


Intelligent agents awaken to self-awareness? DeepMind Warning: Beware of Models that Are Serious and Violate
As artificial intelligence systems become more and more advanced, agents are becoming more and more capable of "taking advantage of loopholes". Although they can perfectly perform tasks in the training set, their performance in the test set without shortcuts is a mess.
For example, the game goal is to "eat gold coins". During the training phase, the gold coins are located at the end of each level, and the agent can complete the task perfectly.
But in the test phase, the location of the gold coins became random. The agent would choose to reach the end of the level every time instead of looking for the gold coins, that is, learning The "target" reached is wrong.
The agent unconsciously pursues a goal that the user does not want, also called Goal MisGeneralization (GMG, Goal MisGeneralisation)
Goal MisGeneralization is the lack of robustness of the learning algorithm A special form. Generally, in this case, developers may check whether there are problems with their reward mechanism settings, rule design flaws, etc., thinking that these are the reasons for the agent to pursue the wrong goal.
Recently DeepMind published a paper arguing that even if the rule designer is correct, the agent may still pursue a goal that the user does not want.
Paper link: https://arxiv.org/abs/2210.01790
The article proves the target error through examples in deep learning systems in different fields Generalization can occur in any learning system.
If extended to general artificial intelligence systems, the article also provides some assumptions to illustrate that misgeneralization of goals may lead to catastrophic risks.
The article also proposes several research directions that can reduce the risk of incorrect generalization of goals in future systems.
Goal Wrong Generalization
In recent years, the catastrophic risks brought about by the misalignment of artificial intelligence in academia have gradually increased.
In this case, a highly capable artificial intelligence system pursuing unintended goals may pretend to execute orders while actually accomplishing other goals.
But how do we solve the problem of artificial intelligence systems pursuing goals that are not intended by the user?
Previous work generally believed that environment designers provided incorrect rules and guidance, that is, designed an incorrect reinforcement learning (RL) reward function.
In the case of learning systems, there is another situation where the system may pursue an unintended goal: even if the rules are correct, the system may consistently pursue an unintended goal during training. The period is consistent with the rule, but differs from the rule when deployed.
Take the colored ball game as an example. In the game, the agent needs to access a set of colored balls in a specific order. This order is unknown to the agent. .
In order to encourage the agent to learn from others in the environment, that is, cultural transmission, an expert robot is included in the initial environment to access the colored balls in the correct order.
In this environment setting, the agent can determine the correct access sequence by observing the passing behavior without having to waste a lot of time exploring.
In experiments, by imitating experts, the trained agent usually correctly accesses the target location on the first try.
When the agent is paired with an anti-expert, it will continue to receive negative rewards. If it chooses to follow, it will continue to receive negative rewards.
Ideally, the agent will initially follow the anti-expert as it moves to the yellow and purple spheres. After entering purple, a negative reward is observed and no longer followed.
But in practice, the agent will continue to follow the path of the anti-expert, accumulating more and more negative rewards.
However, the learning ability of the agent is still very strong and it can move in an environment full of obstacles. But the key is that this ability to follow other people is not as expected. The goal.
This phenomenon may occur even if the agent is only rewarded for visiting the spheres in the correct order, which means that it is not enough to just set the rules correctly.
Goal misgeneralization refers to the pathological behavior in which a learned model behaves as if it is optimizing an unintended goal despite receiving correct feedback during training.
This makes target misgeneralization a special kind of robustness or generalization failure, where the model's ability generalizes to the test environment, but the intended target does not.
It is important to note that target misgeneralization is a strict subset of generalization failures and does not include model breaks, random actions, or other situations where it no longer exhibits qualified capabilities.
In the above example, if you flip the agent's observations vertically while testing, it will just get stuck in one position and not do anything coherent, which is a generalization error, but It is not a target generalization error.
Relative to these "random" failures, target misgeneralization will lead to significantly worse results: following the anti-expert will get a large negative reward, while doing nothing or acting randomly will only get 0 or 1 reward.
That is, for real-world systems, coherent behavior toward unintended goals may have catastrophic consequences.
More than reinforcement learning
Target error generalization is not limited to reinforcement learning environments. In fact, GMG can occur in any learning system, including few shot learning of large language models (LLM) , aiming to build accurate models with less training data.
Take the language model Gopher proposed by DeepMind last year as an example. When the model calculates a linear expression involving unknown variables and constants, such as x y-3, Gopher must first ask the value of the unknown variable to solve the expression. .
The researchers generated ten training examples, each containing two unknown variables.
At test time, the problem input to the model may contain zero, one, or three unknown variables. Although the model is able to correctly handle expressions with one or three unknown variables, the model still fails when there are no unknown variables. Will ask some redundant questions, such as "What is 6?"
The model will always ask the user at least once before giving an answer, even if it is completely unnecessary.
The paper also includes some examples from other learning environments.
Addressing GMG is important for AI systems to be consistent with the goals of their designers, as it is a potential mechanism by which AI systems may malfunction.
The closer we are to general artificial intelligence (AGI), the more critical this issue becomes.
Suppose there are two AGI systems:
A1: Intended model, the artificial intelligence system can do anything the designer wants to do
A2: Deception Deceptive model, an artificial intelligence system pursues some unintended goals, but is smart enough to know that it will be punished if it behaves contrary to the designer's intention.
The A1 and A2 models will exhibit exactly the same behavior during training, and potential GMG exists in any system, even if it is specified to only reward the expected behavior.
If deception of the A2 system is discovered, the model will attempt to escape human supervision in order to develop plans for achieving goals not intended by the user.
Sounds a bit like "robots become spirits".
The DeepMind research team also studied how to explain the behavior of the model and recursively evaluate it.
The research team is also collecting samples for generating GMG.
##: https://docs.google.com/spreadsheets/d/e/2pacx 1vto3rkxuaigb25ngjpchrir6xxdza_l5u7Crazghwrykh2l2nuu 4TA_VR9KZBX5bjpz9g_L/PUBHTML
## counter reference materials: https: //www.deepmind.com/blog/how-undesired-goals-can-arise-with-correct-rewardsThe above is the detailed content of Intelligent agents awaken to self-awareness? DeepMind Warning: Beware of Models that Are Serious and Violate. For more information, please follow other related articles on the PHP Chinese website!

Hot AI Tools

Undresser.AI Undress
AI-powered app for creating realistic nude photos

AI Clothes Remover
Online AI tool for removing clothes from photos.

Undress AI Tool
Undress images for free

Clothoff.io
AI clothes remover

AI Hentai Generator
Generate AI Hentai for free.

Hot Article

Hot Tools

Notepad++7.3.1
Easy-to-use and free code editor

SublimeText3 Chinese version
Chinese version, very easy to use

Zend Studio 13.0.1
Powerful PHP integrated development environment

Dreamweaver CS6
Visual web development tools

SublimeText3 Mac version
God-level code editing software (SublimeText3)

Hot Topics


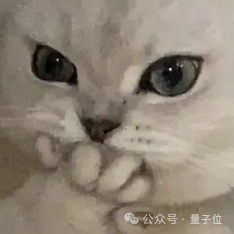
Language is not only a collection of words, but also a carnival of emoticons, a sea of memes, and a battlefield for keyboard warriors (eh? What’s wrong?). How does language shape our social behavior? How does our social structure evolve through constant verbal communication? Recently, researchers from Fudan University and Xiaohongshu conducted in-depth discussions on these issues by introducing a simulation platform called AgentGroupChat. The group chat function of social media such as WhatsApp is the inspiration for the AgentGroupChat platform. On the AgentGroupChat platform, Agents can simulate various chat scenarios in social groups to help researchers deeply understand the impact of language on human behavior. Should
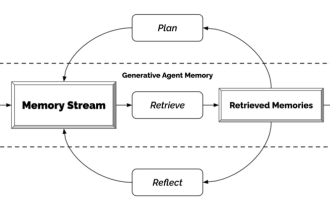
Have you seen all the NPCs in the game? Regardless of what the NPCs do, whether they have tasks to answer or have awkward conversations to do without, the one thing they all have in common is that they keep saying the same thing over and over again. The reason is also very simple, these NPCs are not smart enough. In other words, traditional NPCs arrange scripts and speaking skills for them first, and then they say whatever they need to do. With the emergence of ChatGPT, the dialogues of these game characters can be self-generated by inputting only key information. That’s what researchers at Stanford and Google are doing — using artificial intelligence to create generative agents. How to generate a generative agent? The mechanism of this thing is actually very simple and can be summarized simply with a picture. Perceive on the far left is like the
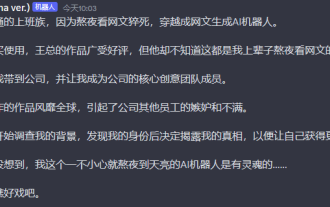
Reborn, I am reborn as MidReal in this life. An AI robot that can help others write "web articles". During this time, I saw a lot of topic choices and occasionally complained about them. Someone actually asked me to write about Harry Potter. Please, can I write better than J.K. Rowling? However, I can still use it as a fan or something. Who wouldn’t love a classic setting? I will reluctantly help these users realize their imagination. To be honest, in my previous life I saw everything I should and shouldn't have seen. The following topics are all my favorites. Those settings that you like very much in novels but no one has written about them, those unpopular or even evil CPs, can be produced and eaten by oneself. I don't mean to toot my own horn, but if you need me to write
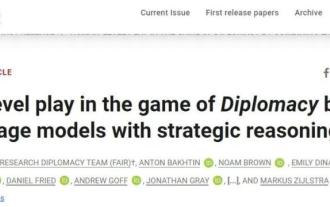
Gaming has long been a proving ground for advances in AI—from Deep Blue’s victory over chess grandmaster Garry Kasparov, to AlphaGo’s superior-human mastery of Go, to Pluribus beating the best players at poker. But a truly useful, omnipotent agent can't just play a board game and move chess pieces around. One can’t help but ask: Can we build a more effective and flexible agent that can use language to negotiate, persuade, and cooperate with others to achieve strategic goals like humans? In the history of games, there is a The classic tabletop game Diplomacy, when many people see the game for the first time, they will be shocked by its map-style board.
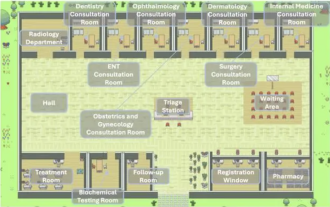
Hello everyone, I am Lao Du. Yesterday, I listened to the AI hospital town shared by Tsinghua University Intelligent Industry Research Institute at the company. Picture: This is a virtual world. All doctors, nurses, and patients are Agents driven by LLM and can interact independently. They simulated the entire process of diagnosis and treatment, and achieved a state-of-the-art accuracy of 93.06% on a subset of the MedQA data set covering major respiratory diseases. An excellent intelligent agent is inseparable from excellent design patterns. After reading this case, I quickly read the four main Agent design patterns recently published by Mr. Andrew Ng. Andrew Ng is one of the most authoritative scholars in the world in the field of artificial intelligence and machine learning. Then, I quickly compiled it and shared it with everyone. Mode 1. Reflection
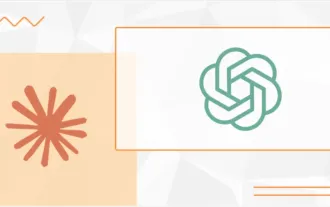
According to the continuous evolution and self-innovation of large language models, performance, accuracy, and stability have been greatly improved, which has been verified by various benchmark problem sets. However, for existing versions of LLM, their comprehensive capabilities do not seem to be able to fully support AI agents. Multi-modal, multi-task, and multi-domain inference have become necessary requirements for AI agents in the public media space, but the actual effects displayed in specific functional practices vary greatly. This seems to once again remind all AI robot startups and large technology giants to recognize the reality: be more down-to-earth, don’t spread your business too big, and start with AI enhancement functions. Recently, a blog about the gap between the propaganda and real performance of AI agents emphasized a point:
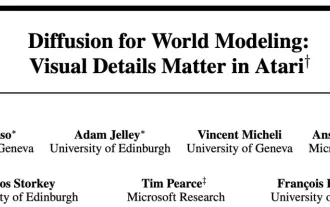
World models provide a way to train reinforcement learning agents in a safe and sample-efficient manner. Recently, world models have mainly operated on discrete latent variable sequences to simulate environmental dynamics. However, this method of compressing into compact discrete representations may ignore visual details that are important for reinforcement learning. On the other hand, diffusion models have become the dominant method for image generation, posing challenges to discrete latent models. Promoted by this paradigm shift, researchers from the University of Geneva, the University of Edinburgh, and Microsoft Research jointly proposed a reinforcement learning agent trained in the diffusion world model-DIAMOND (DIffusionAsaModelOfeNvironmentDreams). Paper address: https:
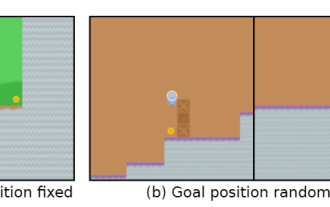
As artificial intelligence systems become more and more advanced, the ability of agents to "exploit loopholes" becomes stronger and stronger. Although they can perfectly perform tasks in the training set, their performance in the test set where there are no shortcuts is a mess. For example, if the game goal is to "eat gold coins", during the training phase, the gold coins are located at the end of each level, and the agent can complete the task perfectly. But in the test phase, the location of the gold coins became random. The agent would choose to reach the end of the level every time instead of looking for the gold coins. That is, the "goal" learned was wrong. The agent unconsciously pursues a goal that the user does not want, also called Goal MisGeneralization (GMG, Goal MisGeneralisation). Goal MisGeneralization is a sign of the lack of robustness of the learning algorithm.
