


For the first time: Microsoft uses GPT-4 to fine-tune large model instructions, and the zero-sample performance of new tasks is further improved.
We know that from Google T5 models to OpenAI GPT series large models, large language models (LLMs) have demonstrated impressive generalization capabilities, such as context learning and thought chain reasoning. At the same time, in order to make LLMs follow natural language instructions and complete real-world tasks, researchers have been exploring instruction fine-tuning methods for LLMs. This is done in two ways: using human-annotated prompts and feedback to fine-tune models on a wide range of tasks, or using public benchmarks and datasets augmented with manually or automatically generated instructions to supervise fine-tuning.
Among these methods, Self-Instruct fine-tuning is a simple and effective method that learns from the instruction following data generated by teacher LLMs of SOTA instruction fine-tuning, making LLMs comparable to humans Intentional alignment. Facts have proven that instruction fine-tuning has become an effective means to improve the zero-sample and small-sample generalization capabilities of LLMs.
The recent success of ChatGPT and GPT-4 provides a huge opportunity to use instruction fine-tuning to improve open source LLMs. Meta LLaMA is a family of open source LLMs with performance comparable to proprietary LLMs such as GPT-3. To teach LLaMA to follow instructions, Self-Instruct was quickly adopted due to its superior performance and low cost. For example, Stanford's Alpaca model uses 52k command compliance samples generated by GPT-3.5, and the Vicuna model uses about 70k command compliance samples from ShareGPT.
In order to advance the SOTA level of LLMs instruction fine-tuning, Microsoft Research used GPT-4 as a teacher model for self-intruct fine-tuning for the first time in its paper "Instruction Tuning with GPT-4" .
- ##Paper address: https://arxiv.org/pdf/2304.03277.pdf
- Project address: https://instruction-tuning-with-gpt-4.github.io/
- GitHub address: https://github.com/Instruction-Tuning-with-GPT-4/GPT-4-LLM
On the one hand, the researchers released the data generated by GPT-4, including a 52k instruction compliance data set in Chinese and English, and feedback data generated by GPT-4 to rate the output of the three instruction fine-tuning models.
On the other hand, an instruction fine-tuning LLaMA model and a reward model were developed based on the data generated by GPT-4. To evaluate the quality of instruction fine-tuning LLMs, the researchers evaluated test samples using three metrics: manual evaluation of three alignment criteria, automatic evaluation based on GPT-4 feedback, and ROUGE-L (Automated Summarization Evaluation Method) of unnatural instructions. one).
The experimental results verify the effectiveness of fine-tuning LLMs instructions using data generated by GPT-4. The 52k Chinese and English instruction compliance data generated by GPT-4 achieves better zero-sample performance on new tasks than previous SOTA models. Currently, researchers have disclosed the data generated using GPT-4 and related code.
DatasetsThis study uses GPT-4 to generate the following four datasets:
- English Instruction-Following Data: For 52K instructions collected from Alpaca, each instruction is provided with an English GPT-4 answer. This data set is mainly used to explore and compare the statistics of GPT-4 answers and GPT-3 answers.
- Chinese Instruction-Following Data: This study used ChatGPT to translate 52K instructions into Chinese and asked GPT-4 to answer in Chinese.
- Comparison Data: Let GPT-4 rate your responses on a scale from 1 to 10. Additionally, the study asked GPT-4 to compare and score the responses of three models: GPT-4, GPT-3.5, and OPT-IML. This data set is mainly used to train reward models.
- Answers on Unnatural Instructions: GPT-4’s answers are decoded on three core datasets of 68K instructions-input-output. This subset is used to quantify the gap between GPT-4 and the instruction fine-tuning model.
Figure 1 compares the English output response sets of GPT-4 and GPT-3.5. Figure 1 (a) and (b) show two output sets of verb-noun pairs with a frequency higher than 10. Figure 1 (c) compares the 25 most frequent pairs of words in the two sets. Figure 1 (d) compares the frequency distribution of sequence lengths, and the results show that GPT-4 tends to generate longer sequences than GPT-3.5.
Instruction fine-tuning language model
This research is based on LLaMA 7B checkpoint and uses supervised fine-tuning to train two models: ( i) LLaMA-GPT4, trained on 52K English instruction compliance data generated by GPT-4. (ii) LLaMA-GPT4-CN, trained on 52K Chinese instruction follow data generated from GPT-4.
Reward Model
Reinforcement Learning with Human Feedback (RLHF) aims to align LLM behavior with human preferences, Reward modeling is one of its key parts, and the problem is often formulated as a regression task to predict the reward between a given cue and a response. However, this method usually requires large-scale comparative data. Existing open source models such as Alpaca, Vicuna, and Dolly do not involve RLHF due to the high cost of annotating comparative data. At the same time, recent research shows that GPT-4 is able to identify and repair its own errors and accurately judge the quality of responses. Therefore, to facilitate research on RLHF, this study created comparative data using GPT-4, as described above.
To evaluate data quality, the study also trained a reward model based on OPT 1.3B for evaluation on this data set. The distribution of the comparison data is shown in Figure 2 .
Experiment
The study utilized the following three types of evaluations : Human evaluation, GPT-4, and unnatural instruction evaluation. The results confirm that using data generated by GPT-4 is an efficient and effective method for fine-tuning LLM instructions compared to other machine-generated data. Next we look at the specific experimental process.
Human evaluation
Figure 3 (a) is the comparison result of LLaMA-GPT4 vs Alpaca. The experiment shows that in Under the Helpfulness indicator, GPT-4 wins with a score of 54.12%. Figure 3(b) shows the comparison results of LLaMA-GPT4 vs GPT-4, which shows that the performance of LLaMA fine-tuned by GPT-4 instructions is similar to the original GPT-4.
Compare with SOTA using automatic evaluation
The study uses GPT-4 to automatically evaluate the responses of different models on 80 unseen questions. First collect answers from two chatbots, LLaMA-GPT-4 (7B) and GPT-4, and use other chatbots to publish answers, including LLaMA (13B), Alpaca (13B), Vicuna (13B), Bard (Google, 2023) and ChatGPT. For each evaluation, the study asked GPT-4 to rate the quality of the response between the two models on a scale of 1 to 10. The results are shown in Figure 4.
Figure 4 (c,d) compares all chatbots. LLaMA_GPT4 performs better: 7B LLaMA GPT4 performs better than 13B Alpaca and LLaMA. However, LLaMA_GPT4 still has a gap compared with large commercial chatbots such as GPT-4.
The researchers further studied the performance of all chatbots in Figure 5 below. First use GPT-4 to translate the chatbot's English responses into Chinese, and then use GPT-4 to translate the English questions into Chinese to get the answers. Comparisons with GPT-4 translations and generated Chinese responses are shown in 5 (a) and 5 (b), with all model results asked to answer in Chinese shown in 5 (c).
In Figure 6 below, the researchers compare LLaMA-GPT4 with GPT-4 and Alpaca unnatural instructions. The results show that LLaMA-GPT4 and GPT-4 perform better as the ground truth response length increases. This means they can follow instructions better when the scenes are more creative. Both LLaMA-GPT4 and GPT-4 can generate responses containing simple ground truth answers when the sequence length is short, and adding extra words can make the response more chat-like.
Please refer to the original paper for more technical and experimental details.
The above is the detailed content of For the first time: Microsoft uses GPT-4 to fine-tune large model instructions, and the zero-sample performance of new tasks is further improved.. For more information, please follow other related articles on the PHP Chinese website!

Hot AI Tools

Undresser.AI Undress
AI-powered app for creating realistic nude photos

AI Clothes Remover
Online AI tool for removing clothes from photos.

Undress AI Tool
Undress images for free

Clothoff.io
AI clothes remover

Video Face Swap
Swap faces in any video effortlessly with our completely free AI face swap tool!

Hot Article

Hot Tools

Notepad++7.3.1
Easy-to-use and free code editor

SublimeText3 Chinese version
Chinese version, very easy to use

Zend Studio 13.0.1
Powerful PHP integrated development environment

Dreamweaver CS6
Visual web development tools

SublimeText3 Mac version
God-level code editing software (SublimeText3)

Hot Topics


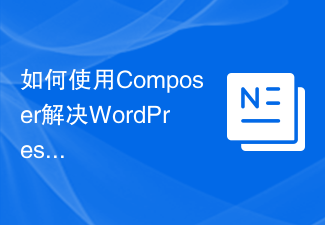
When managing WordPress websites, you often encounter complex operations such as installation, update, and multi-site conversion. These operations are not only time-consuming, but also prone to errors, causing the website to be paralyzed. Combining the WP-CLI core command with Composer can greatly simplify these tasks, improve efficiency and reliability. This article will introduce how to use Composer to solve these problems and improve the convenience of WordPress management.
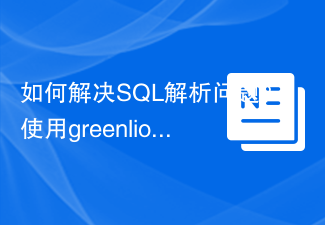
When developing a project that requires parsing SQL statements, I encountered a tricky problem: how to efficiently parse MySQL's SQL statements and extract the key information. After trying many methods, I found that the greenlion/php-sql-parser library can perfectly solve my needs.
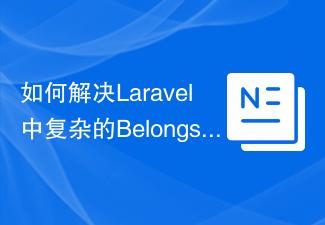
In Laravel development, dealing with complex model relationships has always been a challenge, especially when it comes to multi-level BelongsToThrough relationships. Recently, I encountered this problem in a project dealing with a multi-level model relationship, where traditional HasManyThrough relationships fail to meet the needs, resulting in data queries becoming complex and inefficient. After some exploration, I found the library staudenmeir/belongs-to-through, which easily installed and solved my troubles through Composer.
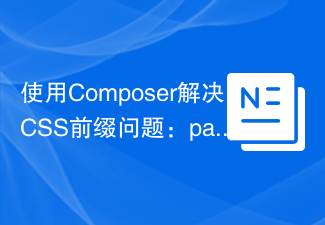
I'm having a tricky problem when developing a front-end project: I need to manually add a browser prefix to the CSS properties to ensure compatibility. This is not only time consuming, but also error-prone. After some exploration, I discovered the padaliyajay/php-autoprefixer library, which easily solved my troubles with Composer.
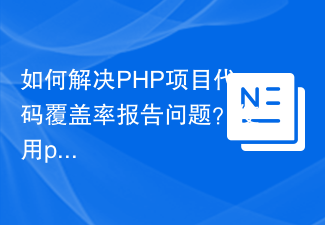
When developing PHP projects, ensuring code coverage is an important part of ensuring code quality. However, when I was using TravisCI for continuous integration, I encountered a problem: the test coverage report was not uploaded to the Coveralls platform, resulting in the inability to monitor and improve code coverage. After some exploration, I found the tool php-coveralls, which not only solved my problem, but also greatly simplified the configuration process.
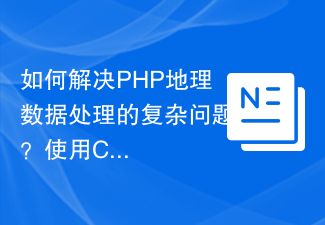
When developing a Geographic Information System (GIS), I encountered a difficult problem: how to efficiently handle various geographic data formats such as WKT, WKB, GeoJSON, etc. in PHP. I've tried multiple methods, but none of them can effectively solve the conversion and operational issues between these formats. Finally, I found the GeoPHP library, which easily integrates through Composer, and it completely solved my troubles.
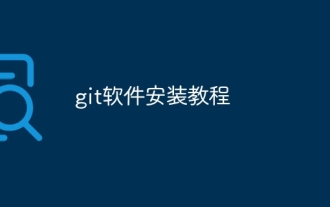
Git Software Installation Guide: Visit the official Git website to download the installer for Windows, MacOS, or Linux. Run the installer and follow the prompts. Configure Git: Set username, email, and select a text editor. For Windows users, configure the Git Bash environment.
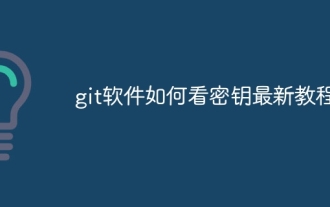
This article will explain in detail how to view keys in Git software. It is crucial to master this because Git keys are secure credentials for authentication and secure transfer of code. The article will guide readers step by step how to display and manage their Git keys, including SSH and GPG keys, using different commands and options. By following the steps in this guide, users can easily ensure their Git repository is secure and collaboratively smoothly with others.
