


LeCun likes: Running LLaMA on Apple M1/M2 chip! The 13 billion parameter model requires only 4GB of memory
Not long ago, Meta released the open source large language model LLaMA, but then netizens released a no-threshold download link, which was "miserably" open.
As soon as the news came out, the circle immediately became lively, and everyone began to download and test it.
But those friends who don’t have top-level graphics cards can only look at the model and sigh.
However, it’s not a big problem. Georgi Gerganov recently made a project called "llama.cpp" - LLaMA can be run without a GPU.
## Project address: https://github.com/ggerganov/llama.cpp
Yes, this also includes Macs equipped with Apple chips. And also received forwarding support from LeCun.
For now, this is a relatively comprehensive tutorial. There are two, based on Apple's M1 and M2 processors.
##First article: https://dev.l1x.be/posts/2023/03/ 12/using-llama-with-m1-mac/
Without further ado, let’s look at the effect first.
For example, on the smallest 7B model, ask: "Who was the first person to land on the moon?"
Very quickly, the results came out after a few seconds.
-p 'The first man to land on the moon was'
There are no obvious factual errors in Armstrong’s age, middle name and date of landing on the moon. This is not easy for such a small model.
If you use the following tips, you can generate some practical Python code.
-p 'def open_and_return_content(filename):'
def open_and_return_content(filename): """Opens file (returning the content) and performs basic sanity checks"""if os.path.isfile(filename): with open(filename) as f: content = f.read() return contentelse: print('WARNING: file "{}" does not exist'.format(filename), file=sys.stderr) return ''def get_file_info(filename, fullpath): """Get file information (i.e., permission, owner, group, size)"""
接下来,我们就来看看具体是如何实现的。
The first thing to do is to download the LLaMA model.
You can submit an application to Meta through the official form, or obtain it directly from the link shared by netizens.
In short, when you are done, you will see the following pile of things:
Step 2: Install dependencies
首先,你需要安装Xcode来编译C++项目。
xcode-select --install
接下来,是构建C++项目的依赖项(pkgconfig和cmake)。
brew install pkgconfig cmake
在环境的配置上,假如你用的是Python 3.11,则可以创建一个虚拟环境:
/opt/homebrew/bin/python3.11 -m venv venv
然后激活venv。(如果是fish以外的shell,只要去掉.fish后缀即可)
. venv/bin/activate.fish
最后,安装Torch。
pip3 install --pre torch torchvision --extra-index-url https://download.pytorch.org/whl/nightly/cpu
如果你对利用新的Metal性能着色器(MPS)后端进行GPU训练加速感兴趣,可以通过运行以下程序来进行验证。但这不是在M1上运行LLaMA的必要条件。
python Python 3.11.2 (main, Feb 16 2023, 02:55:59) [Clang 14.0.0 (clang-1400.0.29.202)] on darwin Type "help", "copyright", "credits" or "license" for more information. >>> import torch; torch.backends.mps.is_available()True
第三步:编译LLaMA CPP
git clone git@github.com:ggerganov/llama.cpp.git
在安装完所有的依赖项后,你可以运行make:
make I llama.cpp build info: I UNAME_S:Darwin I UNAME_P:arm I UNAME_M:arm64 I CFLAGS: -I.-O3 -DNDEBUG -std=c11 -fPIC -pthread -DGGML_USE_ACCELERATE I CXXFLAGS: -I. -I./examples -O3 -DNDEBUG -std=c++11 -fPIC -pthread I LDFLAGS: -framework Accelerate I CC: Apple clang version 14.0.0 (clang-1400.0.29.202)I CXX:Apple clang version 14.0.0 (clang-1400.0.29.202) cc-I.-O3 -DNDEBUG -std=c11 -fPIC -pthread -DGGML_USE_ACCELERATE -c ggml.c -o ggml.o c++ -I. -I./examples -O3 -DNDEBUG -std=c++11 -fPIC -pthread -c utils.cpp -o utils.o c++ -I. -I./examples -O3 -DNDEBUG -std=c++11 -fPIC -pthread main.cpp ggml.o utils.o -o main-framework Accelerate ./main -h usage: ./main [options] options: -h, --helpshow this help message and exit -s SEED, --seed SEEDRNG seed (default: -1) -t N, --threads N number of threads to use during computation (default: 4) -p PROMPT, --prompt PROMPT prompt to start generation with (default: random) -n N, --n_predict N number of tokens to predict (default: 128) --top_k N top-k sampling (default: 40) --top_p N top-p sampling (default: 0.9) --temp Ntemperature (default: 0.8) -b N, --batch_size Nbatch size for prompt processing (default: 8) -m FNAME, --model FNAME model path (default: models/llama-7B/ggml-model.bin) c++ -I. -I./examples -O3 -DNDEBUG -std=c++11 -fPIC -pthread quantize.cpp ggml.o utils.o -o quantize-framework Accelerate
第四步:转换模型
假设你已经把模型放在llama.cpp repo中的models/下。
python convert-pth-to-ggml.py models/7B 1
那么,应该会看到像这样的输出:
{'dim': 4096, 'multiple_of': 256, 'n_heads': 32, 'n_layers': 32, 'norm_eps': 1e-06, 'vocab_size': 32000}n_parts =1Processing part0Processing variable: tok_embeddings.weight with shape:torch.Size([32000, 4096])and type:torch.float16 Processing variable: norm.weight with shape:torch.Size([4096])and type:torch.float16 Converting to float32 Processing variable: output.weight with shape:torch.Size([32000, 4096])and type:torch.float16 Processing variable: layers.0.attention.wq.weight with shape:torch.Size([4096, 4096])and type:torch.f loat16 Processing variable: layers.0.attention.wk.weight with shape:torch.Size([4096, 4096])and type:torch.f loat16 Processing variable: layers.0.attention.wv.weight with shape:torch.Size([4096, 4096])and type:torch.f loat16 Processing variable: layers.0.attention.wo.weight with shape:torch.Size([4096, 4096])and type:torch.f loat16 Processing variable: layers.0.feed_forward.w1.weight with shape:torch.Size([11008, 4096])and type:tor ch.float16 Processing variable: layers.0.feed_forward.w2.weight with shape:torch.Size([4096, 11008])and type:tor ch.float16 Processing variable: layers.0.feed_forward.w3.weight with shape:torch.Size([11008, 4096])and type:tor ch.float16 Processing variable: layers.0.attention_norm.weight with shape:torch.Size([4096])and type:torch.float 16... Done. Output file: models/7B/ggml-model-f16.bin, (part0 )
下一步将是进行量化处理:
./quantize ./models/7B/ggml-model-f16.bin ./models/7B/ggml-model-q4_0.bin 2
输出如下:
llama_model_quantize: loading model from './models/7B/ggml-model-f16.bin'llama_model_quantize: n_vocab = 32000llama_model_quantize: n_ctx = 512llama_model_quantize: n_embd= 4096llama_model_quantize: n_mult= 256llama_model_quantize: n_head= 32llama_model_quantize: n_layer = 32llama_model_quantize: f16 = 1... layers.31.attention_norm.weight - [ 4096, 1], type =f32 size =0.016 MB layers.31.ffn_norm.weight - [ 4096, 1], type =f32 size =0.016 MB llama_model_quantize: model size= 25705.02 MB llama_model_quantize: quant size=4017.27 MB llama_model_quantize: hist: 0.000 0.022 0.019 0.033 0.053 0.078 0.104 0.125 0.134 0.125 0.104 0.078 0.053 0.033 0.019 0.022 main: quantize time = 29389.45 ms main:total time = 29389.45 ms
第五步:运行模型
./main -m ./models/7B/ggml-model-q4_0.bin -t 8 -n 128 -p 'The first president of the USA was '
main: seed = 1678615879llama_model_load: loading model from './models/7B/ggml-model-q4_0.bin' - please wait ... llama_model_load: n_vocab = 32000llama_model_load: n_ctx = 512llama_model_load: n_embd= 4096llama_model_load: n_mult= 256llama_model_load: n_head= 32llama_model_load: n_layer = 32llama_model_load: n_rot = 128llama_model_load: f16 = 2llama_model_load: n_ff= 11008llama_model_load: n_parts = 1llama_model_load: ggml ctx size = 4529.34 MB llama_model_load: memory_size = 512.00 MB, n_mem = 16384llama_model_load: loading model part 1/1 from './models/7B/ggml-model-q4_0.bin'llama_model_load: .................................... donellama_model_load: model size =4017.27 MB / num tensors = 291 main: prompt: 'The first president of the USA was 'main: number of tokens in prompt = 9 1 -> ''1576 -> 'The' 937 -> ' first'6673 -> ' president' 310 -> ' of' 278 -> ' the'8278 -> ' USA' 471 -> ' was' 29871 -> ' ' sampling parameters: temp = 0.800000, top_k = 40, top_p = 0.950000 The first president of the USA was 57 years old when he assumed office (George Washington). Nowadays, the US electorate expects the new president to be more young at heart. President Donald Trump was 70 years old when he was inaugurated. In contrast to his predecessors, he is physically fit, healthy and active. And his fitness has been a prominent theme of his presidency. During the presidential campaign, he famously said he would be the “most active president ever” — a statement Trump has not yet achieved, but one that fits his approach to the office. His tweets demonstrate his physical activity. main: mem per token = 14434244 bytes main: load time =1311.74 ms main: sample time = 278.96 ms main:predict time =7375.89 ms / 54.23 ms per token main:total time =9216.61 ms
资源使用情况
第二位博主表示,在运行时,13B模型使用了大约4GB的内存,以及748%的CPU。(设定的就是让模型使用8个CPU核心)
没有指令微调
GPT-3和ChatGPT效果如此之好的关键原因之一是,它们都经过了指令微调,
这种额外的训练使它们有能力对人类的指令做出有效的反应。比如「总结一下这个」或「写一首关于水獭的诗」或「从这篇文章中提取要点」。
撰写教程的博主表示,据他观察,LLaMA并没有这样的能力。
也就是说,给LLaMA的提示需要采用经典的形式:「一些将由......完成的文本」。这也让提示工程变得更加困难。
举个例子,博主至今都还没有想出一个正确的提示,从而让LLaMA实现文本的总结。
The above is the detailed content of LeCun likes: Running LLaMA on Apple M1/M2 chip! The 13 billion parameter model requires only 4GB of memory. For more information, please follow other related articles on the PHP Chinese website!

Hot AI Tools

Undresser.AI Undress
AI-powered app for creating realistic nude photos

AI Clothes Remover
Online AI tool for removing clothes from photos.

Undress AI Tool
Undress images for free

Clothoff.io
AI clothes remover

AI Hentai Generator
Generate AI Hentai for free.

Hot Article

Hot Tools

Notepad++7.3.1
Easy-to-use and free code editor

SublimeText3 Chinese version
Chinese version, very easy to use

Zend Studio 13.0.1
Powerful PHP integrated development environment

Dreamweaver CS6
Visual web development tools

SublimeText3 Mac version
God-level code editing software (SublimeText3)

Hot Topics
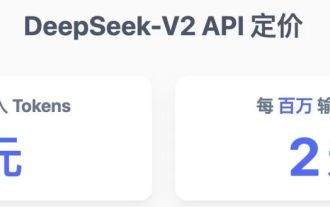
Imagine an artificial intelligence model that not only has the ability to surpass traditional computing, but also achieves more efficient performance at a lower cost. This is not science fiction, DeepSeek-V2[1], the world’s most powerful open source MoE model is here. DeepSeek-V2 is a powerful mixture of experts (MoE) language model with the characteristics of economical training and efficient inference. It consists of 236B parameters, 21B of which are used to activate each marker. Compared with DeepSeek67B, DeepSeek-V2 has stronger performance, while saving 42.5% of training costs, reducing KV cache by 93.3%, and increasing the maximum generation throughput to 5.76 times. DeepSeek is a company exploring general artificial intelligence
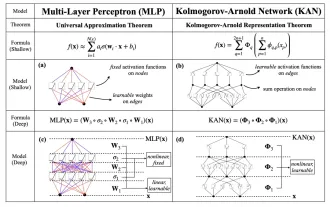
Earlier this month, researchers from MIT and other institutions proposed a very promising alternative to MLP - KAN. KAN outperforms MLP in terms of accuracy and interpretability. And it can outperform MLP running with a larger number of parameters with a very small number of parameters. For example, the authors stated that they used KAN to reproduce DeepMind's results with a smaller network and a higher degree of automation. Specifically, DeepMind's MLP has about 300,000 parameters, while KAN only has about 200 parameters. KAN has a strong mathematical foundation like MLP. MLP is based on the universal approximation theorem, while KAN is based on the Kolmogorov-Arnold representation theorem. As shown in the figure below, KAN has
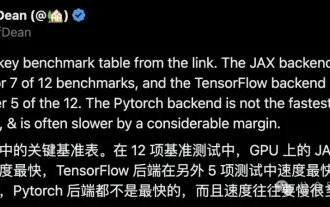
The performance of JAX, promoted by Google, has surpassed that of Pytorch and TensorFlow in recent benchmark tests, ranking first in 7 indicators. And the test was not done on the TPU with the best JAX performance. Although among developers, Pytorch is still more popular than Tensorflow. But in the future, perhaps more large models will be trained and run based on the JAX platform. Models Recently, the Keras team benchmarked three backends (TensorFlow, JAX, PyTorch) with the native PyTorch implementation and Keras2 with TensorFlow. First, they select a set of mainstream
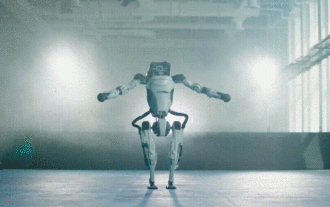
Boston Dynamics Atlas officially enters the era of electric robots! Yesterday, the hydraulic Atlas just "tearfully" withdrew from the stage of history. Today, Boston Dynamics announced that the electric Atlas is on the job. It seems that in the field of commercial humanoid robots, Boston Dynamics is determined to compete with Tesla. After the new video was released, it had already been viewed by more than one million people in just ten hours. The old people leave and new roles appear. This is a historical necessity. There is no doubt that this year is the explosive year of humanoid robots. Netizens commented: The advancement of robots has made this year's opening ceremony look like a human, and the degree of freedom is far greater than that of humans. But is this really not a horror movie? At the beginning of the video, Atlas is lying calmly on the ground, seemingly on his back. What follows is jaw-dropping
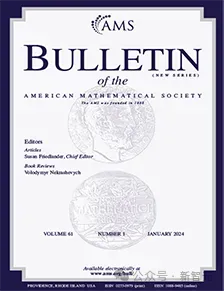
AI is indeed changing mathematics. Recently, Tao Zhexuan, who has been paying close attention to this issue, forwarded the latest issue of "Bulletin of the American Mathematical Society" (Bulletin of the American Mathematical Society). Focusing on the topic "Will machines change mathematics?", many mathematicians expressed their opinions. The whole process was full of sparks, hardcore and exciting. The author has a strong lineup, including Fields Medal winner Akshay Venkatesh, Chinese mathematician Zheng Lejun, NYU computer scientist Ernest Davis and many other well-known scholars in the industry. The world of AI has changed dramatically. You know, many of these articles were submitted a year ago.
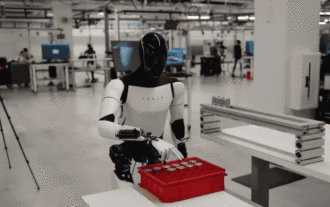
The latest video of Tesla's robot Optimus is released, and it can already work in the factory. At normal speed, it sorts batteries (Tesla's 4680 batteries) like this: The official also released what it looks like at 20x speed - on a small "workstation", picking and picking and picking: This time it is released One of the highlights of the video is that Optimus completes this work in the factory, completely autonomously, without human intervention throughout the process. And from the perspective of Optimus, it can also pick up and place the crooked battery, focusing on automatic error correction: Regarding Optimus's hand, NVIDIA scientist Jim Fan gave a high evaluation: Optimus's hand is the world's five-fingered robot. One of the most dexterous. Its hands are not only tactile
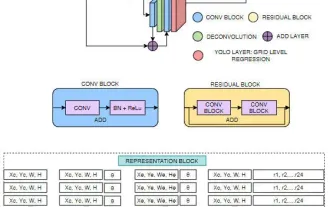
Target detection is a relatively mature problem in autonomous driving systems, among which pedestrian detection is one of the earliest algorithms to be deployed. Very comprehensive research has been carried out in most papers. However, distance perception using fisheye cameras for surround view is relatively less studied. Due to large radial distortion, standard bounding box representation is difficult to implement in fisheye cameras. To alleviate the above description, we explore extended bounding box, ellipse, and general polygon designs into polar/angular representations and define an instance segmentation mIOU metric to analyze these representations. The proposed model fisheyeDetNet with polygonal shape outperforms other models and simultaneously achieves 49.5% mAP on the Valeo fisheye camera dataset for autonomous driving
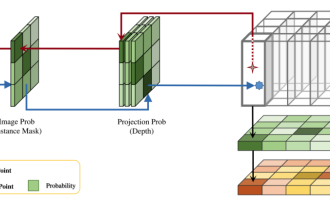
This paper explores the problem of accurately detecting objects from different viewing angles (such as perspective and bird's-eye view) in autonomous driving, especially how to effectively transform features from perspective (PV) to bird's-eye view (BEV) space. Transformation is implemented via the Visual Transformation (VT) module. Existing methods are broadly divided into two strategies: 2D to 3D and 3D to 2D conversion. 2D-to-3D methods improve dense 2D features by predicting depth probabilities, but the inherent uncertainty of depth predictions, especially in distant regions, may introduce inaccuracies. While 3D to 2D methods usually use 3D queries to sample 2D features and learn the attention weights of the correspondence between 3D and 2D features through a Transformer, which increases the computational and deployment time.
