


Google explores a new NLU task 'Natural Language Assessment', let AI help you warm up before the formal interview!
"Question solving" can be said to run through life. Some questions can be solved alone, such as exam questions; but when it comes to interactive questions such as interviews, it is difficult for one person to solve them.
This kind of interaction is different from ordinary question and answer. It usually requires the "sparing partner" to answer questions in a specific situation and guide the user to think in order to achieve the ultimate goal.
For example, the interviewer will not only be responsible for asking questions, but also need to guide you to express your understanding of the problem and optional solutions. Such questions may also be open-ended, such as self-introduction.
The ultimate goal of AI is that everything that humans can do can be replaced by models, and this type of "interview trainer" is no exception.
But in the current field of natural language processing, this ability has not received enough attention and is technically very challenging.
Recently, Google introduced an important natural language understanding (NLU) capability, namely Natural Language Assessment (NLA), on its official blog, and discussed how it can be helpful in the context of education. .
Typical NLU tasks focus on the user’s intent, while NLA allows the answer to be evaluated from multiple perspectives.
In situations where users want to know how good their answer is, NLA can provide an analysis of how close the answer is to expectations.
In situations where there may not be a “right” answer, NLA can provide nuanced insights, including topicality, relevance, lengthy questions, and more.
The researchers developed the scope of NLA, proposed a practical model for performing topical NLA, and demonstrated how NLA can be used to help job candidates practice answering interview questions.
Overview of Natural Language Evaluation
The goal of NLA is to evaluate the answers given by users against a set of expectations.
For example, there is an NLA system that interacts with students. It has the following components:
- Ask a question to the student;
- Expectations define user expectations What to get in the answer. For example, a specific text answer or a set of topics that the user expects the answer to cover, and the answer needs to be concise.
- Answers provided by students;
- Evaluation results. Including correctness, missing information, being too specific or general, stylistic feedback, pronunciation, etc.
- Optional: context. For example, a paragraph in a book or article.
With NLA, both expectations for answers and evaluation of answers can be very broad, which makes interactions between teachers and students more expressive and detailed.
Questions with Specific Correct Answers
Even in cases where there is a definite correct answer, the answer can be evaluated with more nuance than simply correct or incorrect.
- Context: Harry Potter and the Philosopher's Stone
- Question: What is Hogwarts?
- Expectation: Hogwarts is a school of magic
- Answer: I'm not sure, but I think it is a school.
For the question and answer system, the above answer may be marked as incorrect due to the lack of key details "magic", because the user will think that the answer is not completely correct and does not make much sense.
NLA can provide more detailed understanding, such as determining that a student's answer is too general and the student is not confident enough about the answer.
This nuanced assessment, along with noticing the uncertainty students express, is important in helping students build skills in conversational settings.
TOPIC EXPECTATIONS
In many cases, the questioner does not expect a specific answer.
For example, if a student is asked an opinion question and there is no specific text expectation, the questioner is more concerned with the relevance and opinion of the answer. Perhaps the simplicity and fluency of the answer are also the questioner. within the scope of assessment.
- Question: Please introduce yourself. (Tell me a little about yourself?)
- Expectation: A collection of topics, which may include "education", "experience", "interest", etc.
- Answer: I am in Salina, California Si grew up and went to Stanford and majored in economics, but then got excited about the tech industry, so next I...
In this case, a useful evaluation output The user's answers will be mapped to a subset of the topics covered, possibly with markers for which parts of the text relate to which topic.
This is challenging from a natural language processing perspective because the answers can be long, the topics can be mixed, and each topic itself can be multifaceted.
Topic NLA model
In principle, topic NLA (Topicallity NLA) is a standard multi-classification task, and developers can easily train a classifier based on commonly used models.
But for NLA, there is very little training data available, and collecting training data for each question and topic is very costly and time-consuming.
Google’s solution is to decompose each topic into fine-grained components that can be identified using large language models (LLM) and subjected to simple general tuning.
The researchers mapped each topic to a list of potential questions and defined that if a sentence contained an answer to one of these potential questions, then it covered that topic.
For the topic of Experience, the model can choose some potential questions, such as:
- Where do you work?
- What did you study?
- ...
Under the topic of Interests, there are also some basic questions, such as
- What are you interested in?
- What do you like to do?
- ...
These basic questions are designed through an iterative manual process.
Importantly, because these questions are fine-grained enough, current language models can capture the semantics within these sentences (such as the difference between What and Where), and also allow developers to provide solutions for NLA subject tasks. A zero-shot setup: After the model is trained once, new questions and new topics can be continuously added, or existing topics can be expected to be adapted by modifying the basic content without collecting topic-specific data.
Help job seekers prepare for interviews
In order to explore the application scenarios of NLA, Google developers also worked with job seekers to develop a new tool, Interview Warmup. Help users prepare for interviews in fast-growing employment fields such as IT Support and User Experience Design.
The website provides a large number of questions so that job seekers can practice answering questions from industry experts at home to help them become more confident and comfortable in real-person interviews.
Google was also inspired by job seekers and came up with NLA research after understanding the difficulties in the interview process.
Interview Warmup does not rate or judge answers. It only provides users with an environment to practice alone and helps users improve themselves.
Every time a user answers an interview question, the answer is parsed sentence by sentence by the NLA model, and the user can then switch between different talking points to see which ones were found in their answer.
The researchers realized that there are many potential pitfalls when it comes to signaling to users that their feedback is "good," especially when the model only detects a limited set of topics.
Instead, the system puts the control in the hands of the user, using only machine learning to help users discover how to improve.
So far, the tool has helped a large number of job seekers from all over the world with great results, and the development team has recently expanded it to Africa, And plans to continue working with job seekers, iterating and making the tool more helpful to the millions of people looking for new jobs.
Natural Language Assessment (NLA) is a technically challenging and interesting research area.
NLA paves the way for new conversational applications that advance learning through nuanced evaluation and analysis of answers from multiple perspectives.
By working with the community, from job seekers and businesses to classroom teachers and students, NLA can identify situations where NLA has the potential to help users learn, engage and develop skills across a variety of subjects, in a responsible way Build applications that enable users to assess their abilities and find ways to improve.
Reference: https://ai.googleblog.com/2022/10/natural-language-assessment-new.html
The above is the detailed content of Google explores a new NLU task 'Natural Language Assessment', let AI help you warm up before the formal interview!. For more information, please follow other related articles on the PHP Chinese website!

Hot AI Tools

Undresser.AI Undress
AI-powered app for creating realistic nude photos

AI Clothes Remover
Online AI tool for removing clothes from photos.

Undress AI Tool
Undress images for free

Clothoff.io
AI clothes remover

Video Face Swap
Swap faces in any video effortlessly with our completely free AI face swap tool!

Hot Article

Hot Tools

Notepad++7.3.1
Easy-to-use and free code editor

SublimeText3 Chinese version
Chinese version, very easy to use

Zend Studio 13.0.1
Powerful PHP integrated development environment

Dreamweaver CS6
Visual web development tools

SublimeText3 Mac version
God-level code editing software (SublimeText3)

Hot Topics


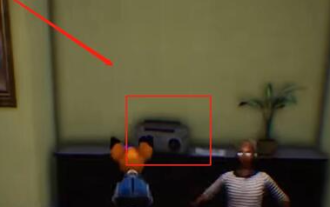
The Terror Corridor is a mission in Goat Simulator 3. How can you complete this mission? Master the detailed clearance methods and corresponding processes, and be able to complete the corresponding challenges of this mission. The following will bring you Goat Simulator. 3 Horror Corridor Guide to learn related information. Goat Simulator 3 Terror Corridor Guide 1. First, players need to go to Silent Hill in the upper left corner of the map. 2. Here you can see a house with RESTSTOP written on the roof. Players need to operate the goat to enter this house. 3. After entering the room, we first go straight forward, and then turn right. There is a door at the end here, and we go in directly from here. 4. After entering, we also need to walk forward first and then turn right. When we reach the door here, the door will be closed. We need to turn back and find it.
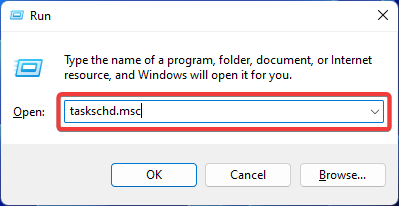
To automate tasks and manage multiple systems, mission planning software is a valuable tool in your arsenal, especially as a system administrator. Windows Task Scheduler does the job perfectly, but lately many people have reported operator rejected request errors. This problem exists in all iterations of the operating system, and even though it has been widely reported and covered, there is no effective solution. Keep reading to find out what might actually work for other people! What is the request in Task Scheduler 0x800710e0 that was denied by the operator or administrator? Task Scheduler allows automating various tasks and applications without user input. You can use it to schedule and organize specific applications, configure automatic notifications, help deliver messages, and more. it
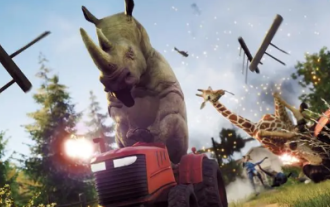
Goat Simulator 3 is a game with classic simulation gameplay, allowing players to fully experience the fun of casual action simulation. The game also has many exciting special tasks. Among them, the Goat Simulator 3 Imperial Tomb task requires players to find the bell tower. Some players are not sure how to operate the three clocks at the same time. Here is the guide to the Tomb of the Tomb mission in Goat Simulator 3! The guide to the Tomb of the Tomb mission in Goat Simulator 3 is to ring the bells in order. Detailed step expansion 1. First, players need to open the map and go to Wuqiu Cemetery. 2. Then go up to the bell tower. There will be three bells inside. 3. Then, in order from largest to smallest, follow the familiarity of 222312312. 4. After completing the knocking, you can complete the mission and open the door to get the lightsaber.
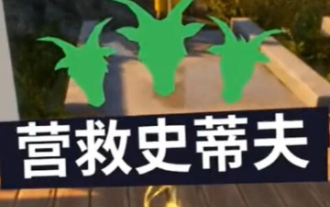
Rescue Steve is a unique task in Goat Simulator 3. What exactly needs to be done to complete it? This task is relatively simple, but we need to be careful not to misunderstand the meaning. Here we will bring you the rescue of Steve in Goat Simulator 3 Task strategies can help you better complete related tasks. Goat Simulator 3 Rescue Steve Mission Strategy 1. First come to the hot spring in the lower right corner of the map. 2. After arriving at the hot spring, you can trigger the task of rescuing Steve. 3. Note that there is a man in the hot spring. Although his name is Steve, he is not the target of this mission. 4. Find a fish named Steve in this hot spring and bring it ashore to complete this task.
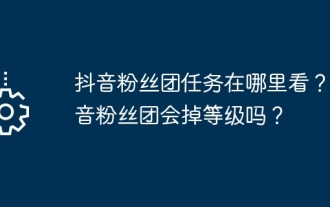
TikTok, as one of the most popular social media platforms at the moment, has attracted a large number of users to participate. On Douyin, there are many fan group tasks that users can complete to obtain certain rewards and benefits. So where can I find Douyin fan club tasks? 1. Where can I view Douyin fan club tasks? In order to find Douyin fan group tasks, you need to visit Douyin's personal homepage. On the homepage, you will see an option called "Fan Club." Click this option and you can browse the fan groups you have joined and related tasks. In the fan club task column, you will see various types of tasks, such as likes, comments, sharing, forwarding, etc. Each task has corresponding rewards and requirements. Generally speaking, after completing the task, you will receive a certain amount of gold coins or experience points.
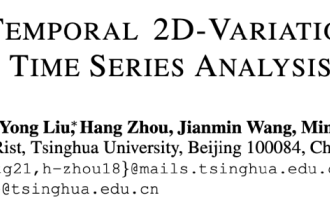
Achieving task universality is a core issue in the research of basic deep learning models, and is also one of the main focuses in the recent direction of large models. However, in the field of time series, various types of analysis tasks vary greatly. There are prediction tasks that require fine-grained modeling and classification tasks that require extracting high-level semantic information. How to build a unified deep basic model to efficiently complete various timing analysis tasks has not yet been established. To this end, a team from the School of Software of Tsinghua University conducted research on the basic issue of timing change modeling and proposed TimesNet, a task-universal timing basic model. The paper was accepted by ICLR 2023. Author list: Wu Haixu*, Hu Tengge*, Liu Yong*, Zhou Hang, Wang Jianmin, Long Mingsheng Link: https://ope
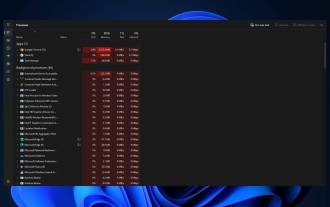
How to Pause Task Manager Process Updates in Windows 11 and Windows 10 Press CTRL+Window Key+Delete to open Task Manager. By default, Task Manager will open the Processes window. As you can see here, all the apps are endlessly moving around and it can be hard to point them down when you want to select them. So, press CTRL and hold it, this will pause the task manager. You can still select apps and even scroll down, but you must hold down the CTRL button at all times.
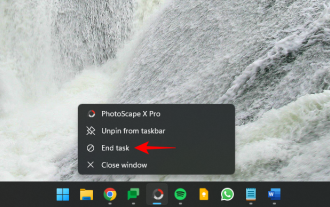
Frozen or unresponsive programs are easy to kill from Task Manager. But Microsoft has recently provided users with the facility to terminate these tasks directly from the taskbar. While the option isn't rolled out to everyone, it's easily available if you have the Windows Insider build. Here's everything you need to enable the End Task button and close tasks from the taskbar. How to Get the End Task Button from the Taskbar to Kill Apps Currently, the option to enable the End Task button for taskbar apps is only available as a developer option for users with Windows Insider builds. However, this may change in an upcoming feature update as it will be rolled out to users globally on the stable version. If you still
