the Technical University of Munich, which proposes GaussianAvatars, which
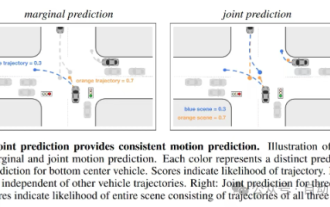
Trajectory prediction plays an important role in autonomous driving. Autonomous driving trajectory prediction refers to predicting the future driving trajectory of the vehicle by analyzing various data during the vehicle's driving process. As the core module of autonomous driving, the quality of trajectory prediction is crucial to downstream planning control. The trajectory prediction task has a rich technology stack and requires familiarity with autonomous driving dynamic/static perception, high-precision maps, lane lines, neural network architecture (CNN&GNN&Transformer) skills, etc. It is very difficult to get started! Many fans hope to get started with trajectory prediction as soon as possible and avoid pitfalls. Today I will take stock of some common problems and introductory learning methods for trajectory prediction! Introductory related knowledge 1. Are the preview papers in order? A: Look at the survey first, p

Original title: SIMPL: ASimpleandEfficientMulti-agentMotionPredictionBaselineforAutonomousDriving Paper link: https://arxiv.org/pdf/2402.02519.pdf Code link: https://github.com/HKUST-Aerial-Robotics/SIMPL Author unit: Hong Kong University of Science and Technology DJI Paper idea: This paper proposes a simple and efficient motion prediction baseline (SIMPL) for autonomous vehicles. Compared with traditional agent-cent
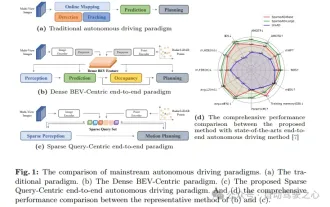
Written in front & starting point The end-to-end paradigm uses a unified framework to achieve multi-tasking in autonomous driving systems. Despite the simplicity and clarity of this paradigm, the performance of end-to-end autonomous driving methods on subtasks still lags far behind single-task methods. At the same time, the dense bird's-eye view (BEV) features widely used in previous end-to-end methods make it difficult to scale to more modalities or tasks. A sparse search-centric end-to-end autonomous driving paradigm (SparseAD) is proposed here, in which sparse search fully represents the entire driving scenario, including space, time, and tasks, without any dense BEV representation. Specifically, a unified sparse architecture is designed for task awareness including detection, tracking, and online mapping. In addition, heavy
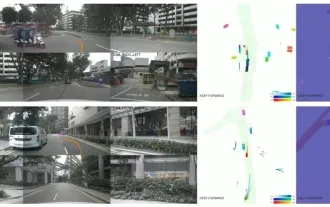
In the past month, due to some well-known reasons, I have had very intensive exchanges with various teachers and classmates in the industry. An inevitable topic in the exchange is naturally end-to-end and the popular Tesla FSDV12. I would like to take this opportunity to sort out some of my thoughts and opinions at this moment for your reference and discussion. How to define an end-to-end autonomous driving system, and what problems should be expected to be solved end-to-end? According to the most traditional definition, an end-to-end system refers to a system that inputs raw information from sensors and directly outputs variables of concern to the task. For example, in image recognition, CNN can be called end-to-end compared to the traditional feature extractor + classifier method. In autonomous driving tasks, input data from various sensors (camera/LiDAR
