How to deploy machine learning models on AWS Lambda using Docker
In this tutorial, we'll walk you through the process of packaging your ML model as a Docker container and deploying it on the serverless computing service AWS Lambda.
At the end of this tutorial, you will have a working ML model that can be called through the API, and you will have a deeper understanding of how to deploy ML models on the cloud. Whether you're a machine learning engineer, data scientist, or developer, this tutorial is designed to be accessible to anyone with a basic understanding of ML and Docker. So, let’s get started!
What is Docker?
Docker is a tool designed to make it easier to create, deploy, and run applications using containers. Containers allow developers to package an application together with all the parts it needs, such as libraries and other dependencies, and send it out as one package. By using containers, developers can ensure that their applications will run on any other machine, regardless of any custom settings the machine may have that may differ from the machine used to write and test the code. Docker provides a way to package applications and their dependencies into lightweight, portable containers that can be easily moved from one environment to another. This makes it easier to create consistent development, test, and production environments and deploy applications faster and more reliably. Install Docker from here: https://docs.docker.com/get-docker/.
What is AWS Lambda?
Amazon Web Services (AWS) Lambda is a serverless computing platform that runs code in response to events and automatically manages underlying computing resources for you. It is a service provided by AWS that allows developers to run their code in the cloud without having to worry about the infrastructure required to run the code. AWS Lambda automatically scales your application in response to incoming request traffic, and you only pay for the compute time you consume. This makes it an attractive choice for building and running microservices, real-time data processing, and event-driven applications.
What is AWS ECR?
Amazon Web Services (AWS) Elastic Container Registry (ECR) is a fully managed Docker container registry that allows developers to easily store, manage, and deploy Docker container images. It is a secure and scalable service that enables developers to store and manage Docker images in the AWS cloud and easily deploy them to Amazon Elastic Container Service (ECS) or other cloud-based container orchestration platforms. ECR integrates with other AWS services, such as Amazon ECS and Amazon EKS, and provides native support for the Docker command line interface (CLI). This makes it easy to use familiar Docker commands to push and pull Docker images from ECR and automate the process of building, testing, and deploying containerized applications.
Installing the AWS CLI
Use this to install the AWS CLI on your system. Obtain the AWS access key ID and AWS secret access key by creating an IAM user in your AWS account. After installation, run the following command to configure your AWS CLI and insert the required fields.
aws configure
Deploying a Lambda function using Docker
We will deploy the OpenAI clip model to vectorize input text in this tutorial. The Lambda function requires amazon Linux 2 in a Docker container, so we use
public.ecr.aws/lambda/python:3.8. Additionally, since Lambda has a read-only file system, it does not allow us to download models internally, so we need to download and copy them when creating the image.
Get the working code from here and extract it.
Change the working directory where the Dockerfile is located and run the following command:
docker build -t lambda_image .
Now we have the image ready to be deployed on Lambda. To check it locally, run the command:
docker run -p 9000:8080 lambda_image
To check it, send it a curl request and it should return a vector of input text:
curl -XPOST "http://localhost:9000/2015-03-31/functions/function/invocations" -d '{"text": "This is a test for text encoding"}'
Output
First deploy the image to Lambda, we need to push it to ECR, so log in to the AWS account and create the warehouse lambda_image in ECR. After creating the repository, go to the created repository and you will see the view push command option click on it and you will get the command to push the image to the repository.
Now run the first command to authenticate your Docker client using the AWS CLI.
We have already created the Docker image, so skip the second step and run the third command to mark the created image.
运行最后一条命令将镜像推送到 ECR 中。运行后你会看到界面是这样的:
推送完成后,您将在 ECR 的存储库中看到带有“:latest”标签的图像。
复制图像的 URI。我们在创建 Lambda 函数时需要它。
现在转到 Lambda 函数并单击“创建函数”选项。我们正在从图像创建一个函数,因此选择容器图像的选项。添加函数名称并粘贴我们从 ECR 复制的 URI,或者您也可以浏览图像。选择architecture x84_64,最后点击create_image选项。
构建 Lambda 函数可能需要一些时间,请耐心等待。执行成功后,你会看到如下界面:
Lambda 函数默认有 3 秒的超时限制和 128 MB 的 RAM,所以我们需要增加它,否则它会抛出错误。为此,请转到配置选项卡并单击“编辑”。
现在将超时设置为 5-10 分钟(最大限制为 15 分钟)并将 RAM 设置为 2-3 GB,然后单击保存按钮。更新 Lambda 函数的配置需要一些时间。
更新更改后,该功能就可以进行测试了。要测试 lambda 函数,请转到“测试”选项卡并将键值添加到事件 JSON 中作为文本:“这是文本编码测试。” 然后点击测试按钮。
由于我们是第一次执行 Lambda 函数,因此执行可能需要一些时间。成功执行后,您将在执行日志中看到输入文本的向量。
现在我们的 Lambda 函数已部署并正常工作。要通过 API 访问它,我们需要创建一个函数 URL。
要为 Lambda 函数创建 URL,请转到 Configuration 选项卡并选择 Function URL 选项。然后单击创建函数 URL 选项。
现在,保留身份验证 None 并单击 Save。
该过程完成后,您将获得用于通过 API 访问 Lambda 函数的 URL。以下是使用 API 访问 Lambda 函数的示例 Python 代码:
import requests function_url = ""url = f"{function_url}?text=this is test text" payload={}headers = {} response = requests.request("GET", url, headers=headers, data=payload) print(response.text)
成功执行代码后,您将获得输入文本的向量。
所以这是一个如何使用 Docker 在 AWS Lambda 上部署 ML 模型的示例。如果您有任何疑问,请告诉我们。
The above is the detailed content of How to deploy machine learning models on AWS Lambda using Docker. For more information, please follow other related articles on the PHP Chinese website!

Hot AI Tools

Undresser.AI Undress
AI-powered app for creating realistic nude photos

AI Clothes Remover
Online AI tool for removing clothes from photos.

Undress AI Tool
Undress images for free

Clothoff.io
AI clothes remover

AI Hentai Generator
Generate AI Hentai for free.

Hot Article

Hot Tools

Notepad++7.3.1
Easy-to-use and free code editor

SublimeText3 Chinese version
Chinese version, very easy to use

Zend Studio 13.0.1
Powerful PHP integrated development environment

Dreamweaver CS6
Visual web development tools

SublimeText3 Mac version
God-level code editing software (SublimeText3)

Hot Topics
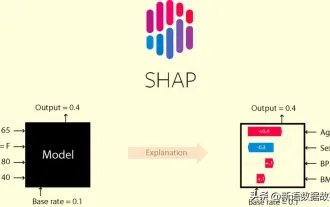
In the fields of machine learning and data science, model interpretability has always been a focus of researchers and practitioners. With the widespread application of complex models such as deep learning and ensemble methods, understanding the model's decision-making process has become particularly important. Explainable AI|XAI helps build trust and confidence in machine learning models by increasing the transparency of the model. Improving model transparency can be achieved through methods such as the widespread use of multiple complex models, as well as the decision-making processes used to explain the models. These methods include feature importance analysis, model prediction interval estimation, local interpretability algorithms, etc. Feature importance analysis can explain the decision-making process of a model by evaluating the degree of influence of the model on the input features. Model prediction interval estimate
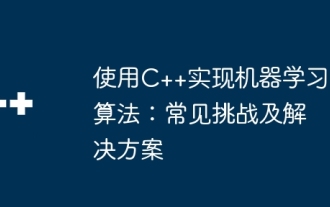
Common challenges faced by machine learning algorithms in C++ include memory management, multi-threading, performance optimization, and maintainability. Solutions include using smart pointers, modern threading libraries, SIMD instructions and third-party libraries, as well as following coding style guidelines and using automation tools. Practical cases show how to use the Eigen library to implement linear regression algorithms, effectively manage memory and use high-performance matrix operations.
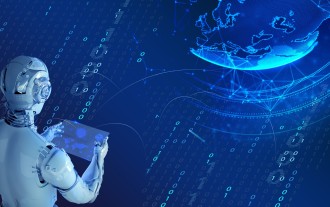
Translator | Reviewed by Li Rui | Chonglou Artificial intelligence (AI) and machine learning (ML) models are becoming increasingly complex today, and the output produced by these models is a black box – unable to be explained to stakeholders. Explainable AI (XAI) aims to solve this problem by enabling stakeholders to understand how these models work, ensuring they understand how these models actually make decisions, and ensuring transparency in AI systems, Trust and accountability to address this issue. This article explores various explainable artificial intelligence (XAI) techniques to illustrate their underlying principles. Several reasons why explainable AI is crucial Trust and transparency: For AI systems to be widely accepted and trusted, users need to understand how decisions are made
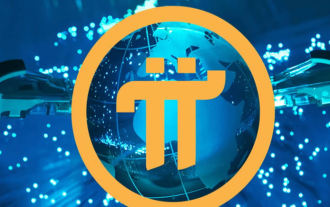
Detailed explanation and installation guide for PiNetwork nodes This article will introduce the PiNetwork ecosystem in detail - Pi nodes, a key role in the PiNetwork ecosystem, and provide complete steps for installation and configuration. After the launch of the PiNetwork blockchain test network, Pi nodes have become an important part of many pioneers actively participating in the testing, preparing for the upcoming main network release. If you don’t know PiNetwork yet, please refer to what is Picoin? What is the price for listing? Pi usage, mining and security analysis. What is PiNetwork? The PiNetwork project started in 2019 and owns its exclusive cryptocurrency Pi Coin. The project aims to create a one that everyone can participate
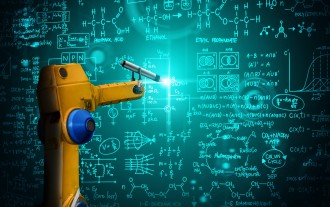
Machine learning is an important branch of artificial intelligence that gives computers the ability to learn from data and improve their capabilities without being explicitly programmed. Machine learning has a wide range of applications in various fields, from image recognition and natural language processing to recommendation systems and fraud detection, and it is changing the way we live. There are many different methods and theories in the field of machine learning, among which the five most influential methods are called the "Five Schools of Machine Learning". The five major schools are the symbolic school, the connectionist school, the evolutionary school, the Bayesian school and the analogy school. 1. Symbolism, also known as symbolism, emphasizes the use of symbols for logical reasoning and expression of knowledge. This school of thought believes that learning is a process of reverse deduction, through existing
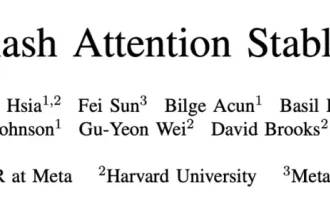
MetaFAIR teamed up with Harvard to provide a new research framework for optimizing the data bias generated when large-scale machine learning is performed. It is known that the training of large language models often takes months and uses hundreds or even thousands of GPUs. Taking the LLaMA270B model as an example, its training requires a total of 1,720,320 GPU hours. Training large models presents unique systemic challenges due to the scale and complexity of these workloads. Recently, many institutions have reported instability in the training process when training SOTA generative AI models. They usually appear in the form of loss spikes. For example, Google's PaLM model experienced up to 20 loss spikes during the training process. Numerical bias is the root cause of this training inaccuracy,
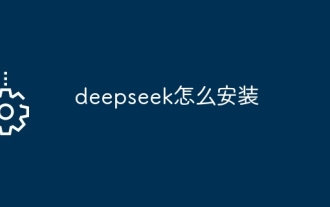
There are many ways to install DeepSeek, including: compile from source (for experienced developers) using precompiled packages (for Windows users) using Docker containers (for most convenient, no need to worry about compatibility) No matter which method you choose, Please read the official documents carefully and prepare them fully to avoid unnecessary trouble.
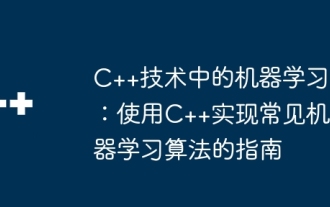
In C++, the implementation of machine learning algorithms includes: Linear regression: used to predict continuous variables. The steps include loading data, calculating weights and biases, updating parameters and prediction. Logistic regression: used to predict discrete variables. The process is similar to linear regression, but uses the sigmoid function for prediction. Support Vector Machine: A powerful classification and regression algorithm that involves computing support vectors and predicting labels.
