


NVIDIA 64 A100 training StyleGAN-T; review of nine types of generative AI models
Directory:
- Quantum machine learning beyond kernel methods
- Wearable in- sensor computing reservoir using optoelectronic polymers with through-space charge-transport characteristics for multi-task learning
- Dash: Semi-Supervised Learning with Dynamic Thresholding
- StyleGAN-T: Unlocking the Power of GANs for Fast Large-Scale Text-to-Image Synthesis
- Open-Vocabulary Multi-Label Classification via Multi-Modal Knowledge Transfer
- ChatGPT is not all you need. A State of the Art Review of large Generative AI models
- ClimaX: A foundation model for weather and climate
- ArXiv Weekly Radiostation: NLP, CV, ML More selected papers (with audio)
Paper 1: Quantum machine learning beyond kernel methods
- Author: Sofiene Jerbi et al
- ##paper Address: https://www.nature.com/articles/s41467-023-36159-y
##Abstract:In this article, A research team from the University of Innsbruck, Austria, has identified a constructive framework that captures all standard models based on parameterized quantum circuits: the linear quantum model.
The researchers show how using tools from quantum information theory to efficiently map data re-upload circuits into a simpler picture of a linear model in quantum Hilbert space. Furthermore, the experimentally relevant resource requirements of these models are analyzed in terms of the number of qubits and the amount of data that needs to be learned. Recent results based on classical machine learning demonstrate that linear quantum models must use many more qubits than data reupload models to solve certain learning tasks, while kernel methods also require many more data points.The results provide a more comprehensive understanding of quantum machine learning models, as well as insights into the compatibility of different models with NISQ constraints.
Recommended:
Quantum machine learning beyond kernel methods, a unified framework for quantum learning models.
Paper 2: Wearable in-sensor reservoir computing using optoelectronic polymers with through-space charge-transport characteristics for multi-task learning
- Author: Xiaosong Wu et al
- Paper address: https://www.nature.com/articles/s41467 -023-36205-9
In-sensor multi-task learning is not only a key advantage of biological vision, but also a major advantage of artificial intelligence Target. However, traditional silicon vision chips have a large time and energy overhead. Additionally, training traditional deep learning models is neither scalable nor affordable on edge devices. In this article,
The research team from the Chinese Academy of Sciences and the University of Hong Kong proposes a materials algorithm co-design to simulate the learning paradigm of the human retina with low overhead . Based on the bottlebrush-shaped semiconductor p-NDI with efficient exciton dissociation and through-space charge transport properties, a wearable transistor-based dynamic sensor reservoir computing system is developed that exhibits excellent separability on different tasks properties, attenuation memory and echo state characteristics. Combined with the "readout function" on the memristive organic diode, RC can recognize handwritten letters and numbers, and classify various clothing, with an accuracy of 98.04%, 88.18% and 91.76% (higher than all reported organic semiconductors).
Comparison of the photocurrent response of conventional semiconductors and p-NDI, and detailed semiconductor design principles of the RC system within the sensor.
Recommendation: Low energy consumption and low time consumption, the Chinese Academy of Sciences & University of Hong Kong team used a new method to perform multi-task learning for internal reservoir calculations in wearable sensors.
Paper 3: Dash: Semi-Supervised Learning with Dynamic Thresholding
- Author: Yi Xu et al
- Paper address: https://proceedings.mlr.press/v139/xu21e/xu21e.pdf
Abstract: This paper innovatively proposes to use dynamic threshold to filter unlabeled samples for semi-supervised learning (SSL). Method, we transformed the training framework of semi-supervised learning, improved the selection strategy of unlabeled samples during the training process, and selected more effective unlabeled samples for training through dynamically changing thresholds. Dash is a general strategy that can be easily integrated with existing semi-supervised learning methods.
In terms of experiments, we have fully verified its effectiveness on standard data sets such as CIFAR-10, CIFAR-100, STL-10 and SVHN. In theory, the paper proves the convergence properties of the Dash algorithm from the perspective of non-convex optimization.
#Fixmatch Training Framework
Recommendation: Damo Academy’s open source semi-supervised learning framework Dash refreshes many SOTAs.
Paper 4: StyleGAN-T: Unlocking the Power of GANs for Fast Large-Scale Text-to-Image Synthesis
- Author: Axel Sauer et al
- Paper address: https://arxiv.org/pdf/2301.09515.pdf
Abstract: Are diffusion models the best at text-to-image generation? Not necessarily, the results of the new StyleGAN-T launched by Nvidia and others show that GAN is still competitive. StyleGAN-T only takes 0.1 seconds to generate a 512×512 resolution image:
Paper 5: Open-Vocabulary Multi-Label Classification via Multi-Modal Knowledge Transfer
- Author: Sunan He et al
- ##Paper address: https://arxiv.org/abs/2207.01887
- Abstract:
To this end,
Tencent Youtu Lab, together with Tsinghua University and Shenzhen University, proposed a framework MKT based on multi-modal knowledge transfer, utilize the powerful image-text matching capabilities of the image-text pre-training model to retain key visual consistency information in image classification, and realize Open Vocabulary classification of multi-label scenes. This work has been selected for AAAI 2023 Oral.
Comparison of ML-ZSL and MKT methods.
Recommended: AAAI 2023 Oral | How to identify unknown tags? Multimodal knowledge transfer framework to achieve new SOTA.
Paper 6: ChatGPT is not all you need. A State of the Art Review of large Generative AI models
- Author: Roberto Gozalo-Brizuela et al
- ##Paper address: https://arxiv.org/abs/2301.04655
Abstract: In the past two years, a large number of large-scale generative models have appeared in the AI field, such as ChatGPT or Stable Diffusion. Specifically, these models are able to perform tasks like general question answering systems or automatically creating artistic images, which are revolutionizing many fields.
In a recent review paper submitted by researchers from Comillas Pontifical University in Spain, the author tried to describe the impact of generative AI on many current models in a concise way. And classify the major recently released generative AI models.
Recommendation:
ChatGPT is not all you need, a review of 9 types of generative AI models from 6 major companies.Paper 7: ClimaX: A foundation model for weather and climate
Author: Tung Nguyen et al
- Paper address: https://arxiv.org/abs/2301.10343
- Abstract:
The Microsoft Autonomous Systems and Robotics research group and the Microsoft Research Center for Scientific Intelligence have developed ClimaX, a flexible and scalable deep learning for weather and climate science Model , can be trained using heterogeneous data sets spanning different variables, spatiotemporal coverage, and physical basis. ClimaX extends the Transformer architecture with novel encoding and aggregation blocks that allow efficient use of available computation while maintaining generality. ClimaX is pretrained using a self-supervised learning objective on climate datasets derived from CMIP6. The pretrained ClimaX can then be fine-tuned to solve a wide range of climate and weather tasks, including those involving atmospheric variables and spatiotemporal scales not seen during pretraining.
Recommended:
The Microsoft team released the first AI-based weather and climate basic model ClimaX.The above is the detailed content of NVIDIA 64 A100 training StyleGAN-T; review of nine types of generative AI models. For more information, please follow other related articles on the PHP Chinese website!

Hot AI Tools

Undresser.AI Undress
AI-powered app for creating realistic nude photos

AI Clothes Remover
Online AI tool for removing clothes from photos.

Undress AI Tool
Undress images for free

Clothoff.io
AI clothes remover

AI Hentai Generator
Generate AI Hentai for free.

Hot Article

Hot Tools

Notepad++7.3.1
Easy-to-use and free code editor

SublimeText3 Chinese version
Chinese version, very easy to use

Zend Studio 13.0.1
Powerful PHP integrated development environment

Dreamweaver CS6
Visual web development tools

SublimeText3 Mac version
God-level code editing software (SublimeText3)

Hot Topics


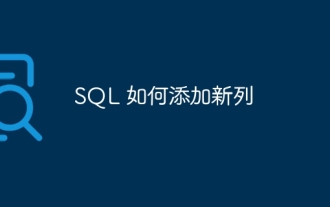
Add new columns to an existing table in SQL by using the ALTER TABLE statement. The specific steps include: determining the table name and column information, writing ALTER TABLE statements, and executing statements. For example, add an email column to the Customers table (VARCHAR(50)): ALTER TABLE Customers ADD email VARCHAR(50);
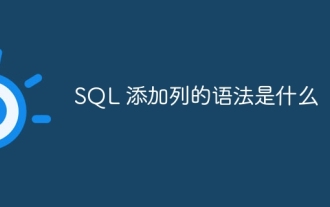
The syntax for adding columns in SQL is ALTER TABLE table_name ADD column_name data_type [NOT NULL] [DEFAULT default_value]; where table_name is the table name, column_name is the new column name, data_type is the data type, NOT NULL specifies whether null values are allowed, and DEFAULT default_value specifies the default value.
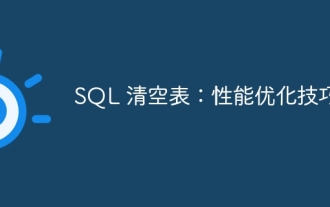
Tips to improve SQL table clearing performance: Use TRUNCATE TABLE instead of DELETE, free up space and reset the identity column. Disable foreign key constraints to prevent cascading deletion. Use transaction encapsulation operations to ensure data consistency. Batch delete big data and limit the number of rows through LIMIT. Rebuild the index after clearing to improve query efficiency.
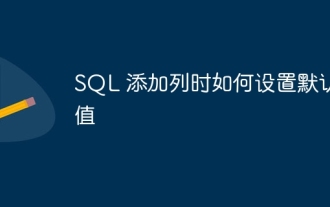
Set the default value for newly added columns, use the ALTER TABLE statement: Specify adding columns and set the default value: ALTER TABLE table_name ADD column_name data_type DEFAULT default_value; use the CONSTRAINT clause to specify the default value: ALTER TABLE table_name ADD COLUMN column_name data_type CONSTRAINT default_constraint DEFAULT default_value;
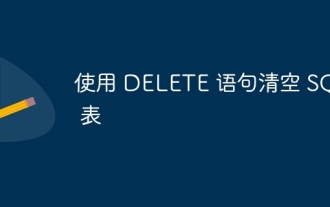
Yes, the DELETE statement can be used to clear a SQL table, the steps are as follows: Use the DELETE statement: DELETE FROM table_name; Replace table_name with the name of the table to be cleared.
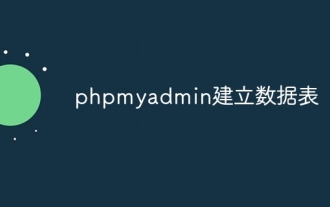
To create a data table using phpMyAdmin, the following steps are essential: Connect to the database and click the New tab. Name the table and select the storage engine (InnoDB recommended). Add column details by clicking the Add Column button, including column name, data type, whether to allow null values, and other properties. Select one or more columns as primary keys. Click the Save button to create tables and columns.
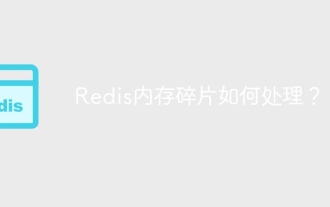
Redis memory fragmentation refers to the existence of small free areas in the allocated memory that cannot be reassigned. Coping strategies include: Restart Redis: completely clear the memory, but interrupt service. Optimize data structures: Use a structure that is more suitable for Redis to reduce the number of memory allocations and releases. Adjust configuration parameters: Use the policy to eliminate the least recently used key-value pairs. Use persistence mechanism: Back up data regularly and restart Redis to clean up fragments. Monitor memory usage: Discover problems in a timely manner and take measures.
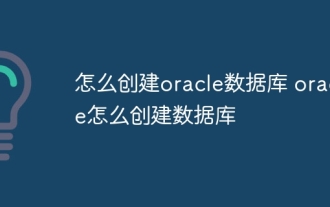
Creating an Oracle database is not easy, you need to understand the underlying mechanism. 1. You need to understand the concepts of database and Oracle DBMS; 2. Master the core concepts such as SID, CDB (container database), PDB (pluggable database); 3. Use SQL*Plus to create CDB, and then create PDB, you need to specify parameters such as size, number of data files, and paths; 4. Advanced applications need to adjust the character set, memory and other parameters, and perform performance tuning; 5. Pay attention to disk space, permissions and parameter settings, and continuously monitor and optimize database performance. Only by mastering it skillfully requires continuous practice can you truly understand the creation and management of Oracle databases.
