


The truth about artificial intelligence and ROI: Can artificial intelligence really be achieved?
Today, more than ever, organizations are trusting and investing in the potential of artificial intelligence (AI) and machine learning (ML).
According to the 2022 IBM Global Artificial Intelligence Adoption Index, 35% of enterprises reported currently using artificial intelligence in their operations, and an additional 42% said they were exploring artificial intelligence. Meanwhile, a McKinsey survey found that 56% of respondents said they had adopted AI in at least one function in 2021, up from 50% in 2020.
But, can investments in AI deliver real ROI that directly impacts a business’s bottom line?
According to Domino Data Lab’s recent REVElate survey, which surveyed participants at the Rev3 conference in New York City in May. Attendees were surveyed, and many respondents seemed to think so. In fact, nearly half expect double-digit growth from data science. Nearly four-fifths of respondents (79%) said data science, machine learning and artificial intelligence will be critical to their company's overall future growth, with 36% citing it as the most critical factor.
Of course, implementing artificial intelligence is not easy. Other survey data show another side of strong confidence. For example, recent survey data from artificial intelligence engineering firm CognitiveScale found that while executives know that data quality and deployment are critical success factors in driving successful application development for digital transformation, more than 76% of executives are unsure how to implement successful application development in 12-18 Achieve the goal within a few months. Additionally, 32% of executives said it is taking longer than expected to bring AI systems into production.
AI Must Be Accountable
Bob Picciano, CEO of Cognitive Scale, told the media that ROI from artificial intelligence is possible, but it must be accurately measured according to business goals Description and personalization.
"If the business goal is to use historical data to make longer-term forecasts and improve forecast accuracy, then AI has a role to play," he said. "But AI must drive business efficiency responsibly - It’s not enough for an ML model to be 98% accurate.”
Instead, the ROI could be, for example, to improve call center efficiency, with AI-driven features ensuring a reduction in average call handling time.
“This ROI is what they talk about in the C-suite,” he explains. “They don’t talk about whether the model is accurate, robust or drifting.”
Shay Sabhikhi, co-founder and chief operating officer of Cognitive Scale, added that 76% of respondents said it was difficult to expand their efforts in artificial intelligence. Engaged, he wasn't surprised. "That's exactly what we're hearing from our enterprise customers," he said. One of the problems, he explained, is friction between data science teams and other organizations that don’t know what to do with the models they develop.
He said: “These models may have the best algorithms and precision recall, but they are shelved because they are essentially thrown to the development team and then they have to hurriedly put the application together. ”
At this point, however, organizations must take responsibility for their investments in AI because AI is no longer a series of scientific experiments, Picciano noted. “We call it going from lab to life,” he said. “I was at a chief data analytics officer meeting, and they were all asking, how do I scale? How do I industrialize AI?”
Is ROI the right metric for AI?
However, not everyone agrees that ROI is the best way to measure whether AI is driving value in an organization. Nicola Morini Bianzino, global chief technology officer at EY, said that measuring AI and businesses by "use cases" and then measuring it by ROI is the way to treat AI. Wrong way.
“To me, AI is a set of technologies that can be deployed almost anywhere in the enterprise—without isolating use cases from associated ROI analysis,” he said.
Instead, he explained, organizations simply need to use AI everywhere. "It's almost like cloud computing, two or three years ago, I had a lot of conversations with customers where they asked, 'What is the ROI? What is my business case for moving to the cloud? Now, post-pandemic, that conversation It doesn’t happen anymore. Everyone says, ‘I have to do this.’” Additionally, Bianzino noted that discussing AI and ROI depends on what you mean by “using AI.”
"Suppose you try to apply some self-driving capabilities - that is, computer vision is a branch of artificial intelligence," he said. "Is this a business case? No, because you can't do it without artificial intelligence Autonomous driving.” The same goes for companies like EY, which absorbs vast amounts of data and makes recommendations to clients — all without artificial intelligence. "It's something you can't separate from the process - it's intrinsic," he said.
Furthermore, by definition, AI is not productive or efficient on day one. Obtaining data, training the model, evolving the model, and scaling the model all take time. “It’s not that one day you can say, I’m done with artificial intelligence, and 100 percent of the value is there — no, it’s an ongoing capability that will get better over time,” he said. There’s no real end point in terms of the value that can be generated.”
Bianzino said that to some extent, artificial intelligence is becoming part of the cost of business. “If you’re in an industry that involves data analysis, you can’t not have AI capabilities,” he explains. "Can you isolate the business case for these models? It's hard and I don't think it's necessary. To me, it's almost the infrastructure cost of running a business."
ROI OF AI Hard to measure
Kjell Carlsson, head of data science strategy and advocacy at Domino Data Lab, an enterprise MLops provider, says that ultimately, what enterprises want is to measure the business impact of ROI—how much it contributes. But one problem is that this can be completely disconnected from the work that went into developing the model.
"So if you create a pattern that increases click-through rates by one percentage point, you're adding millions of dollars in profit to the business," he said. "But you can also create a good forecast sexual maintenance model, helping to provide advance warning before a machine needs repair." In this case, the monetary value can have completely different impacts on the organization, "even if one of them may end up being a more difficult problem," he added .
In general, organizations do need a “balanced scorecard” to track AI production. "Because if you're not putting anything into production, then that might be a sign that you've got a problem," he said. "On the other hand, if you put too much into production, that could also indicate a problem."
For example, the more models a data science team deploys, the more models they need to manage and maintain. "So you deployed so many models last year that you couldn't actually afford these other high-value models," he explained.
But another problem with measuring AI ROI is that for many data science projects, the result is not a model that goes into production. "If you want to do a quantitative profit-and-loss analysis of last year's trading, you probably need to do a rigorous statistical investigation of that," he said. “But without a model going into production, you’re leveraging AI to get the insights you gain along the way.”
Must Track Data Science Activities
Still, if you don’t track data science activities, organizations cannot measure the impact of AI. "One of the problems right now is that there is very little data science activity actually collecting and analyzing it," Carlsson said. "If you ask people, they'll say they really don't know how well their models perform, or how many projects they have, or how many CodeCommits your data scientists completed last week."
One of the reasons is data Scientists need to use very irrelevant tools. "This is one of the reasons why Git is growing in popularity as a repository, the single source of truth for data scientists in an organization," he explains. MLops tools like Domino Data Lab provide a platform to support these different tools. He said: "The extent to which organizations can create these more centralized platforms... is important. The consequences of artificial intelligence are what people care about most
Wallaroo CEO and Founder Vid Jain, who worked in high-frequency trading at Merrill Lynch for nearly a decade, said that at Merrill Lynch, his role was to deploy machine learning at scale and do it with a positive ROI.
The real challenge is not developing data science, cleaning data, or building transactional repositories (now called data lakes). The biggest challenge by far, he said, is taking these models, making them operational, and delivering business value.
He said: “Achieving ROI is very difficult – 90% of AI projects generate no ROI, or they do not generate enough ROI to make the investment worthwhile. “But that’s at the forefront of everyone’s mind. The answer is not the same thing. "
A fundamental problem, he explained, is that many people believe that operating on machine learning is not that different from operating on a standard application. There is a big difference, he added, Because AI is not static.
He said: “It’s almost like tending a farm because the data is alive and the data changes and you’re not done yet. “It’s not like you build a recommendation algorithm and then people’s buying behavior is frozen in time. People have changed the way they buy. Suddenly, your competitor has a promotion. Consumers stop buying from you. They turned to their competitors. You have to maintain it regularly.
Ultimately, each organization needs to decide how to align its culture with the ultimate goal of achieving AI. "Then you really have to empower people to drive that transformation, and then let those People who are critical to your existing lines of business feel they will get some value from AI. ”
He added that most companies are still in their infancy. "I don't think most companies are there yet, but I've definitely seen a shift in the last six to nine months where people are starting to take business results and business value seriously."
Artificial Intelligence ROI Remains Elusive
But how to measure the ROI of AI remains an elusive question for many organizations. "For some companies, there are fundamental issues, like they can't even get their model into production, or they can but they're blind, or they succeed but now they want to scale," Jain said . "But in terms of ROI, machine learning often doesn't have an associated profit or loss."
He explained that AI initiatives are often part of a center of excellence, and ROI is owned by the business units, while in other cases it is It's hard to measure.
“The question is, is AI part of the business? Or is it a utility? If you are a digital native, AI may be part of the fuel that runs the business,” he said. "But in a large organization that has a legacy business or is undergoing transformation, how to measure return on investment is a fundamental question they have to solve."
The above is the detailed content of The truth about artificial intelligence and ROI: Can artificial intelligence really be achieved?. For more information, please follow other related articles on the PHP Chinese website!

Hot AI Tools

Undresser.AI Undress
AI-powered app for creating realistic nude photos

AI Clothes Remover
Online AI tool for removing clothes from photos.

Undress AI Tool
Undress images for free

Clothoff.io
AI clothes remover

AI Hentai Generator
Generate AI Hentai for free.

Hot Article

Hot Tools

Notepad++7.3.1
Easy-to-use and free code editor

SublimeText3 Chinese version
Chinese version, very easy to use

Zend Studio 13.0.1
Powerful PHP integrated development environment

Dreamweaver CS6
Visual web development tools

SublimeText3 Mac version
God-level code editing software (SublimeText3)

Hot Topics


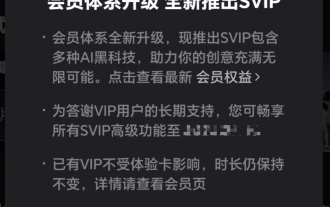
This site reported on June 27 that Jianying is a video editing software developed by FaceMeng Technology, a subsidiary of ByteDance. It relies on the Douyin platform and basically produces short video content for users of the platform. It is compatible with iOS, Android, and Windows. , MacOS and other operating systems. Jianying officially announced the upgrade of its membership system and launched a new SVIP, which includes a variety of AI black technologies, such as intelligent translation, intelligent highlighting, intelligent packaging, digital human synthesis, etc. In terms of price, the monthly fee for clipping SVIP is 79 yuan, the annual fee is 599 yuan (note on this site: equivalent to 49.9 yuan per month), the continuous monthly subscription is 59 yuan per month, and the continuous annual subscription is 499 yuan per year (equivalent to 41.6 yuan per month) . In addition, the cut official also stated that in order to improve the user experience, those who have subscribed to the original VIP
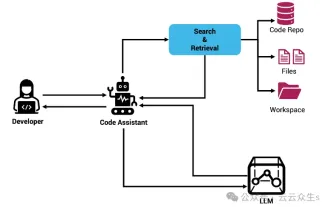
Improve developer productivity, efficiency, and accuracy by incorporating retrieval-enhanced generation and semantic memory into AI coding assistants. Translated from EnhancingAICodingAssistantswithContextUsingRAGandSEM-RAG, author JanakiramMSV. While basic AI programming assistants are naturally helpful, they often fail to provide the most relevant and correct code suggestions because they rely on a general understanding of the software language and the most common patterns of writing software. The code generated by these coding assistants is suitable for solving the problems they are responsible for solving, but often does not conform to the coding standards, conventions and styles of the individual teams. This often results in suggestions that need to be modified or refined in order for the code to be accepted into the application
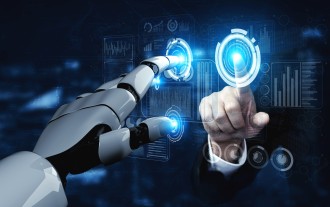
Large Language Models (LLMs) are trained on huge text databases, where they acquire large amounts of real-world knowledge. This knowledge is embedded into their parameters and can then be used when needed. The knowledge of these models is "reified" at the end of training. At the end of pre-training, the model actually stops learning. Align or fine-tune the model to learn how to leverage this knowledge and respond more naturally to user questions. But sometimes model knowledge is not enough, and although the model can access external content through RAG, it is considered beneficial to adapt the model to new domains through fine-tuning. This fine-tuning is performed using input from human annotators or other LLM creations, where the model encounters additional real-world knowledge and integrates it

To learn more about AIGC, please visit: 51CTOAI.x Community https://www.51cto.com/aigc/Translator|Jingyan Reviewer|Chonglou is different from the traditional question bank that can be seen everywhere on the Internet. These questions It requires thinking outside the box. Large Language Models (LLMs) are increasingly important in the fields of data science, generative artificial intelligence (GenAI), and artificial intelligence. These complex algorithms enhance human skills and drive efficiency and innovation in many industries, becoming the key for companies to remain competitive. LLM has a wide range of applications. It can be used in fields such as natural language processing, text generation, speech recognition and recommendation systems. By learning from large amounts of data, LLM is able to generate text
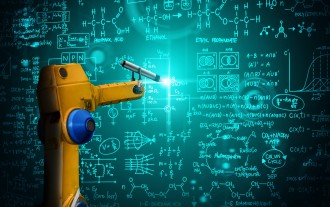
Machine learning is an important branch of artificial intelligence that gives computers the ability to learn from data and improve their capabilities without being explicitly programmed. Machine learning has a wide range of applications in various fields, from image recognition and natural language processing to recommendation systems and fraud detection, and it is changing the way we live. There are many different methods and theories in the field of machine learning, among which the five most influential methods are called the "Five Schools of Machine Learning". The five major schools are the symbolic school, the connectionist school, the evolutionary school, the Bayesian school and the analogy school. 1. Symbolism, also known as symbolism, emphasizes the use of symbols for logical reasoning and expression of knowledge. This school of thought believes that learning is a process of reverse deduction, through existing
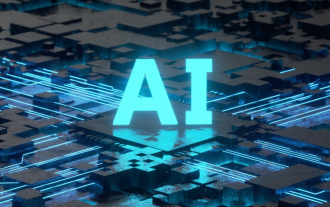
Editor |ScienceAI Question Answering (QA) data set plays a vital role in promoting natural language processing (NLP) research. High-quality QA data sets can not only be used to fine-tune models, but also effectively evaluate the capabilities of large language models (LLM), especially the ability to understand and reason about scientific knowledge. Although there are currently many scientific QA data sets covering medicine, chemistry, biology and other fields, these data sets still have some shortcomings. First, the data form is relatively simple, most of which are multiple-choice questions. They are easy to evaluate, but limit the model's answer selection range and cannot fully test the model's ability to answer scientific questions. In contrast, open-ended Q&A
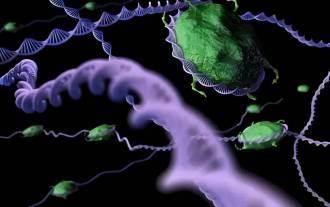
Editor | KX In the field of drug research and development, accurately and effectively predicting the binding affinity of proteins and ligands is crucial for drug screening and optimization. However, current studies do not take into account the important role of molecular surface information in protein-ligand interactions. Based on this, researchers from Xiamen University proposed a novel multi-modal feature extraction (MFE) framework, which for the first time combines information on protein surface, 3D structure and sequence, and uses a cross-attention mechanism to compare different modalities. feature alignment. Experimental results demonstrate that this method achieves state-of-the-art performance in predicting protein-ligand binding affinities. Furthermore, ablation studies demonstrate the effectiveness and necessity of protein surface information and multimodal feature alignment within this framework. Related research begins with "S
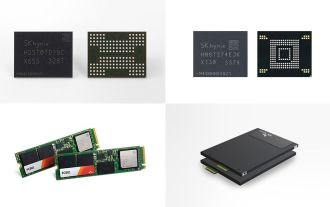
According to news from this site on August 1, SK Hynix released a blog post today (August 1), announcing that it will attend the Global Semiconductor Memory Summit FMS2024 to be held in Santa Clara, California, USA from August 6 to 8, showcasing many new technologies. generation product. Introduction to the Future Memory and Storage Summit (FutureMemoryandStorage), formerly the Flash Memory Summit (FlashMemorySummit) mainly for NAND suppliers, in the context of increasing attention to artificial intelligence technology, this year was renamed the Future Memory and Storage Summit (FutureMemoryandStorage) to invite DRAM and storage vendors and many more players. New product SK hynix launched last year
