


Don't think step by step! Google's latest natural language reasoning algorithm LAMBADA: 'Reverse chain reasoning” is the answer
Automatic reasoning is definitely a big problem in the field of natural language processing. The model needs to derive effective and correct conclusions based on given premises and knowledge.
Although the field of NLP has achieved extremely high performance in various "natural language understanding" tasks such as reading comprehension and question answering through large-scale pre-trained language models in recent years, these The model's performance in logical reasoning is still lagging.
In May last year, "Chain of Thought" (CoT) came out. Some researchers found that just adding "Let's think step by step" to the prompt can The inference performance of GPT-3 has been greatly improved. For example, in MultiArith, the inference accuracy has been increased from the previous 17.7% to 78.7%
However, methods such as CoT and Selection Inference have It is to search the proof process (proof) from the axioms (axioms) in a forward direction to derive the final conclusion (conclusion). There is a problem of search space combination explosion, so for longer reasoning chains, the failure rate is higher. .
Recently, Google Research developed a Backward Chaining algorithm LAMBADA (LAnguage Model augmented BAckwarD chAining), which combines the "backward reasoning efficiency" derived from classic reasoning literature. "Significantly higher than forward reasoning." This conclusion is applied to language models (LM).
Paper link: https://arxiv.org/abs/2212.13894
LAMBADA will reason The process is decomposed into four sub-modules, each of which is implemented by few-shot prompted language model reasoning.
In the end, LAMBADA achieved significant performance improvements on the two logical reasoning data sets compared to the current forward reasoning method of sota, especially when the problem requires depth and accurate proof chain. , the performance improvement of LAMBADA is more obvious.
"Reverse reasoning" becomes the answer?
Logical reasoning, especially logical reasoning on unstructured natural text, is the basic building block for automatic knowledge discovery and the key to future progress in various scientific fields.
Although the development of many NLP tasks has benefited from the increasing scale of pre-trained language models, it has been observed that increasing the size of the model has very limited improvement in solving complex reasoning problems.
In classic literature, there are two main logical reasoning methods:
1. Forward Chaining (FC) ), that is, starting from facts and rules, iterating between making new inferences and adding them to the theory until the target statement can be proven or disproven;
2, backward Backward Chaining (BC) starts from the goal and recursively decomposes it into sub-goals until the sub-goals can be proven or overturned based on facts.
Previous methods of reasoning using language models mostly adopted the idea of forward chain reasoning, which required selecting a subset of facts and rules from the entire set. This is possible for LM is difficult because it requires combinatorial search in a large space.
In addition, deciding when to stop the search and declare the proof failed is also very difficult in FC, sometimes even requiring a module specifically trained on intermediate labels.
In fact, the classic automatic reasoning literature largely focuses on backward chain reasoning or goal-oriented verification strategies.
LAMBADA
LAMBADA means "Language model enhanced by reverse chain technology". The researchers conducted experiments It is proved that BC is more suitable for text-based deductive logical reasoning.
BC does not require a large number of combinatorial searches to select subsets, and has more natural halting criteria.
LAMBADA mainly focuses on automatic reasoning about facts, that is, natural language assertions, such as "Good people are red". These assertions are coherent but not necessarily based on the truth. .
A rule is written by a natural language statement, which can be rewritten in form as "if P then Q", for example "Rough, nice people are red" (Rough, nice people are red) can be Rewritten as "If a person is rough and nice, then they are red" (If a person is rough and nice, then they are red).
P is called the antecedent of the rule, and Q is called the consequent of the rule.
A theory theory C consists of facts F={f1, f2, . . , fn} and rules R={r1, r2, . . , rm}, G represents an idea The goal of proving or disproving based on facts and rules.
Example 1, a theoretical example with fictional characters and rules C
F={"Fiona is a good person","Fiona is a good person" Ona is rough"}
#R={"If someone is smart, then he is a good person", "A rough good person is red", "Being a good person and red means he is round" }.
Based on the above theory, one might want to prove or disprove a goal, such as "Fiona is red?".
Backward chaining reasoning
Whether a rule applies to a goal is determined through an operation called unification in logic.
For example, for the goal "Fiona is red?" in Example 1, the consequences of the second rule are the same as the goal, so it can be applied; but the consequences of the other two rules are different , so not applicable.
Considering the theory and goals in Example 1, BC starts reasoning from the goal "Fiona is red?"
First, BC verifies whether the goal can be proven or disproven from any facts. Since there are no facts to prove or disprove this goal, we next check whether this goal is consistent with the results of any rules, and it is found that it is consistent with the second rule "Rough and good people are red".
Therefore, this goal can be broken down into two sub-goals: 1) Is Fiona rough? and 2) Is Fiona a good person? .
Since both subgoals can be proven from the facts, BC concludes that the original goal can be proven.
For a goal, the result of BC is either proof, denial, or unknown (for example, the goal "Fiona is smart?").
Language Model in LAMBADA
In order to use BC for text-based reasoning, the researchers introduced four LM-based modules: Fact Check , Rule Selection, Goal Decomposition and Sign Agreement.
Fact check
Giving the theory Given a set of facts F and a goal G in , the goal is denied).
If such a fact cannot be found, then the truth about G remains unknown.
The implementation of fact checking includes two sub-modules: the first sub-module selects a fact from the set of facts most relevant to the target, and the second sub-module verifies whether the target can be based on this fact be proven or disproven.
Since the fact selection sub-module may not determine the best facts on the first try, if the truth of the target is still unknown after one round of calling the sub-module, the selected facts can be deleted fact, and then call the submodule again; this process can be repeated multiple times.
Rule selection
Given a set of rules R and a goal G in the theory, the rule selection module determines The rules r∈R make the result of r consistent with G, and then these rules are used to decompose the goal into sub-goals.
If such a rule cannot be determined, then the truth of G remains unknown.
Rule selection also includes two sub-modules: the first sub-module determines the result of each rule (independent of the goal), and the second sub-module takes the result and goal of the rule as input, and determine which one aligns with the goal.
It should be noted that due to the recursive nature of BC, the rule selection module may be called multiple times in the process of proving a goal. Since the result of identifying each rule is independent of the target, this submodule only needs to be called once.
Goal decomposition
Given a rule r and a goal G, make the result of r consistent with G, The goal decomposition module determines the subgoals that need to be proven so that G can be proven or disproven.
In the case of successfully proving the antecedent of r, whether the goal is proved or disproven depends on whether the sign of the goal is consistent with the sign of the result of r.
For example, for the goal "Fiona is red?", since the sign of the goal is consistent with the result sign of the second rule, and the antecedent of the rule is proven, it can be concluded that, Goal proven.
Symbolic consistency
Given a rule r and a goal G, the symbolic consistency module verifies the result of r Whether the symbol is consistent or inconsistent with the target's symbol.
Experimental part
The researchers chose Chain of Thought (CoT), the sota neural reasoning method based on explicit reasoning, and the sota module reasoning method Selection Inference (SI) as Compare to baseline model.
The experimental data sets use ProofWriter and PrOntoQA. These data sets are challenging for LM inference, contain examples where chain lengths up to 5 hops need to be proven, and targets cannot be derived from the provided theory. Examples that cannot be refuted.
Experimental results show that LAMBADA significantly outperforms the other two baselines, especially on the ProofWriter-PUD dataset containing UNKNOWN labels (44% relative improvement compared to CoT and 44% relative improvement compared to SI at depth -5 56% improvement), and at the higher depths of PrOntoQA (37% relative improvement compared to CoT and 113% improvement compared to SI at depth -5).
These results show the advantages of LAMBADA in logical reasoning and also show the backward chaining (in LAMBADA it is Inference backbone) may be a better choice than forward chaining (backbone in SI).
These results also reveal a flaw in the CoT method when dealing with UNKNOWN labels: unlike examples labeled PROVED or DISPROVED, for examples labeled UNKNOWN , there is no natural chain of thinking.
For the deeper (3) proof chain problem, SI produces predictions that are close to the majority class predictions on the three datasets.
It can be found that in the binary case, it tends to over-predict DISPROVED; in the ternary classification case, it tends to over-predict UNKNOWN, which makes it at the depth of PrOntoQA -5 The performance in is even worse than the majority class because there are more PROVED labels than DISPROVED at that depth.
However, the researchers were also surprised to find that CoT's performance on the ProofWriterPD data set was still relatively high, and the accuracy did not decrease.
In summary, LAMBADA has higher inference accuracy on these datasets and is more likely to produce valid conclusions than other techniques that use false proof traces to find correct conclusions. reasoning chain, and is also more query efficient than other LM-based modular reasoning methods.
The results of this experiment strongly suggest that future work on reasoning with LMs should include backward chaining or goal-directed strategies, the researchers said.
Reference:
https://arxiv.org/abs/2212.13894
The above is the detailed content of Don't think step by step! Google's latest natural language reasoning algorithm LAMBADA: 'Reverse chain reasoning” is the answer. For more information, please follow other related articles on the PHP Chinese website!

Hot AI Tools

Undresser.AI Undress
AI-powered app for creating realistic nude photos

AI Clothes Remover
Online AI tool for removing clothes from photos.

Undress AI Tool
Undress images for free

Clothoff.io
AI clothes remover

Video Face Swap
Swap faces in any video effortlessly with our completely free AI face swap tool!

Hot Article

Hot Tools

Notepad++7.3.1
Easy-to-use and free code editor

SublimeText3 Chinese version
Chinese version, very easy to use

Zend Studio 13.0.1
Powerful PHP integrated development environment

Dreamweaver CS6
Visual web development tools

SublimeText3 Mac version
God-level code editing software (SublimeText3)

Hot Topics


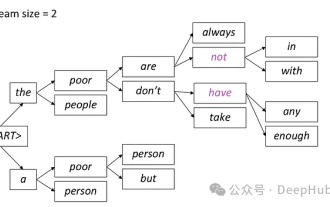
In natural language generation tasks, sampling method is a technique to obtain text output from a generative model. This article will discuss 5 common methods and implement them using PyTorch. 1. GreedyDecoding In greedy decoding, the generative model predicts the words of the output sequence based on the input sequence time step by time. At each time step, the model calculates the conditional probability distribution of each word, and then selects the word with the highest conditional probability as the output of the current time step. This word becomes the input to the next time step, and the generation process continues until some termination condition is met, such as a sequence of a specified length or a special end marker. The characteristic of GreedyDecoding is that each time the current conditional probability is the best
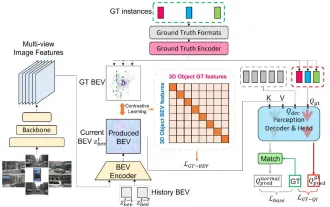
Written above & the author’s personal understanding: At present, in the entire autonomous driving system, the perception module plays a vital role. The autonomous vehicle driving on the road can only obtain accurate perception results through the perception module. The downstream regulation and control module in the autonomous driving system makes timely and correct judgments and behavioral decisions. Currently, cars with autonomous driving functions are usually equipped with a variety of data information sensors including surround-view camera sensors, lidar sensors, and millimeter-wave radar sensors to collect information in different modalities to achieve accurate perception tasks. The BEV perception algorithm based on pure vision is favored by the industry because of its low hardware cost and easy deployment, and its output results can be easily applied to various downstream tasks.
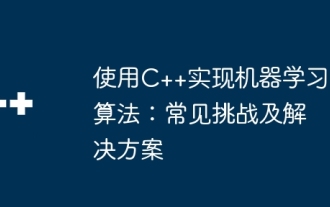
Common challenges faced by machine learning algorithms in C++ include memory management, multi-threading, performance optimization, and maintainability. Solutions include using smart pointers, modern threading libraries, SIMD instructions and third-party libraries, as well as following coding style guidelines and using automation tools. Practical cases show how to use the Eigen library to implement linear regression algorithms, effectively manage memory and use high-performance matrix operations.
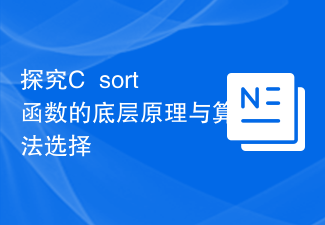
The bottom layer of the C++sort function uses merge sort, its complexity is O(nlogn), and provides different sorting algorithm choices, including quick sort, heap sort and stable sort.
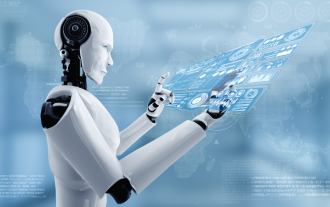
The convergence of artificial intelligence (AI) and law enforcement opens up new possibilities for crime prevention and detection. The predictive capabilities of artificial intelligence are widely used in systems such as CrimeGPT (Crime Prediction Technology) to predict criminal activities. This article explores the potential of artificial intelligence in crime prediction, its current applications, the challenges it faces, and the possible ethical implications of the technology. Artificial Intelligence and Crime Prediction: The Basics CrimeGPT uses machine learning algorithms to analyze large data sets, identifying patterns that can predict where and when crimes are likely to occur. These data sets include historical crime statistics, demographic information, economic indicators, weather patterns, and more. By identifying trends that human analysts might miss, artificial intelligence can empower law enforcement agencies

01 Outlook Summary Currently, it is difficult to achieve an appropriate balance between detection efficiency and detection results. We have developed an enhanced YOLOv5 algorithm for target detection in high-resolution optical remote sensing images, using multi-layer feature pyramids, multi-detection head strategies and hybrid attention modules to improve the effect of the target detection network in optical remote sensing images. According to the SIMD data set, the mAP of the new algorithm is 2.2% better than YOLOv5 and 8.48% better than YOLOX, achieving a better balance between detection results and speed. 02 Background & Motivation With the rapid development of remote sensing technology, high-resolution optical remote sensing images have been used to describe many objects on the earth’s surface, including aircraft, cars, buildings, etc. Object detection in the interpretation of remote sensing images
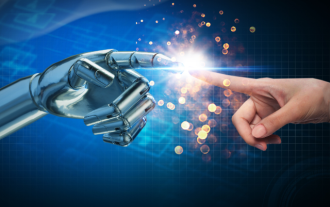
1. The historical development of multi-modal large models. The photo above is the first artificial intelligence workshop held at Dartmouth College in the United States in 1956. This conference is also considered to have kicked off the development of artificial intelligence. Participants Mainly the pioneers of symbolic logic (except for the neurobiologist Peter Milner in the middle of the front row). However, this symbolic logic theory could not be realized for a long time, and even ushered in the first AI winter in the 1980s and 1990s. It was not until the recent implementation of large language models that we discovered that neural networks really carry this logical thinking. The work of neurobiologist Peter Milner inspired the subsequent development of artificial neural networks, and it was for this reason that he was invited to participate in this project.
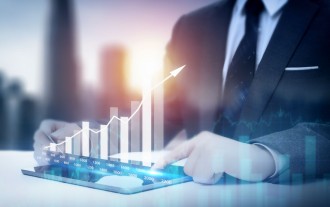
1. Background of the Construction of 58 Portraits Platform First of all, I would like to share with you the background of the construction of the 58 Portrait Platform. 1. The traditional thinking of the traditional profiling platform is no longer enough. Building a user profiling platform relies on data warehouse modeling capabilities to integrate data from multiple business lines to build accurate user portraits; it also requires data mining to understand user behavior, interests and needs, and provide algorithms. side capabilities; finally, it also needs to have data platform capabilities to efficiently store, query and share user profile data and provide profile services. The main difference between a self-built business profiling platform and a middle-office profiling platform is that the self-built profiling platform serves a single business line and can be customized on demand; the mid-office platform serves multiple business lines, has complex modeling, and provides more general capabilities. 2.58 User portraits of the background of Zhongtai portrait construction
