


Multimodal image synthesis and editing are so popular that the Max Planck Institute, Nanyang Technological Institute and others have published a detailed review
The recent DALLE-2 released by OpenAI and Imagen released by Google have achieved stunning text-to-image generation effects, which have attracted widespread attention and spawned many interesting applications. Text-to-image generation is a typical task in the field of multi-modal image synthesis and editing. Recently, researchers from Max Planck Institute, Nanyang Technological Institute and other institutions conducted a detailed investigation and analysis on the research status and future development of the large field of multi-modal image synthesis and editing.
- Paper address: https://arxiv.org/pdf/2112.13592 .pdf
- Project address: https://github.com/fnzhan/MISE
#In the first chapter, this review describes the significance and overall development of multi-modal image synthesis and editing tasks, as well as the contributions of this paper and The overall structure.
In the second chapter, based on the data modalities that guide image synthesis and editing, this review paper introduces the more commonly used visual guidance (such as semantic maps, key point maps, edge maps ), text guidance, voice guidance, scene graph guidance and corresponding modal data processing methods and a unified representation framework.
In the third chapter, according to the model framework of image synthesis and editing, the paper classifies various current methods, including GAN-based methods, autoregressive methods, diffusion model method, and neural radiation field (NeRF) method.
##Since GAN-based methods generally use conditional GAN and unconditional GAN inversion, this paper will One category is further divided into intra-modal conditions (e.g. semantic map, edge map), cross-modal conditions (e.g. text and speech), and GAN inversion (unified modality) and described in detail.
Compared with GAN-based methods, the autoregressive model method can process multi-modal data more naturally and utilize the currently popular Transformer model. . Autoregressive methods generally first learn a vector quantization encoder to discretely represent images as token sequences, and then autoregressively model the distribution of tokens. Since data such as text and speech can be represented as tokens and used as conditions for autoregressive modeling, various multi-modal image synthesis and editing tasks can be unified into a single framework.
The above methods mainly focus on multi-modal synthesis and editing of 2D images. With the recent rapid development of Neural Radiation Fields (NeRF), multi-modal synthesis and editing for 3D perception have attracted more and more attention. Multimodal synthesis and editing for 3D perception is a more challenging task due to the need to consider multi-view consistency. This paper classifies and summarizes the existing work on three methods of single-scene optimization NeRF, generative NeRF and NeRF inversion.
Subsequently, this review compares and discusses the above four model methods. Overall, current state-of-the-art models favor autoregressive and diffusion models over GANs. The application of NeRF in multi-modal synthesis and editing tasks opens a new window for research in this field.
In Chapter 4, this review brings together popular data in the field of multimodal synthesis and editing Sets and corresponding modal annotations are provided, and current methods are quantitatively compared for typical tasks of each modality (semantic image synthesis, text-to-image synthesis, and voice-guided image editing).
In Chapter 5, the review discusses and analyzes the current challenges and future directions in this field, including large-scale multi-modal data sets, accurate and reliable evaluation indicators , efficient network architecture, and the development direction of 3D perception.
In Chapters 6 and 7, the review elaborates on the potential social impact of this field and summarizes the content and contributions of the article respectively.
The above is the detailed content of Multimodal image synthesis and editing are so popular that the Max Planck Institute, Nanyang Technological Institute and others have published a detailed review. For more information, please follow other related articles on the PHP Chinese website!

Hot AI Tools

Undresser.AI Undress
AI-powered app for creating realistic nude photos

AI Clothes Remover
Online AI tool for removing clothes from photos.

Undress AI Tool
Undress images for free

Clothoff.io
AI clothes remover

AI Hentai Generator
Generate AI Hentai for free.

Hot Article

Hot Tools

Notepad++7.3.1
Easy-to-use and free code editor

SublimeText3 Chinese version
Chinese version, very easy to use

Zend Studio 13.0.1
Powerful PHP integrated development environment

Dreamweaver CS6
Visual web development tools

SublimeText3 Mac version
God-level code editing software (SublimeText3)

Hot Topics
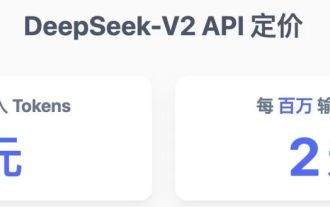
Imagine an artificial intelligence model that not only has the ability to surpass traditional computing, but also achieves more efficient performance at a lower cost. This is not science fiction, DeepSeek-V2[1], the world’s most powerful open source MoE model is here. DeepSeek-V2 is a powerful mixture of experts (MoE) language model with the characteristics of economical training and efficient inference. It consists of 236B parameters, 21B of which are used to activate each marker. Compared with DeepSeek67B, DeepSeek-V2 has stronger performance, while saving 42.5% of training costs, reducing KV cache by 93.3%, and increasing the maximum generation throughput to 5.76 times. DeepSeek is a company exploring general artificial intelligence
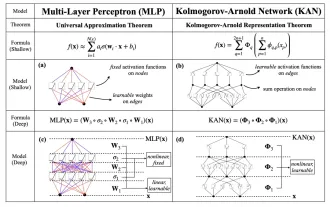
Earlier this month, researchers from MIT and other institutions proposed a very promising alternative to MLP - KAN. KAN outperforms MLP in terms of accuracy and interpretability. And it can outperform MLP running with a larger number of parameters with a very small number of parameters. For example, the authors stated that they used KAN to reproduce DeepMind's results with a smaller network and a higher degree of automation. Specifically, DeepMind's MLP has about 300,000 parameters, while KAN only has about 200 parameters. KAN has a strong mathematical foundation like MLP. MLP is based on the universal approximation theorem, while KAN is based on the Kolmogorov-Arnold representation theorem. As shown in the figure below, KAN has
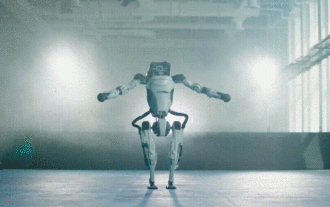
Boston Dynamics Atlas officially enters the era of electric robots! Yesterday, the hydraulic Atlas just "tearfully" withdrew from the stage of history. Today, Boston Dynamics announced that the electric Atlas is on the job. It seems that in the field of commercial humanoid robots, Boston Dynamics is determined to compete with Tesla. After the new video was released, it had already been viewed by more than one million people in just ten hours. The old people leave and new roles appear. This is a historical necessity. There is no doubt that this year is the explosive year of humanoid robots. Netizens commented: The advancement of robots has made this year's opening ceremony look like a human, and the degree of freedom is far greater than that of humans. But is this really not a horror movie? At the beginning of the video, Atlas is lying calmly on the ground, seemingly on his back. What follows is jaw-dropping
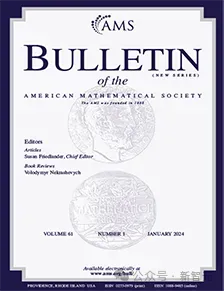
AI is indeed changing mathematics. Recently, Tao Zhexuan, who has been paying close attention to this issue, forwarded the latest issue of "Bulletin of the American Mathematical Society" (Bulletin of the American Mathematical Society). Focusing on the topic "Will machines change mathematics?", many mathematicians expressed their opinions. The whole process was full of sparks, hardcore and exciting. The author has a strong lineup, including Fields Medal winner Akshay Venkatesh, Chinese mathematician Zheng Lejun, NYU computer scientist Ernest Davis and many other well-known scholars in the industry. The world of AI has changed dramatically. You know, many of these articles were submitted a year ago.
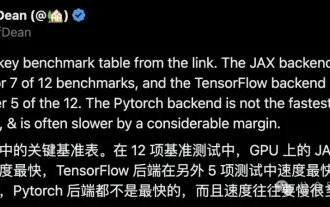
The performance of JAX, promoted by Google, has surpassed that of Pytorch and TensorFlow in recent benchmark tests, ranking first in 7 indicators. And the test was not done on the TPU with the best JAX performance. Although among developers, Pytorch is still more popular than Tensorflow. But in the future, perhaps more large models will be trained and run based on the JAX platform. Models Recently, the Keras team benchmarked three backends (TensorFlow, JAX, PyTorch) with the native PyTorch implementation and Keras2 with TensorFlow. First, they select a set of mainstream
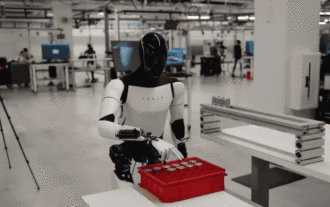
The latest video of Tesla's robot Optimus is released, and it can already work in the factory. At normal speed, it sorts batteries (Tesla's 4680 batteries) like this: The official also released what it looks like at 20x speed - on a small "workstation", picking and picking and picking: This time it is released One of the highlights of the video is that Optimus completes this work in the factory, completely autonomously, without human intervention throughout the process. And from the perspective of Optimus, it can also pick up and place the crooked battery, focusing on automatic error correction: Regarding Optimus's hand, NVIDIA scientist Jim Fan gave a high evaluation: Optimus's hand is the world's five-fingered robot. One of the most dexterous. Its hands are not only tactile
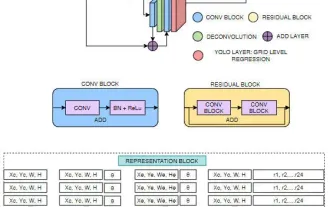
Target detection is a relatively mature problem in autonomous driving systems, among which pedestrian detection is one of the earliest algorithms to be deployed. Very comprehensive research has been carried out in most papers. However, distance perception using fisheye cameras for surround view is relatively less studied. Due to large radial distortion, standard bounding box representation is difficult to implement in fisheye cameras. To alleviate the above description, we explore extended bounding box, ellipse, and general polygon designs into polar/angular representations and define an instance segmentation mIOU metric to analyze these representations. The proposed model fisheyeDetNet with polygonal shape outperforms other models and simultaneously achieves 49.5% mAP on the Valeo fisheye camera dataset for autonomous driving
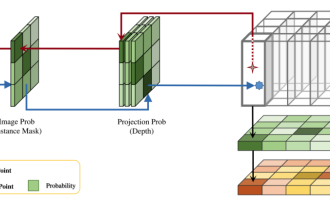
This paper explores the problem of accurately detecting objects from different viewing angles (such as perspective and bird's-eye view) in autonomous driving, especially how to effectively transform features from perspective (PV) to bird's-eye view (BEV) space. Transformation is implemented via the Visual Transformation (VT) module. Existing methods are broadly divided into two strategies: 2D to 3D and 3D to 2D conversion. 2D-to-3D methods improve dense 2D features by predicting depth probabilities, but the inherent uncertainty of depth predictions, especially in distant regions, may introduce inaccuracies. While 3D to 2D methods usually use 3D queries to sample 2D features and learn the attention weights of the correspondence between 3D and 2D features through a Transformer, which increases the computational and deployment time.
