


Key technologies for safety assurance of expected functions of smart cars
Anticipated functional safety issues arising from performance limitations, insufficient specifications or reasonably foreseeable misuse have emerged in an endless stream, seriously hindering the rapid development of smart cars. This review focuses on the key technologies for ensuring the expected functional safety of smart cars. It systematically summarizes the three stages of system development, function improvement and operation, and finally makes an outlook from the three aspects of basic theory, risk protection and update mechanism. This article can provide an important reference for research on expected functional safety of smart cars.
Preface
According to statistics from the National Transportation Safety Administration (NHTSA), about 94% of traffic accidents are caused by human factors. Cars replace human drivers with machines, which is of great significance in improving driving safety. However, existing technologies cannot yet fully realize their safety potential. In addition, when new technologies are introduced to eliminate original problems, new safety problems also arise, such as functional safety, information safety and safety of the intended functionality. SOTIF) problem. Especially as smart car systems become more complex and intelligent, and their operating environments become more open and challenging, the SOTIF problem caused by insufficient functions is gradually exposed and has become a key problem restricting the safety of smart cars. In addition, the autonomous driving/assisted driving accidents that have occurred in recent years due to insufficient functions such as perception and decision-making also reflect the seriousness of the SOTIF problem. Figure 1 is an analysis of the causes of the world’s first road test unmanned vehicle accident that killed a pedestrian in 2018. Insufficient sensing and prediction functions were the main causes of the accident. Therefore, promoting research on SOTIF assurance technology has become a top priority.
## Figure 1 Analysis of the causes of Uber road test unmanned vehicle accidents
Anticipated functional safety aims to avoid unreasonable risks arising from hazards due to insufficient functionality of the intended function or its implementation. Its basic concept is proposed by ISO 21448 and Definition, since ISO started the development of this standard in February 2016, draft versions of PAS, CD, DIS and FDIS have been formed. As an extension of ISO 26262, ISO 21448 addresses the problem of insufficient functionality without random hardware failures and system failures.
SOTIF research involves many aspects such as system function design improvement, analysis and evaluation, verification and certification, and new requirements are constantly put forward with the development of technology and the introduction of new technologies. Therefore, ISO 21448 cannot cover all relevant aspects in detail. In recent years, many other international standards have been proposed and SOTIF has been an important research object, as shown in Figure 2.
#Figure 2 SOTIF related standards
In terms of safety assessment of automated products, UL 4600 aims to supplement functional safety and SOTIF standards, proposing a safety goal-oriented approach that focuses on "how to evaluate" fully autonomous driving safety Situation; For the safety design, verification and validation of high-level autonomous driving systems, ISO/TR 4804 determines the SOTIF functional design process in compliance with ISO/PAS 21448, and ISO/AWI TS 5083 is to be further developed; for scenario-based safety assessment, ISO 34502 proposes a set of scenario generation and evaluation processes, and specifically considers the typical trigger conditions of SOTIF in the process of establishing the scenario library; in view of the problems after the introduction of new technologies such as artificial intelligence (AI), the ISO/ AWI PAS 8800 aims to provide specifications that address the full life cycle of AI-related system development and deployment issues to make up for the lack of consideration of AI issues in ISO 21448. Along with the SOTIF standardization process, domestic and foreign governments, enterprises and research institutions have conducted many explorations in SOTIF practical solutions in recent years: In terms of product development, many companies such as BMW and Baidu have tried to introduce SOTIF into the entire life of their products. Cycle safety development process; in terms of product safety analysis and evaluation, companies such as Continental and ANSYS have tried to introduce safety analysis tools. The EU ENSEMBLE project and NHTSA have conducted SOTIF analysis and evaluation practices and provided results reports; in terms of safety verification and confirmation, the EU PEGASUS Its extension projects VVM, SetLevel, Japan's SAKURA project and the China Intelligent Connected Vehicle Alliance's expected functional safety working group have been combined with SOTIF in practice; in terms of functional improvements, many companies have proposed their own plans, such as the European Union DENSE, etc. The project studies the specific functional deficiencies of components such as sensors. The above standards and practical activities provide framework guidance for smart car SOTIF assurance (see Figure 3). In the actual research and development process, specific assurance technologies must be used to effectively to solve specific problems faced at each stage. However, this field has not yet formed a complete technical research system: on the one hand, although the current literature directly on the subject of SOTIF is showing a growing trend, the total amount is still relatively small, and the content mainly involves the elaboration of concepts and meanings, security analysis, test verification and In terms of systems engineering and other aspects, there is a lack of systematic research and sorting out of key SOTIF assurance technologies; on the other hand, although high-level research results in many related fields have important inspiration and reference significance for solving the problem of insufficient functions, they have not yet been clearly included in SOTIF Protection technology research scope. #Figure 3 Basic activity process of SOTIF assurance Therefore, based on a large number of domestic and foreign research reports and literature, this article systematically analyzes and sorts out the key technologies of SOTIF assurance, and puts forward prospects based on the existing research shortcomings. Clear problem definition and risk source analysis are the prerequisites for ensuring SOTIF. Analyzing from the perspective of the system itself, the SOTIF problem mainly stems from two aspects: (1) Insufficient specification of expected functions at the vehicle level, limitations such as scene openness, system complexity, and incomplete expert experience may lead to poor vehicle behavior design Problems arise in the specification process, making it difficult to achieve ideal safety goals; (2) Insufficiency in the realization of expected functions. Even if the specification of the expected functions of the vehicle layer is complete enough, due to the performance limitations and insufficient specifications of system components, functions such as perception, decision-making, and control cannot be achieved The implementation may not be as expected. For example, sensors and actuators have performance limitations such as upper limits of perception and execution capabilities or are susceptible to interference from external environmental factors; perception and decision-making algorithms may have limitations in robustness, generalization, interpretability, logical completeness, rule coverage, etc. question. In addition, the emergence and evolution of SOTIF hazards depend on specific scenarios. First, the above-mentioned insufficient specifications or performance limitations are triggered by specific conditions in the scene, resulting in harmful behaviors; in addition, the above-mentioned harmful behaviors eventually evolve into harm because the current scene contains relevant risk sources and the scene controllability is low. Therefore, during the SOTIF assurance process, it is necessary to integrate the system's own limitations and operating scenario risks to establish a security assurance system. According to whether the scenario is known and whether it will cause SOTIF harm, it is divided into four types of scenarios: known safe, known unsafe, unknown unsafe and unknown safe. SOTIF guarantee goals In order to minimize the corresponding areas of the two types of unsafe scenarios through a series of activities and related technologies, the core is the discovery and processing of unknown unsafe scenarios. As shown in Figure 4, the realization of SOTIF assurance goals can be decomposed into two aspects: transforming the unknown into the known, and transforming the unsafe into safe. First of all, activities such as SOTIF analysis and evaluation, verification and confirmation, as well as key data collection, recording and feedback in the operation phase help to fully explore unknown scenarios; in addition, the development phase directly targets the improvement of insufficient functions, unknown risk monitoring, protection and based on the operation phase. Improvement of system functions for collecting data is a necessary activity to transform unsafe scenarios into safe scenarios; in addition, verification and confirmation, residual risk assessment and safety demonstration are important activities to ensure that residual risks are low enough, thus providing a basis for SOTIF release. The following will sort out the key SOTIF assurance technologies corresponding to each activity from the development stage and operation stage, and conduct a detailed discussion on the function improvement technology of smart car systems. ##Figure 4 SOTIF assurance goals and implementation process SOTIF assurance activities during the system development phase mainly include SOTIF analysis and evaluation, verification and confirmation, function improvement and release, etc. This document Each section will focus on introducing the key technologies of each link. The use of effective security analysis technology can improve the efficiency, comprehensiveness and analysis of SOTIF hazards, potential functional deficiencies and triggering conditions. scientific. Traditional safety analysis techniques, such as fault tree analysis, failure mode and effect analysis, hazard and operability analysis, etc., have been applied in SOTIF analysis and evaluation; new technologies represented by smart cars have brought about changes in the nature of accidents and new New safety challenges such as type hazards, reduced tolerance of single accidents, increased system complexity, and complicated human-computer interaction require more effective safety analysis techniques, systems-theoretic process analysis (STPA) (see Figure 5) has the potential to analyze complex systems, including four steps of defining the purpose of analysis, building a control structure, identifying unsafe control behaviors, and identifying causal scenarios. It has been used for SOTIF analysis of perception, decision-making, and fully autonomous driving systems. However, the availability of a single technology is limited and their respective advantages can be combined to develop more effective SOTIF analysis techniques. #Figure 5 STPA technology implementation process A risk assessment should be conducted for the identified SOTIF hazards. Technologies such as STPA themselves do not have the function of risk quantification, so they need to be expanded accordingly. Hazard analysis and risk assessment (HARA) and automotive safety integrity level (ASIL) in the field of functional safety have been Several studies have improved and used it in SOTIF risk assessment. Bayesian probability models, as a statistical method, have also been used to quantify SOTIF-related risks and their boundaries. However, due to the increasing complexity of scenarios and statistical difficulties, the dependence of trigger conditions on scenarios, and the uncertainty of AI algorithms, existing research has not been able to clarify and unify SOTIF risk definitions and their quantification methods. Therefore, there is an urgent need to explore and propose new methods. Effective SOTIF quantitative analysis indicators and techniques. In addition, in order to avoid the unacceptable complexity of smart car HARA, techniques such as task decomposition, equivalence class and impact analysis, and model reconstruction can be combined to manage its complexity. In response to unreasonable risks caused by insufficient functions, function improvements should be made to reduce the unsafe area. There are many functional improvement technologies at this stage, which can be mainly divided into three technical routes: ① performance improvement, such as increasing the performance upper limit of a specific sensor or sensing model itself; ② risk monitoring and protection, that is, by controlling trigger conditions (including reasonably foreseeable Misoperation), insufficient function status, etc. to monitor SOTIF risks, so as to adopt targeted protection technologies, such as elimination of risk sources, functional restrictions or permission transfer, etc. In addition, it can also directly monitor the operational design domain (operational design domain, etc.) The clarification, monitoring and limitation of ODD) provide a reference for risk protection; ③ Functional redundancy, such as designing redundant functional modules to improve overall performance. Section 3 will systematically sort out the corresponding function improvement technologies for each module and vehicle layer of the smart car. Verification and confirmation is an important activity to further discover unsafe scenarios and prove that SOTIF is fully protected. SOTIF verification aims to provide objective evidence to prove compliance with specified requirements, including sensors, sensing algorithms, decision-making algorithms, actuators and integrated systems, etc. Verification indicators such as accuracy, reliability and anti-interference, etc. SOTIF validation aims to use reasonable validation objectives and methods to assess whether residual risks are acceptable under known and unknown unsafe scenarios. The SOTIF confirmation goal is used to quantify the conditions for meeting the acceptance criteria, which can analyze risk acceptance principles such as risk tolerance, positive risk balance, lowest reasonably feasible, and lowest endogenous based on accident statistics, human driver performance, etc. Mortality rate etc. SOTIF verification confirmation must comprehensively consider the effectiveness, feasibility and cost of the technology used. For example, the cost of verification, simulation and software and hardware in the loop based on analysis and comparison is relatively low. However, the validity and scope of application of the evidence provided are limited; open road testing can reflect the most realistic performance of the vehicle in the environment, which is conducive to breaking through the limitations of empirical knowledge and models, and mining rare unknown unsafe scenarios. However, it is difficult to use such methods alone. The cost is unacceptable. In recent years, scenario-based testing (see Figure 6) has been widely researched and practiced. On the one hand, this method can reasonably allocate test resources by combining different platforms such as simulation, software and hardware-in-the-loop, and test sites, and further reduce testing costs by combining test scenario coverage assessment, importance sampling, hazardous behavior identification and other technologies; on the other hand, This method takes scenarios as the core and can be used for SOTIF verification in scenarios containing potential trigger conditions. It can also assist SOTIF confirmation through sampling tests based on real scenario distribution or full exploration of unknown scenarios. ##Figure 6 Scenario-based testing method and process The generation of specific scenarios or use cases is a prerequisite for verification and confirmation. According to different sources of information, it is mainly divided into knowledge-driven and data-driven. The former can refer to expert knowledge and standards. and related experience, etc. Typical methods include ontology, which generally relies on natural driving or accident data for extraction. Depending on the generation goals, it mainly includes random scene generation and key scene generation. Key scenes can be derived from the mapping and combination of identified potential trigger conditions, or can be automatically generated by defining indicators such as the danger level of the scene. Adversarial sample generation is an effective key scenario generation method. It combines gradient and other information to automatically generate safety-critical scenarios that are more likely to trigger insufficient system functions, thereby improving testing efficiency; in the scenario generation process, similarity with the real world is a guarantee It is an important prerequisite for test effectiveness, and acceptable disturbance generation is an important technology to achieve the above goals. In addition, appropriate functional decomposition is of great significance to overcome parameter space explosion and reduce the amount of testing. According to different test objects, differentiated considerations should be made when generating scenarios for different functional modules. For example, for sensors and sensing modules, you can choose to include rain Scenes of severe weather such as snow and fog or specific target detection objects; for the decision-making module, it can focus on the selection of scenarios such as traffic interference; for controllers and actuators, scenarios including extreme working conditions, harsh roads and environmental conditions need to be Important considerations. Selecting specific scenarios from the generated scenarios or scenario libraries is a key step in determining test representativeness, coverage and cost. The parameter space is complex and continuous, so a sampling method can be used to first The difference in empirical information is divided into sampling based on parameter range and sampling based on parameter distribution. Typical technologies of the former include combinatorial testing, interactive experimental design, randomization technology, etc.; typical technologies of the latter include Monte Carlo sampling, etc. Accelerated testing is an important way to improve testing costs. Typical techniques include extreme value theory, importance sampling, and Markov chain Monte Carlo. In addition, some research focuses on scenario selection based on falsification, such as key scenario screening by considering characteristics such as accident data or scenario criticality and complexity, or using simulation for adaptive stress testing, alternative modeling and stochastic optimization, and adaptive search. wait. The test platform includes virtual simulation, software and hardware-in-the-loop, vehicle-in-the-loop, and test sites. Its test authenticity increases gradually, but test costs, safety risks, and scalability gradually increase. To reduce the risk, in order to make full use of limited resources, simulation and in-the-loop testing technologies should be given priority while meeting the testing requirements. In addition, the applicability of simulation and in-the-loop testing techniques can be further improved by developing high-fidelity sensor models (e.g. using phenomenological models). Evaluation indicators are the basis for judging whether a system or component meets specified requirements or the residual risk is low enough. Traditional security indicators can include subjective/objective, micro/macro, short-term/long-term and other types. , but it is mainly used to evaluate the behavior of the entire vehicle and is not suitable for specific functional components; at present, the evaluation of perception and prediction models also has problems such as different standards, mainly focusing on accuracy evaluation and insufficient consideration of safety. Therefore, SOTIF indicators suitable for smart car function evaluation need to be proposed. In addition, formal verification technology uses mathematical modeling methods to ensure system correctness and rigorous verification results, so it is of great significance to safety-critical systems such as smart cars. In terms of vehicle behavior verification, technologies such as theorem proving and reachability analysis have received a lot of attention; in terms of system integration, formal verification can be used to standardize the correctness of the integration of different components (such as controllers); in addition, formal methods are used in The AI field represented by machine learning has been extensively studied and can be further used to verify related functional modules such as perception and prediction. However, the implementation cost of this technology is high, and its scalability to complex systems, open scenarios, and black-box models is limited, so it still needs to be further explored and improved. In summary, there are currently a variety of technologies that can be used for SOTIF verification and confirmation, and the results can be further improved by combining the advantages of different technologies. However, SOTIF verification and confirmation still faces severe challenges due to complex and changeable scenarios and long-tail effects, complex and diverse smart car systems and fast update iterations, as well as the lack of SOTIF evaluation specifications. At the end of the development phase, it must be demonstrated whether the system complies with the SOTIF release criteria. Schwalb et al. proposed a probabilistic framework to gradually quantify SOTIF residual risk. In addition, after the above-mentioned analysis and evaluation, design improvement, verification and confirmation activities, a complete safety document can be formed, and then technologies such as target structure representation and expanded evidence network can be used to conduct safety demonstrations. For example, Misra proposed a state machine to explore expected functions. Conditions that may cause harm, and the corresponding safety statements are asserted. On this basis, the SOTIF argumentation structure is constructed based on the target structure representation. In addition to the targeted assurance technologies for the above-mentioned stages of activities, the optimization of the system development process is also an important direction of SOTIF assurance. For example, the use of agile system engineering can improve system development efficiency, economy and Traceability. In addition, some scholars have tried to integrate formal methods, rule manuals, etc. into the SOTIF system development process, and have initially achieved optimization effects such as accelerating development, improving traceability and evaluability. However, these methods themselves still have problems in complexity, scalability, and applicability. In addition, their combination with SOTIF is still in the exploratory stage, and has limited guiding significance for the actual development process. The realization of smart car functions depends on each sub-module, as shown in Figure 7. Under the influence of triggering conditions such as reasonably foreseeable misuse, insufficient functions such as perception, positioning, decision-making, and control may cause SOTIF harm, and targeted improvements can be made according to the characteristics of each module. This section will summarize respectively from four aspects: perception positioning, decision-making control, reasonably foreseeable misuse processing and vehicle layer function improvement. Figure 7 SOTIF issues at each level of smart cars The realization of perception function mainly relies on sensors and perception models, so its function improvements are mainly aimed at the limitations of sensor performance and the insufficient functions of perception models. conduct. a. Improvement of sensor and perception model performance ##Improve detection range, accuracy and resistance through sensor optimization technology Basic performance such as interference capability. For example, to address the problem that Lidar is susceptible to interference from rain, fog, and dust, there are multiple echo technology and surface laser technology. In addition, the performance improvement technology for the perception model is closely related to the perception algorithm used. At this stage, the perception function of smart cars generally uses machine learning algorithms. According to its working principle, the performance improvement of the perception model can be mainly divided into the following aspects. (1) Training data improvement. First, the richness of training data can be improved by using large-scale low-cost data collection solutions combined with automatic/semi-automatic annotation methods to reduce costs, thereby increasing the amount of training data. In addition, data collection technology can be improved to improve data quality, and data cleaning, filtering, and correction technologies can be combined to reduce training data problems caused by collection or labeling errors. In addition, the training effect can be improved through reasonable distribution of training data distribution. (2) Training model improvement. The design of the model architecture directly affects the perceptual performance. For example, due to the natural advantages of convolutional neural networks for image information processing, the performance of the network added with this design is generally better than that of a simple multi-layer perceptron network. Optimizing the design of perceptual models is currently the main research direction in fields such as computer vision, so perceptual performance has also been rapidly improved. In addition, optimizing the model design can also improve its detection effect on unknown objects, thereby reducing residual risks. (3) Training process improvement. To address the problem of insufficient training data or potentially unknown scenarios, technologies such as data enhancement, transfer learning, and active learning can be used to improve the utilization efficiency of limited data or labels. Among them, data enhancement for perception algorithms, in addition to traditional methods such as image flipping and cropping, , rendering of rain, snow and fog weather conditions is also a way to improve perceived performance in severe weather. To address the problem of potential insufficient functionality, techniques such as adversarial training can help reduce model defects and improve its robustness based on limited data. In addition, improving the loss or reward function and rationally using techniques such as normalization and regularization can help further improve model performance. b. Perceived SOTIF risk monitoring and protection Divide perceived SOTIF risk sources into external trigger conditions and internal Insufficient functions can be used as a reference for risk monitoring. Among them, adverse weather conditions such as rain, snow, fog, and hail are important triggering conditions for sensing SOTIF problems. Some studies have established their influence relationship through experimental analysis, providing a basis for monitoring external triggering conditions. Monitoring of adverse weather conditions can use specific environmental models or weather sensors. For example, vehicle rain sensors include capacitive, optical, piezoelectric, resistive, CCD imaging and other types; in addition, combined with statistics or deep learning Using other methods, the data output by cameras themselves can also be directly used to monitor severe weather conditions or the interference caused by them. In addition, some studies focus on direct monitoring of perceptual underperformance performance, such as by modifying the model, adjusting the training process, and introducing other information to achieve online estimation of perceptual performance. Interference elimination can be performed on sensor data affected by environmental conditions. First, internal tuning of sensor parameters can be used to improve its data quality in severe weather. In addition, interference can be eliminated by adding additional devices, such as cleaning dirt on the sensor with liquid or wipers, and a self-heating device can be added to prevent adverse effects on the camera caused by rain, snow, ice or frost. In addition, preprocessing techniques such as data denoising can also be used to remove environmental interference. For example, typical algorithms for image defogging include image enhancement, image restoration based on atmospheric degradation models, and deep learning-based methods; other research focuses on image dehazing. Rain technology is mainly divided into two categories: raindrop (adhered to the lens) removal and rainfall (distributed in the air) removal; for Lidar, some commercial products already have automatic image correction functions that can filter raindrops through pixel-oriented evaluation and snowflakes. In addition, you can also skip the interference elimination step and directly improve the perceptual model's ability to process data containing interference. For example, Huang et al. introduced a new type of dual subnet network - DSNet to solve the problem of target detection in foggy images. While maintaining high speed, the detection performance is better than many advanced target detectors and "defogging detection" combined models. c. Perception function redundancy In view of the performance limitations of a single sensor and its perception model, multi-sensor fusion It is an important improvement technology. First, similar sensor fusion can increase the sensing range through the rational layout of multiple sensors, such as arranging multiple cameras around the vehicle to obtain a 360° perception perspective; in addition, multi-type sensor fusion will help overcome the inherent limitations of a single type of sensor. Performance limitations increase the diversity and accuracy of environmental information acquisition, such as using Lidar's advantage in accurate ranging to make up for the lack of camera functionality, or combining redundant information analysis to determine sensor abnormalities. According to the characteristics of fused sensors, it can be divided into fusion based on different combinations of cameras, Lidar and Radar; according to the level of fusion information, it can be divided into data-level, feature-level and target-level fusion; common fusion methods such as adaptive weighted average method, clustering Class algorithms, Bayesian inference, etc. The current research considers the impact of triggering conditions such as severe weather, and has conducted many explorations on optimal fusion architecture, model design, training strategies, multi-modal data sets, etc., and has achieved some significant results. In addition, in complex urban traffic scenarios, by introducing roadside and city sensing information to achieve an integrated collaborative sensing solution, it is also an important research direction to solve the problem of insufficient bicycle sensing functions. d. Improvement of positioning function The implementation of positioning function mainly includes absolute positioning and positioning based on global navigation satellite systems, etc. Relative positioning based on simultaneous localization and mapping (SLAM), etc. Typical SOTIF problems of the former include multipath phenomena caused by building reflections, positioning confusion or positioning signal loss caused by obstructions such as traffic facilities or mountain canyons. Methods such as GPS altitude or air pressure absolute value comparison can be used to deal with positioning signal confusion on elevated sections. Problem; the latter mainly includes SLAM positioning based on cameras or Lidar, etc. Therefore, the SOTIF problems it faces are similar to perception, such as poor weather leading to reduced positioning accuracy, etc., which can be improved through technologies such as multi-sensor fusion and algorithm optimization. a. Decision-making method classification and performance improvement Currently Mainstream decision-making methods include two categories: rule-based decision-making and learning-based decision-making. The advantages of the former are strong interpretability, easy introduction of expert experience, and strong reliability. However, it is prone to limitations such as insufficient specifications, insufficient cognitive reasoning capabilities in dynamic and complex scenarios, insufficient generalization, and insufficient algorithm scalability. In response to the above problems, first of all, the decision-making logic can be continuously optimized through methods such as experience accumulation and brainstorming. System analysis techniques such as STPA also have certain guiding significance for improving the completeness of decision-making rule design. In addition, the introduction of new modeling theory and information and techniques such as scenario templates can improve the generalizability of decision-making methods to complex and unknown scenarios. In addition, the introduction of a separate prediction module can improve the decision-making ability to recognize the scenario, thus making up for the shortcomings of the original model. In recent years, more and more research has focused on learning-based decision-making methods, such as imitation learning and reinforcement learning. The improvement ideas for this type of method are similar to the performance improvement of the perception model mentioned above, that is, the decision-making performance can be improved by improving the training data, model and training process. b. Decision-making SOTIF risk monitoring and protection The decision-making function module formulates corresponding strategies based on the obtained environmental information. Assuming that the information obtained by the sensing positioning module is accurate enough, the decision-making SOTIF risk mainly comes from the trigger conditions in the operating environment (such as the impact of traffic disturbance on the decision-making algorithm). Challenges) and security issues caused by insufficient functions of the decision-making module itself correspond to the two main factors considered in its risk monitoring and protection. Targeting the triggering conditions in the environment, such as specific road types, constraints can be achieved through OOD, etc., combined with analysis, evaluation and verification results, to gradually clarify the ODD applicable to the decision model, so as to As a reference for monitoring environmental conditions, it uses technologies such as maps, positioning, and specific scene recognition to determine current risks in real time. Aiming at the uncertain movements of traffic participants in the environment, safer decision-making results can be obtained by designing corresponding risk quantification models and risk-sensitive safety decision-making methods; in addition, abnormal behavior detection technology can be used to detect unexpected traffic participants in the environment. Identification of behavior. In view of the potential functional deficiencies of the decision-making module itself, formal verification technology has been extensively studied in the field of decision-making security verification. The basic idea is to verify whether the current decision-making results will lead to accidents, and the rationality of this assumption is also an important factor affecting the effectiveness of safety verification. In addition, the decision-making module is divided into two key sub-modules: prediction and behavior selection, and the quantification of the insufficient prediction function can be used for risk monitoring and protection, as shown in Figure 8. Safe decision-making is enabled by quantifying and propagating uncertainty in predictive models. Figure 8 Safety decision-making considering forecast uncertainty In addition, for scenarios where low-level autonomous driving decisions are difficult to handle, risks can be mitigated by limiting functions or requesting the driver to take over. c. Decision-making function redundancy In view of the limitations of the single-class decision-making model, hybrid decision-making (see figure 9) Complementary advantages can be used to further improve functions. For example, rule-based decision-making is difficult to model high-dimensional uncertainty environments, but its interpretability and reliability can make up for learning-based decision-making. Taking the self-learning hybrid decision-making that integrates rules as an example, it includes adjusting the reward function through knowledge or rules, adjusting the exploration process, adjusting the output action, or adjusting the strategy training iteration process, etc., which can improve the reliability of the decision-making results. In addition, technological developments such as vehicle-road cloud collaboration and cloud control systems provide strong support for safety decision-making. The introduction of traffic status monitoring information, macro decision-making control guidance, computing power support and other assistance provided by the cloud and roadside can alleviate the problems of on-board decision-making systems. Insufficient functionality issues. #Figure 9 General framework of hybrid decision-making d. Improvement of control function The SOTIF problem of control function mainly includes two aspects: (1) Control The limitations of the dynamic modeling layer lead to insufficient representation of vehicle dynamics characteristics, and the controller itself also has performance limitations such as real-time performance; (2) The actuator has execution accuracy, maximum steering or braking capability boundaries, and real-time response capabilities and other limitations, and may be affected by external interference such as road conditions, machinery, strong winds, etc. Therefore, its function improvement can mainly focus on the above two aspects, such as improving the performance of actuator accuracy, response time, etc., monitoring high-risk working conditions for protection, adding new controllers or actuators to achieve redundancy, etc.; in the algorithm level, robust fault-tolerant control, etc. are typical technologies to improve control models. Full identification of reasonably foreseeable misuse in the analysis and evaluation stage is an important prerequisite for dealing with such risks. STPA and other technologies can be used to assist analysis. . There are many ways to deal with potential misuse: First, optimizing user manuals and training can reduce misuse by drivers and passengers due to unclear rules or insufficient knowledge. During driving, early warning can be obtained by monitoring the status of drivers and passengers, such as posture status, extreme abnormal status, seat belt status, etc. Typical monitoring information acquisition methods include driver monitoring cameras, seat positions, steering wheel sensors, etc. , Abbood et al. proposed a fatigue detection and prediction model, which uses sensor sensing information such as pupil responses and EEG signals and customized information such as driver profiles for behavioral prediction and intervention. When potential risks are detected and intervention is carried out, warnings or behavioral suggestions can be provided through visual, auditory, tactile and other interactive forms; at the same time, interactive content should be reasonably designed. Koo et al. studied how the information content transmitted by semi-autonomous driving affects driver attitude and safety. , proposing that the quantity and type of information provided must be reasonably regulated. In addition, in view of unavoidable potential misuse, safety can be improved by designing functional operation methods that are difficult to implement (such as seats, button positions or activation actions), and limiting the power of drivers and passengers in specific scenarios, such as in high-speed scenarios. Disables activation of the automatic urban parking function. Smart cars integrate complex interactions of multiple modules, and the improvement of a single functional module is not enough to fully guarantee SOTIF: On the one hand, each module Corresponding SOTIF problems are difficult to completely eliminate, and residual risks must be minimized by optimizing the vehicle system design. On the other hand, even if each functional module can achieve the expected function, insufficient specifications in the vehicle design may still lead to harmful behaviors. Therefore, the problem of insufficient functions of each module and the triggering conditions it faces should be comprehensively considered from the vehicle level to formulate system solutions. In the design of the vehicle system, SOTIF risk propagation between different functional modules should be fully considered. In recent years, more and more studies have focused on the systematicness and complementarity between the upstream and downstream functions of smart cars. The assumption about the perfect performance of the upstream sensing and positioning module in the above decision-making SOTIF risk is actually difficult to establish. In view of the problems caused by the insufficient sensing and positioning function, This can be compensated for by decision design. For example, by considering insufficient perception caused by sensor input noise and occlusion in the decision-making module, as well as information such as category uncertainty and position uncertainty in the perception results, the impact of insufficient perception function on vehicle safety can be alleviated. In addition, risks due to insufficient sensing or decision-making functions can also be mitigated through control modules. In addition, some current research focuses on the development of system self-awareness (self-awareness), thereby improving its comprehensive awareness and risk protection capabilities of the external operating environment and internal functional status. . The realization of self-awareness requires a full understanding of the system architecture and its modules from the entire vehicle level, such as building a skill map, capability map, and multi-layer view of the entire vehicle architecture for smart cars, and integrating them into the development process; Based on the self-awareness of the vehicle, system safety monitoring can be carried out, such as using environmental sensors and vehicle intrinsic sensors to perceive and represent internal and external states, and combining safety decision-making or system self-regulation technology to achieve risk protection. As the complexity of the system and the coupling degree of each module increase, the demand for comprehensive technical solutions for SOTIF improvement at the vehicle level is also increasing. However, this is limited by unclear risk mechanisms and quantitative indicators. , imperfect monitoring technology, diversity of system architecture and functional modules, and difficulties in analyzing complex systems, etc., which are still difficult to effectively deal with with current technology. The SOTIF risk protection system at the vehicle level needs to be further developed (see Figure 10), and the system guarantee of SOTIF can be achieved through comprehensive consideration of the vertical propagation of SOTIF risks and overall monitoring. ##Figure 10 Vehicle-level SOTIF risk protection system Meeting SOTIF release guidelines does not mean the complete elimination of risks. On the one hand, due to the long-tail effect of the scenario, the operation phase will inevitably encounter functional deficiencies or triggering conditions that were not considered in the development phase; on the other hand, factors such as environment, infrastructure, policies and regulations, behavioral habits, etc. may occur compared to the situation in the development phase. changes, resulting in new unknown unsafe scenarios, as shown in Figure 11. In order to effectively deal with the above unknown risks, some technologies can be used for SOTIF assurance during the operation phase, which mainly include two categories: short-term risk protection and long-term functional improvement. #Figure 11 Analysis of unknown risk sources during operation Short-term risk protection aims at real-time protection against unknown risks during the operation phase, and the key lies in risk monitoring. Anomaly detection technology can be used to identify inputs that deviate from normal data instance areas and assign anomaly scores or labels to them. It has certain monitoring capabilities for unknown risks caused by distribution shifts or out-of-distribution inputs. Common methods include supervised, semi-supervised and Unsupervised and other methods have been initially applied in tasks such as semantic segmentation and vision-based safe navigation. In addition, some research focuses on the comparison of different anomaly detection methods. Henriksson et al. proposed a structured deep learning monitor evaluation framework, using 7 evaluation indicators to compare the two types of monitors. The performance of the automatic driving monitor (convolutional neural network classifier and variational autoencoder) on different test cases, in which the autonomous driving monitor can identify new traffic scenes through anomaly detection; they expanded the above work in subsequent research and chose Four types of deep neural networks and three different monitors were compared to monitor performance during different training stages of the network, detecting the point at which monitor performance began to deteriorate. In addition, cognitive uncertainty can reflect the degree of confidence shown by the model when processing actual operating inputs. Research shows that it has certain detection capabilities for distribution shifts, unknown data inputs, etc. Typical methods for extracting cognitive uncertainty include Bayesian approximate inference, Monte Carlo dropout, deep integration and deep evidence regression, etc., as shown in Figure 12. In response to the monitored risks, security can be guaranteed through decision-making model design and strategy switching that are sensitive to uncertainty. ##Figure 12 Typical method for extracting cognitive uncertainty Research Prospects and Summary (1) Strengthen basic theoretical research on SOTIF assurance. Starting from the essence of SOTIF problems, study the generation, spread and evolution mechanism of SOTIF risks. Through theoretical analysis and experimental verification, we sorted out the potential functional deficiencies of smart cars, triggering conditions and the impact relationship between them; combined with the typical functional architecture of smart cars, we explored the impact and propagation mechanism of SOTIF issues between different modules, and studied the risk dynamics based on scenario evolution. Evolutionary theory; at the same time, in view of the uncertainty and black box problems existing in new technologies such as AI, we conduct in-depth research on the essential reasons for insufficient system functions. In addition, combined with research in statistics, information theory and other disciplines, the SOTIF risk quantification model is constructed to lay a theoretical foundation for the implementation of offline assessment certification and online risk prevention and control technology. (2) Build a SOTIF risk protection technology system. Explore system improvement ideas based on theoretical research to reduce vehicle SOTIF risks. Combining the SOTIF hazard generation mechanism and risk model, explore and optimize the function improvement technology of each module of smart cars, and further build a vehicle-level SOTIF risk protection system with self-perception and self-regulation capabilities. As shown in Figure 13, information such as the internal status of the system (such as AI model), external operating environment (such as ODD), and other constraints (such as traffic regulations) are integrated to monitor, and then an adaptive security decision-making model is designed to protect SOTIF risks. . #Figure 13 SOTIF risk protection system # (3) Promote the formation of a benign update mechanism for SOTIF assurance technology. The current smart car field itself is still in the exploratory stage, with the characteristics of coexistence of multiple routes and rapid iteration of technology. At the same time, with the development of technology, environmental changes and the long-term existence of long-tail problems in scenarios, new unknown and unsafe May keep appearing. Therefore, a healthy update mechanism for SOTIF assurance technology research should be established, the automated process of problem monitoring, feedback and update should be improved, and a flexible, fast and sustainable automatic analysis, self-learning growth and re-certification system should be explored to realize the integration of SOTIF assurance technology and smart car technology. Synchronous development. In short, SOTIF research is of great significance to whether smart cars can ultimately be accepted by society. However, the current standards in this field are not yet complete, industry practice is still in the exploratory stage and lacks support from a technical research system. Starting from the essence of the SOTIF problem, this article sorted out the SOTIF assurance technology system and proposed It provides research prospects, thereby assisting the technological research and industrial implementation of smart car SOTIF. SOTIF Overview
Key technologies for SOTIF assurance during the development phase
2. SOTIF function improvement
3. SOTIF verification and confirmation
4. SOTIF Release
Key technologies for improving smart car functions
1. Improvement of perception (including positioning) function
2 Improvement of decision-making control function
3. Handling of reasonably foreseeable misuse
4. Improvement of vehicle layer functions
SOTIF guarantee key technologies during the operation phase
On the basis of sorting out the existing key technologies for SOTIF assurance, and integrating research deficiencies and development trends, the following research is proposed Outlook.
The above is the detailed content of Key technologies for safety assurance of expected functions of smart cars. For more information, please follow other related articles on the PHP Chinese website!

Hot AI Tools

Undresser.AI Undress
AI-powered app for creating realistic nude photos

AI Clothes Remover
Online AI tool for removing clothes from photos.

Undress AI Tool
Undress images for free

Clothoff.io
AI clothes remover

Video Face Swap
Swap faces in any video effortlessly with our completely free AI face swap tool!

Hot Article

Hot Tools

Notepad++7.3.1
Easy-to-use and free code editor

SublimeText3 Chinese version
Chinese version, very easy to use

Zend Studio 13.0.1
Powerful PHP integrated development environment

Dreamweaver CS6
Visual web development tools

SublimeText3 Mac version
God-level code editing software (SublimeText3)

Hot Topics


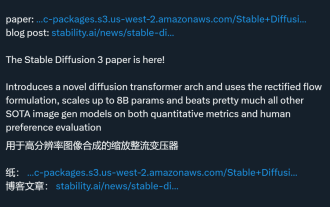
StableDiffusion3’s paper is finally here! This model was released two weeks ago and uses the same DiT (DiffusionTransformer) architecture as Sora. It caused quite a stir once it was released. Compared with the previous version, the quality of the images generated by StableDiffusion3 has been significantly improved. It now supports multi-theme prompts, and the text writing effect has also been improved, and garbled characters no longer appear. StabilityAI pointed out that StableDiffusion3 is a series of models with parameter sizes ranging from 800M to 8B. This parameter range means that the model can be run directly on many portable devices, significantly reducing the use of AI
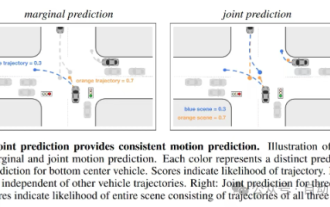
Trajectory prediction plays an important role in autonomous driving. Autonomous driving trajectory prediction refers to predicting the future driving trajectory of the vehicle by analyzing various data during the vehicle's driving process. As the core module of autonomous driving, the quality of trajectory prediction is crucial to downstream planning control. The trajectory prediction task has a rich technology stack and requires familiarity with autonomous driving dynamic/static perception, high-precision maps, lane lines, neural network architecture (CNN&GNN&Transformer) skills, etc. It is very difficult to get started! Many fans hope to get started with trajectory prediction as soon as possible and avoid pitfalls. Today I will take stock of some common problems and introductory learning methods for trajectory prediction! Introductory related knowledge 1. Are the preview papers in order? A: Look at the survey first, p
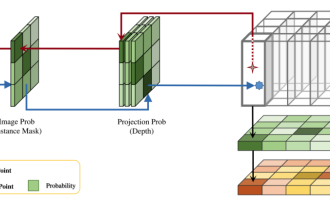
This paper explores the problem of accurately detecting objects from different viewing angles (such as perspective and bird's-eye view) in autonomous driving, especially how to effectively transform features from perspective (PV) to bird's-eye view (BEV) space. Transformation is implemented via the Visual Transformation (VT) module. Existing methods are broadly divided into two strategies: 2D to 3D and 3D to 2D conversion. 2D-to-3D methods improve dense 2D features by predicting depth probabilities, but the inherent uncertainty of depth predictions, especially in distant regions, may introduce inaccuracies. While 3D to 2D methods usually use 3D queries to sample 2D features and learn the attention weights of the correspondence between 3D and 2D features through a Transformer, which increases the computational and deployment time.
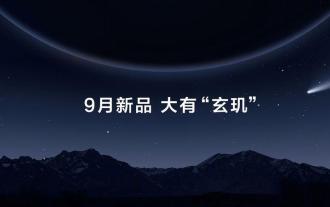
Recently, Huawei announced that it will launch a new smart wearable product equipped with Xuanji sensing system in September, which is expected to be Huawei's latest smart watch. This new product will integrate advanced emotional health monitoring functions. The Xuanji Perception System provides users with a comprehensive health assessment with its six characteristics - accuracy, comprehensiveness, speed, flexibility, openness and scalability. The system uses a super-sensing module and optimizes the multi-channel optical path architecture technology, which greatly improves the monitoring accuracy of basic indicators such as heart rate, blood oxygen and respiration rate. In addition, the Xuanji Sensing System has also expanded the research on emotional states based on heart rate data. It is not limited to physiological indicators, but can also evaluate the user's emotional state and stress level. It supports the monitoring of more than 60 sports health indicators, covering cardiovascular, respiratory, neurological, endocrine,
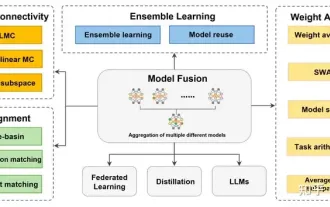
In September 23, the paper "DeepModelFusion:ASurvey" was published by the National University of Defense Technology, JD.com and Beijing Institute of Technology. Deep model fusion/merging is an emerging technology that combines the parameters or predictions of multiple deep learning models into a single model. It combines the capabilities of different models to compensate for the biases and errors of individual models for better performance. Deep model fusion on large-scale deep learning models (such as LLM and basic models) faces some challenges, including high computational cost, high-dimensional parameter space, interference between different heterogeneous models, etc. This article divides existing deep model fusion methods into four categories: (1) "Pattern connection", which connects solutions in the weight space through a loss-reducing path to obtain a better initial model fusion
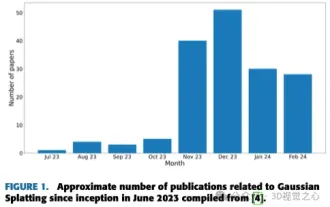
Written above & The author’s personal understanding is that image-based 3D reconstruction is a challenging task that involves inferring the 3D shape of an object or scene from a set of input images. Learning-based methods have attracted attention for their ability to directly estimate 3D shapes. This review paper focuses on state-of-the-art 3D reconstruction techniques, including generating novel, unseen views. An overview of recent developments in Gaussian splash methods is provided, including input types, model structures, output representations, and training strategies. Unresolved challenges and future directions are also discussed. Given the rapid progress in this field and the numerous opportunities to enhance 3D reconstruction methods, a thorough examination of the algorithm seems crucial. Therefore, this study provides a comprehensive overview of recent advances in Gaussian scattering. (Swipe your thumb up
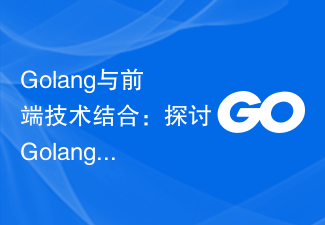
Combination of Golang and front-end technology: To explore how Golang plays a role in the front-end field, specific code examples are needed. With the rapid development of the Internet and mobile applications, front-end technology has become increasingly important. In this field, Golang, as a powerful back-end programming language, can also play an important role. This article will explore how Golang is combined with front-end technology and demonstrate its potential in the front-end field through specific code examples. The role of Golang in the front-end field is as an efficient, concise and easy-to-learn
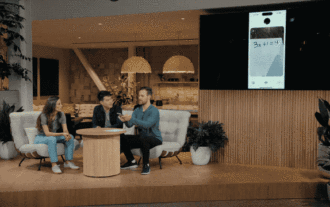
The GPT-4o model released by OpenAI is undoubtedly a huge breakthrough, especially in its ability to process multiple input media (text, audio, images) and generate corresponding output. This ability makes human-computer interaction more natural and intuitive, greatly improving the practicality and usability of AI. Several key highlights of GPT-4o include: high scalability, multimedia input and output, further improvements in natural language understanding capabilities, etc. 1. Cross-media input/output: GPT-4o+ can accept any combination of text, audio, and images as input and directly generate output from these media. This breaks the limitation of traditional AI models that only process a single input type, making human-computer interaction more flexible and diverse. This innovation helps power smart assistants
