


With low energy consumption and low time consumption, the Chinese Academy of Sciences & University of Hong Kong team uses a new method to perform multi-task learning for internal reservoir calculations in wearable sensors.
In-sensor multi-task learning is not only a key benefit of biological vision, but also a major goal of artificial intelligence. However, traditional silicon vision chips have a large time and energy overhead. Additionally, training traditional deep learning models is neither scalable nor affordable on edge devices.
Here, a research team from the Chinese Academy of Sciences and the University of Hong Kong proposes a materials algorithm co-design to simulate the learning paradigm of the human retina with low overhead. Based on the bottlebrush-shaped semiconductor p-NDI with efficient exciton dissociation and through-space charge transport properties, a wearable transistor-based dynamic sensor reservoir computing system is developed that exhibits excellent separability on different tasks properties, attenuation memory and echo state characteristics.
Combined with the "readout function" on the memristive organic diode, RC can recognize handwritten letters and numbers, and classify various clothing, with an accuracy of 98.04%, 88.18% and 91.76% (higher than all reported organic semiconductors).
In addition to 2D images, RC’s spatiotemporal dynamics naturally extract features from event-based videos to classify 3 types of gestures with 98.62% accuracy. In addition, the computational cost is significantly lower than traditional artificial neural networks. This work provides a promising materials-algorithm co-design for affordable and efficient photonic neuromorphic systems.
The research is titled "Wearable in-sensor reservoir computing using optoelectronic polymers with through-space charge-transport characteristics for multi-task learning" and will be published in 2023 Published in "Nature Communications" on January 28th.
The human retina not only senses, but also processes light signals simultaneously by collecting rich dynamic signals, thereby accelerating task-related learning in the downstream visual cortex. The synergy of the retina and visual cortex underlies the brain's ability to efficiently, compactly and rapidly learn multitasking and is a fundamental goal of artificial general intelligence (AGI).
In contrast, traditional silicon vision chips with physically separated sensing, processing, and storage units incur significant time and overhead due to the large and frequent data shuttling between these units. Energy overhead, as well as sequential analog-to-digital conversion, is a fundamental limitation on potential energy efficiency. This situation is further exacerbated by the slowdown in Moore's Law. Furthermore, learning in traditional deep learning models, such as recurrent neural networks for temporal signals, employs tedious training on very specific tasks (e.g., gradient descent via backpropagation through time, BPTT), which is difficult in battery access and form factors. It is neither scalable nor affordable on edge devices with limited form factors.
Significant efforts have been made to simulate the human retina and affordable learning paradigms. In terms of materials, inorganic photoresponsive two-dimensional semiconductors, such as MoS2 with defects and impurity sites, SnS with double-type defect states related to Sn and S, layered Oxidation-related defects of black phosphorus, perovskite quantum dots showing strong light control effect, h-BN/WSe2 heterostructure and performance bid that can capture and release electrons State-changing MoOx is the most widely used material for artificial retina. Additionally, organic semiconductors that are inherently biocompatible, wearable, and scalable, such as PDVT-10, chlorophyll-doped PDPP4T, and pentacene/silk and CDs bilayers, mimic biological counterparts in a more faithful manner. things.
In terms of algorithms, Reservoir Computing (RC) non-linearly projects temporal signals into feature space by collecting the fading memory of a fixed dynamic system and is considered a promising edge Learn solutions. Since the learning of RC is limited to the readout layer of long-term memory, the training cost is significantly reduced compared to traditional deep learning models. However, it still has not devised a paired materials algorithm to combine efficient artificial retina and affordable RC-based edge learning to unleash the multi-tasking potential of biomimetic neuromorphic vision.
#Illustration: Comparison of photocurrent response of traditional semiconductor and p-NDI, and RC system within the sensor Detailed semiconductor design principles. (Source: paper)
Here, researchers from the Chinese Academy of Sciences and the University of Hong Kong propose a material algorithm co-design of a photoresponsive semiconductor polymer (p-NDI) with efficient exciton dissociation and full-space charge transport properties. , to build an in-sensor RC for multi-task pattern classification. The flexible neuromorphic device is based on a three-terminal transistor with a p-NDI semiconductor channel. Due to its excellent photoresponse behavior and nonlinear fading memory, the device is able to simultaneously sense, remember, and preprocess optical inputs in situ (i.e., contrast enhancement and noise reduction).
Illustration: Multi-task classification performance. (Source: Paper)
#In addition, the synergy between exciton dissociation/charge recombination dynamics, photogating effects and through-space charge transport properties in polymers This enables the transistor-based dynamic RC system to exhibit excellent separability, attenuation memory and echo state characteristics on different tasks. These RC-based retinas are paired with a "readout function" implemented on a memristive organic ion gel diode.
The synergistic functions of signal preprocessing and dynamic RC provided by all organic optoelectronic materials achieve an accuracy of 98.04% in identifying handwritten letters and numbers and classifying various clothing respectively. , 88.18% and 91.76%, which means multi-task learning of clothing styles and sizes. The overall accuracy of the system is 88.00%, not only correctly identifying clothes, but also correctly identifying the size of the clothes. Despite being 2D images, the spatiotemporal dynamics of RC were used to classify event-based videos of left-hand waving, right-hand waving, and clapping gestures with 98.62% accuracy.
Illustration: Event-based video classification using the DVSGesture128 dataset. (Source: paper)
However, this p-NDI transistor-based RC does not contain the liquid electrolyte widely used in synaptic organic electrochemical transistors, thereby enhancing the reliability Scalability and operability. This work provides a promising material-algorithm co-design strategy for wearable, affordable, and efficient photonic neuromorphic systems with multi-task learning capabilities.
Paper link: https://www.nature.com/articles/s41467-023-36205-9
The above is the detailed content of With low energy consumption and low time consumption, the Chinese Academy of Sciences & University of Hong Kong team uses a new method to perform multi-task learning for internal reservoir calculations in wearable sensors.. For more information, please follow other related articles on the PHP Chinese website!

Hot AI Tools

Undresser.AI Undress
AI-powered app for creating realistic nude photos

AI Clothes Remover
Online AI tool for removing clothes from photos.

Undress AI Tool
Undress images for free

Clothoff.io
AI clothes remover

AI Hentai Generator
Generate AI Hentai for free.

Hot Article

Hot Tools

Notepad++7.3.1
Easy-to-use and free code editor

SublimeText3 Chinese version
Chinese version, very easy to use

Zend Studio 13.0.1
Powerful PHP integrated development environment

Dreamweaver CS6
Visual web development tools

SublimeText3 Mac version
God-level code editing software (SublimeText3)

Hot Topics


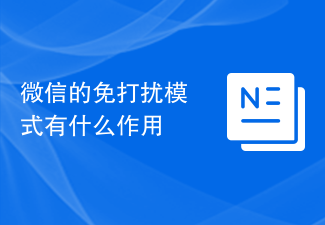
What does WeChat Do Not Disturb mode mean? Nowadays, with the popularity of smartphones and the rapid development of mobile Internet, social media platforms have become an indispensable part of people's daily lives. WeChat is one of the most popular social media platforms in China, and almost everyone has a WeChat account. We can communicate with friends, family, and colleagues in real time through WeChat, share moments in our lives, and understand each other’s current situation. However, in this era, we are also inevitably faced with the problems of information overload and privacy leakage, especially for those who need to focus or
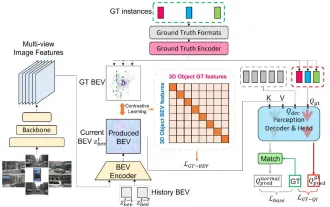
Written above & the author’s personal understanding: At present, in the entire autonomous driving system, the perception module plays a vital role. The autonomous vehicle driving on the road can only obtain accurate perception results through the perception module. The downstream regulation and control module in the autonomous driving system makes timely and correct judgments and behavioral decisions. Currently, cars with autonomous driving functions are usually equipped with a variety of data information sensors including surround-view camera sensors, lidar sensors, and millimeter-wave radar sensors to collect information in different modalities to achieve accurate perception tasks. The BEV perception algorithm based on pure vision is favored by the industry because of its low hardware cost and easy deployment, and its output results can be easily applied to various downstream tasks.
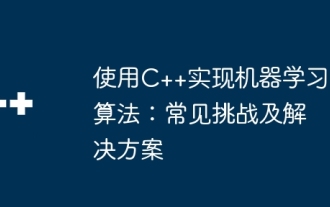
Common challenges faced by machine learning algorithms in C++ include memory management, multi-threading, performance optimization, and maintainability. Solutions include using smart pointers, modern threading libraries, SIMD instructions and third-party libraries, as well as following coding style guidelines and using automation tools. Practical cases show how to use the Eigen library to implement linear regression algorithms, effectively manage memory and use high-performance matrix operations.
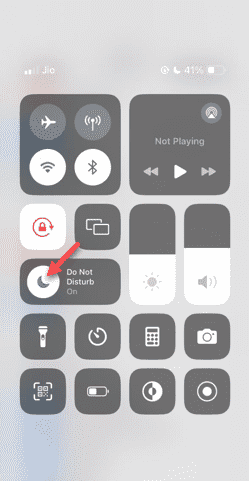
Even answering calls in Do Not Disturb mode can be a very annoying experience. As the name suggests, Do Not Disturb mode turns off all incoming call notifications and alerts from emails, messages, etc. You can follow these solution sets to fix it. Fix 1 – Enable Focus Mode Enable focus mode on your phone. Step 1 – Swipe down from the top to access Control Center. Step 2 – Next, enable “Focus Mode” on your phone. Focus Mode enables Do Not Disturb mode on your phone. It won't cause any incoming call alerts to appear on your phone. Fix 2 – Change Focus Mode Settings If there are some issues in the focus mode settings, you should fix them. Step 1 – Open your iPhone settings window. Step 2 – Next, turn on the Focus mode settings
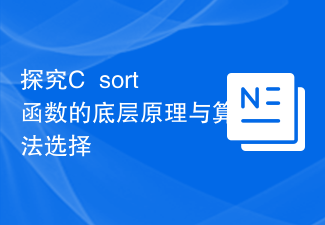
The bottom layer of the C++sort function uses merge sort, its complexity is O(nlogn), and provides different sorting algorithm choices, including quick sort, heap sort and stable sort.
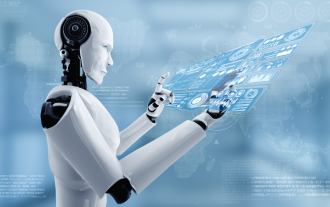
The convergence of artificial intelligence (AI) and law enforcement opens up new possibilities for crime prevention and detection. The predictive capabilities of artificial intelligence are widely used in systems such as CrimeGPT (Crime Prediction Technology) to predict criminal activities. This article explores the potential of artificial intelligence in crime prediction, its current applications, the challenges it faces, and the possible ethical implications of the technology. Artificial Intelligence and Crime Prediction: The Basics CrimeGPT uses machine learning algorithms to analyze large data sets, identifying patterns that can predict where and when crimes are likely to occur. These data sets include historical crime statistics, demographic information, economic indicators, weather patterns, and more. By identifying trends that human analysts might miss, artificial intelligence can empower law enforcement agencies

01 Outlook Summary Currently, it is difficult to achieve an appropriate balance between detection efficiency and detection results. We have developed an enhanced YOLOv5 algorithm for target detection in high-resolution optical remote sensing images, using multi-layer feature pyramids, multi-detection head strategies and hybrid attention modules to improve the effect of the target detection network in optical remote sensing images. According to the SIMD data set, the mAP of the new algorithm is 2.2% better than YOLOv5 and 8.48% better than YOLOX, achieving a better balance between detection results and speed. 02 Background & Motivation With the rapid development of remote sensing technology, high-resolution optical remote sensing images have been used to describe many objects on the earth’s surface, including aircraft, cars, buildings, etc. Object detection in the interpretation of remote sensing images
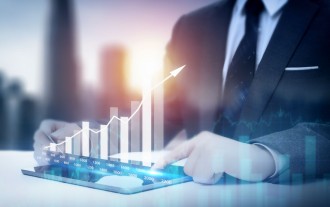
1. Background of the Construction of 58 Portraits Platform First of all, I would like to share with you the background of the construction of the 58 Portrait Platform. 1. The traditional thinking of the traditional profiling platform is no longer enough. Building a user profiling platform relies on data warehouse modeling capabilities to integrate data from multiple business lines to build accurate user portraits; it also requires data mining to understand user behavior, interests and needs, and provide algorithms. side capabilities; finally, it also needs to have data platform capabilities to efficiently store, query and share user profile data and provide profile services. The main difference between a self-built business profiling platform and a middle-office profiling platform is that the self-built profiling platform serves a single business line and can be customized on demand; the mid-office platform serves multiple business lines, has complex modeling, and provides more general capabilities. 2.58 User portraits of the background of Zhongtai portrait construction
