A review of few-shot learning: techniques, algorithms and models
Machine learning has made great progress recently, but there is still a major challenge: the need for large amounts of labeled data to train models.
Sometimes this data is not available in the real world. Take healthcare as an example, we may not have enough X-ray scans to check for a new disease. But through few-shot learning, the model can learn knowledge from only a few examples!
So few-shot learning (FSL) is a subfield of machine learning, which solves the task of learning new tasks with only a small number of labeled examples The problem. The whole point of FSL is to enable machine learning models to learn new things with a little bit of data, which is useful in situations where collecting a bunch of labeled data is too expensive, takes too long, or is impractical.
Few-shot learning method
Support sample/query set: Use a small number of images to classify the query set.
There are three main methods to understand in few-shot learning: meta-learning, data-level and parameter-level.
- Meta-learning: Meta-learning involves training a model to learn how to learn new tasks efficiently;
- Data-level: Data-level methods focus on increasing available data to improve the generalization of the model. Performance;
- Parameter level: Parameter-level methods aim to learn more robust feature representations for better generalization to new tasks
Meta-learning
metalearning (learning how to learn). This method trains a model to learn how to learn new tasks efficiently. This model is about identifying commonalities between different tasks and using this knowledge to learn new things quickly with a few examples.
Meta-learning algorithms typically train a model on a set of related tasks and learn to extract task-independent features and task-specific features from the available data. Task-independent features capture general knowledge about the data, while task-specific features capture the details of the current task. During training, the algorithm learns to adapt to new tasks by updating the model parameters using only a few labeled examples of each new task. This allows the model to generalize to new tasks with few examples.
Data-level methods
Data-level methods focus on expanding existing data, which can help the model better understand the underlying structure of the data, thereby improving the generalization performance of the model.
The main idea is to create new examples by applying various transformations to existing examples, which can help the model better understand the underlying structure of the data.
There are two types of data-level methods:
- Data augmentation: Data augmentation involves creating new examples by applying different transformations to existing data;
- Data generation: Data generation involves generating new examples from scratch using generative adversarial networks (GANs).
Data-level methods:
The goal of parameter-level methods is to learn more robust feature representations that can better generalize to new tasks.
There are two parameter-level methods:
- Feature extraction: Feature extraction involves learning a set of features from the data that can be used for new tasks;
- Fine-tuning: Fine-tuning involves adapting a pre-trained model to a new task by learning optimal parameters.
For example, suppose you have a pre-trained model that can recognize different shapes and colors in images. By fine-tuning the model on new data sets, it can quickly learn to recognize new categories with just a few examples.
Meta-learning algorithm
Meta-learning is a popular approach to FSL, which involves training a model on a variety of related tasks so that it can learn how to learn new tasks efficiently. The algorithm learns to extract task-independent and task-specific features from available data, quickly adapting to new tasks.
Meta-learning algorithms can be broadly divided into two types: metric-based and gradient-based.
Metric-based meta-learning
Metric-based meta-learning algorithms learn a special way to compare different examples for each new task. They achieve this by mapping input examples into a special feature space, where similar examples are close together and dissimilar examples are far apart. The model can use this distance metric to classify new examples into the correct category.
A popular metric-based algorithm is Siamese Network, which learns to measure the distance between two input examples by using two identical subnetworks. These sub-networks generate feature representations for each input example and then compare their outputs using distance measures such as Euclidean distance or cosine similarity.
Gradient-based meta-learning
Gradient-based meta-learning learns how to update their parameters so that they can quickly adapt to new challenges.
These algorithms train models to learn an initial set of parameters and quickly adapt to new tasks with just a few examples. MAML (model-agnostic meta-learning) is a popular gradient-based meta-learning algorithm that learns how to optimize the parameters of a model to quickly adapt to new tasks. It trains the model through a series of related tasks and updates the model's parameters using some examples from each task. Once the model learns these parameters, it can fine-tune them using other examples from the current task, improving its performance.
Image classification algorithm based on few-shot learning
FSL has several algorithms, including:
- Model-Agnostic Meta-Learning ): MAML is a meta-learning algorithm that learns a good initialization for the model and can then adapt to new tasks with a small number of examples.
- Matching Networks: Matching networks learn to match new examples with labeled examples by calculating similarities.
- Prototypical Networks: Prototypical Networks learn prototype representations for each class and classify new examples based on their similarity to the prototypes.
- Relation Networks: Relation networks learn to compare pairs of examples and make predictions about new examples.
Model-agnostic meta-learning
The key idea of MAML is to learn the initialization of model parameters that can be adapted to new tasks with a few examples. During training, MAML accepts a set of related tasks and learns to update model parameters using only a few labeled examples of each task. This process enables the model to generalize to new tasks by learning good initializations of model parameters that can be quickly adapted to new tasks.
Matching network
Matching network is another commonly used few-shot image classification algorithm. Instead of learning fixed metrics or parameters, it learns dynamic metrics based on the current support set. This means that the metric used to compare the query image and the support set differs for each query image.
The matching network algorithm uses an attention mechanism to calculate a weighted sum of the support set features for each query image. Weights are learned based on the similarity between the query image and each support set image. The weighted sum of the support set features is then concatenated with the query image features, and the resulting vectors are passed through several fully connected layers to produce the final classification.
Prototype Network
Prototype Network is a simple and effective few-sample image classification algorithm. It learns a representation of the image and computes a prototype for each class using the average of the embedding features of the supporting examples. During testing, the distance between the query image and each class prototype is calculated and the class with the closest prototype is assigned to the query.
Relationship Network
Relationship network learning compares pairs of examples that support sets and uses this information to classify query examples. The relationship network includes two sub-networks: feature embedding network and relationship network. The feature embedding network maps each example in the support set and the query example into a feature space. The relation network then computes the relation score between the query example and each support set example. Finally these relationship scores are used to classify the query examples.
Applications of few-shot learning
Few-shot learning has many applications in different fields, including:
In various computer vision tasks, including image classification and target detection and segmentation. Few-shot learning can identify new objects in images that are not present in the training data.
In natural language processing tasks, such as text classification, sentiment analysis, and language modeling, few-shot learning helps improve the performance of language models on low-resource languages.
Using minority learning in robotics enables robots to quickly learn new tasks and adapt to new environments. For example, a robot can learn to pick up new objects with just a few examples.
Few samples are used in medical diagnostics to identify rare diseases and abnormalities when data is limited, and can help personalize treatments and predict patient outcomes.
Summary
Few-shot learning is a powerful technique that enables a model to learn from a small number of examples. It has numerous applications in various fields and has the potential to revolutionize machine learning. With continued research and development, few-shot learning can pave the way for more efficient and effective machine learning systems.
The above is the detailed content of A review of few-shot learning: techniques, algorithms and models. For more information, please follow other related articles on the PHP Chinese website!

Hot AI Tools

Undresser.AI Undress
AI-powered app for creating realistic nude photos

AI Clothes Remover
Online AI tool for removing clothes from photos.

Undress AI Tool
Undress images for free

Clothoff.io
AI clothes remover

Video Face Swap
Swap faces in any video effortlessly with our completely free AI face swap tool!

Hot Article

Hot Tools

Notepad++7.3.1
Easy-to-use and free code editor

SublimeText3 Chinese version
Chinese version, very easy to use

Zend Studio 13.0.1
Powerful PHP integrated development environment

Dreamweaver CS6
Visual web development tools

SublimeText3 Mac version
God-level code editing software (SublimeText3)

Hot Topics


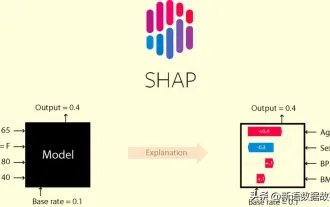
In the fields of machine learning and data science, model interpretability has always been a focus of researchers and practitioners. With the widespread application of complex models such as deep learning and ensemble methods, understanding the model's decision-making process has become particularly important. Explainable AI|XAI helps build trust and confidence in machine learning models by increasing the transparency of the model. Improving model transparency can be achieved through methods such as the widespread use of multiple complex models, as well as the decision-making processes used to explain the models. These methods include feature importance analysis, model prediction interval estimation, local interpretability algorithms, etc. Feature importance analysis can explain the decision-making process of a model by evaluating the degree of influence of the model on the input features. Model prediction interval estimate
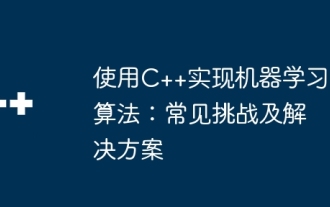
Common challenges faced by machine learning algorithms in C++ include memory management, multi-threading, performance optimization, and maintainability. Solutions include using smart pointers, modern threading libraries, SIMD instructions and third-party libraries, as well as following coding style guidelines and using automation tools. Practical cases show how to use the Eigen library to implement linear regression algorithms, effectively manage memory and use high-performance matrix operations.
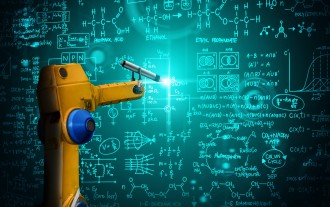
Machine learning is an important branch of artificial intelligence that gives computers the ability to learn from data and improve their capabilities without being explicitly programmed. Machine learning has a wide range of applications in various fields, from image recognition and natural language processing to recommendation systems and fraud detection, and it is changing the way we live. There are many different methods and theories in the field of machine learning, among which the five most influential methods are called the "Five Schools of Machine Learning". The five major schools are the symbolic school, the connectionist school, the evolutionary school, the Bayesian school and the analogy school. 1. Symbolism, also known as symbolism, emphasizes the use of symbols for logical reasoning and expression of knowledge. This school of thought believes that learning is a process of reverse deduction, through existing
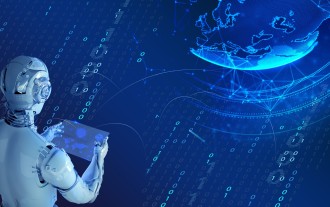
Translator | Reviewed by Li Rui | Chonglou Artificial intelligence (AI) and machine learning (ML) models are becoming increasingly complex today, and the output produced by these models is a black box – unable to be explained to stakeholders. Explainable AI (XAI) aims to solve this problem by enabling stakeholders to understand how these models work, ensuring they understand how these models actually make decisions, and ensuring transparency in AI systems, Trust and accountability to address this issue. This article explores various explainable artificial intelligence (XAI) techniques to illustrate their underlying principles. Several reasons why explainable AI is crucial Trust and transparency: For AI systems to be widely accepted and trusted, users need to understand how decisions are made
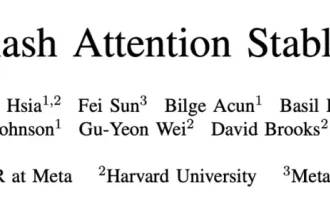
MetaFAIR teamed up with Harvard to provide a new research framework for optimizing the data bias generated when large-scale machine learning is performed. It is known that the training of large language models often takes months and uses hundreds or even thousands of GPUs. Taking the LLaMA270B model as an example, its training requires a total of 1,720,320 GPU hours. Training large models presents unique systemic challenges due to the scale and complexity of these workloads. Recently, many institutions have reported instability in the training process when training SOTA generative AI models. They usually appear in the form of loss spikes. For example, Google's PaLM model experienced up to 20 loss spikes during the training process. Numerical bias is the root cause of this training inaccuracy,

01 Outlook Summary Currently, it is difficult to achieve an appropriate balance between detection efficiency and detection results. We have developed an enhanced YOLOv5 algorithm for target detection in high-resolution optical remote sensing images, using multi-layer feature pyramids, multi-detection head strategies and hybrid attention modules to improve the effect of the target detection network in optical remote sensing images. According to the SIMD data set, the mAP of the new algorithm is 2.2% better than YOLOv5 and 8.48% better than YOLOX, achieving a better balance between detection results and speed. 02 Background & Motivation With the rapid development of remote sensing technology, high-resolution optical remote sensing images have been used to describe many objects on the earth’s surface, including aircraft, cars, buildings, etc. Object detection in the interpretation of remote sensing images
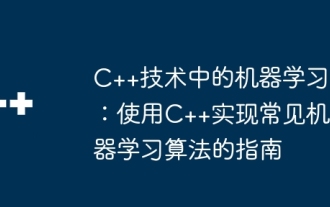
In C++, the implementation of machine learning algorithms includes: Linear regression: used to predict continuous variables. The steps include loading data, calculating weights and biases, updating parameters and prediction. Logistic regression: used to predict discrete variables. The process is similar to linear regression, but uses the sigmoid function for prediction. Support Vector Machine: A powerful classification and regression algorithm that involves computing support vectors and predicting labels.
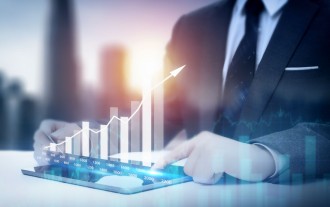
1. Background of the Construction of 58 Portraits Platform First of all, I would like to share with you the background of the construction of the 58 Portrait Platform. 1. The traditional thinking of the traditional profiling platform is no longer enough. Building a user profiling platform relies on data warehouse modeling capabilities to integrate data from multiple business lines to build accurate user portraits; it also requires data mining to understand user behavior, interests and needs, and provide algorithms. side capabilities; finally, it also needs to have data platform capabilities to efficiently store, query and share user profile data and provide profile services. The main difference between a self-built business profiling platform and a middle-office profiling platform is that the self-built profiling platform serves a single business line and can be customized on demand; the mid-office platform serves multiple business lines, has complex modeling, and provides more general capabilities. 2.58 User portraits of the background of Zhongtai portrait construction
