


One line of text to achieve 3D face-changing! UC Berkeley proposes 'Chat-NeRF' to complete blockbuster-level rendering in just one sentence
Thanks to the development of neural 3D reconstruction technology, capturing feature representations of real-world 3D scenes has never been easier.
However, there has never been a simple and effective solution for 3D scene editing above this.
Recently, researchers from UC Berkeley proposed a method of editing NeRF scenes using text instructions - Instruct-NeRF2NeRF, based on the previous work InstructPix2Pix.
##Paper address: https://arxiv.org/abs/2303.12789
Using Instruct-NeRF2NeRF, we can edit large-scale real-world scenes with just one sentence, and make it more realistic and targeted than previous work.
For example, if you want him to have a beard, a tuft of beard will appear on his face!
Or just change your head and become Einstein in seconds.
#In addition, since the model can continuously update the data set with new edited images, the reconstruction of the scene will gradually improve.
NeRF InstructPix2Pix = Instruct-NeRF2NeRFSpecifically, humans are given an input image, and written instructions that tell the model what to do, which the model then follows. These instructions are used to edit images.
The implementation steps are as follows:
- Render an image from the scene in the training perspective.
- Use the InstructPix2Pix model to edit this image based on global text instructions.
- Replace the original images in the training dataset with the edited images.
- The NeRF model continues training as usual.
Implementation
Compared with traditional 3D editing, NeRF2NeRF is a new 3D scene editing method. Its biggest highlight is the use of "iterative data set update" technology.
Although editing is performed on a 3D scene, a 2D rather than a 3D diffusion model is used in the paper to extract form and appearance priors because the data used to train the 3D generative model is very limited. .
This 2D diffusion model is the InstructPix2Pix developed not long ago by the research team - a 2D image editing model based on command text. When you input image and text commands, it can output editing image after.
However, this 2D model will cause uneven changes in different angles of the scene. Therefore, "iterative data set update" came into being. This technology alternately modifies NeRF's "input image data". Set" and update the underlying 3D representation.
This means that the text-guided diffusion model (InstructPix2Pix) will generate new image variations according to the instructions and use these new images as input for NeRF model training. Therefore, the reconstructed 3D scene will be based on new text-guided editing.
In the initial iterations, InstructPix2Pix often fails to perform consistent edits across different views, however, during NeRF re-rendering and updating, they will converge to a globally consistent Scenes.
In summary, the NeRF2NeRF method improves the editing efficiency of 3D scenes by iteratively updating image content and integrating these updated contents into the 3D scene, while also maintaining Scene coherence and realism.
It can be said that this work of the UC Berkeley research team is an extended version of the previous InstructPix2Pix. By combining NeRF with InstructPix2Pix and working with "iterative data set update", a Key editing can still play with 3D scenes!
There are still limitations, but the flaws are not hidden
However, since Instruct-NeRF2NeRF is based on the previous InstructPix2Pix, it inherits many limitations of the latter, such as the inability to carry out large-scale space operations.
Additionally, like DreamFusion, Instruct-NeRF2NeRF can only use the diffusion model on one view at a time, so you may encounter similar artifact issues.
The following figure shows two types of failure cases:
(1) Pix2Pix cannot perform editing in 2D, so NeRF2NeRF cannot perform editing in 3D It also failed;
(2) Pix2Pix can complete editing in 2D, but there is a big inconsistency in 3D, so NeRF2NeRF also failed.
Another example is the "panda" below, which not only looks very fierce (the prototype statue is very fierce) , and the fur color is somewhat weird, and the eyes are obviously "out of shape" when moving in the screen.
Since ChatGPT, Diffusion, and NeRFs have been pulled into the spotlight, this article can be said to give full play to the advantages of the three, from "AI Sentence "Word drawing" has advanced to "AI one-sentence editing of 3D scenes".
Although the method has some limitations, it still has its flaws and provides a simple and feasible solution for 3D feature editing, which is expected to become a milestone in the development of NeRF.
Editing 3D scenes in one sentence
Finally, let’s take a look at the effects released by the author.
It is not difficult to see that this one-click PS 3D scene editing artifact is more in line with expectations in terms of command understanding ability and image realism. In the future, it may become a popular choice among academics and The "new favorite" among netizens has created Chat-NeRFs after ChatGPT.
Even if you change the environmental background, seasonal characteristics, and weather of the image at will, The new images given are also completely consistent with the logic of reality.
Original picture:
##Autumn:
Snow day:
Reference: https://www .php.cn/link/ebeb300882677f350ea818c8f333f5b9
The above is the detailed content of One line of text to achieve 3D face-changing! UC Berkeley proposes 'Chat-NeRF' to complete blockbuster-level rendering in just one sentence. For more information, please follow other related articles on the PHP Chinese website!

Hot AI Tools

Undresser.AI Undress
AI-powered app for creating realistic nude photos

AI Clothes Remover
Online AI tool for removing clothes from photos.

Undress AI Tool
Undress images for free

Clothoff.io
AI clothes remover

AI Hentai Generator
Generate AI Hentai for free.

Hot Article

Hot Tools

Notepad++7.3.1
Easy-to-use and free code editor

SublimeText3 Chinese version
Chinese version, very easy to use

Zend Studio 13.0.1
Powerful PHP integrated development environment

Dreamweaver CS6
Visual web development tools

SublimeText3 Mac version
God-level code editing software (SublimeText3)

Hot Topics
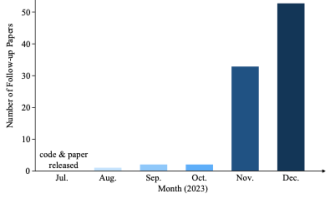
Written above & the author’s personal understanding Three-dimensional Gaussiansplatting (3DGS) is a transformative technology that has emerged in the fields of explicit radiation fields and computer graphics in recent years. This innovative method is characterized by the use of millions of 3D Gaussians, which is very different from the neural radiation field (NeRF) method, which mainly uses an implicit coordinate-based model to map spatial coordinates to pixel values. With its explicit scene representation and differentiable rendering algorithms, 3DGS not only guarantees real-time rendering capabilities, but also introduces an unprecedented level of control and scene editing. This positions 3DGS as a potential game-changer for next-generation 3D reconstruction and representation. To this end, we provide a systematic overview of the latest developments and concerns in the field of 3DGS for the first time.
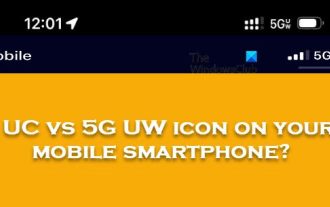
T-Mobile users have started noticing that the network icon on their phone screens sometimes reads 5GUC, while other carriers read 5GUW. This is not a typo, but represents a different type of 5G network. In fact, operators are constantly expanding their 5G network coverage. In this topic, we will take a look at the meaning of the 5GUC and 5GUW icons displayed on T-Mobile smartphones. The two logos represent different 5G technologies, each with its own unique characteristics and advantages. By understanding what these signs mean, users can better understand the type of 5G network they are connected to so they can choose the network service that best suits their needs. 5GUCVS5GUW icon in T
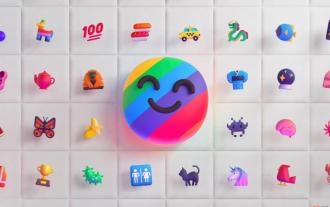
You must remember, especially if you are a Teams user, that Microsoft added a new batch of 3DFluent emojis to its work-focused video conferencing app. After Microsoft announced 3D emojis for Teams and Windows last year, the process has actually seen more than 1,800 existing emojis updated for the platform. This big idea and the launch of the 3DFluent emoji update for Teams was first promoted via an official blog post. Latest Teams update brings FluentEmojis to the app Microsoft says the updated 1,800 emojis will be available to us every day
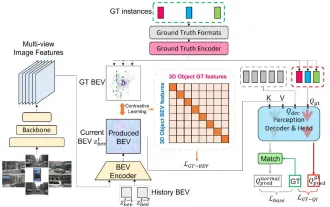
Written above & the author’s personal understanding: At present, in the entire autonomous driving system, the perception module plays a vital role. The autonomous vehicle driving on the road can only obtain accurate perception results through the perception module. The downstream regulation and control module in the autonomous driving system makes timely and correct judgments and behavioral decisions. Currently, cars with autonomous driving functions are usually equipped with a variety of data information sensors including surround-view camera sensors, lidar sensors, and millimeter-wave radar sensors to collect information in different modalities to achieve accurate perception tasks. The BEV perception algorithm based on pure vision is favored by the industry because of its low hardware cost and easy deployment, and its output results can be easily applied to various downstream tasks.
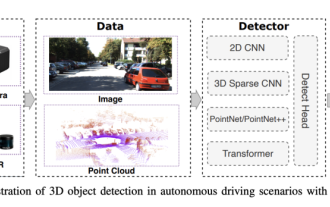
0.Written in front&& Personal understanding that autonomous driving systems rely on advanced perception, decision-making and control technologies, by using various sensors (such as cameras, lidar, radar, etc.) to perceive the surrounding environment, and using algorithms and models for real-time analysis and decision-making. This enables vehicles to recognize road signs, detect and track other vehicles, predict pedestrian behavior, etc., thereby safely operating and adapting to complex traffic environments. This technology is currently attracting widespread attention and is considered an important development area in the future of transportation. one. But what makes autonomous driving difficult is figuring out how to make the car understand what's going on around it. This requires that the three-dimensional object detection algorithm in the autonomous driving system can accurately perceive and describe objects in the surrounding environment, including their locations,
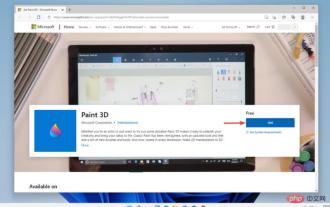
When the gossip started spreading that the new Windows 11 was in development, every Microsoft user was curious about how the new operating system would look like and what it would bring. After speculation, Windows 11 is here. The operating system comes with new design and functional changes. In addition to some additions, it comes with feature deprecations and removals. One of the features that doesn't exist in Windows 11 is Paint3D. While it still offers classic Paint, which is good for drawers, doodlers, and doodlers, it abandons Paint3D, which offers extra features ideal for 3D creators. If you are looking for some extra features, we recommend Autodesk Maya as the best 3D design software. like
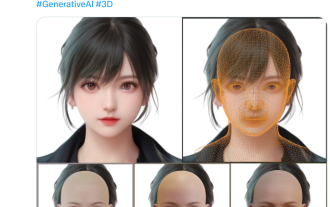
ChatGPT has injected a dose of chicken blood into the AI industry, and everything that was once unthinkable has become basic practice today. Text-to-3D, which continues to advance, is regarded as the next hotspot in the AIGC field after Diffusion (images) and GPT (text), and has received unprecedented attention. No, a product called ChatAvatar has been put into low-key public beta, quickly garnering over 700,000 views and attention, and was featured on Spacesoftheweek. △ChatAvatar will also support Imageto3D technology that generates 3D stylized characters from AI-generated single-perspective/multi-perspective original paintings. The 3D model generated by the current beta version has received widespread attention.
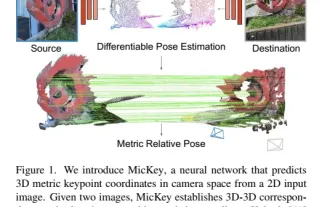
Project link written in front: https://nianticlabs.github.io/mickey/ Given two pictures, the camera pose between them can be estimated by establishing the correspondence between the pictures. Typically, these correspondences are 2D to 2D, and our estimated poses are scale-indeterminate. Some applications, such as instant augmented reality anytime, anywhere, require pose estimation of scale metrics, so they rely on external depth estimators to recover scale. This paper proposes MicKey, a keypoint matching process capable of predicting metric correspondences in 3D camera space. By learning 3D coordinate matching across images, we are able to infer metric relative
