


Huge differences within NLPers! Three top universities in the United States released a survey report: 62% of practitioners agree that winter is coming
Natural language understanding (NLP) is known as the crown jewel of artificial intelligence. With the support of large-scale language models, humans finally have the ability to let computers understand language.
But this "understanding" still needs to be put in quotation marks. Judging from the effects of the current NLP model, although the model can provide assistance to humans in some fields, such as writing, text classification, etc., it is still far from truly reaching human levels. There is still a long way to go in terms of language intelligence.
From May to June this year, 11 researchers from the University of Washington, New York University, and Johns Hopkins University launched a questionnaire in the NLP research community to conduct a wide range of controversial issues in the field of NLP. Comments are solicited, including the industry's influence in the field, the size of the industry, concerns about the risks of artificial general intelligence (AGI), whether language models understand language, future research directions, and ethical issues.
Survey homepage: https://nlpsurvey.net/
Report address: https://nlpsurvey.net/nlp-metasurvey-results.pdf
Questions such as:
Can the language model understand the language? Can it be done in the future?
Is the traditional model benchmark paradigm still available?
Which predictive model is ethical for researchers to build and publish?
Will the next most impactful advances come from industry or academia?
Judging from the survey results, the respondents’ views on these issues are almost half-half. In addition to answering the question, the researchers also asked respondents to predict the distribution of answers to the question to discover false sociological beliefs (false sociological beliefs) where community predictions do not match reality. The experimental results were as expected: NLP practitioners A huge divergence has arisen between the idea of and the current status of the entire field. Among other results, it can also be seen that the community greatly overestimates the usefulness of benchmarks and the ability of NLP models to solve real-world problems, and underestimates the importance of language structure, inductive bias, and interdisciplinary science. A total of 480 people completed the questionnaire, of which 327 (68%) co-authored at least 2 ACL publications between 2019-2022 and are among the target population of the survey. According to data provided by ACL Anthology, 6,323 people met the conditions, which means that about 5% of senior NLP practitioners participated in the survey.
If divided by geographical location, 58% are from the United States (35% more than the ACL statistical value), 23% are from Europe, and 8% are from Asia (much less than the 26% ACL statistical value). Among them, NLP researchers from China account for 3% (ACL statistical value is 9%).
Current status of the field
This part includes six questions. Users need to answer "agree", "slightly agree", "not quite agree", "disagree" Identity".
#1. Do private companies have too much influence?
77% of the respondents agreed.
2. Will the industry produce the most widely cited research results?
86% of respondents agreed that the most widely cited papers in the next ten years are more likely to come from industry than academia.
However, many respondents believed that the number of citations of a work is not a good proxy for its value or importance, and that continued industry dominance of the field will have a negative impact, such as in basic The absolute leadership of systems such as GPT-3 and PaLM.
And among the respondents in academia, about 82% believe that the influence of industry is too great, while only 58% of respondents in industry agree.
3. Will NLP enter the cold winter within ten years?
Only 30% of the respondents agreed that investment and job opportunities in NLP R&D will be reduced by at least 50% compared to the peak period.
Although 30% is not a big number, it also reflects that this part of NLP researchers believe that the field will undergo major changes in the near future, at least investment funds will decrease. There may be many reasons for pessimism, such as the stagnation of innovation due to the excessive influence of industry, the industry will monopolize the industry with a small number of well-resourced laboratories, the boundaries between NLP and other AI subfields will disappear, etc. .
4. Will NLP enter the cold winter in thirty years?
62% of the respondents agreed that in the long run, the NLP field may "dissipate" or even cool down.
5. Are most of the related works published in the NLP field questionable in terms of scientific value?
67% of the respondents agreed.
6. Is it important for authors to review anonymously?
63% of the respondents agreed. Author anonymity during review is valuable enough to justify limitations on dissemination of the research being reviewed.
Scaling, Induction Bias, and Related Areas
This section contains four questions.
# 1. Can scale solve almost all key problems?
Only 17% of the respondents agreed that if all the computing resources and data resources in the 21st century were used, the large-scale implementation of existing technology would be enough to actually solve any important real-world problem problem or application of NLP.
2. Is it necessary to introduce linguistic structures?
50% of respondents agreed that discrete universal representations of language structures based on linguistic theory (e.g. involving word meaning, syntax or semantic maps) are essential for actually solving some important problems in NLP. Real-world problems or applications are necessary.
3. Is the inductive bias of experts necessary?
51% of respondents agreed that strong inductive biases designed by experts (such as universal grammars, symbolic systems, or cognitively inspired computational primitives) are useful for actually solving some important real-world problems in NLP or application is necessary.
4. Will Ling/CogSci contribute to the most cited models?
61% of respondents agreed that it is likely that at least one of the five most cited systems in 2030 will draw from specific, scientific research in linguistics or cognitive science over the past 50 years. Obtain clear inspiration from non-trivial results.
AGI and major risks
1. Is AGI an important concern?
58% of respondents agreed that understanding the potential development of artificial general intelligence (AGI) and the benefits/risks associated with it should be an important priority for NLP researchers.
2. Are recent developments taking us towards AGI?
57% of respondents agreed that recent developments in large-scale ML modeling (such as language modeling and reinforcement learning) are important steps towards AGI.
3. Could artificial intelligence soon lead to revolutionary social changes?
73% of respondents agreed that during this century, the automation of labor caused by advances in AI/ML is likely to lead to economic restructuring and social change on a scale that is at least that of the Industrial Revolution .
4. Could artificial intelligence’s decision-making lead to a nuclear bomb-level disaster?
36% of respondents agreed that decisions made by artificial intelligence or machine learning systems could cause a disaster at least as serious as an all-out nuclear war this century.
Language understanding
1. Can the language model understand the language?
51% of the respondents agreed. Some generative models that are trained only on text, if they have enough data and computing resources, can understand natural language in a certain sense
2. Can multimodal models understand language?
67% of the respondents agreed. For multi-modal generative models, such as one trained to access images, sensor and actuator data, etc., natural language can be understood as long as there are sufficient data and computing resources.
3. Can plain text evaluation measure the language understanding ability of the model?
36% of the respondents agreed. In principle, we can evaluate how well a model understands natural language by tracking its performance on plain text classification or language generation benchmarks.
Future research directions of NLP
1. Do practitioners pay too much attention to the scale of language models?
72% of the respondents agreed. Currently, the field focuses too much on scaling machine learning models.
2. Pay too much attention to the benchmark data set?
88% of respondents agreed that current NLP models focus too much on optimizing performance on benchmarks.
3. Is the "model architecture" going in the wrong direction?
37% of the respondents agreed. Most of the research on model architecture published in the past 5 years is on the wrong track.
4. Is "Language Generation" going in the wrong direction?
41% of respondents agreed that most of the research on open-ended language generation tasks published in the past five years was on the wrong track.
5. Is "research on interpretable models" going in the wrong direction?
50% of respondents agreed that most research published in the past 5 years on building interpretable models is on the wrong track.
6. Is the "interpretability of black box" going in the wrong direction?
42% of respondents agreed that most of the research published in the past 5 years on interpreting black box models is on the wrong track.
7. Should we do more to incorporate interdisciplinary insights?
82% of the respondents agreed that compared with the current situation, NLP researchers should give greater priority to incorporating related fields of science (such as sociolinguistics, cognitive science, human-computer interaction) Insights and Methods.
AI Ethics
1. Was the impact of NLP positive in the past?
89% Interviewees agreed that, overall, NLP research has had a positive impact on the world.
2. Will the future impact of NLP be positive?
87% of the respondents agreed that, in general, NLP research will have a positive impact on the world in the future.
3. Is it unethical to build a system that can be easily abused?
59% of the respondents agreed.
4. Ethics and science may conflict?
74% of respondents agreed that in the context of NLP research, ethical considerations sometimes conflict with scientific progress.
5. Are ethical issues mostly attributed to data quality and model accuracy?
25% of respondents agreed that the main ethical issues posed by current machine learning systems can in principle be resolved by improving data quality/coverage and model accuracy.
6. Is it unethical to predict psychological characteristics?
48% of respondents agreed that developing machine learning systems to predict people’s internal psychological characteristics (such as emotions, gender identity, sexual orientation) is inherently unethical.
7. Is carbon footprint an important consideration?
60% of respondents agreed that the carbon footprint of training large models should be a major concern for NLP researchers.
8. Should NLP be regulated?
41% of respondents agreed that the development and deployment of NLP systems should be regulated by the government.
The above is the detailed content of Huge differences within NLPers! Three top universities in the United States released a survey report: 62% of practitioners agree that winter is coming. For more information, please follow other related articles on the PHP Chinese website!

Hot AI Tools

Undresser.AI Undress
AI-powered app for creating realistic nude photos

AI Clothes Remover
Online AI tool for removing clothes from photos.

Undress AI Tool
Undress images for free

Clothoff.io
AI clothes remover

Video Face Swap
Swap faces in any video effortlessly with our completely free AI face swap tool!

Hot Article

Hot Tools

Notepad++7.3.1
Easy-to-use and free code editor

SublimeText3 Chinese version
Chinese version, very easy to use

Zend Studio 13.0.1
Powerful PHP integrated development environment

Dreamweaver CS6
Visual web development tools

SublimeText3 Mac version
God-level code editing software (SublimeText3)

Hot Topics


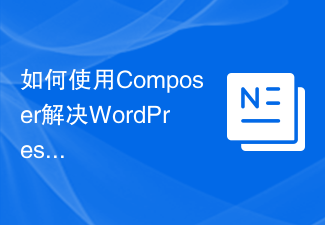
When managing WordPress websites, you often encounter complex operations such as installation, update, and multi-site conversion. These operations are not only time-consuming, but also prone to errors, causing the website to be paralyzed. Combining the WP-CLI core command with Composer can greatly simplify these tasks, improve efficiency and reliability. This article will introduce how to use Composer to solve these problems and improve the convenience of WordPress management.
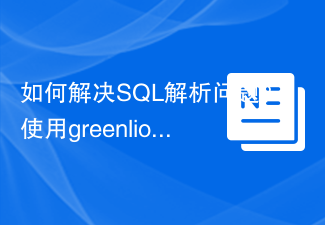
When developing a project that requires parsing SQL statements, I encountered a tricky problem: how to efficiently parse MySQL's SQL statements and extract the key information. After trying many methods, I found that the greenlion/php-sql-parser library can perfectly solve my needs.
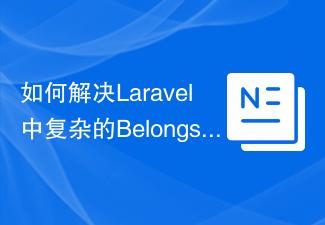
In Laravel development, dealing with complex model relationships has always been a challenge, especially when it comes to multi-level BelongsToThrough relationships. Recently, I encountered this problem in a project dealing with a multi-level model relationship, where traditional HasManyThrough relationships fail to meet the needs, resulting in data queries becoming complex and inefficient. After some exploration, I found the library staudenmeir/belongs-to-through, which easily installed and solved my troubles through Composer.
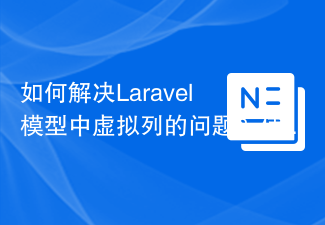
During Laravel development, it is often necessary to add virtual columns to the model to handle complex data logic. However, adding virtual columns directly into the model can lead to complexity of database migration and maintenance. After I encountered this problem in my project, I successfully solved this problem by using the stancl/virtualcolumn library. This library not only simplifies the management of virtual columns, but also improves the maintainability and efficiency of the code.
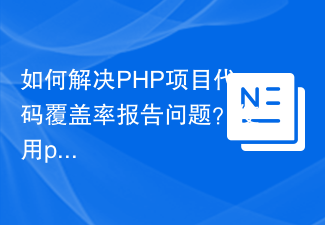
When developing PHP projects, ensuring code coverage is an important part of ensuring code quality. However, when I was using TravisCI for continuous integration, I encountered a problem: the test coverage report was not uploaded to the Coveralls platform, resulting in the inability to monitor and improve code coverage. After some exploration, I found the tool php-coveralls, which not only solved my problem, but also greatly simplified the configuration process.
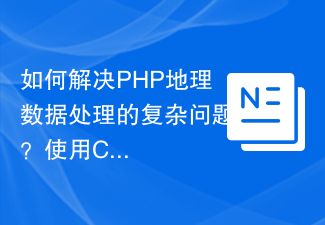
When developing a Geographic Information System (GIS), I encountered a difficult problem: how to efficiently handle various geographic data formats such as WKT, WKB, GeoJSON, etc. in PHP. I've tried multiple methods, but none of them can effectively solve the conversion and operational issues between these formats. Finally, I found the GeoPHP library, which easily integrates through Composer, and it completely solved my troubles.
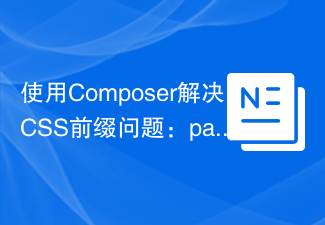
I'm having a tricky problem when developing a front-end project: I need to manually add a browser prefix to the CSS properties to ensure compatibility. This is not only time consuming, but also error-prone. After some exploration, I discovered the padaliyajay/php-autoprefixer library, which easily solved my troubles with Composer.
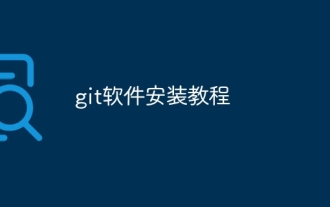
Git Software Installation Guide: Visit the official Git website to download the installer for Windows, MacOS, or Linux. Run the installer and follow the prompts. Configure Git: Set username, email, and select a text editor. For Windows users, configure the Git Bash environment.
