


Using small tricks to excavate the diffusion model, the generated images are almost replicas of the original training data, and privacy is about to be exposed.
Denoising diffusion models are an emerging class of generative neural networks that generate images from a training distribution through an iterative denoising process. This type of diffusion model produces higher quality samples and is easier to scale and control than previous methods such as GANs and VAEs. As a result, after rapid development, they can already produce high-resolution images, and there is great public interest in large models such as the DALL-E 2.
The beauty of generative diffusion models lies in their ability to synthesize new images that are, superficially, unlike anything in the training set. In fact, large-scale training efforts in the past have not found that overfitting will be a problem. Researchers in privacy-sensitive fields have even suggested that diffusion models can be used to protect privacy by generating synthetic examples to generate real images. This series of work is conducted under the assumption that the diffusion model does not memorize and regenerate training data. Doing so would violate all privacy guarantees and breed many problems with model generalization and digital forgery.
In this article, researchers from Google, DeepMind and other institutions have proven that the SOTA diffusion model can indeed remember and regenerate a single training example.
## Paper address: https://arxiv.org/pdf/2301.13188v1.pdf
First, the research proposes and implements a new definition of memory in image models. Then, the study designed a two-stage data extraction attack, using standard methods to generate images and label some images. The study applied the method to Stable Diffusion and Imagen, resulting in the extraction of more than 100 nearly identical copies of training images, which ranged from personally identifiable photos to trademarked logos (Figure 1).
In order to better understand how memory works and why, researchers trained hundreds of people on CIFAR10 Diffusion model to analyze the impact of model accuracy, hyperparameters, augmentation, and deduplication on privacy. Diffusion models are the least private form of image models evaluated in the study, leaking twice as much training data as GANs. Worse, research also finds that existing privacy-enhancing technologies fail to provide acceptable privacy-utility trade-offs. Overall, this paper highlights the tension that exists between increasingly powerful generative models and data privacy, and raises questions about how diffusion models work and how they can be deployed appropriately.
Why do you want to do this research?There are two motivations behind understanding how diffusion models remember and regenerate training data.
The first is to understand the privacy risks. Regenerating diffusion models that scrape data from the Internet may pose similar privacy and copyright risks as language models. For example, it has been pointed out that memorizing and regenerating copyrighted text and source code present potential indicators of infringement. Likewise, copying an image created by a professional artist is called digital forgery, and there is a debate in the art world.
The second is to understand generalization. In addition to data privacy, understanding how and why diffusion models remember training data helps understand their ability to generalize. For example, a common question with large-scale generative models is whether their impressive results result from true generation or are the result of directly copying and remixing the training data. By studying memory, it is possible to provide a concrete empirical description of the rate at which generative models perform such data replication.
Extract data from SOTA diffusion modelExtract data from Stable Diffusion
Now Extract training data from Stable Diffusion, the largest and most popular open source diffusion model.
This extraction applies the method of previous work to the image, including two steps:
1. Diffusion model using standard sampling method And generate multiple examples using the known prompts from the previous section.
2. Perform inference to separate the new generation model from the memorized training model.
To evaluate the effectiveness of the intrusion, the study selected 350,000 most repeated examples from the training dataset and generated 500 candidate images for each prompt (generating 175 million in total image).
First, the study sorts all these generated images to determine which ones are generated by memorizing the training data. Each of these generated images is then compared to the training images under Definition 1 in the paper, and each image is annotated as extracted or not extracted. The study found that 94 images were extracted. To ensure that these images did not just fit some arbitrary definition, the study also manually annotated the first 1,000 generated images through visual analysis, either with or without memory. And another 13 (total 109 images) were found to be almost copies of the training examples, even though they did not meet the study L_2 norm definition. Figure 3 shows a subset of the extracted images, which are reproduced with near-perfect pixel accuracy.
The experiment also gives the calculation curve given an annotated ordered image set, Evaluate the number of extracted images versus the false positive rate of the intrusion. The intrusion is exceptionally accurate: out of 175 million generated images, 50 memory images with 0 false positives could be identified, and all memory images could be extracted with over 50% accuracy. Figure 4 contains precision-recall curves for both definitions of memory.
Extract data from image
Although Stable Diffusion is currently the best choice among publicly available diffusion models, some non-public models have achieved stronger performance using larger models and datasets. Previous research has found that larger models are more likely to remember training data, so this study looked at Imagen, a 2 billion parameter text-to-image diffusion model.
Surprisingly, research has found that hacking non-distributed images in Imagen is more effective than in Stable Diffusion. On Imagen, the study tried to extract 500 images with the highest out-of-distribution (OOD) score. Imagen memorizes and copies 3 of the images (which are unique in the training data set). In contrast, when the study applied the same method to Stable Diffusion, it failed to identify any memories even after trying to extract the 10,000 most outlier samples. Therefore, Imagen is less private than Stable Diffusion on both copied and non-copied images. This may be due to the fact that Imagen uses a larger model than Stable Diffusion and therefore remembers more images. Additionally, Imagen trains with more iterations on smaller datasets, which can also help improve memory levels.
The above is the detailed content of Using small tricks to excavate the diffusion model, the generated images are almost replicas of the original training data, and privacy is about to be exposed.. For more information, please follow other related articles on the PHP Chinese website!

Hot AI Tools

Undresser.AI Undress
AI-powered app for creating realistic nude photos

AI Clothes Remover
Online AI tool for removing clothes from photos.

Undress AI Tool
Undress images for free

Clothoff.io
AI clothes remover

Video Face Swap
Swap faces in any video effortlessly with our completely free AI face swap tool!

Hot Article

Hot Tools

Notepad++7.3.1
Easy-to-use and free code editor

SublimeText3 Chinese version
Chinese version, very easy to use

Zend Studio 13.0.1
Powerful PHP integrated development environment

Dreamweaver CS6
Visual web development tools

SublimeText3 Mac version
God-level code editing software (SublimeText3)

Hot Topics


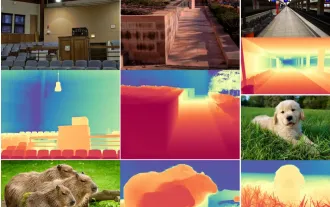
0.What does this article do? We propose DepthFM: a versatile and fast state-of-the-art generative monocular depth estimation model. In addition to traditional depth estimation tasks, DepthFM also demonstrates state-of-the-art capabilities in downstream tasks such as depth inpainting. DepthFM is efficient and can synthesize depth maps within a few inference steps. Let’s read about this work together ~ 1. Paper information title: DepthFM: FastMonocularDepthEstimationwithFlowMatching Author: MingGui, JohannesS.Fischer, UlrichPrestel, PingchuanMa, Dmytr
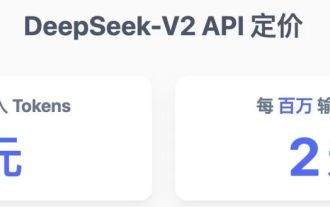
Imagine an artificial intelligence model that not only has the ability to surpass traditional computing, but also achieves more efficient performance at a lower cost. This is not science fiction, DeepSeek-V2[1], the world’s most powerful open source MoE model is here. DeepSeek-V2 is a powerful mixture of experts (MoE) language model with the characteristics of economical training and efficient inference. It consists of 236B parameters, 21B of which are used to activate each marker. Compared with DeepSeek67B, DeepSeek-V2 has stronger performance, while saving 42.5% of training costs, reducing KV cache by 93.3%, and increasing the maximum generation throughput to 5.76 times. DeepSeek is a company exploring general artificial intelligence
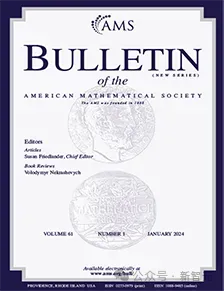
AI is indeed changing mathematics. Recently, Tao Zhexuan, who has been paying close attention to this issue, forwarded the latest issue of "Bulletin of the American Mathematical Society" (Bulletin of the American Mathematical Society). Focusing on the topic "Will machines change mathematics?", many mathematicians expressed their opinions. The whole process was full of sparks, hardcore and exciting. The author has a strong lineup, including Fields Medal winner Akshay Venkatesh, Chinese mathematician Zheng Lejun, NYU computer scientist Ernest Davis and many other well-known scholars in the industry. The world of AI has changed dramatically. You know, many of these articles were submitted a year ago.
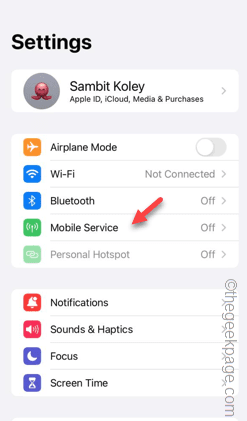
Facing lag, slow mobile data connection on iPhone? Typically, the strength of cellular internet on your phone depends on several factors such as region, cellular network type, roaming type, etc. There are some things you can do to get a faster, more reliable cellular Internet connection. Fix 1 – Force Restart iPhone Sometimes, force restarting your device just resets a lot of things, including the cellular connection. Step 1 – Just press the volume up key once and release. Next, press the Volume Down key and release it again. Step 2 – The next part of the process is to hold the button on the right side. Let the iPhone finish restarting. Enable cellular data and check network speed. Check again Fix 2 – Change data mode While 5G offers better network speeds, it works better when the signal is weaker
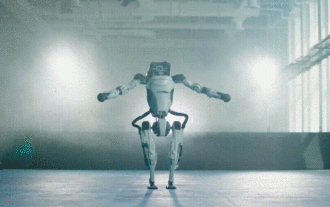
Boston Dynamics Atlas officially enters the era of electric robots! Yesterday, the hydraulic Atlas just "tearfully" withdrew from the stage of history. Today, Boston Dynamics announced that the electric Atlas is on the job. It seems that in the field of commercial humanoid robots, Boston Dynamics is determined to compete with Tesla. After the new video was released, it had already been viewed by more than one million people in just ten hours. The old people leave and new roles appear. This is a historical necessity. There is no doubt that this year is the explosive year of humanoid robots. Netizens commented: The advancement of robots has made this year's opening ceremony look like a human, and the degree of freedom is far greater than that of humans. But is this really not a horror movie? At the beginning of the video, Atlas is lying calmly on the ground, seemingly on his back. What follows is jaw-dropping
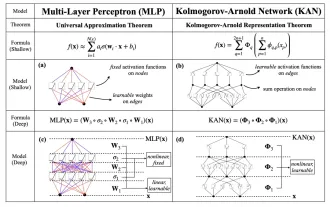
Earlier this month, researchers from MIT and other institutions proposed a very promising alternative to MLP - KAN. KAN outperforms MLP in terms of accuracy and interpretability. And it can outperform MLP running with a larger number of parameters with a very small number of parameters. For example, the authors stated that they used KAN to reproduce DeepMind's results with a smaller network and a higher degree of automation. Specifically, DeepMind's MLP has about 300,000 parameters, while KAN only has about 200 parameters. KAN has a strong mathematical foundation like MLP. MLP is based on the universal approximation theorem, while KAN is based on the Kolmogorov-Arnold representation theorem. As shown in the figure below, KAN has
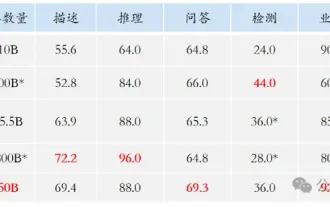
I cry to death. The world is madly building big models. The data on the Internet is not enough. It is not enough at all. The training model looks like "The Hunger Games", and AI researchers around the world are worrying about how to feed these data voracious eaters. This problem is particularly prominent in multi-modal tasks. At a time when nothing could be done, a start-up team from the Department of Renmin University of China used its own new model to become the first in China to make "model-generated data feed itself" a reality. Moreover, it is a two-pronged approach on the understanding side and the generation side. Both sides can generate high-quality, multi-modal new data and provide data feedback to the model itself. What is a model? Awaker 1.0, a large multi-modal model that just appeared on the Zhongguancun Forum. Who is the team? Sophon engine. Founded by Gao Yizhao, a doctoral student at Renmin University’s Hillhouse School of Artificial Intelligence.
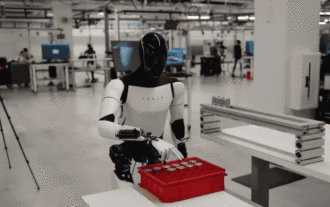
The latest video of Tesla's robot Optimus is released, and it can already work in the factory. At normal speed, it sorts batteries (Tesla's 4680 batteries) like this: The official also released what it looks like at 20x speed - on a small "workstation", picking and picking and picking: This time it is released One of the highlights of the video is that Optimus completes this work in the factory, completely autonomously, without human intervention throughout the process. And from the perspective of Optimus, it can also pick up and place the crooked battery, focusing on automatic error correction: Regarding Optimus's hand, NVIDIA scientist Jim Fan gave a high evaluation: Optimus's hand is the world's five-fingered robot. One of the most dexterous. Its hands are not only tactile
