


Interpretation of the research power behind ChatGPT: The post-90s generation has become the main force, and large manufacturers are no longer the first choice for top AI talents
The popularity of ChatGPT has not only brought capital attention and user favor to OpenAI. In the discussion about "Why OpenAI can make ChatGPT", its personnel advantages have also become the focus of attention from the outside world.
Recently, Wisdom Research and AMiner released a statistical report from the research team behind OpenAI. According to the report, there are 87 people who contributed to the ChatGPT project this time, including those who are "very young", "have a luxurious background", "focus on technology", "have deep accumulation", "advocate entrepreneurship" and "Chinese" Eye-catching” and other distinctive features.
##Report link: https://mp.weixin.qq.com/s/Y_LjjsuoEEmhIg5WO_iQhA
In such a team of less than a hundred people, the phenomenal large-scale language model ChatGPT was born, which is no small feat for major companies such as Google, Microsoft, Baidu, and Alibaba. Pressure has followed closely to release or pre-release ChatGPT-like products.
As a non-profit artificial intelligence research institution, OpenAI has always been regarded as a technological paradise by many young talents interested in developing AI careers. Here, they can directly participate in the most cutting-edge and creative AI projects, mobilize the core scientific research resources, and devote themselves to technological innovation without distraction.
In recent years, marginalization and indecision have caused AI research institutes and scientific research talents in large domestic manufacturers to face survival difficulties. However, I believe that under the impact of ChatGPT, AI talents will regain their competitiveness. Returning to the public eye will also usher in a new round of competition and reshuffle.
1
Technical personnel account for nearly 90%The post-90s generation is the main forcefrom Judging from the job division of the ChatGPT team (Figure 1), among the 87 people participating in this project, the number of R&D personnel reached 77, accounting for 88%, including the company’s co-founder Wojciech Zaremba, who was previously Selected as the 2023 AI 2000 most influential scholar in the field of robotics.
There are 4 product personnel, accounting for 5%. In addition, the position information of 6 participants cannot be obtained.
##Figure 1: ChatGPT team position division
In terms of the age distribution of members (Figure 2), the post-90s generation is the main force of the team. Among them, there are 28 members in the age range of 20-29 years old, accounting for 34%; the largest number of members are in the age range of 30-39 years old, with a total of 28 members. 50 people, accounting for 61%; in addition, there are three people in the age range of 40-49 years old, and only one person is over 60 years old.According to statistics, the average age of this research team is 32 years old.
"Strong age" and "focus on technology" are two significant characteristics of the members of the ChatGPT team.
Although the average age is only 32 years old, the team members are highly focused on technology research and development. Based on their great interest and full dedication in AI innovation and research and development, they have created this new technology that has detonated the world. A phenomenal model of round technology. It can be seen that it is entirely possible for young people who are considered to have insufficient research and development experience to make major breakthroughs in the field of cutting-edge science and technology.
Currently, there is no shortage of young talents like OpenAI in China.
After the advent of ChatGPT, Zhang Jiaxing, a chair scientist at the IDEA Research Institute, quickly shifted the team’s large model development to ChatGPT’s conversational task line at the end of last year.
According to his disclosure, the main research forces in his team are also outstanding young talents born in the 1990s. Currently, the ChatGPT-like model they developed is as effective as ChatGPT, has only 5 billion parameters, and the text generation speed is also very fast. It is currently in internal testing and will be in public testing in the near future.
2
9 people have education from prestigious schools and are Chinese
Big factories are no longer the first choice for top talents
ChatGPT The number of team members with bachelor’s, master’s and doctoral degrees is relatively balanced. Among them, 27 have bachelor’s degrees, 25 have master’s degrees, and 28 have doctoral degrees, accounting for 33% and 30% respectively. ,37%.
Among them, Stanford University has the largest number of alumni, with 14 in total, followed by UC Berkeley with 10, and MIT ranking third with 7.
##Figure 3: Top 10 number of ChatGPT team members graduating from universities
Chinese scholars are an important force in scientific and technological innovation in the team, with a total of 9 people, accounting for nearly 10%.
Among them, 5 people have graduated from Chinese universities, and 3 people have undergraduate degrees from Tsinghua University, namely Weng Jiayi, Zhao Shengjia, and Yuan Qiming. They currently serve as R&D engineers in the team positions; each has one person with a bachelor's degree from Huazhong University of Science and Technology and Peking University/University of Hong Kong, namely Jiang Xu and Weng Lilian.
They all went to the United States for further studies after graduating from top domestic universities and received master's or doctorate degrees.
## Figure 4: ChatGPT Team Chinese members of favored by them. Among the team members, a total of 5 have been named 2023 AI 2000 Global Artificial Intelligence Scholars. They are:
1. OpenAI co-founder Wojciech Zaremba (selected field and ranking: Robotics, 10th place)
2. ChatGPT researcher Lukasz Kaiser (selected field and ranking: machine learning, 10th)
3. OpenAI co-founder and ChatGPT research scientist John Schulman (selected field and ranking: machine learning, No. 41)
4.ChatGPT R&D Engineer Tomer Kaftan (selected field and ranking: database, No. 52)
5.ChatGPT Research scientist Barret Zoph (selected field and ranking: machine learning, No. 95)
The proportion of staff from external companies, recent college graduates, scientific research institutions and university faculty are respectively 81%, 13%, 4% and 3%, most of them are from top or well-known technology companies such as Google, Microsoft, Meta, Intel, NVIDIA, Apple, etc. A total of 10 people joined from Google, and 1 person worked at Baidu Served.
##Figure 5: ChatGPT team member flow
The CodeX project has the largest number of participants, with a total of 22 people participating, accounting for 25% of the team; followed by webGPT and instructGPT, with a total of 9 people participating; GPT3 has a total of 6 people participating, ranking third; The fourth one is RLHF, with 3 people participating.
##Figure 6: The number of people involved in the previous seven major technology R&Ds of the ChatGPT team
It can be said that ChatGPT is the result of OpenAI’s years of technology accumulation in the field of large-scale language models, the gathering of top talent leaders and outstanding AI technical personnel, which has laid a solid foundation for the successful development of ChatGPT.
3
AI talent ushered in the battleIn fact, in the past few years, AI research institutes and AI Talents have long faced the dilemma of marginalization and strategic swings within large companies. Just like Google mentioned above, many talents have flowed to pure scientific research holy places like OpenAI.
AI personnel within large factories often find it difficult to develop their capabilities and achievements within the company’s organizational structure model.
But unlike traditional technology giants, if companies such as OpenAI take "developing artificial intelligence" as their own mission, AI R&D and innovation are their mission, that is, Participate in the most cutting-edge AI projects closely and use the most core resources for research and development. Behind the scenes, you must be able to withstand the responsibility of not producing results for a long time. Among them, the GPT model took up to three years from the first launch to the completion of training, requiring a complete set of systematic guarantees of funds, technology, and talents from the team.
The emergence of ChatGPT has brought AI talents back into the public eye, reiterated the importance of pure scientific research, and is bound to set off a new round of talent competition. At the same time, it will also increase the emphasis of large manufacturers on infrastructure such as large models and computing resources, and accelerate the catching up and complementation of underlying technical capabilities.
As former Sogou CEO Wang Xiaochuan said on Weibo, "The success of OpenAI is first of all the victory of technical idealism." The success of ChatGPT is inevitably inseparable from industry, academia and , research cooperation, behind which is the team members’ interest in artificial intelligence technology and their belief in it. There is no shortage of top scientific research forces in the country. Focusing on cutting-edge technological innovation and proceeding steadily is of great significance to promoting the development of AI innovation in China.
The above is the detailed content of Interpretation of the research power behind ChatGPT: The post-90s generation has become the main force, and large manufacturers are no longer the first choice for top AI talents. For more information, please follow other related articles on the PHP Chinese website!

Hot AI Tools

Undresser.AI Undress
AI-powered app for creating realistic nude photos

AI Clothes Remover
Online AI tool for removing clothes from photos.

Undress AI Tool
Undress images for free

Clothoff.io
AI clothes remover

Video Face Swap
Swap faces in any video effortlessly with our completely free AI face swap tool!

Hot Article

Hot Tools

Notepad++7.3.1
Easy-to-use and free code editor

SublimeText3 Chinese version
Chinese version, very easy to use

Zend Studio 13.0.1
Powerful PHP integrated development environment

Dreamweaver CS6
Visual web development tools

SublimeText3 Mac version
God-level code editing software (SublimeText3)

Hot Topics
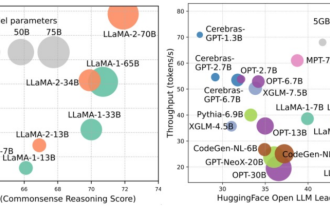
Large-scale language models (LLMs) have demonstrated compelling capabilities in many important tasks, including natural language understanding, language generation, and complex reasoning, and have had a profound impact on society. However, these outstanding capabilities require significant training resources (shown in the left image) and long inference times (shown in the right image). Therefore, researchers need to develop effective technical means to solve their efficiency problems. In addition, as can be seen from the right side of the figure, some efficient LLMs (LanguageModels) such as Mistral-7B have been successfully used in the design and deployment of LLMs. These efficient LLMs can significantly reduce inference memory while maintaining similar accuracy to LLaMA1-33B
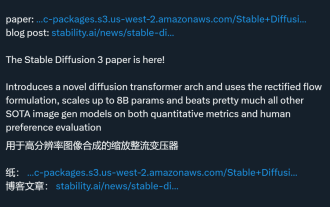
StableDiffusion3’s paper is finally here! This model was released two weeks ago and uses the same DiT (DiffusionTransformer) architecture as Sora. It caused quite a stir once it was released. Compared with the previous version, the quality of the images generated by StableDiffusion3 has been significantly improved. It now supports multi-theme prompts, and the text writing effect has also been improved, and garbled characters no longer appear. StabilityAI pointed out that StableDiffusion3 is a series of models with parameter sizes ranging from 800M to 8B. This parameter range means that the model can be run directly on many portable devices, significantly reducing the use of AI
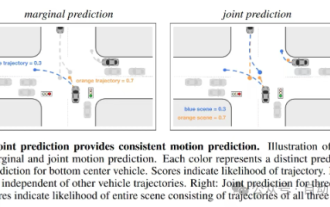
Trajectory prediction plays an important role in autonomous driving. Autonomous driving trajectory prediction refers to predicting the future driving trajectory of the vehicle by analyzing various data during the vehicle's driving process. As the core module of autonomous driving, the quality of trajectory prediction is crucial to downstream planning control. The trajectory prediction task has a rich technology stack and requires familiarity with autonomous driving dynamic/static perception, high-precision maps, lane lines, neural network architecture (CNN&GNN&Transformer) skills, etc. It is very difficult to get started! Many fans hope to get started with trajectory prediction as soon as possible and avoid pitfalls. Today I will take stock of some common problems and introductory learning methods for trajectory prediction! Introductory related knowledge 1. Are the preview papers in order? A: Look at the survey first, p
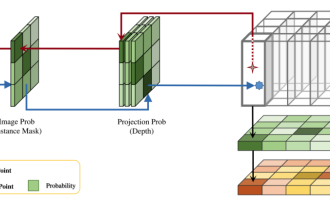
This paper explores the problem of accurately detecting objects from different viewing angles (such as perspective and bird's-eye view) in autonomous driving, especially how to effectively transform features from perspective (PV) to bird's-eye view (BEV) space. Transformation is implemented via the Visual Transformation (VT) module. Existing methods are broadly divided into two strategies: 2D to 3D and 3D to 2D conversion. 2D-to-3D methods improve dense 2D features by predicting depth probabilities, but the inherent uncertainty of depth predictions, especially in distant regions, may introduce inaccuracies. While 3D to 2D methods usually use 3D queries to sample 2D features and learn the attention weights of the correspondence between 3D and 2D features through a Transformer, which increases the computational and deployment time.
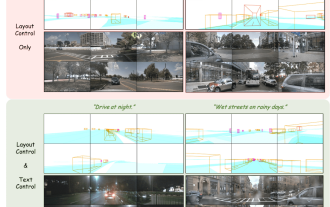
Some of the author’s personal thoughts In the field of autonomous driving, with the development of BEV-based sub-tasks/end-to-end solutions, high-quality multi-view training data and corresponding simulation scene construction have become increasingly important. In response to the pain points of current tasks, "high quality" can be decoupled into three aspects: long-tail scenarios in different dimensions: such as close-range vehicles in obstacle data and precise heading angles during car cutting, as well as lane line data. Scenes such as curves with different curvatures or ramps/mergings/mergings that are difficult to capture. These often rely on large amounts of data collection and complex data mining strategies, which are costly. 3D true value - highly consistent image: Current BEV data acquisition is often affected by errors in sensor installation/calibration, high-precision maps and the reconstruction algorithm itself. this led me to
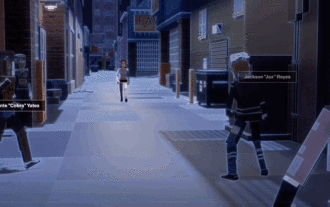
Please note that this square man is frowning, thinking about the identities of the "uninvited guests" in front of him. It turned out that she was in a dangerous situation, and once she realized this, she quickly began a mental search to find a strategy to solve the problem. Ultimately, she decided to flee the scene and then seek help as quickly as possible and take immediate action. At the same time, the person on the opposite side was thinking the same thing as her... There was such a scene in "Minecraft" where all the characters were controlled by artificial intelligence. Each of them has a unique identity setting. For example, the girl mentioned before is a 17-year-old but smart and brave courier. They have the ability to remember and think, and live like humans in this small town set in Minecraft. What drives them is a brand new,
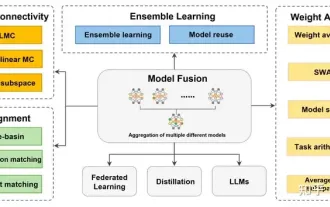
In September 23, the paper "DeepModelFusion:ASurvey" was published by the National University of Defense Technology, JD.com and Beijing Institute of Technology. Deep model fusion/merging is an emerging technology that combines the parameters or predictions of multiple deep learning models into a single model. It combines the capabilities of different models to compensate for the biases and errors of individual models for better performance. Deep model fusion on large-scale deep learning models (such as LLM and basic models) faces some challenges, including high computational cost, high-dimensional parameter space, interference between different heterogeneous models, etc. This article divides existing deep model fusion methods into four categories: (1) "Pattern connection", which connects solutions in the weight space through a loss-reducing path to obtain a better initial model fusion
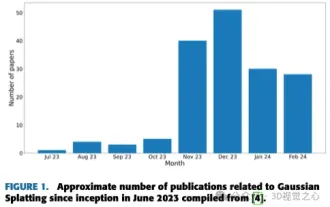
Written above & The author’s personal understanding is that image-based 3D reconstruction is a challenging task that involves inferring the 3D shape of an object or scene from a set of input images. Learning-based methods have attracted attention for their ability to directly estimate 3D shapes. This review paper focuses on state-of-the-art 3D reconstruction techniques, including generating novel, unseen views. An overview of recent developments in Gaussian splash methods is provided, including input types, model structures, output representations, and training strategies. Unresolved challenges and future directions are also discussed. Given the rapid progress in this field and the numerous opportunities to enhance 3D reconstruction methods, a thorough examination of the algorithm seems crucial. Therefore, this study provides a comprehensive overview of recent advances in Gaussian scattering. (Swipe your thumb up
