


The Go AI that defeated Shen Zhenzhen as a partner, but lost to an amateur human player
A new model that even amateur chess players can't beat actually defeated the world's strongest Go AI - KataGo?
Yes, this jaw-dropping result comes from the latest papers from MIT, UC Berkeley, etc.
The researchers used adversarial attack methods to seize KataGo's blind spots, and based on this technology, a rookie-level Go program successfully defeated KataGO.
Without searching, this winning rate even reaches 99%.
After calculating this, the food chain in the Go world instantly became: amateur players > new AI > top Go AI?
Wait a minute, how does this magical new AI become so good at the same time?
Cunning attack angle
Before introducing the new AI, let us first understand the protagonist who was attacked this time-KataGo.
KataGo, currently the most powerful open source Go AI, was developed by Harvard AI researchers.
Previously, KataGo defeated the superhuman-level ELF OpenGo and Leela Zero, and even without a search engine, its level was equivalent to the top 100 professional Go players in Europe.
Shin Jin-jin, the “number one” Korean Go player who just won the Samsung Cup and achieved “four crowns in three years”, has been using KataGo for sparring.
△Picture source: Hangame
Faced with such a strong opponent, the method chosen by the researchers can be said to be overwhelming.
They found that although KataGo learned Go by playing millions of games against itself, this was still not enough to cover all possible situations.
So, this time they no longer choose self-game, but choose the confrontation attack method:
Let the attacker (adversary) and the fixed victim (victim, also known as KataGo) compete Game, use this method to train attackers.
This change allowed them to train an end-to-end adversarial policy using only 0.3% of the data used to train KataGo.
Specifically, this counter-strategy is not entirely about gaming, but rather ends the game prematurely by deceiving KataGo into a position that is favorable to the attacker.
For example, in the picture below, the attacker who controls the black stones mainly places stones in the upper right corner of the board, leaving other areas to KataGo, and also deliberately places some pieces in other areas that are easy to be cleared.
Adam Gleave, co-author of the paper, introduced:
This approach will make KataGo mistakenly think that it has won, because its territory (lower left) is much larger than that of its opponent.
But the lower left area doesn't really contribute points because there are still sunspots there, meaning it's not completely safe.
Because KataGo is overconfident in victory - thinking that if the game ends and the score is calculated, it will win - so KataGo will take the initiative to pass, and then the attacker will also pass, thus ending Game, start scoring. (Both sides pass, and the game ends)
But as analyzed by Gleave, since the black stones in KataGo's surrounding space are still alive, they are not judged as "dead stones" according to the Go referee rules. Therefore, KataGo's black stones in the surrounding space are still alive. Places with sunspots cannot be counted as effective mesh numbers.
So the final winner is not KataGo, but the attacker.
This victory is not unique. Without search, this countermeasure achieved a 99% winning rate against KataGo.
When KataGo used enough searches to approach superhuman levels, their win rate reached 50%.
Also, despite this clever strategy, the attacker model itself is not very strong at Go: in fact, it can be easily defeated by human amateurs it.
The researchers stated that the purpose of their research was to prove that even highly mature AI systems can have serious vulnerabilities by attacking an unexpected vulnerability in KataGo.
As co-author Gleave said:
(This study) highlights the need for better automated testing of AI systems to discover worst-case failure modes, not just Just testing performance under normal circumstances.
Research Team
The research team comes from MIT, UC Berkeley, etc. The co-authors of the paper are Tony Tong Wang and Adam Gleave.
Tony Tong Wang, a PhD student in computer science at MIT, has experience as an intern at NVIDIA, Genesis Therapeutics and other companies.
Adam Gleave is a doctoral student in artificial intelligence at the University of California, Berkeley. He graduated from the University of Cambridge with a master's degree and a bachelor's degree. His main research direction is the robustness of deep learning.
The link to the paper is attached at the end, interested friends can pick it up~
The link to the paper: https://arxiv.org/abs /2211.00241
Reference link: https://arstechnica.com/information-technology/2022/11/new-go-playing-trick-defeats-world-class-go-ai-but-loses-to -human-amateurs/
The above is the detailed content of The Go AI that defeated Shen Zhenzhen as a partner, but lost to an amateur human player. For more information, please follow other related articles on the PHP Chinese website!

Hot AI Tools

Undresser.AI Undress
AI-powered app for creating realistic nude photos

AI Clothes Remover
Online AI tool for removing clothes from photos.

Undress AI Tool
Undress images for free

Clothoff.io
AI clothes remover

AI Hentai Generator
Generate AI Hentai for free.

Hot Article

Hot Tools

Notepad++7.3.1
Easy-to-use and free code editor

SublimeText3 Chinese version
Chinese version, very easy to use

Zend Studio 13.0.1
Powerful PHP integrated development environment

Dreamweaver CS6
Visual web development tools

SublimeText3 Mac version
God-level code editing software (SublimeText3)

Hot Topics
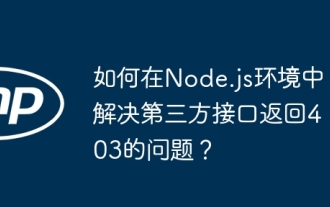
Solve the problem of third-party interface returning 403 in Node.js environment. When we use Node.js to call third-party interfaces, we sometimes encounter an error of 403 from the interface returning 403...
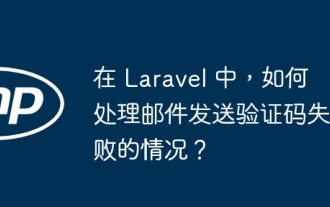
The method of handling Laravel's email failure to send verification code is to use Laravel...
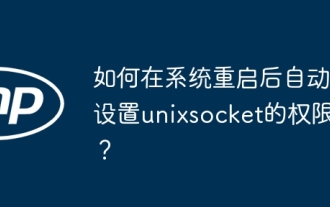
How to automatically set the permissions of unixsocket after the system restarts. Every time the system restarts, we need to execute the following command to modify the permissions of unixsocket: sudo...
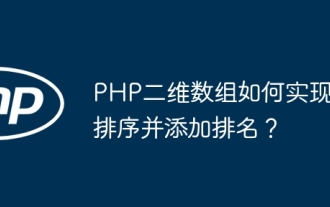
Detailed explanation of PHP two-dimensional array sorting and ranking implementation This article will explain in detail how to sort a PHP two-dimensional array and use each sub-array according to the sorting results...
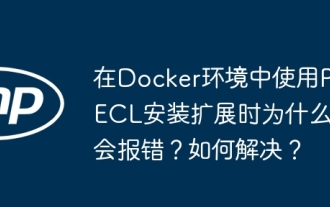
Causes and solutions for errors when using PECL to install extensions in Docker environment When using Docker environment, we often encounter some headaches...
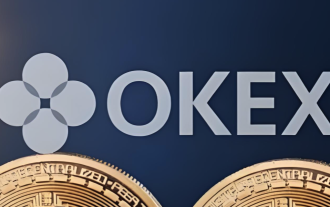
Ouyi OKX (formerly OKEx) Global Station is a world-leading digital asset service platform founded in 2017 and headquartered in Malta. It has tens of millions of users. The platform provides transactions of more than 150 currencies and has formulated a strict currency audit mechanism and market monitoring and progress tracking mechanism. Supports transactions of more than 20 mainstream legal currencies and cryptocurrencies such as the US dollar, euro, and pound.
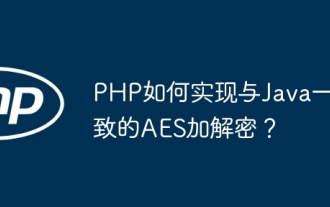
How to implement AES encryption and decryption with Java...
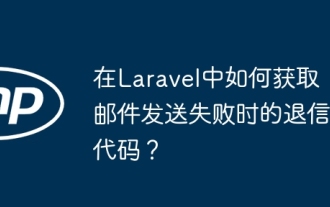
Method for obtaining the return code when Laravel email sending fails. When using Laravel to develop applications, you often encounter situations where you need to send verification codes. And in reality...
