How will we conduct information searches in the future?
Guest | Dou Zhicheng
Organization | Zhang Feng
Planning | Xu Jiecheng
It has been more than 20 years since the birth of search engines, and their form and structure have not changed much. With the continuous development of Internet technology, the search environment in the future will become more complex and diverse, and the way users obtain information will also undergo many changes. Various input forms such as natural language, voice, and vision will inevitably replace simple keywords; Multiple modal content outputs such as answers, high-level knowledge, analysis results, and generated content will replace the simple result list; the interaction method may also transition from a single round of retrieval to multiple rounds of natural language interaction.
So in the new search environment, what characteristics will future intelligent search technology show? Recently, at the AISummit Global Artificial Intelligence Technology Conference hosted by 51CTO, Mr. Dou Zhicheng, Vice Dean of Hillhouse School of Artificial Intelligence, Renmin University of China Through the keynote speech - "Next Generation Intelligent Search Technology", the development trends and core features of the new generation of intelligent search technology were shared with the audience. At the same time, interactive, multi-modal, explainable search, and large model-centered De-indexed search and other technologies have been analyzed in detail. This article has edited and organized the content of Mr. Dou Zhicheng’s speech, hoping to bring you some new inspiration:
Main features of future search
We think the future Search may have at least these five characteristics:
- Conversational, People and search engines are a multi-round interaction through natural language way.
- Personalization, will feedback different results according to the needs of different users, instead of giving the same results to everyone in a cookie-cutter manner.
- Multi-modal, The returned content and input method may not be limited to using text as a medium or method.
- Rich knowledge, The information returned by the search is not only in the form of a result list, but may be in various display forms, with various knowledge, Physically displayed.
- De-indexing,Inverted index or dense index also urgently needs to make big changes.
Conversational
The commonly used mode of search engines nowadays is to enter one or two words in a box to search. The future of search may involve us interacting with search engines in a conversational manner.
In the keyword retrieval method used by traditional search engines, we hope to describe all the core information we are looking for through keywords, that is, we assume that a single query can complete and accurately Express the need for this information. But when expressing a more complex information, keywords are actually difficult to meet the needs. Conversational search can fully express information needs through multiple rounds of interaction, which is more in line with the progressive information interaction method when people communicate.
Wanting to achieve this kind of interactive search will bring great challenges to the system or algorithm. It is necessary for the search engine to accurately understand the user's intention from multiple rounds of natural language interaction. , and at the same time, it is necessary to match the understood intention with the information the user wants.
Compared with traditional keyword search, conversational search requires more complex query understanding (such as the need to solve omissions, coreferences, etc. in the current query) to restore the user's True search intent. The simplest way is to stitch together all historical queries and encode them using a pre-trained language model.
Although the simple splicing dialogue method is simple, it may introduce noise. Not all historical queries are helpful for understanding the current query, so we only select the ones that are related to it. Dependency context, which can also solve the length problem.
Conversational retrieval model COTED
Based on the above ideas, we proposed the conversational dense retrieval model COTED, which mainly includes the following three parts:
1. By identifying dependencies in dialogue queries, we can remove the noise in the dialogue and better predict the user's intention.
2. Data enhancement (imitating various noise situations) and denoising loss function based on contrastive learning effectively allow the model to learn to ignore irrelevant context and combine it with the final matching loss function Joint, multitasking learning.
3. Reduce the learning difficulty of model multi-task learning through course learning, and ultimately improve model performance.
However, the data sufficient for training the conversational search model is actually very limited. In the case of limited few samples, the conversational search model Search model training is very difficult.
how to solve this problem? The starting point is whether search engine logs can be migrated for conversational search engine training. Based on this idea, large-scale web search logs are converted into conversational search logs, and then a conversational search model is trained on the converted data. But this method is also accompanied by two obvious problems:
First, traditional web search uses keyword search, and conversational search is a natural language conversation method. The query form is different and cannot be directly migrated. Second, there is a lot of noise in the query itself, and the user data in the search log needs to be cleaned, filtered, and converted before it can be used in conversational search.
Conversational search training model ConvTrans
In order to solve these problems, we made the conversational search training model ConvTrans and implemented the following functions.
First of all, the logs in traditional web search engines are organized in a graph, and the graph is constructed by establishing connections between queries and queries, queries and documents. On the basis of the graph, a two-stage query rewriting model based on T5 is used to rewrite a keyword query into the form of a question. After rewriting, each query in the graph will use natural language to express the new query, and then design a sampling algorithm to do a random walk on the graph to generate a conversation session, and then train the conversation model based on this data.
Experiments show that conversational search models trained with this automatically generated training data can achieve the same effect as using expensive artificial or manually labeled data, and as time goes by As the size of automatically generated training data increases, performance will continue to improve. This approach makes it possible to train conversational search models based on large-scale search logs.
Although the conversational search model has taken a big step forward in search, this conversational method is still passive, and search engines have always been passive. It accepts user input and returns results based on the input. The search engine does not actively ask the user what exactly you are looking for. But in the process of communication between people, when you are asked a question, sometimes you will take the initiative to ask some questions for clarification.
For example, in Bing search, if the Query is "Headaches", it will be a headache. It will ask you "What do want to know about this medical condition", "What do you want to know about this disease", such as its symptoms, treatment, diagnosis, causes or triggers. Because Headaches itself is a very broad Query, in this case, the system hopes to further clarify the information you want to find.
There are two problems here. The first is the candidate item, which specific item you want the user to clarify. The second is to clarify the question. The search engine takes the initiative to ask the user this question. The core word is the most crucial part of clarifying the problem.
In this aspect of exploration, the first is to generate some clarification candidates when a query is given through query logs and knowledge bases. Second, some core words of this clarification question can be predicted from the search results based on rules. At the same time, some data are also labeled, and a supervised model is used to classify text labels. Third, further train an end-to-end generative model based on this annotated data.
Personalization
Personalization refers to Future search will be user-centered. Today's search engines, no matter who searches, return the same results. This does not meet the specific information needs of users.
The current personalized search model adopts a model that first learns knowledge and information that the user is familiar with through user history, and performs personalized entity disambiguation on the query. Secondly, personalized matching is enhanced through disambiguated query entities.
In addition, we have also explored the construction of users' multi-interest models based on product categories. It is assumed that users may have some brand (specification, model) tendencies across all categories, but this tendency cannot be simple. is characterized by one or two vectors. A knowledge graph should be constructed based on the user's shopping history, and different interests for different categories should be learned through the knowledge graph, and ultimately more accurate personalized search results can be pushed.
You can also use the same personalized method to build a chatbot. The core idea is to learn the user’s personalized interests and language patterns through the user’s historical conversations, and train a personalized dialogue model that can be imitated. The (agent) user speaks.
Multimodal
Today’s search engines actually have quite a few limitations when processing multimodal information. In the future, the information users obtain may not only be some text and web pages, but may also include pictures, videos and more complex structural information. Therefore, future search engines still have a lot of work to do in acquiring multi-modal information.
The current search engine still has many flaws when it comes to understanding or performing cross-modal retrieval, that is, giving a text description and looking for its corresponding picture. of. If similar searches are migrated to mobile phones, the limitations will be even greater.
The so-called multi-modal means that the language, images, pictures, videos and other modalities you are looking for are mapped to a unified space, which means that you can find pictures through text. , pictures to find text, pictures to find pictures, etc.
In this regard, we made a large-scale multi-modal pre-training model - Wenlan. It focuses on training based on information contributed by weakly supervised correlations of massive Internet images and nearby text. Using the twin-tower mode, the final training is a picture encoder and a text encoder. These two encoders pass the end-to-end matching optimization learning process so that the final representation vector can be mapped to a unified space, rather than The fine grain of the picture and the fine grain of the text are spliced together.
This cross-modal retrieval capability actually not only provides users with more space end-to-end when using web search engines, but also At the same time, it can also support many applications, such as creation, whether it is social media or cultural and creative categories, it can be used to support it.
RICH KNOWLEDGE
Nowadays search engines generally search for web pages, but in the future the units processed by search engines will not only be web pages, but should be based on knowledge. The unit, including the returned results, should also be high-level knowledge, rather than a page-by-page list. Many times users actually want to use search engines to complete some complex information needs, so they hope that search engines will help analyze the results, rather than letting people analyze them one by one.
Based on this idea, we built an analysis engine, which is equivalent to a search engine that can provide in-depth text analysis and help users obtain high-level knowledge efficiently and quickly. Help users read and understand large-scale documents, and extract, mine, and summarize key information and knowledge contained in them. Finally, through an interactive analysis process, users can browse and analyze the high-level knowledge mined. , and then provide users with decision support.
For example, if a user wants to find information related to haze, he can directly enter "haze". The rich knowledge model is different from the results returned by traditional search engines. It may return a timeline to tell the user the distribution of information about smog on the timeline, etc. It will also summarize the sub-topics about smog and the institutions. Which ones, what characters are there. Of course, it can also provide a detailed list of results like a search engine.
This ability to directly provide analysis and interactive analysis can better help users obtain complex information. What is provided to users is no longer a simple list of search results. Of course, this kind of interactive multi-dimensional knowledge analysis is just a display method, and more methods can be used in the future. For example, one of the things we are doing now is from retrieval to generating (reasonable) content.
Go to index
Nowadays search engines widely adopt a staged approach with indexing as the core, crawling back the required content from a large number of Internet web pages and then building an Index, which is an inverted index or Dense vector indexing. After the user's Query comes, a recall is first performed, and then refined sorting is performed based on the recall results.
This model has many disadvantages, because it needs to be divided into stages. If there is a problem in one stage, for example, the desired result is not found in the recall stage, no matter how good it is in the sorting stage, It is also unlikely to return very good results.
In future search engines, this structure may be broken. The new idea is to use a large model to replace the current index schema, and all queries can be satisfied through the model. This no longer requires the use of indexes, but directly feeds back the desired results through this model.
On this basis, you can directly provide a list of results, or directly provide the answers required by the user, and even the answers can be images. The modes are better integrated together. Removing the index and feeding back the results directly through the model means that the model can directly return or directly return the document identifier. The document identifier must be embedded in the model to build a model-centered search.
Summary
Today’s search engines widely use the simple model of keywords as input and document list as output. There are already some problems in meeting people's complex information acquisition needs. The search engine of the future will be conversational, personalized, user-centered, and able to break through stereotypes. At the same time, it can process multi-modal information, process knowledge, and return knowledge. In terms of architecture, in the future, we will definitely break through the existing index-centered model that uses inverted index or dense vector index, and gradually transition to a model-centered model.
Guest introduction
Dou Zhicheng, Renmin University of China Vice President of Hillhouse Institute of Artificial Intelligence, Project Manager of "Intelligent Information Retrieval and Mining" of Beijing Zhiyuan Artificial Intelligence Research Institute. In 2008, he joined Microsoft Research Asia and engaged in Internet search-related work, developing rich experience in information retrieval technology research and development. He started teaching at Renmin University of China in 2014. His main research directions are intelligent information retrieval and natural language processing. He has won the Best Paper Nomination Award at the International Conference on Information Retrieval (SIGIR 2013), the Best Paper Award at the Asian Conference on Information Retrieval (AIRS 2012), and the Best Paper Award at the National Academic Conference on Information Retrieval (CCIR 2018, CCIR 2021). He serves as the chairman of the program committee of SIGIR 2019 (short article), the chairman of the program committee of the Information Retrieval Evaluation Conference NTCIR-16, and the deputy secretary-general of the Big Data Expert Committee of the China Computer Federation. In the past two years, he has mainly focused on personalized and diversified search ranking, interactive and conversational search models, pre-training methods for information retrieval, interpretability of search and recommendation models, personalized product search, etc.
The above is the detailed content of How will we conduct information searches in the future?. For more information, please follow other related articles on the PHP Chinese website!

Hot AI Tools

Undresser.AI Undress
AI-powered app for creating realistic nude photos

AI Clothes Remover
Online AI tool for removing clothes from photos.

Undress AI Tool
Undress images for free

Clothoff.io
AI clothes remover

AI Hentai Generator
Generate AI Hentai for free.

Hot Article

Hot Tools

Notepad++7.3.1
Easy-to-use and free code editor

SublimeText3 Chinese version
Chinese version, very easy to use

Zend Studio 13.0.1
Powerful PHP integrated development environment

Dreamweaver CS6
Visual web development tools

SublimeText3 Mac version
God-level code editing software (SublimeText3)

Hot Topics


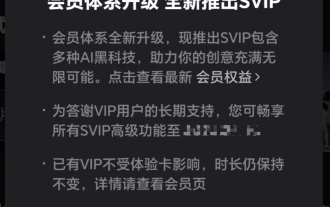
This site reported on June 27 that Jianying is a video editing software developed by FaceMeng Technology, a subsidiary of ByteDance. It relies on the Douyin platform and basically produces short video content for users of the platform. It is compatible with iOS, Android, and Windows. , MacOS and other operating systems. Jianying officially announced the upgrade of its membership system and launched a new SVIP, which includes a variety of AI black technologies, such as intelligent translation, intelligent highlighting, intelligent packaging, digital human synthesis, etc. In terms of price, the monthly fee for clipping SVIP is 79 yuan, the annual fee is 599 yuan (note on this site: equivalent to 49.9 yuan per month), the continuous monthly subscription is 59 yuan per month, and the continuous annual subscription is 499 yuan per year (equivalent to 41.6 yuan per month) . In addition, the cut official also stated that in order to improve the user experience, those who have subscribed to the original VIP
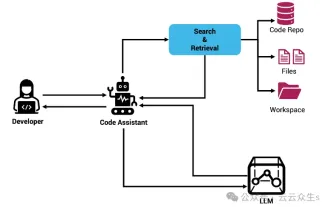
Improve developer productivity, efficiency, and accuracy by incorporating retrieval-enhanced generation and semantic memory into AI coding assistants. Translated from EnhancingAICodingAssistantswithContextUsingRAGandSEM-RAG, author JanakiramMSV. While basic AI programming assistants are naturally helpful, they often fail to provide the most relevant and correct code suggestions because they rely on a general understanding of the software language and the most common patterns of writing software. The code generated by these coding assistants is suitable for solving the problems they are responsible for solving, but often does not conform to the coding standards, conventions and styles of the individual teams. This often results in suggestions that need to be modified or refined in order for the code to be accepted into the application
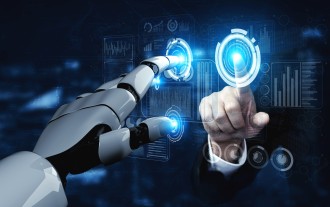
Large Language Models (LLMs) are trained on huge text databases, where they acquire large amounts of real-world knowledge. This knowledge is embedded into their parameters and can then be used when needed. The knowledge of these models is "reified" at the end of training. At the end of pre-training, the model actually stops learning. Align or fine-tune the model to learn how to leverage this knowledge and respond more naturally to user questions. But sometimes model knowledge is not enough, and although the model can access external content through RAG, it is considered beneficial to adapt the model to new domains through fine-tuning. This fine-tuning is performed using input from human annotators or other LLM creations, where the model encounters additional real-world knowledge and integrates it

To learn more about AIGC, please visit: 51CTOAI.x Community https://www.51cto.com/aigc/Translator|Jingyan Reviewer|Chonglou is different from the traditional question bank that can be seen everywhere on the Internet. These questions It requires thinking outside the box. Large Language Models (LLMs) are increasingly important in the fields of data science, generative artificial intelligence (GenAI), and artificial intelligence. These complex algorithms enhance human skills and drive efficiency and innovation in many industries, becoming the key for companies to remain competitive. LLM has a wide range of applications. It can be used in fields such as natural language processing, text generation, speech recognition and recommendation systems. By learning from large amounts of data, LLM is able to generate text
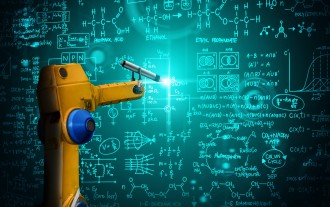
Machine learning is an important branch of artificial intelligence that gives computers the ability to learn from data and improve their capabilities without being explicitly programmed. Machine learning has a wide range of applications in various fields, from image recognition and natural language processing to recommendation systems and fraud detection, and it is changing the way we live. There are many different methods and theories in the field of machine learning, among which the five most influential methods are called the "Five Schools of Machine Learning". The five major schools are the symbolic school, the connectionist school, the evolutionary school, the Bayesian school and the analogy school. 1. Symbolism, also known as symbolism, emphasizes the use of symbols for logical reasoning and expression of knowledge. This school of thought believes that learning is a process of reverse deduction, through existing
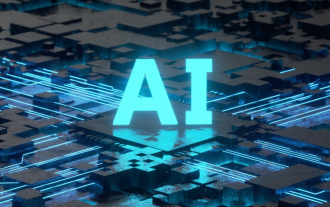
Editor |ScienceAI Question Answering (QA) data set plays a vital role in promoting natural language processing (NLP) research. High-quality QA data sets can not only be used to fine-tune models, but also effectively evaluate the capabilities of large language models (LLM), especially the ability to understand and reason about scientific knowledge. Although there are currently many scientific QA data sets covering medicine, chemistry, biology and other fields, these data sets still have some shortcomings. First, the data form is relatively simple, most of which are multiple-choice questions. They are easy to evaluate, but limit the model's answer selection range and cannot fully test the model's ability to answer scientific questions. In contrast, open-ended Q&A
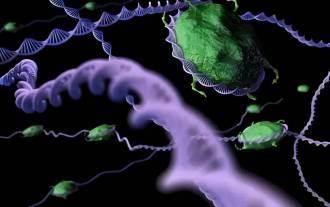
Editor | KX In the field of drug research and development, accurately and effectively predicting the binding affinity of proteins and ligands is crucial for drug screening and optimization. However, current studies do not take into account the important role of molecular surface information in protein-ligand interactions. Based on this, researchers from Xiamen University proposed a novel multi-modal feature extraction (MFE) framework, which for the first time combines information on protein surface, 3D structure and sequence, and uses a cross-attention mechanism to compare different modalities. feature alignment. Experimental results demonstrate that this method achieves state-of-the-art performance in predicting protein-ligand binding affinities. Furthermore, ablation studies demonstrate the effectiveness and necessity of protein surface information and multimodal feature alignment within this framework. Related research begins with "S
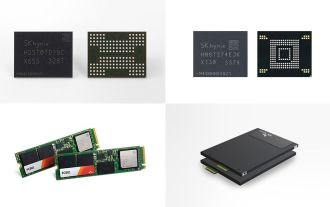
According to news from this site on August 1, SK Hynix released a blog post today (August 1), announcing that it will attend the Global Semiconductor Memory Summit FMS2024 to be held in Santa Clara, California, USA from August 6 to 8, showcasing many new technologies. generation product. Introduction to the Future Memory and Storage Summit (FutureMemoryandStorage), formerly the Flash Memory Summit (FlashMemorySummit) mainly for NAND suppliers, in the context of increasing attention to artificial intelligence technology, this year was renamed the Future Memory and Storage Summit (FutureMemoryandStorage) to invite DRAM and storage vendors and many more players. New product SK hynix launched last year
