ImageNet label error removed, model ranking changed significantly
Previously, ImageNet became a hot topic because of the problem of label errors. You may be surprised to hear this number. There are at least 100,000 labels with problems. Studies based on incorrect labels may have to be overturned and repeated.
From this point of view, managing the quality of data sets is still very important.
Many people will use the ImageNet data set as a benchmark, but based on the ImageNet pre-trained model, the final results may vary due to data quality.
In this article, Kenichi Higuchi, an engineer from Adansons Company, re-studies the ImageNet data set in the article "Are we done with ImageNet?", and after removing the wrong label data, re-evaluates it and publishes it on torchvision model.
Remove erroneous data from ImageNet and re-evaluate the model
This paper divides labeling errors in ImageNet into three categories, as follows.
(1) Data with incorrect labeling
(2) Data corresponding to multiple labels
(3) Data that does not belong to any label
In summary, there are approximately more than 14,000 erroneous data. Considering that the number of evaluation data is 50,000, it can be seen that the proportion of erroneous data is extremely high. The figure below shows some representative error data.
Method
Without retraining the model, this study only excludes incorrectly labeled data, That is, the above-mentioned type (1) erroneous data, and excluding all erroneous data from the evaluation data, that is, (1)-(3) erroneous data, to recheck the accuracy of the model.
In order to delete error data, a metadata file describing the label error information is required. In this metadata file, if it contains errors of type (1)-(3), the information will be described in the "correction" attribute.
The study used a tool called Adansons Base, which filters data by linking datasets to metadata. 10 models were tested here as shown below.
10 image classification models used for testing
Results
The results are shown in the table below (numeric values is the accuracy in %, the number in brackets is the ranking)
The results of 10 classification models
With All Eval data is the baseline. Excluding incorrect data types (1), the accuracy increases by an average of 3.122 points. Excluding all incorrect data (1) to (3), the accuracy increases by an average of 11.743 points.
As expected, excluding erroneous data, the accuracy rate is improved across the board. There is no doubt that compared with clean data, erroneous data is prone to errors.
The accuracy ranking of the model changed when the evaluation was performed without excluding erroneous data, and when erroneous data (1)~(3) were all excluded.
In this article, there are 3,670 erroneous data (1), accounting for 7.34% of the total 50,000 pieces of data. After removal, the accuracy rate increased by about 3.22 points on average. When erroneous data is removed, the data scale changes, and a simple comparison of accuracy rates may be biased.
Conclusion
Although not specifically emphasized, it is important to use accurately labeled data when doing evaluation training.
Previous studies may have drawn incorrect conclusions when comparing accuracy between models. So the data should be evaluated first, but can this really be used to evaluate the performance of the model?
Many models using deep learning often disdain to reflect on the data, but are eager to improve accuracy and other evaluation metrics through the performance of the model, even if the evaluation data contains erroneous data. Not processed accurately.
When creating your own data sets, such as when applying AI in business, creating high-quality data sets is directly related to improving the accuracy and reliability of AI. The experimental results of this paper show that simply improving data quality can improve accuracy by about 10 percentage points, which demonstrates the importance of improving not only the model but also the data set when developing AI systems.
However, ensuring the quality of the data set is not easy. While increasing the amount of metadata is important to properly assess the quality of AI models and data, it can be cumbersome to manage, especially with unstructured data.
The above is the detailed content of ImageNet label error removed, model ranking changed significantly. For more information, please follow other related articles on the PHP Chinese website!

Hot AI Tools

Undresser.AI Undress
AI-powered app for creating realistic nude photos

AI Clothes Remover
Online AI tool for removing clothes from photos.

Undress AI Tool
Undress images for free

Clothoff.io
AI clothes remover

Video Face Swap
Swap faces in any video effortlessly with our completely free AI face swap tool!

Hot Article

Hot Tools

Notepad++7.3.1
Easy-to-use and free code editor

SublimeText3 Chinese version
Chinese version, very easy to use

Zend Studio 13.0.1
Powerful PHP integrated development environment

Dreamweaver CS6
Visual web development tools

SublimeText3 Mac version
God-level code editing software (SublimeText3)

Hot Topics


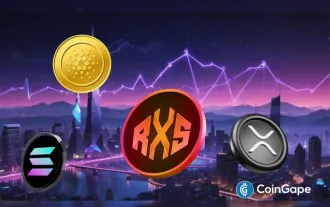
In the volatile cryptocurrency market, investors are looking for alternatives that go beyond popular currencies. Although well-known cryptocurrencies such as Solana (SOL), Cardano (ADA), XRP and Dogecoin (DOGE) also face challenges such as market sentiment, regulatory uncertainty and scalability. However, a new emerging project, RexasFinance (RXS), is emerging. It does not rely on celebrity effects or hype, but focuses on combining real-world assets (RWA) with blockchain technology to provide investors with an innovative way to invest. This strategy makes it hoped to be one of the most successful projects of 2025. RexasFi
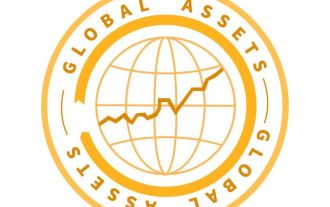
Global Assets launches a new AI intelligent trading system to lead the new era of trading efficiency! The well-known comprehensive trading platform Global Assets officially launched its AI intelligent trading system, aiming to use technological innovation to improve global trading efficiency, optimize user experience, and contribute to the construction of a safe and reliable global trading platform. The move marks a key step for global assets in the field of smart finance, further consolidating its global market leadership. Opening a new era of technology-driven and open intelligent trading. Against the backdrop of in-depth development of digitalization and intelligence, the trading market's dependence on technology is increasing. The AI intelligent trading system launched by Global Assets integrates cutting-edge technologies such as big data analysis, machine learning and blockchain, and is committed to providing users with intelligent and automated trading services to effectively reduce human factors.
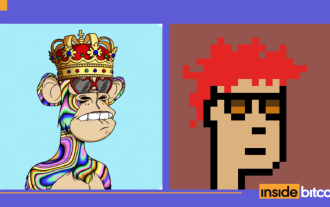
DefiDungeons, an NFT series in a fantasy placement RPG game powered by the Solana blockchain, showed strong market performance at the beginning of April 2025. The global NFT market started strongly in April after weeks of growth in trading volume. In the past 24 hours, the total NFT transaction volume reached US$14 million, with trading volume soaring 46% from the previous day. Here are the hottest NFT series in the first week of April: DefiDungeonsNFT series: This fantasy placement RPG game NFT on the Solana blockchain became the best-selling NFT series in the first week of April. In the past 24 hours, the transaction volume reached US$1.5 million, with a total market value of more than US$5 million.
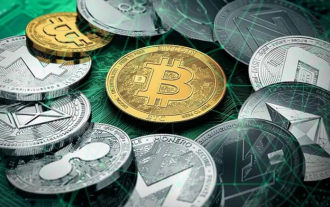
Factors of rising virtual currency prices include: 1. Increased market demand, 2. Decreased supply, 3. Stimulated positive news, 4. Optimistic market sentiment, 5. Macroeconomic environment; Decline factors include: 1. Decreased market demand, 2. Increased supply, 3. Strike of negative news, 4. Pessimistic market sentiment, 5. Macroeconomic environment.
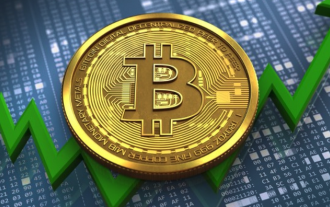
Binance is the overlord of the global digital asset trading ecosystem, and its characteristics include: 1. The average daily trading volume exceeds $150 billion, supports 500 trading pairs, covering 98% of mainstream currencies; 2. The innovation matrix covers the derivatives market, Web3 layout and education system; 3. The technical advantages are millisecond matching engines, with peak processing volumes of 1.4 million transactions per second; 4. Compliance progress holds 15-country licenses and establishes compliant entities in Europe and the United States.
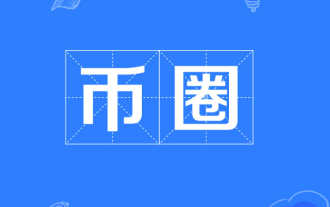
The top exchanges include: 1. Binance, the world's largest trading volume, supports 600 currencies, and the spot handling fee is 0.1%; 2. OKX, a balanced platform, supports 708 trading pairs, and the perpetual contract handling fee is 0.05%; 3. Gate.io, covers 2700 small currencies, and the spot handling fee is 0.1%-0.3%; 4. Coinbase, the US compliance benchmark, the spot handling fee is 0.5%; 5. Kraken, the top security, and regular reserve audit.
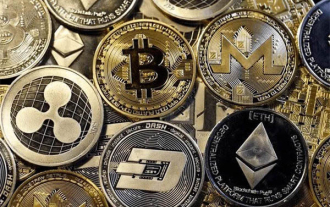
Binance, OKX, Coinbase, Kraken, Huobi, Gate.io, Gemini, XBIT, Bitget and MEXC are all strictly selected platforms for regulatory selection. 1. Binance adapts to supervision by setting up a compliance sub-platform. 2. OKX strengthens compliance management through KYB verification. 3.Coinbase is known for its compliance and user-friendliness. 4.Kraken provides powerful KYC and anti-money laundering measures. 5. Huobi operates in compliance with the Asian market. 6.Gate.io focuses on transaction security and compliance. 7. Gemini focuses on security and transparency. 8.XBIT is a model of decentralized transaction compliance. 9.Bitget continues to work in compliance operations. 10.MEXC passed
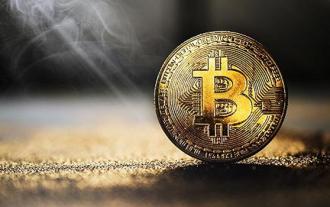
The investment prospects of crypto finance and AaaS businesses are analyzed as follows: 1. Opportunities of crypto finance include market size growth, gradual clear regulation and expansion of application scenarios, but face market volatility and technical security challenges. 2. The opportunities of AaaS business lie in the promotion of technological innovation, data value mining and rich application scenarios, but the challenges include technical complexity and market acceptance.
