eBay uses machine learning to improve sale listings
Translator | Bugatti
Reviewer | Sun Shujuan
The online marketplace eBay has added additional buying signals to its machine learning model, such as “add to watchlist”, “ Bid" and "Add to Cart" to increase the relevance of recommended ad listings based on the initial product being searched. Chen Xue gave a very detailed introduction in this recent article.
- Retrieve the subset candidate promotion list criteria that are most closely related to the seed item ("Check the complete collection").
- Use a trained machine learning sorter to sort the product list in the search set according to the likelihood of purchase.
- Reorder the product list based on advertising rates to balance seller sales speed achieved through promotions with recommendation relevance.
- Data of recommended products
- Recommended products similar to the seed product
- Context (Country and Product Category)
- User Personalization Features
- False Negatives: Since users typically only purchase one item from the recommended list, the purchase does not occur when the purchase is not made. In some cases, good recommendations may be viewed as bad recommendations, leading to false positives.
- Few purchases: Compared to other user events, it is becoming challenging to train a model with sufficient number and diversity of purchases to predict the forward class.
- Missing data: From clicks to add to cart, numerous user actions reveal a wealth of user information and reveal possible outcomes.
- Buy Now (only applicable At Buy-It-Now i.e. BIN Listing)
- Add to Cart (BIN Listing Only)
- Bid (Best Bid Listing Only)
- Ask for Bid (Applies to Auction Listings only)
- Add to Watchlist (Applies to BIN, Best Bid, or Auction Listings)
Sample weights for multiple correlation feedback
Gradient boosted trees support multiple labels to capture a range of correlations, but there is no direct way to achieve the magnitude of the correlation.
eBay had to run the tests iteratively until it came up with numbers that made the model work. The researchers added additional weights (called "sample weights") that were fed into the pairwise loss function. They optimized the hyperparameter tuning and ran it for 25 iterations before arriving at the best sample weights - "Add to Watchlist" (6), "Add to Cart" (15), "Bid" (38 ), "Buy Now" (8) and "Buy" (15). Without sample weights, the new model will perform worse. With sample weights, the new model outperforms the binary model.
They tried adding only clicks as additional relevant feedback and applied the tuned hyperparameter "Purchase" sample weight of 150. Offline results are also shown below, where "BOWC" stands for Buy Now, Make a Bid, Add to Watchlist, and Add to Cart actions. Purchase ranking reflects the average ranking of items purchased. The smaller the better.
Conclusion
The trained model has a total of more than 2000 instances. A/B testing is conducted in two stages. The first phase, which only included additional select tags and showed a 2.97% increase in purchase volume on the eBay mobile app and a 2.66% increase in ad revenue, was deemed successful enough to move the model into global production.
The second phase added more actions to the model, such as "Add to Watchlist", "Add to Cart", "Bid" and "Buy Now", and A/B testing showed better customer engagement (e.g. more clicks and BWC).
Original title: EBay Uses Machine Learning to Refine Promoted Listings, Author: Jessica Wachtel
The above is the detailed content of eBay uses machine learning to improve sale listings. For more information, please follow other related articles on the PHP Chinese website!

Hot AI Tools

Undresser.AI Undress
AI-powered app for creating realistic nude photos

AI Clothes Remover
Online AI tool for removing clothes from photos.

Undress AI Tool
Undress images for free

Clothoff.io
AI clothes remover

AI Hentai Generator
Generate AI Hentai for free.

Hot Article

Hot Tools

Notepad++7.3.1
Easy-to-use and free code editor

SublimeText3 Chinese version
Chinese version, very easy to use

Zend Studio 13.0.1
Powerful PHP integrated development environment

Dreamweaver CS6
Visual web development tools

SublimeText3 Mac version
God-level code editing software (SublimeText3)

Hot Topics
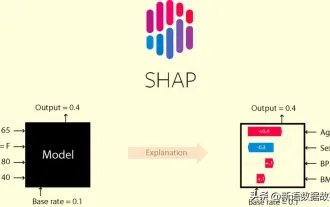
In the fields of machine learning and data science, model interpretability has always been a focus of researchers and practitioners. With the widespread application of complex models such as deep learning and ensemble methods, understanding the model's decision-making process has become particularly important. Explainable AI|XAI helps build trust and confidence in machine learning models by increasing the transparency of the model. Improving model transparency can be achieved through methods such as the widespread use of multiple complex models, as well as the decision-making processes used to explain the models. These methods include feature importance analysis, model prediction interval estimation, local interpretability algorithms, etc. Feature importance analysis can explain the decision-making process of a model by evaluating the degree of influence of the model on the input features. Model prediction interval estimate
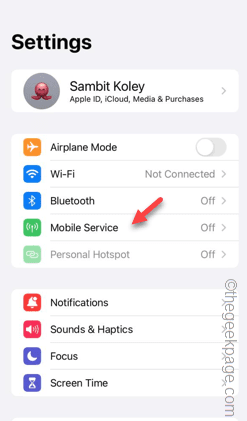
Facing lag, slow mobile data connection on iPhone? Typically, the strength of cellular internet on your phone depends on several factors such as region, cellular network type, roaming type, etc. There are some things you can do to get a faster, more reliable cellular Internet connection. Fix 1 – Force Restart iPhone Sometimes, force restarting your device just resets a lot of things, including the cellular connection. Step 1 – Just press the volume up key once and release. Next, press the Volume Down key and release it again. Step 2 – The next part of the process is to hold the button on the right side. Let the iPhone finish restarting. Enable cellular data and check network speed. Check again Fix 2 – Change data mode While 5G offers better network speeds, it works better when the signal is weaker
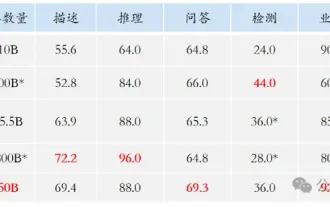
I cry to death. The world is madly building big models. The data on the Internet is not enough. It is not enough at all. The training model looks like "The Hunger Games", and AI researchers around the world are worrying about how to feed these data voracious eaters. This problem is particularly prominent in multi-modal tasks. At a time when nothing could be done, a start-up team from the Department of Renmin University of China used its own new model to become the first in China to make "model-generated data feed itself" a reality. Moreover, it is a two-pronged approach on the understanding side and the generation side. Both sides can generate high-quality, multi-modal new data and provide data feedback to the model itself. What is a model? Awaker 1.0, a large multi-modal model that just appeared on the Zhongguancun Forum. Who is the team? Sophon engine. Founded by Gao Yizhao, a doctoral student at Renmin University’s Hillhouse School of Artificial Intelligence.
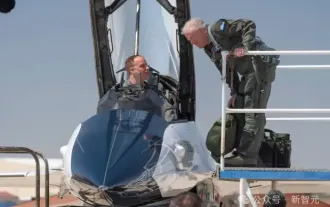
Recently, the military circle has been overwhelmed by the news: US military fighter jets can now complete fully automatic air combat using AI. Yes, just recently, the US military’s AI fighter jet was made public for the first time and the mystery was unveiled. The full name of this fighter is the Variable Stability Simulator Test Aircraft (VISTA). It was personally flown by the Secretary of the US Air Force to simulate a one-on-one air battle. On May 2, U.S. Air Force Secretary Frank Kendall took off in an X-62AVISTA at Edwards Air Force Base. Note that during the one-hour flight, all flight actions were completed autonomously by AI! Kendall said - "For the past few decades, we have been thinking about the unlimited potential of autonomous air-to-air combat, but it has always seemed out of reach." However now,
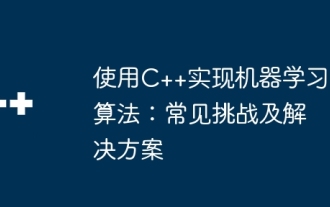
Common challenges faced by machine learning algorithms in C++ include memory management, multi-threading, performance optimization, and maintainability. Solutions include using smart pointers, modern threading libraries, SIMD instructions and third-party libraries, as well as following coding style guidelines and using automation tools. Practical cases show how to use the Eigen library to implement linear regression algorithms, effectively manage memory and use high-performance matrix operations.
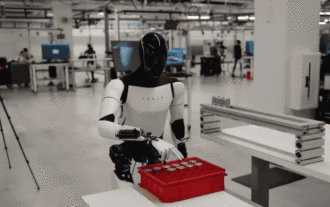
The latest video of Tesla's robot Optimus is released, and it can already work in the factory. At normal speed, it sorts batteries (Tesla's 4680 batteries) like this: The official also released what it looks like at 20x speed - on a small "workstation", picking and picking and picking: This time it is released One of the highlights of the video is that Optimus completes this work in the factory, completely autonomously, without human intervention throughout the process. And from the perspective of Optimus, it can also pick up and place the crooked battery, focusing on automatic error correction: Regarding Optimus's hand, NVIDIA scientist Jim Fan gave a high evaluation: Optimus's hand is the world's five-fingered robot. One of the most dexterous. Its hands are not only tactile
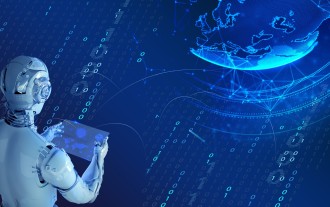
Translator | Reviewed by Li Rui | Chonglou Artificial intelligence (AI) and machine learning (ML) models are becoming increasingly complex today, and the output produced by these models is a black box – unable to be explained to stakeholders. Explainable AI (XAI) aims to solve this problem by enabling stakeholders to understand how these models work, ensuring they understand how these models actually make decisions, and ensuring transparency in AI systems, Trust and accountability to address this issue. This article explores various explainable artificial intelligence (XAI) techniques to illustrate their underlying principles. Several reasons why explainable AI is crucial Trust and transparency: For AI systems to be widely accepted and trusted, users need to understand how decisions are made
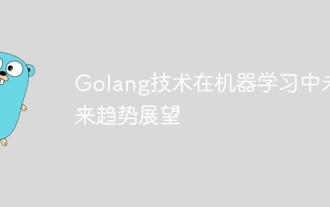
The application potential of Go language in the field of machine learning is huge. Its advantages are: Concurrency: It supports parallel programming and is suitable for computationally intensive operations in machine learning tasks. Efficiency: The garbage collector and language features ensure that the code is efficient, even when processing large data sets. Ease of use: The syntax is concise, making it easy to learn and write machine learning applications.
