


Three keys to achieving high-level autonomous driving under the trend of multi-sensor fusion
In order to capture the surrounding environment more accurately and provide performance redundancy, autonomous vehicles are equipped with a large number of complementary sensors, including millimeter wave radar, cameras, lidar, infrared thermal imaging, ultrasonic radar, etc. In order to give full play to the respective advantages of different sensors, high-end intelligent driving perception systems are bound to evolve in the direction of deep fusion of multiple sensors.
Through the fusion of multiple sensors, the autonomous driving system can obtain a more accurate result model, thereby improving the safety and reliability of the autonomous driving system. sex. For example, millimeter-wave radar can make up for the disadvantages of cameras that are affected by rainy days and can identify obstacles that are relatively far away, but cannot identify the specific shape of obstacles; lidar can make up for the shortcomings of millimeter-wave radar that cannot identify the specific shape of obstacles. Therefore, in order to fuse the external data collected from different sensors to provide a basis for the controller to make decisions, it is necessary to process the multi-sensor fusion algorithm to form a panoramic perception.
The following is an introduction to the three key sensors for achieving high-level autonomous driving: 4D millimeter wave radar, lidar and infrared thermal imaging.
4D Millimeter Wave Radar
Millimeter wave radar can be said to be the earliest sensor used in mass production of autonomous driving, although its accuracy is not as high as lidar , but it is still at a high level among many sensor categories. It has strong penetration ability into fog, smoke, and dust. It performs better overall under severe weather conditions. It mainly exists as a ranging and speed sensor. Currently, the number of millimeter wave radars installed on bicycles is still at a low level. From January to August 2022, new passenger cars were delivered with only 0.86 millimeter-wave radars per vehicle.
This is not to say that the performance of traditional millimeter-wave radar is not excellent. For L2-level cars, the stable point cloud collection brought by the high resolution of millimeter-wave radar is necessary for the vehicle to complete 360° The key to environmental awareness. But this is not enough. For L3, L4 and above models, the perception accuracy and fusion effect are greatly reduced. As 4D millimeter-wave radars begin to be put into vehicles this year, 2023 will be the year when large-scale front-mounted mass production truly enters. According to Yelo's forecast, the global 4D millimeter wave radar market will reach US$3.5 billion by 2027.
Currently, the application of 4D imaging radar on the market is mainly in two directions. One is to replace the traditional low-resolution forward radar to meet the multi-sensory fusion performance of high-end intelligent driving. improvement. The second main application scenario is the traveling and parking integrated 4D surround high-resolution (divided into point cloud enhancement and imaging) radar, whose performance will be slightly lower than that of the forward radar.
Lidar
Since this year, "Lidar on the car" has become the latest "label" for automobile intelligence. At the Guangzhou Auto Show , including Xpeng G9, WM7, Nezha S, Salon Mecha Dragon and other more and more models are equipped with lidar. Compared with ordinary radar, lidar has the advantages of high resolution, good concealment, and strong anti-interference ability. It is likened to the "eyes" of autonomous vehicles. It determines the evolutionary level of the autonomous driving industry and is the key to realizing the implementation of autonomous driving. The last mile is an extremely important part of the journey.
Lidar has irreplaceable advantages in high-level autonomous driving that has strict requirements on information accuracy. At present, whether it is new car-making forces, traditional OEMs, or Internet companies, they are all making arrangements, which has led to a sudden increase in demand for lidar production capacity. According to statistics from Zuosi Auto Research, the installation volume of lidar in new domestic passenger cars in H1 will reach 24,700 in 2022; in the second half of 2022, more than 10 new lidar cars will be delivered in China, including Xpeng G9, WM7, etc. , will significantly increase the number of lidars on the vehicle, and the total installed volume is expected to exceed 80,000 units throughout the year.
Infrared Thermal Imaging
Compared with traditional CIS and lidar, infrared thermal imaging can be used in high dynamic range, rainy days, foggy days, dark days, etc. The advantages are obvious in various scenarios such as light and sandstorms, and the introduction of high-level autonomous driving solutions is an inevitable trend. Infrared thermal imaging equipment with integrated infrared detectors is particularly suitable for distinguishing pedestrians and other inanimate obstacles due to its ability to detect heat. It has advantages that other sensors do not have. It is not affected by rain, fog, haze and light conditions, and the observation distance can be up to several hundred meters. In the future, it will occupy a place in the field of autonomous driving.
Previously, the main reason why infrared thermal imaging failed to achieve "onboard use" was that the price remained high. In recent years, with the localization of key raw materials such as infrared thermal imaging chips, costs have dropped, and they have been widely used in the civilian field. Autonomous driving will quickly open up the scale of the infrared detector market. According to data from the China Industrial Research Institute, China's infrared thermal imaging camera market size will reach US$6.68 billion in 2020, and is expected to continue to grow at a compound annual growth rate of 10.8% in 2021. It is expected that China's infrared thermal imaging camera market size will reach US$12.34 billion in 2025 Dollar.
Conclusion: Multi-sensor fusion autonomous driving solutions are an inevitable trend in future automobile development. Fusion of multiple sensor information can make up for the limitations of a single sensor and improve the safety redundancy and data reliability of the autonomous driving system. However, each sensor has different coordinate systems, different data forms, and even different collection frequencies, so the design of the fusion algorithm is not a simple task.
The above is the detailed content of Three keys to achieving high-level autonomous driving under the trend of multi-sensor fusion. For more information, please follow other related articles on the PHP Chinese website!

Hot AI Tools

Undresser.AI Undress
AI-powered app for creating realistic nude photos

AI Clothes Remover
Online AI tool for removing clothes from photos.

Undress AI Tool
Undress images for free

Clothoff.io
AI clothes remover

AI Hentai Generator
Generate AI Hentai for free.

Hot Article

Hot Tools

Notepad++7.3.1
Easy-to-use and free code editor

SublimeText3 Chinese version
Chinese version, very easy to use

Zend Studio 13.0.1
Powerful PHP integrated development environment

Dreamweaver CS6
Visual web development tools

SublimeText3 Mac version
God-level code editing software (SublimeText3)

Hot Topics


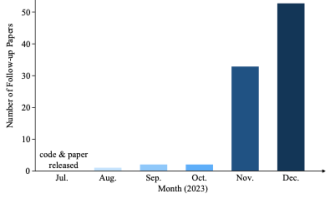
Written above & the author’s personal understanding Three-dimensional Gaussiansplatting (3DGS) is a transformative technology that has emerged in the fields of explicit radiation fields and computer graphics in recent years. This innovative method is characterized by the use of millions of 3D Gaussians, which is very different from the neural radiation field (NeRF) method, which mainly uses an implicit coordinate-based model to map spatial coordinates to pixel values. With its explicit scene representation and differentiable rendering algorithms, 3DGS not only guarantees real-time rendering capabilities, but also introduces an unprecedented level of control and scene editing. This positions 3DGS as a potential game-changer for next-generation 3D reconstruction and representation. To this end, we provide a systematic overview of the latest developments and concerns in the field of 3DGS for the first time.
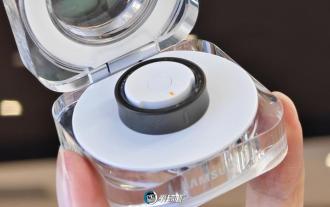
Samsung officially released the national version of Samsung Galaxy Ring on July 17, priced at 2,999 yuan. Galaxy Ring's real phone is really the 2024 version of "WowAwesome, this is my exclusive moment". It is the electronic product that makes us feel the freshest in recent years (although it sounds like a flag) besides Apple's Vision Pro. (In the picture, the rings on the left and right are Galaxy Ring↑) Samsung Galaxy Ring specifications (data from the official website of the Bank of China): ZephyrRTOS system, 8MB storage; 10ATM waterproof + IP68; battery capacity 18mAh to 23.5mAh (different sizes
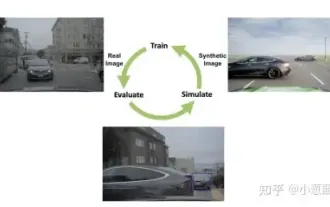
Yesterday during the interview, I was asked whether I had done any long-tail related questions, so I thought I would give a brief summary. The long-tail problem of autonomous driving refers to edge cases in autonomous vehicles, that is, possible scenarios with a low probability of occurrence. The perceived long-tail problem is one of the main reasons currently limiting the operational design domain of single-vehicle intelligent autonomous vehicles. The underlying architecture and most technical issues of autonomous driving have been solved, and the remaining 5% of long-tail problems have gradually become the key to restricting the development of autonomous driving. These problems include a variety of fragmented scenarios, extreme situations, and unpredictable human behavior. The "long tail" of edge scenarios in autonomous driving refers to edge cases in autonomous vehicles (AVs). Edge cases are possible scenarios with a low probability of occurrence. these rare events
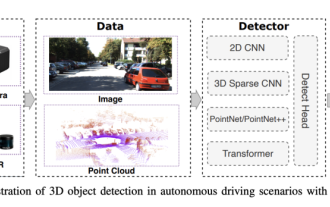
0.Written in front&& Personal understanding that autonomous driving systems rely on advanced perception, decision-making and control technologies, by using various sensors (such as cameras, lidar, radar, etc.) to perceive the surrounding environment, and using algorithms and models for real-time analysis and decision-making. This enables vehicles to recognize road signs, detect and track other vehicles, predict pedestrian behavior, etc., thereby safely operating and adapting to complex traffic environments. This technology is currently attracting widespread attention and is considered an important development area in the future of transportation. one. But what makes autonomous driving difficult is figuring out how to make the car understand what's going on around it. This requires that the three-dimensional object detection algorithm in the autonomous driving system can accurately perceive and describe objects in the surrounding environment, including their locations,
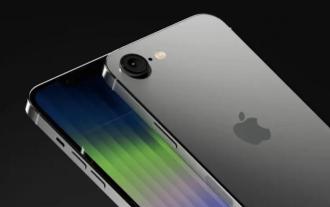
Recently, new news about iPhone SE4 was revealed on Weibo. It is said that the back cover process of iPhone SE4 is exactly the same as that of the iPhone 16 standard version. In other words, iPhone SE4 will use a glass back panel and a straight screen and straight edge design. It is reported that iPhone SE4 will be released in advance to September this year, which means it is likely to be unveiled at the same time as iPhone 16. 1. According to the exposed renderings, the front design of iPhone SE4 is similar to that of iPhone 13, with a front camera and FaceID sensor on the notch screen. The back uses a layout similar to the iPhoneXr, but it only has one camera and does not have an overall camera module.
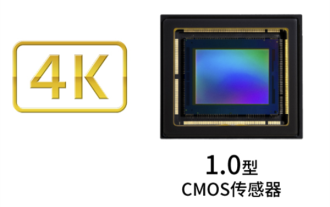
Yesterday's article didn't mention "sensor size". I didn't expect people to have so many misunderstandings... How much is 1 inch? Because of some historical issues*, whether it is a camera or a mobile phone, "1 inch" in the diagonal length of the sensor is not 25.4mm. *When it comes to vacuum tubes, there is no expansion here. It is a bit like a horse’s butt deciding the width of a railroad track. In order to avoid misunderstanding, the more rigorous writing is "Type 1.0" or "Type1.0". Moreover, when the sensor size is less than 1/2 type, type 1 = 18mm; and when the sensor size is greater than or equal to 1/2 type, type 1 =
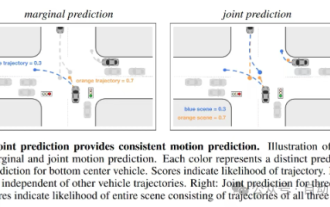
Trajectory prediction plays an important role in autonomous driving. Autonomous driving trajectory prediction refers to predicting the future driving trajectory of the vehicle by analyzing various data during the vehicle's driving process. As the core module of autonomous driving, the quality of trajectory prediction is crucial to downstream planning control. The trajectory prediction task has a rich technology stack and requires familiarity with autonomous driving dynamic/static perception, high-precision maps, lane lines, neural network architecture (CNN&GNN&Transformer) skills, etc. It is very difficult to get started! Many fans hope to get started with trajectory prediction as soon as possible and avoid pitfalls. Today I will take stock of some common problems and introductory learning methods for trajectory prediction! Introductory related knowledge 1. Are the preview papers in order? A: Look at the survey first, p

Original title: SIMPL: ASimpleandEfficientMulti-agentMotionPredictionBaselineforAutonomousDriving Paper link: https://arxiv.org/pdf/2402.02519.pdf Code link: https://github.com/HKUST-Aerial-Robotics/SIMPL Author unit: Hong Kong University of Science and Technology DJI Paper idea: This paper proposes a simple and efficient motion prediction baseline (SIMPL) for autonomous vehicles. Compared with traditional agent-cent
