


Deployment of domestic ChatGPT only requires 6G of video memory! The ChatYuan model is open for download: the industry's first functional dialogue open source Chinese large model
Some time ago, Yuanyu Intelligent Development Team trained a functional dialogue large model ChatYuan similar to ChatGPT, and opened a trial interface in the web version.
Now you can also deploy a ChatYuan on your own machine!
The model can be used in question and answer scenarios, and can conduct dialogues and various generation tasks based on context, including creative writing. It can also answer questions in fields such as law and COVID-19.
And supports zero-sample learning in all Chinese tasks. Users can use it by providing prompts. It supports nearly 30 kinds of Chinese tasks under the categories of text generation, information extraction and understanding. .
ChatYuan is further trained based on PromptCLUE-large combined with hundreds of millions of functional question and answer and multi-round dialogue data. The model parameters are 770 million, the video memory is about 6G, and a civilian graphics card can be loaded and used. , the model is currently open for download.
PromptCLUE is pre-trained on 100 billion token Chinese corpus, has learned a total of 1.5 trillion Chinese tokens, and conducted Prompt task-based training on hundreds of tasks.
For understanding tasks, such as classification, sentiment analysis, extraction, etc., the label system can be customized; for a variety of generation tasks, sampling can be freely generated.
How to use
1. Github
##Project address: https://github.com/clue-ai/ChatYuan
2. Huggingface
Project address: https://huggingface.co/ClueAI/ChatYuan- large-v1
#3. ModelScope
Load model:
##Project address: https://aistudio.baidu.com /aistudio/projectdetail/5404182
Model address: https://huggingface.co/ClueAI/ChatYuan-large-v1-paddle
Use since Have data to train the model
1. Organize the data
Organize the data into a unified format, which consists of two parts: input ( Input) and output (Output).
For a single round of question and answer or generation:
Input: task description input text
Output: The text that the system needs to predict, such as the content after "Xiaoyuan" in the figure below.
For multiple rounds of dialogue:
Input: Enter the text above the task description
Output: The text that the system needs to predict, such as the content after "Xiaoyuan" in the figure below.
Single round dialogue data format:
##Multiple rounds of dialogue data format:
##2. Training model
For training code, please refer to:
Use pCLUE data set for training, prediction and Effect verification, pytorch implementation - online colab.
Github project: https://colab.research.google.com/drive/1lEyFhEfoc-5Z5xqpEKkZt_iMaojH1MP_ ?usp=sharingFor example
==========Example 1======= ===
##User:What can you do
Xiao Yuan:
Hello! I am Yuanyu AI. I can answer your questions, write articles, assignments, translate, and I can also provide you with information on issues in the legal and other fields.
==========Example 2==========
User:
Write a poem about winter
Xiao Yuan:
The winter night is quiet and cold,
Clouds are floating in the sky,
On the frozen white snow,
Cold like snow.
Snowflakes melt into ice,
Like those snowflakes falling,
In the cold winter ,
Feel the joy of spring.
In the winter,
the wind and snow gradually subsided,
there was silence,
Bring happiness and warmth home.
==========Example 3==========
User:
During the internship in the preschool education major, there will be problems with students. Please propose improvement measures. 800 words
Xiao Yuan:
In the internship of preschool education majors, there will be some problems with students. , such as students' lack of professional knowledge, lack of cooperation awareness, lack of basic sense of responsibility, etc., we need to take measures to solve these problems.
First of all, during the internship process, we need to cultivate students' team awareness, strengthen cooperation between students and teachers, strengthen communication with parents, and improve their communication skills. In addition, we also need to strengthen students' independence, let students learn to think independently, learn to communicate with teachers and parents, and cultivate their independence.
In addition, in daily work, we must also provide students with appropriate opportunities and opportunities to have more contact with society and participate in more social activities, deepening their understanding of society and school. Understand and cultivate their initiative, positive and innovative spirit.
Finally, we also need to pay attention to the problems that students have during internships, especially students’ lack of cooperation, dependence, not knowing how to share, procrastination, etc. These students should cause us Pay attention to it and formulate reasonable improvement measures to improve these problems.
Technology and training process
ChatYuan is a model obtained by further training based on PromptCLUE. It removes text understanding and information extraction tasks, and strengthens the learning and training of question and answer, dialogue and various generative tasks; The addition of anti-interference data allows the model to ignore irrelevant context when necessary; the addition of learning from user feedback data enables the model to not only have certain general language understanding capabilities and specific task generation capabilities, but also better respond to user intentions. .
The learning process of PromptCLUE
- Three major unifications: unified model framework (text-to-text), unified task form (prompt), unified application method (zero-shot/few-shot) (T0)
- 大Scale pre-training: Based on the t5-large version, hundreds of G Chinese corpus were used, 1 million steps were trained, and 1.5 trillion Chinese word-level tokens were trained cumulatively
- Large-scale task data: used 16 task types, hundreds of tasks, accumulated task data of 100 million levels
- Hybrid pre-training: On the one hand, downstream tasks are used as pre-training corpus, and on the other hand, downstream tasks and pre-training corpus are trained together to reduce tasks Disaster forgetting and shortening the distance between pre-training and downstream tasks, better adapting to downstream tasks (ExT5)
- Hybrid sampling: For many tasks with greatly different amounts of data, use all training batches within each training batch Tasks are sampled according to proportion, smooth sampling is performed according to the data volume of the task, and at the same time, the upper limit of the task data volume sampling pool is limited. Smooth sampling can reduce the harm of biased task training, and training within each batch can reduce the negative transfer of training between heterogeneous tasks (T5)
- Phased training: On the one hand, it refers to the pre-training phase, Involves the phasing of training sequence length (128 and 512) to speed up pre-training (Bert); on the other hand, in the downstream training phasing, it involves changes in learning rate and sequence length and decreasing data volume restrictions on downstream tasks. Better adapt to different downstream tasks.
- Increase the training of language model: refer to t5.1.1, in addition to using the Span Corrpution construction method for unsupervised training, and also using the prefix LM method to train to enhance the ability to generate tasks (LM adapted)
- Increase the training of the encoder and decoder of the model: Construct Data_text and Data_target pre-training data corpora respectively based on the downstream task data, and add them to the pre-training to respectively enhance the model's encoder understanding ability and decoder generation ability (see UIE)
- Reconstruct the model Chinese dictionary: Use sentencepiece to learn and build a model dictionary on Qianyi token, which is more in line with Chinese language habits
Follow-up work
The current version can carry out question and answer, dialogue and various creative writing or text generation. Compared with the online version, its intention understanding and generation capabilities still have a lot of room for improvement in some situations; it also cannot achieve reasoning well. or complex tasks. The existing version will then be further improved based on feedback.
The above is the detailed content of Deployment of domestic ChatGPT only requires 6G of video memory! The ChatYuan model is open for download: the industry's first functional dialogue open source Chinese large model. For more information, please follow other related articles on the PHP Chinese website!

Hot AI Tools

Undresser.AI Undress
AI-powered app for creating realistic nude photos

AI Clothes Remover
Online AI tool for removing clothes from photos.

Undress AI Tool
Undress images for free

Clothoff.io
AI clothes remover

Video Face Swap
Swap faces in any video effortlessly with our completely free AI face swap tool!

Hot Article

Hot Tools

Notepad++7.3.1
Easy-to-use and free code editor

SublimeText3 Chinese version
Chinese version, very easy to use

Zend Studio 13.0.1
Powerful PHP integrated development environment

Dreamweaver CS6
Visual web development tools

SublimeText3 Mac version
God-level code editing software (SublimeText3)

Hot Topics




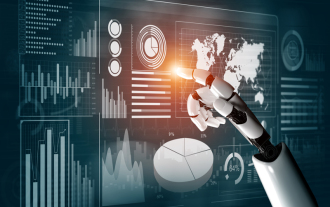
Text annotation is the work of corresponding labels or tags to specific content in text. Its main purpose is to provide additional information to the text for deeper analysis and processing, especially in the field of artificial intelligence. Text annotation is crucial for supervised machine learning tasks in artificial intelligence applications. It is used to train AI models to help more accurately understand natural language text information and improve the performance of tasks such as text classification, sentiment analysis, and language translation. Through text annotation, we can teach AI models to recognize entities in text, understand context, and make accurate predictions when new similar data appears. This article mainly recommends some better open source text annotation tools. 1.LabelStudiohttps://github.com/Hu
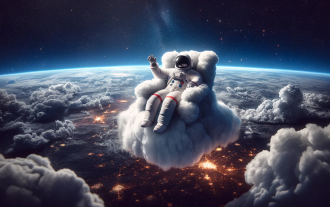
DALL-E 3 was officially introduced in September of 2023 as a vastly improved model than its predecessor. It is considered one of the best AI image generators to date, capable of creating images with intricate detail. However, at launch, it was exclus
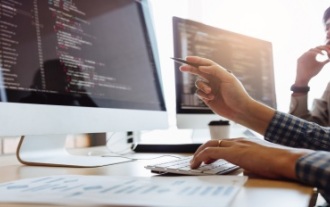
Image annotation is the process of associating labels or descriptive information with images to give deeper meaning and explanation to the image content. This process is critical to machine learning, which helps train vision models to more accurately identify individual elements in images. By adding annotations to images, the computer can understand the semantics and context behind the images, thereby improving the ability to understand and analyze the image content. Image annotation has a wide range of applications, covering many fields, such as computer vision, natural language processing, and graph vision models. It has a wide range of applications, such as assisting vehicles in identifying obstacles on the road, and helping in the detection and diagnosis of diseases through medical image recognition. . This article mainly recommends some better open source and free image annotation tools. 1.Makesens
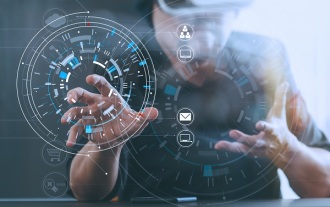
Face detection and recognition technology is already a relatively mature and widely used technology. Currently, the most widely used Internet application language is JS. Implementing face detection and recognition on the Web front-end has advantages and disadvantages compared to back-end face recognition. Advantages include reducing network interaction and real-time recognition, which greatly shortens user waiting time and improves user experience; disadvantages include: being limited by model size, the accuracy is also limited. How to use js to implement face detection on the web? In order to implement face recognition on the Web, you need to be familiar with related programming languages and technologies, such as JavaScript, HTML, CSS, WebRTC, etc. At the same time, you also need to master relevant computer vision and artificial intelligence technologies. It is worth noting that due to the design of the Web side
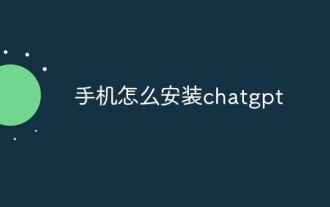
Installation steps: 1. Download the ChatGTP software from the ChatGTP official website or mobile store; 2. After opening it, in the settings interface, select the language as Chinese; 3. In the game interface, select human-machine game and set the Chinese spectrum; 4 . After starting, enter commands in the chat window to interact with the software.
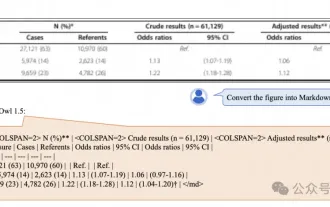
New SOTA for multimodal document understanding capabilities! Alibaba's mPLUG team released the latest open source work mPLUG-DocOwl1.5, which proposed a series of solutions to address the four major challenges of high-resolution image text recognition, general document structure understanding, instruction following, and introduction of external knowledge. Without further ado, let’s look at the effects first. One-click recognition and conversion of charts with complex structures into Markdown format: Charts of different styles are available: More detailed text recognition and positioning can also be easily handled: Detailed explanations of document understanding can also be given: You know, "Document Understanding" is currently An important scenario for the implementation of large language models. There are many products on the market to assist document reading. Some of them mainly use OCR systems for text recognition and cooperate with LLM for text processing.
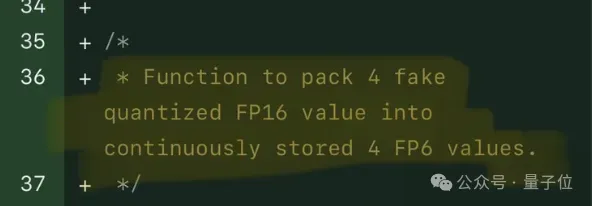
FP8 and lower floating point quantification precision are no longer the "patent" of H100! Lao Huang wanted everyone to use INT8/INT4, and the Microsoft DeepSpeed team started running FP6 on A100 without official support from NVIDIA. Test results show that the new method TC-FPx's FP6 quantization on A100 is close to or occasionally faster than INT4, and has higher accuracy than the latter. On top of this, there is also end-to-end large model support, which has been open sourced and integrated into deep learning inference frameworks such as DeepSpeed. This result also has an immediate effect on accelerating large models - under this framework, using a single card to run Llama, the throughput is 2.65 times higher than that of dual cards. one
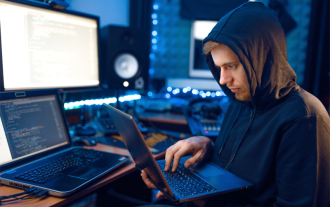
Let me introduce to you the latest AIGC open source project-AnimagineXL3.1. This project is the latest iteration of the anime-themed text-to-image model, aiming to provide users with a more optimized and powerful anime image generation experience. In AnimagineXL3.1, the development team focused on optimizing several key aspects to ensure that the model reaches new heights in performance and functionality. First, they expanded the training data to include not only game character data from previous versions, but also data from many other well-known anime series into the training set. This move enriches the model's knowledge base, allowing it to more fully understand various anime styles and characters. AnimagineXL3.1 introduces a new set of special tags and aesthetics
