


An introduction to four methods that combine mathematical optimization and machine learning
Mathematical Optimization and Machine Learning
Mathematical optimization (or mathematical programming) is a powerful decision-making tool. By setting goals and specifying constraints and variables, mathematical optimization can help make the best decisions under current real-world circumstances. It has proven its worth in many different industries including aviation, logistics, power and finance.
Machine learning is a branch of artificial intelligence. Computers can recognize patterns in data and learn to predict the future. They can perform clustering, detect anomalies, or generate new music or images. The three types of machine learning (supervised, unsupervised, and reinforcement learning) can be applied to all industries, such as healthcare and even art. Machine learning models are all about probability, and predicting the probability of something happening
Both approaches have advantages and disadvantages. When the data changes too much, the machine learning model becomes useless and the model needs to be retrained or rebuilt from scratch. Mathematical optimization requires good mathematical description, and it cannot handle unstructured data like machine learning. Additionally, if the problem becomes too large, you may need a commercial fix to fix the problem, which can be quite expensive.
Some problems are better suited to machine learning, while other problems are better suited to mathematical optimization. Machine learning should be used when you want to discover patterns in data, find similar data samples, or predict the weather. If you want to create a schedule, find the optimal location of a facility or minimize the cost of a problem, mathematical optimization is a better choice.
How to combine mathematical optimization and machine learning?
It is useful to combine mathematical optimization and machine learning. They have different advantages and disadvantages, and some problems are too complex to use just one of the two. They can complement each other. Here are four methods and practical examples of how to combine them.
1. Using Machine Learning Predictions as Constraints in Optimization Models
First, you use machine learning to make predictions that are used as input to the optimization problem. You can use the output of a machine learning model to set constraints.
Example: Use machine learning to predict viewership, using them as input to create an optimized optimal plan
Suppose you are a data scientist for a platform and you sell ad space to other companies. Advertisers buy airtime, and the platform predicts based on experience how many people will see the advertiser's ads. As a data scientist, you want to use your advertising space in the best possible way. First, use machine learning to predict viewing data based on historical data. Then, create an optimization model that uses viewing data as input. You optimize your plan by watching the data. By doing this, you can maximize the platform’s profits.
2. Use optimization decisions as training features in machine learning models
Compared to method 1, this is the opposite way: first the optimization model makes decisions, and the decisions are used as machine learning features in the model. In practice, this approach is less common since most decisions (MO) follow predictions (ML). This approach may be useful in certain projects.
Example: Using transportation decisions in machine learning models
Mathematical optimization has a wide range of applications in logistics. If you use optimization to decide how much supply needs to be shipped from the production plant to the market, it can save a lot of time, money, and resources. After you obtain these results, you can use them in machine learning problems, such as predicting how many employees each factory will need on a specific day.
3. Utilize machine learning outputs to scope mathematical optimization models
In addition to using machine learning outputs directly in optimization problems, you can also choose to treat them independently combined. You can use them in the same project, but not in the same process. You can use machine learning output to simplify mathematical optimization problems: You can use machine learning to determine the scope of an optimization model. An added benefit here is that the optimized model can be solved in less time.
Example: Using Predictive Maintenance and Clustering to Determine the Scope of Routing Issues
In this example, we look at a company that repairs power boxes. They have a limited number of repairmen and want to use them in the best possible way. First, you can use predictive maintenance (machine learning) to decide which power boxes have the highest risk of failure. Then, clustering (machine learning) is used to cluster high-risk power boxes. Clustering occurs because you want a group of power boxes to be close to each other. You can choose a number of clusters equal to the number of repairmen available. Finally, through mathematical optimization, you can create the best route between the power boxes for each cluster, one route for each repairman.
4. Use optimization to solve machine learning problems
You can use optimization to find an optimal set of parameters for a machine learning problem. Machine learning and mathematical optimization are closely coupled here, since optimization is used in machine learning problems. The following example shows that Mixed Integer Programming (MIP) has proven its worth in solving a classic research problem.
Example: Solving the optimal subset selection problem in linear regression
When building a regression model, removing irrelevant features will make the model easier to interpret and less likely to overfit the data. It is difficult to find the optimal subset of features, which is called the optimal subset selection problem. Mixed integer programming (mathematical optimization) has seen significant improvements in speed over the past few decades, which makes it useful to test it on existing problems.
Finally
I hope this article inspired you to try an interesting combination of mathematical optimization and machine learning! They are suitable for different types of problems and can complement each other. Besides the obvious way (using the output of one as the input of the other), there are other ways to combine them. You can loosely combine them, as in the third approach, using machine learning to scope the optimization problem. Or you can integrate them tightly to solve a research problem, as in the last example.
The above is the detailed content of An introduction to four methods that combine mathematical optimization and machine learning. For more information, please follow other related articles on the PHP Chinese website!

Hot AI Tools

Undresser.AI Undress
AI-powered app for creating realistic nude photos

AI Clothes Remover
Online AI tool for removing clothes from photos.

Undress AI Tool
Undress images for free

Clothoff.io
AI clothes remover

AI Hentai Generator
Generate AI Hentai for free.

Hot Article

Hot Tools

Notepad++7.3.1
Easy-to-use and free code editor

SublimeText3 Chinese version
Chinese version, very easy to use

Zend Studio 13.0.1
Powerful PHP integrated development environment

Dreamweaver CS6
Visual web development tools

SublimeText3 Mac version
God-level code editing software (SublimeText3)

Hot Topics


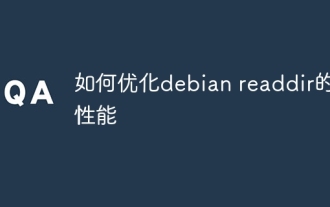
In Debian systems, readdir system calls are used to read directory contents. If its performance is not good, try the following optimization strategy: Simplify the number of directory files: Split large directories into multiple small directories as much as possible, reducing the number of items processed per readdir call. Enable directory content caching: build a cache mechanism, update the cache regularly or when directory content changes, and reduce frequent calls to readdir. Memory caches (such as Memcached or Redis) or local caches (such as files or databases) can be considered. Adopt efficient data structure: If you implement directory traversal by yourself, select more efficient data structures (such as hash tables instead of linear search) to store and access directory information
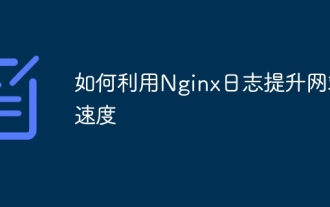
Website performance optimization is inseparable from in-depth analysis of access logs. Nginx log records the detailed information of users visiting the website. Cleverly using this data can effectively improve the speed of the website. This article will introduce several website performance optimization methods based on Nginx logs. 1. User behavior analysis and optimization. By analyzing the Nginx log, we can gain a deep understanding of user behavior and make targeted optimization based on this: High-frequency access IP identification: Find the IP address with the highest access frequency, and optimize the server resource configuration for these IP addresses, such as increasing bandwidth or improving the response speed of specific content. Status code analysis: analyze the frequency of different HTTP status codes (such as 404 errors), find out problems in website navigation or content management, and proceed
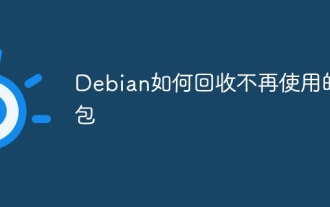
This article describes how to clean useless software packages and free up disk space in the Debian system. Step 1: Update the package list Make sure your package list is up to date: sudoaptupdate Step 2: View installed packages Use the following command to view all installed packages: dpkg--get-selections|grep-vdeinstall Step 3: Identify redundant packages Use the aptitude tool to find packages that are no longer needed. aptitude will provide suggestions to help you safely delete packages: sudoaptitudesearch '~pimportant' This command lists the tags
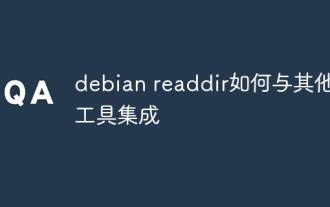
The readdir function in the Debian system is a system call used to read directory contents and is often used in C programming. This article will explain how to integrate readdir with other tools to enhance its functionality. Method 1: Combining C language program and pipeline First, write a C program to call the readdir function and output the result: #include#include#include#includeintmain(intargc,char*argv[]){DIR*dir;structdirent*entry;if(argc!=2){
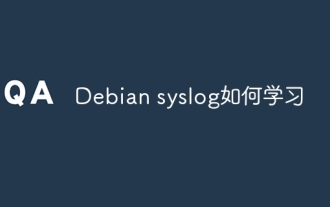
This guide will guide you to learn how to use Syslog in Debian systems. Syslog is a key service in Linux systems for logging system and application log messages. It helps administrators monitor and analyze system activity to quickly identify and resolve problems. 1. Basic knowledge of Syslog The core functions of Syslog include: centrally collecting and managing log messages; supporting multiple log output formats and target locations (such as files or networks); providing real-time log viewing and filtering functions. 2. Install and configure Syslog (using Rsyslog) The Debian system uses Rsyslog by default. You can install it with the following command: sudoaptupdatesud
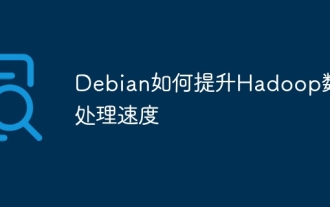
This article discusses how to improve Hadoop data processing efficiency on Debian systems. Optimization strategies cover hardware upgrades, operating system parameter adjustments, Hadoop configuration modifications, and the use of efficient algorithms and tools. 1. Hardware resource strengthening ensures that all nodes have consistent hardware configurations, especially paying attention to CPU, memory and network equipment performance. Choosing high-performance hardware components is essential to improve overall processing speed. 2. Operating system tunes file descriptors and network connections: Modify the /etc/security/limits.conf file to increase the upper limit of file descriptors and network connections allowed to be opened at the same time by the system. JVM parameter adjustment: Adjust in hadoop-env.sh file
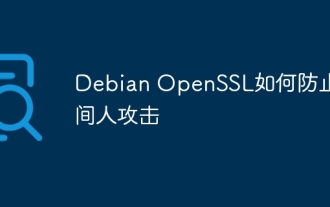
In Debian systems, OpenSSL is an important library for encryption, decryption and certificate management. To prevent a man-in-the-middle attack (MITM), the following measures can be taken: Use HTTPS: Ensure that all network requests use the HTTPS protocol instead of HTTP. HTTPS uses TLS (Transport Layer Security Protocol) to encrypt communication data to ensure that the data is not stolen or tampered during transmission. Verify server certificate: Manually verify the server certificate on the client to ensure it is trustworthy. The server can be manually verified through the delegate method of URLSession
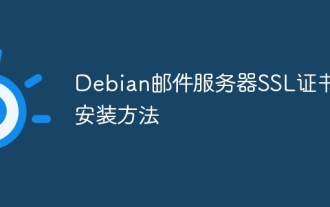
The steps to install an SSL certificate on the Debian mail server are as follows: 1. Install the OpenSSL toolkit First, make sure that the OpenSSL toolkit is already installed on your system. If not installed, you can use the following command to install: sudoapt-getupdatesudoapt-getinstallopenssl2. Generate private key and certificate request Next, use OpenSSL to generate a 2048-bit RSA private key and a certificate request (CSR): openss
