


Berkeley open sourced the first high-definition data set and prediction model in parking scenarios, supporting target recognition and trajectory prediction.
As autonomous driving technology continues to iterate, vehicle behavior and trajectory prediction are of extremely important significance for efficient and safe driving. Although traditional trajectory prediction methods such as dynamic model deduction and accessibility analysis have the advantages of clear form and strong interpretability, their modeling capabilities for the interaction between the environment and objects are relatively limited in complex traffic environments. Therefore, in recent years, a large number of research and applications have been based on various deep learning methods (such as LSTM, CNN, Transformer, GNN, etc.), and various data sets such as BDD100K, nuScenes, Stanford Drone, ETH/UCY, INTERACTION, ApolloScape, etc. have also emerged. , which provides strong support for training and evaluating deep neural network models. Many SOTA models such as GroupNet, Trajectron, MultiPath, etc. have shown good performance.
The above models and data sets are concentrated in normal road driving scenarios, and make full use of infrastructure and features such as lane lines and traffic lights to assist in the prediction process; due to limitations of traffic regulations, The movement patterns of most vehicles are also relatively clear. However, in the "last mile" of autonomous driving - autonomous parking scenarios, we will face many new difficulties:
- Traffic rules in the parking lot The requirements for lane lines and lane lines are not strict, and vehicles often drive at will and "take shortcuts"
- In order to complete the parking task, vehicles need to complete more complex parking actions, including frequent reversing, Parking, steering, etc. When the driver is inexperienced, parking may become a long process
- There are many obstacles and clutter in the parking lot, and the distance between vehicles is close. If you are not careful, you may cause Collisions and scratches
-
Pedestrians often walk through the parking lot at will, and vehicles need more avoidance actions
In such a scenario, simply apply the existing It is difficult for the trajectory prediction model to achieve ideal results, and the retraining model lacks the support of corresponding data. Current parking scene-based data sets such as CNRPark EXT and CARPK are only designed for free parking space detection. The pictures come from the first-person perspective of surveillance cameras, have low sampling rates, and have many occlusions, making them unable to be used for trajectory prediction.
In the just concluded 25th IEEE International Conference on Intelligent Transportation Systems (IEEE ITSC 2022) in October 2022, from University of California, Berkeley Researchers released the first high-definition video & trajectory data set for parking scenes, and based on this data set, used CNN and Transformer architecture to propose a trajectory prediction model called "ParkPredict" .
- Paper link: https://arxiv.org/abs/2204.10777
- Dataset home page, trial and download application: https://sites.google.com/berkeley.edu/dlp-dataset (If you cannot access, you can Try the alternative page https://www.php.cn/link/966eaa9527eb956f0dc8788132986707 )
- ##Dataset Python API: https://github.com/MPC- Berkeley/dlp-dataset
The data set was collected by drone, with a total duration of 3.5 hours, video resolution For 4K, the sampling rate is 25Hz. The view covers a car park area of approximately 140m x 80m, with a total of approximately 400 parking spaces. The dataset is accurately annotated, and a total of 1216 motor vehicles, 3904 bicycles, and 3904 pedestrian trajectories were collected.
After reprocessing, the trajectory data can be read in the form of JSON and loaded into the data structure of the connection graph (Graph):
- Individual (Agent): Each individual (Agent) is an object moving in the current scene (Scene). It has attributes such as geometric shape and type. Its movement trajectory is stored as a file containing Linked List of Instances
- Instance: Each instance is the state of an individual (Agent) in a frame (Frame). Contains its position, angle, speed and acceleration. Each instance contains a pointer to the instance of the individual in the previous frame and the next frame
- Frame (Frame): Each frame (Frame) is a sampling point, and its Contains all visible instances (Instance) at the current time, and pointers to the previous frame and the next frame
- Obstacle (Obstacle): The obstacle is in this record Objects that do not move at all, including the position, corner and geometric size of each object
- Scene (Scene): Each scene (Scene) corresponds to a recorded video file, which contains pointers , pointing to the first and last frames of the recording, all individuals (Agents) and all obstacles (Obstacles)
provided by the data set Two download formats:
JSON only (recommended) : JSON file contains the types, shapes of all individuals , trajectories and other information can be directly read, previewed, and generated semantic images (Semantic Images) through the open source Python API. If the research goal is only trajectory and behavior prediction, the JSON format can meet all needs.
##Original video and annotation: If the research is based on the original camera For topics in the field of machine vision such as target detection, separation, and tracking of raw images, you may need to download the original video and annotation. If this is required, the research needs need to be clearly described in the dataset application. In addition, the annotation file needs to be parsed by itself.
Behavior and trajectory prediction model: ParkPredictAs an application example, in the paper "ParkPredict: Multimodal Intent and Motion Prediction for Vehicles in Parking Lots with CNN" at IEEE ITSC 2022 and Transformer", the research team used this data set to predict the vehicle's intent (Intent) and trajectory (Trajectory) in the parking lot scene based on the CNN and Transformer architecture.
The team used the CNN model to predict the distribution probability of vehicle intent (Intent) by constructing semantic images. This model only needs to construct local environmental information of the vehicle, and can continuously change the number of available intentions according to the current environment.
The team improved the Transformer model and provided the intent (Intent) prediction results, the vehicle's movement history, and the semantic map of the surrounding environment as inputs to achieve Multi-modal intention and behavior prediction.
Summary
- As the first high-precision data set for parking scenarios, the Dragon Lake Parking (DLP) data set can achieve large-scale target recognition and tracking, idle Parking space detection, vehicle and pedestrian behavior and trajectory prediction, imitation learning and other research provide data and API support
- By using CNN and Transformer architecture, the ParkPredict model’s behavior and performance in parking scenarios In addition to showing good capabilities in trajectory prediction
- Dragon Lake Parking (DLP) data set is open for trial and application. You can visit the data set homepage https://sites.google.com/ berkeley.edu/dlp-dataset for more information (if you cannot access, you can try the alternative page https://www.php.cn/link/966eaa9527eb956f0dc8788132986707)
The above is the detailed content of Berkeley open sourced the first high-definition data set and prediction model in parking scenarios, supporting target recognition and trajectory prediction.. For more information, please follow other related articles on the PHP Chinese website!

Hot AI Tools

Undresser.AI Undress
AI-powered app for creating realistic nude photos

AI Clothes Remover
Online AI tool for removing clothes from photos.

Undress AI Tool
Undress images for free

Clothoff.io
AI clothes remover

Video Face Swap
Swap faces in any video effortlessly with our completely free AI face swap tool!

Hot Article

Hot Tools

Notepad++7.3.1
Easy-to-use and free code editor

SublimeText3 Chinese version
Chinese version, very easy to use

Zend Studio 13.0.1
Powerful PHP integrated development environment

Dreamweaver CS6
Visual web development tools

SublimeText3 Mac version
God-level code editing software (SublimeText3)

Hot Topics


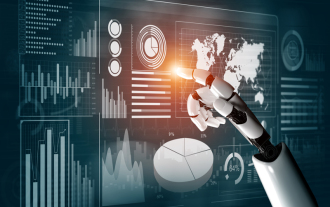
Text annotation is the work of corresponding labels or tags to specific content in text. Its main purpose is to provide additional information to the text for deeper analysis and processing, especially in the field of artificial intelligence. Text annotation is crucial for supervised machine learning tasks in artificial intelligence applications. It is used to train AI models to help more accurately understand natural language text information and improve the performance of tasks such as text classification, sentiment analysis, and language translation. Through text annotation, we can teach AI models to recognize entities in text, understand context, and make accurate predictions when new similar data appears. This article mainly recommends some better open source text annotation tools. 1.LabelStudiohttps://github.com/Hu
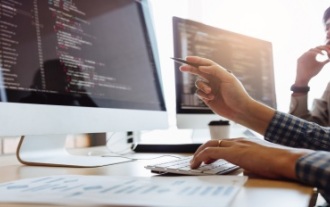
Image annotation is the process of associating labels or descriptive information with images to give deeper meaning and explanation to the image content. This process is critical to machine learning, which helps train vision models to more accurately identify individual elements in images. By adding annotations to images, the computer can understand the semantics and context behind the images, thereby improving the ability to understand and analyze the image content. Image annotation has a wide range of applications, covering many fields, such as computer vision, natural language processing, and graph vision models. It has a wide range of applications, such as assisting vehicles in identifying obstacles on the road, and helping in the detection and diagnosis of diseases through medical image recognition. . This article mainly recommends some better open source and free image annotation tools. 1.Makesens
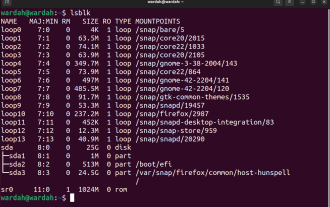
DDREASE is a tool for recovering data from file or block devices such as hard drives, SSDs, RAM disks, CDs, DVDs and USB storage devices. It copies data from one block device to another, leaving corrupted data blocks behind and moving only good data blocks. ddreasue is a powerful recovery tool that is fully automated as it does not require any interference during recovery operations. Additionally, thanks to the ddasue map file, it can be stopped and resumed at any time. Other key features of DDREASE are as follows: It does not overwrite recovered data but fills the gaps in case of iterative recovery. However, it can be truncated if the tool is instructed to do so explicitly. Recover data from multiple files or blocks to a single
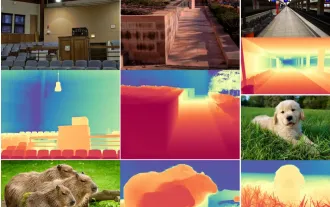
0.What does this article do? We propose DepthFM: a versatile and fast state-of-the-art generative monocular depth estimation model. In addition to traditional depth estimation tasks, DepthFM also demonstrates state-of-the-art capabilities in downstream tasks such as depth inpainting. DepthFM is efficient and can synthesize depth maps within a few inference steps. Let’s read about this work together ~ 1. Paper information title: DepthFM: FastMonocularDepthEstimationwithFlowMatching Author: MingGui, JohannesS.Fischer, UlrichPrestel, PingchuanMa, Dmytr
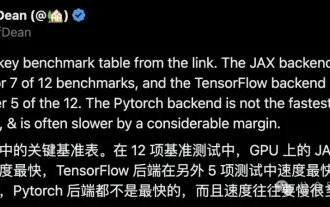
The performance of JAX, promoted by Google, has surpassed that of Pytorch and TensorFlow in recent benchmark tests, ranking first in 7 indicators. And the test was not done on the TPU with the best JAX performance. Although among developers, Pytorch is still more popular than Tensorflow. But in the future, perhaps more large models will be trained and run based on the JAX platform. Models Recently, the Keras team benchmarked three backends (TensorFlow, JAX, PyTorch) with the native PyTorch implementation and Keras2 with TensorFlow. First, they select a set of mainstream
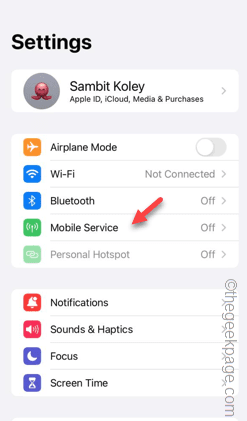
Facing lag, slow mobile data connection on iPhone? Typically, the strength of cellular internet on your phone depends on several factors such as region, cellular network type, roaming type, etc. There are some things you can do to get a faster, more reliable cellular Internet connection. Fix 1 – Force Restart iPhone Sometimes, force restarting your device just resets a lot of things, including the cellular connection. Step 1 – Just press the volume up key once and release. Next, press the Volume Down key and release it again. Step 2 – The next part of the process is to hold the button on the right side. Let the iPhone finish restarting. Enable cellular data and check network speed. Check again Fix 2 – Change data mode While 5G offers better network speeds, it works better when the signal is weaker
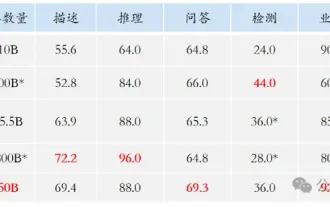
I cry to death. The world is madly building big models. The data on the Internet is not enough. It is not enough at all. The training model looks like "The Hunger Games", and AI researchers around the world are worrying about how to feed these data voracious eaters. This problem is particularly prominent in multi-modal tasks. At a time when nothing could be done, a start-up team from the Department of Renmin University of China used its own new model to become the first in China to make "model-generated data feed itself" a reality. Moreover, it is a two-pronged approach on the understanding side and the generation side. Both sides can generate high-quality, multi-modal new data and provide data feedback to the model itself. What is a model? Awaker 1.0, a large multi-modal model that just appeared on the Zhongguancun Forum. Who is the team? Sophon engine. Founded by Gao Yizhao, a doctoral student at Renmin University’s Hillhouse School of Artificial Intelligence.
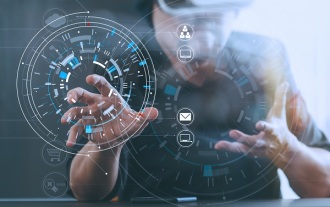
Face detection and recognition technology is already a relatively mature and widely used technology. Currently, the most widely used Internet application language is JS. Implementing face detection and recognition on the Web front-end has advantages and disadvantages compared to back-end face recognition. Advantages include reducing network interaction and real-time recognition, which greatly shortens user waiting time and improves user experience; disadvantages include: being limited by model size, the accuracy is also limited. How to use js to implement face detection on the web? In order to implement face recognition on the Web, you need to be familiar with related programming languages and technologies, such as JavaScript, HTML, CSS, WebRTC, etc. At the same time, you also need to master relevant computer vision and artificial intelligence technologies. It is worth noting that due to the design of the Web side
