


GitHub open source 130+Stars: teach you step by step to reproduce the target detection algorithm based on the PPYOLO series
Object detection is a basic task in the field of computer vision. How can we do it without a suitable Model Zoo?
Today I will give you a simple and easy-to-use target detection algorithm model library miemiedetection. It has currently gained 130 stars on GitHub
Code link: https ://github.com/miemie2013/miemiedetection
miemiedetection is a personal detection library developed based on YOLOX. It also supports PPYOLO, PPYOLOv2, PPYOLOE, FCOS and other algorithms.
Thanks to the excellent architecture of YOLOX, the algorithm training speed in miemiedetection is very fast, and data reading is no longer the bottleneck of training speed.
The deep learning framework used in code development is pyTorch, which implements deformable convolution DCNv2, Matrix NMS and other difficult operators, and supports single-machine single-card, single-machine multi-card, and multi-machine multi-card training modes. (Linux system is recommended for multi-card training mode), supports Windows and Linux systems.
And because miemiedetection is a detection library that does not require installation, users can directly change its code to change the execution logic, so it is also easy to add new algorithms to the library.
The author stated that more algorithm support (and women’s clothing) will be added in the future.
The algorithm is guaranteed to be genuine
The most important thing to reproduce the model is that the accuracy rate should be basically the same as the original one.
Let’s first look at the three models of PPYOLO, PPYOLOv2, and PPYOLOE. The author has all undergone experiments on loss alignment and gradient alignment.
In order to preserve evidence, you can also see the commented out reading and writing *.npz parts in the source code, which are all left over from the alignment experiment. code.
And the author also recorded the process of performance alignment in detail. For novices, following this path is also a good learning process!
All training logs are also recorded and stored in the warehouse, which is enough to prove the correctness of reproducing the PPYOLO series algorithms!
The final training results show that the reproduced PPYOLO algorithm has the same loss and gradient as the original warehouse.
In addition, the author also tried to use the original warehouse and miemiedetection transfer learning voc2012 data set, and also obtained the same accuracy (using the same hyperparameters).
The same as the original implementation, using the same learning rate, the same learning rate decay strategy warm_piecewisedecay (used by PPYOLO and PPYOLOv2) and warm_cosinedecay (used by PPYOLOE), and the same exponential moving average EMA , the same data preprocessing method, the same parameter L2 weight attenuation, the same loss, the same gradient, the same pre-training model, transfer learning has obtained the same accuracy.
We have done enough experiments and done a lot of testing to ensure that everyone has a wonderful experience!
No 998 or 98, just click star and take home all the target detection algorithms for free!
Model download and conversion
If you want to run through the model, the parameters are very important. The author provides the converted pre-training pth weight file, which can be downloaded directly through Baidu Netdisk .
Link: https://pan.baidu.com/s/1ehEqnNYKb9Nz0XNeqAcwDw
Extraction code: qe3i
Or follow the steps below to obtain:
The first step is to download the weight file and execute it in the project root directory (that is, download the file. Windows users can use Thunder or browser to download wget. Link, here to show the beauty, only ppyoloe_crn_l_300e_coco is used as an example):
## Note that the model with the word pretrained is pre-trained on ImageNet Backbone network, PPYOLO, PPYOLOv2, PPYOLOE load these weights to train the COCO data set. The rest are pre-trained models on COCO.
The second step, convert the weight, execute in the project root directory:
The meaning of each parameter is:
- -f represents the configuration file used;
- -c represents the read source weight file;
- -oc represents the output (saved) pytorch weight file;
- -nc represents the number of categories in the data set;
- --only_backbone means only converting the weight of the backbone network when it is True ;
After execution, the converted *.pth weight file will be obtained in the project root directory.
Step-by-step tutorial
Most of the following commands will use the model's configuration file, so it is necessary to explain the configuration file in detail at the beginning.
mmdet.exp.base_exp.BaseExp is the configuration file base class. It is an abstract class that declares a bunch of abstract methods, such as get_model() indicating how to obtain the model, and get_data_loader() indicating how to obtain the model. How to obtain the trained dataloader, get_optimizer() indicates how to obtain the optimizer, etc.
mmdet.exp.datasets.coco_base.COCOBaseExp is the configuration of the data set and inherits BaseExp. It only gives the configuration of the data set. This warehouse only supports training of data sets in COCO annotation format!
Datasets in other annotation formats need to be converted into COCO annotation format before training (if too many annotation formats are supported, the workload will be too large). Customized data sets can be converted into COCO label format through miemieLabels. All detection algorithm configuration classes will inherit COCOBaseExp, which means that all detection algorithms share the same data set configuration.
The configuration items of COCOBaseExp are:
Among them,
- self.num_classes represents the number of categories in the data set;
- self.data_dir represents the root directory of the data set;
- self.cls_names represents the category name file path of the data set. It is a txt file, and one line represents a category name. If it is a custom data set, you need to create a new txt file and edit the category name, and then modify self.cls_names to point to it;
- self.ann_folder represents the annotation file of the data set The root directory needs to be located in the self.data_dir directory;
- self.train_ann represents the annotation file name of the training set of the data set and needs to be located in the self.ann_folder directory;
- self.val_ann represents the annotation file name of the verification set of the data set, which needs to be located in the self.ann_folder directory;
- self. train_image_folder represents the image folder name of the training set of the data set, which needs to be located in the self.data_dir directory;
- self.val_image_folder represents the image file of the verification set of the data set The folder name needs to be located in the self.data_dir directory;
For the VOC 2012 data set, you need to modify the configuration of the data set to:
In addition, you can also modify the configuration of self.num_classes and self.data_dir in the subclass as in exps/ppyoloe/ppyoloe_crn_l_voc2012.py, so that the configuration of COCOBaseExp will be overwritten. It's gone (invalid).
After downloading the previously mentioned model, create a new folder annotations2 in the self.data_dir directory of the VOC2012 data set, and put voc2012_train.json and voc2012_val.json into this file folder.
Finally, the placement locations of the COCO data set, VOC2012 data set, and this project should be like this:
The data set root directory and miemiedetection-master are the same level directory. I personally do not recommend putting the data set in miemiedetection-master, otherwise PyCharm will be extremely laggy when opening it; moreover, when multiple projects (such as mmdetection, PaddleDetection, AdelaiDet) share data sets, you can set the data set path and project The name doesn't matter.
mmdet.exp.ppyolo.ppyolo_method_base.PPYOLO_Method_Exp is a class that implements all abstract methods of specific algorithms. It inherits COCOBaseExp, which implements all abstract methods.
exp.ppyolo.ppyolo_r50vd_2x.Exp is the final configuration class of the Resnet50Vd model of the PPYOLO algorithm, which inherits PPYOLO_Method_Exp;
#PPYOLOE configuration file It is also a similar structure.
Prediction
First, if the input data is a picture, execute it in the project root directory:
The meaning of each parameter is:
- -f represents the configuration file used;
- -c represents is the weight file read;
- --path represents the path of the image;
- --conf represents the score Threshold, only prediction boxes higher than this threshold will be drawn;
- --tsize represents the resolution of resize the image to --tsize during prediction;
After the prediction is completed, the console will print the saving path of the result image, which the user can open and view. If you are using a model saved in a training custom data set for prediction, just modify -c to the path of your model.
If the prediction is for all pictures in a folder, execute it in the project root directory:
Change --path to the path of the corresponding image folder.
Training COCO2017 data set
If you read the ImageNet pre-training backbone network training COCO data set, execute it in the project root directory:
One command directly starts the single-machine eight-card training. Of course, the premise is that you really have a single-machine eight-card supercomputer.
The meaning of each parameter is:
-f represents the configuration file used;
-d represents the number of graphics cards;
-b represents the batch size during training (for all cards);
-eb represents the batch size during evaluation (for all cards);
-c represents the read weight file;
--fp16, automatic mixed precision training;
--num_machines, the number of machines, it is recommended to train with multiple cards on a single machine;
-- resume indicates whether to resume training;
Train custom data set
It is recommended to read COCO pre-training weights for training because the convergence is fast.
Take the above VOC2012 data set as an example. For the ppyolo_r50vd model, if it is 1 machine and 1 card, enter the following command to start training:
If training is interrupted for some reason and you want to read the previously saved model to resume training, just modify -c to the path to the model you want to read, and add the --resume parameter. Can.
If it is 2 machines and 2 cards, that is, 1 card on each machine, enter the following command on machine 0:
And enter the following command on machine 1:
You only need to change 192.168.0.107 in the above two commands to 0 The LAN IP of the machine is enough.
If it is 1 machine and 2 cards, enter the following command to start training:
Transfer learning VOC2012 data set, the measured AP (0.50:0.95) of ppyolo_r50vd_2x can reach 0.59, AP (0.50) can reach 0.82, and AP (small) can reach 0.18. Regardless of whether it is a single card or multiple cards, this result can be obtained.
During transfer learning, it has the same accuracy and convergence speed as PaddleDetection. The training logs of both are located in the train_ppyolo_in_voc2012 folder.
If it is the ppyoloe_l model, enter the following command on a single machine to start training (the backbone network is frozen)
Transfer learning VOC2012 data set, the measured AP (0.50:0.95) of ppyoloe_l can reach 0.66, AP (0.50) can reach 0.85, and AP (small) can reach 0.28.
Evaluation
The commands and specific parameters are as follows.
The result of running in the project root directory is:
The accuracy after converting the weights is A little loss, about 0.4%.
The above is the detailed content of GitHub open source 130+Stars: teach you step by step to reproduce the target detection algorithm based on the PPYOLO series. For more information, please follow other related articles on the PHP Chinese website!

Hot AI Tools

Undresser.AI Undress
AI-powered app for creating realistic nude photos

AI Clothes Remover
Online AI tool for removing clothes from photos.

Undress AI Tool
Undress images for free

Clothoff.io
AI clothes remover

AI Hentai Generator
Generate AI Hentai for free.

Hot Article

Hot Tools

Notepad++7.3.1
Easy-to-use and free code editor

SublimeText3 Chinese version
Chinese version, very easy to use

Zend Studio 13.0.1
Powerful PHP integrated development environment

Dreamweaver CS6
Visual web development tools

SublimeText3 Mac version
God-level code editing software (SublimeText3)

Hot Topics


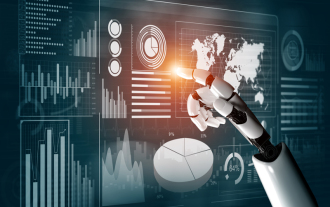
Text annotation is the work of corresponding labels or tags to specific content in text. Its main purpose is to provide additional information to the text for deeper analysis and processing, especially in the field of artificial intelligence. Text annotation is crucial for supervised machine learning tasks in artificial intelligence applications. It is used to train AI models to help more accurately understand natural language text information and improve the performance of tasks such as text classification, sentiment analysis, and language translation. Through text annotation, we can teach AI models to recognize entities in text, understand context, and make accurate predictions when new similar data appears. This article mainly recommends some better open source text annotation tools. 1.LabelStudiohttps://github.com/Hu
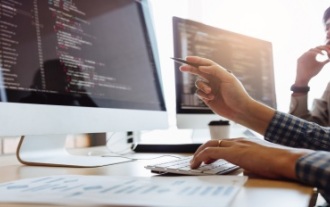
Image annotation is the process of associating labels or descriptive information with images to give deeper meaning and explanation to the image content. This process is critical to machine learning, which helps train vision models to more accurately identify individual elements in images. By adding annotations to images, the computer can understand the semantics and context behind the images, thereby improving the ability to understand and analyze the image content. Image annotation has a wide range of applications, covering many fields, such as computer vision, natural language processing, and graph vision models. It has a wide range of applications, such as assisting vehicles in identifying obstacles on the road, and helping in the detection and diagnosis of diseases through medical image recognition. . This article mainly recommends some better open source and free image annotation tools. 1.Makesens
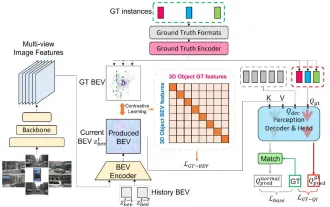
Written above & the author’s personal understanding: At present, in the entire autonomous driving system, the perception module plays a vital role. The autonomous vehicle driving on the road can only obtain accurate perception results through the perception module. The downstream regulation and control module in the autonomous driving system makes timely and correct judgments and behavioral decisions. Currently, cars with autonomous driving functions are usually equipped with a variety of data information sensors including surround-view camera sensors, lidar sensors, and millimeter-wave radar sensors to collect information in different modalities to achieve accurate perception tasks. The BEV perception algorithm based on pure vision is favored by the industry because of its low hardware cost and easy deployment, and its output results can be easily applied to various downstream tasks.
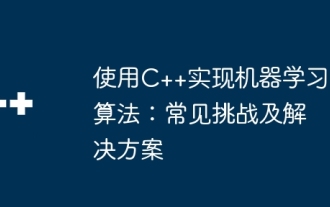
Common challenges faced by machine learning algorithms in C++ include memory management, multi-threading, performance optimization, and maintainability. Solutions include using smart pointers, modern threading libraries, SIMD instructions and third-party libraries, as well as following coding style guidelines and using automation tools. Practical cases show how to use the Eigen library to implement linear regression algorithms, effectively manage memory and use high-performance matrix operations.
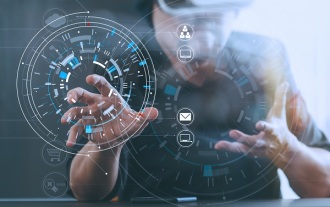
Face detection and recognition technology is already a relatively mature and widely used technology. Currently, the most widely used Internet application language is JS. Implementing face detection and recognition on the Web front-end has advantages and disadvantages compared to back-end face recognition. Advantages include reducing network interaction and real-time recognition, which greatly shortens user waiting time and improves user experience; disadvantages include: being limited by model size, the accuracy is also limited. How to use js to implement face detection on the web? In order to implement face recognition on the Web, you need to be familiar with related programming languages and technologies, such as JavaScript, HTML, CSS, WebRTC, etc. At the same time, you also need to master relevant computer vision and artificial intelligence technologies. It is worth noting that due to the design of the Web side
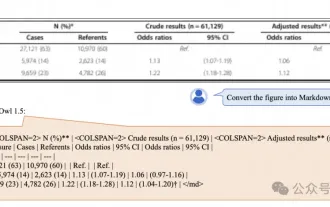
New SOTA for multimodal document understanding capabilities! Alibaba's mPLUG team released the latest open source work mPLUG-DocOwl1.5, which proposed a series of solutions to address the four major challenges of high-resolution image text recognition, general document structure understanding, instruction following, and introduction of external knowledge. Without further ado, let’s look at the effects first. One-click recognition and conversion of charts with complex structures into Markdown format: Charts of different styles are available: More detailed text recognition and positioning can also be easily handled: Detailed explanations of document understanding can also be given: You know, "Document Understanding" is currently An important scenario for the implementation of large language models. There are many products on the market to assist document reading. Some of them mainly use OCR systems for text recognition and cooperate with LLM for text processing.
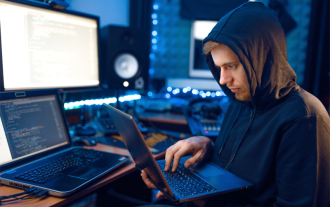
Let me introduce to you the latest AIGC open source project-AnimagineXL3.1. This project is the latest iteration of the anime-themed text-to-image model, aiming to provide users with a more optimized and powerful anime image generation experience. In AnimagineXL3.1, the development team focused on optimizing several key aspects to ensure that the model reaches new heights in performance and functionality. First, they expanded the training data to include not only game character data from previous versions, but also data from many other well-known anime series into the training set. This move enriches the model's knowledge base, allowing it to more fully understand various anime styles and characters. AnimagineXL3.1 introduces a new set of special tags and aesthetics
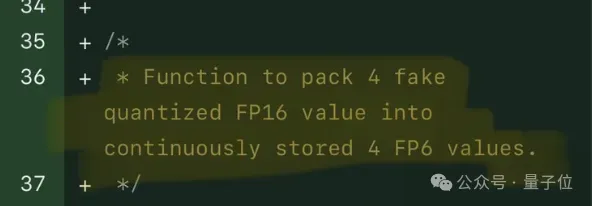
FP8 and lower floating point quantification precision are no longer the "patent" of H100! Lao Huang wanted everyone to use INT8/INT4, and the Microsoft DeepSpeed team started running FP6 on A100 without official support from NVIDIA. Test results show that the new method TC-FPx's FP6 quantization on A100 is close to or occasionally faster than INT4, and has higher accuracy than the latter. On top of this, there is also end-to-end large model support, which has been open sourced and integrated into deep learning inference frameworks such as DeepSpeed. This result also has an immediate effect on accelerating large models - under this framework, using a single card to run Llama, the throughput is 2.65 times higher than that of dual cards. one
