Like TensorFlow, will NVIDIA's CUDA monopoly be broken?
In the past decade, the landscape of machine learning software development has undergone significant changes. Many frameworks have sprung up, but most rely heavily on NVIDIA's CUDA and get the best performance on NVIDIA's GPUs. However, with the arrival of PyTorch 2.0 and OpenAI Triton, Nvidia’s dominance in this field is being broken.
Google had great advantages in machine learning model architecture, training, and model optimization in the early days, but now it is difficult to fully utilize these advantages. On the hardware side, it will be difficult for other AI hardware companies to weaken Nvidia's dominance. Until PyTorch 2.0 and OpenAI Triton emerge, the default software stack for machine learning models will no longer be Nvidia’s closed-source CUDA.
TensorFlow vs. PyTorch
A similar competition occurs in machine learning frameworks. A few years ago, the framework ecosystem was quite fragmented, but TensorFlow was the front-runner. On the surface, Google seems to be firmly in the machine learning framework industry. They designed the AI application-specific accelerator TPU with TensorFlow, thus gaining a first-mover advantage.
- Computation (FLOPS): run dense matrix multiplication within each layer;
- memory bandwidth.
##In 2018, the most advanced model was BERT, and NVIDIA V100 was the most advanced GPU. At that time, matrix multiplication had already is no longer the main factor in improving model performance. Afterwards, models grew by 3 to 4 orders of magnitude in number of parameters, while the fastest GPUs grew by 1 order of magnitude in FLOPS.
Even in 2018, pure compute-bound workloads accounted for 99.8% of FLOPS but only 61% of runtime. Compared to matrix multiplication, normalization and pointwise ops use only 1/250 and 1/700 of the FLOPS of matrix multiplication, but they consume nearly 40% of the model run time.
Increasing the GPU's FLOPS will not help if all the time is spent on memory transfers (i.e., being memory bandwidth limited). On the other hand, if all your time is spent executing large matmuls, then even rewriting the model logic into C to reduce overhead will not help.
The reason why PyTorch can outperform TensorFlow is because Eager mode improves flexibility and usability, but moving to Eager mode is not the only benefit. When running in eager mode, each operation is read from memory, calculated, and then sent to memory before processing the next operation. Without extensive optimization, this can significantly increase memory bandwidth requirements.
So for models executed in Eager mode, one of the main optimization methods is operator fusion. Fusion operations compute multiple functions in a single pass to minimize memory reads/writes, rather than writing each intermediate result to memory. Operator fusion improves operator scheduling, memory bandwidth, and memory size costs.
This kind of optimization usually involves writing a custom CUDA kernel, but this is better than using a simple Python scripts are much harder. Over time, more and more operators have been steadily implemented in PyTorch, many of which simply combine multiple common operations into a more complex function.
The addition of operators makes it easier to create models in PyTorch, and Eager mode performs faster due to fewer memory reads/writes. The downside is that PyTorch has exploded to over 2000 operators within a few years.
We can say that software developers are too lazy, but to be honest, who has not been lazy. Once they get used to a new operator in PyTorch, they keep using it. The developer may not even realize that performance is improving but continue to use the operator because it eliminates the need to write more code.
In addition, not all operators can be fused. Deciding which operations to combine and which to allocate to specific computing resources at the chip and cluster levels takes a lot of time. Although the strategies for where operators are fused are generally similar, they can vary greatly due to different architectures.
NVIDIA WAS THE KING
The growth and default position of operators is an advantage for NVIDIA because each operator targets Its architecture is optimized for speed, but is not optimized for any other hardware. If an AI hardware startup wanted to fully implement PyTorch, that would mean supporting a growing list of 2,000 operators with high performance.
Because extracting maximum performance requires so much skill, training large models with high FLOPS utilization on GPUs requires an increasingly high level of talent. Eager mode execution of additive operator fusion means that the software, techniques and models developed are constantly being pushed to accommodate the compute and memory ratios that current generation GPUs have.
Everyone developing a machine learning chip is constrained by the same memory wall. ASICs are limited by supporting the most commonly used frameworks, by default development methods, GPU-optimized PyTorch code, and a mix of NVIDIA and external libraries. In this case, it makes little sense to have an architecture that eschews the various non-computational baggage of the GPU in favor of more FLOPS and a stricter programming model.
However, ease of use comes first. The only way to break the vicious cycle is to make the software that runs models on Nvidia’s GPUs as easy and seamlessly transferable to other hardware as possible. As model architectures stabilize and abstractions from PyTorch 2.0, OpenAI Triton, and MLOps companies like MosaicML become the default, the architecture and economics of chip solutions begin to be the biggest drivers of purchase, rather than the ease of use provided by Nvidia's advanced software sex.
PyTorch 2.0
A few months ago, the PyTorch Foundation was established and separated from Meta. In addition to changes to the open development and governance model, 2.0 was released in early beta and became generally available in March. PyTorch 2.0 brings many changes, but the main difference is that it adds a compilation solution that supports a graphical execution model. This shift will make it easier to properly utilize various hardware resources.
PyTorch 2.0 improves training performance by 86% on NVIDIA A100 and inference performance on CPU by 26%. This significantly reduces the computational time and cost required to train the model. These benefits extend to other GPUs and accelerators from AMD, Intel, Tenstorrent, Luminous Computing, Tesla, Google, Amazon, Microsoft, Marvell, Meta, Graphcore, Cerebras, SambaNova, and more.
For currently unoptimized hardware, PyTorch 2.0 has greater room for performance improvement. Meta and other companies are making such huge contributions to PyTorch because they want to achieve higher FLOPS utilization with less effort on their multi-billion dollar GPU training clusters. This way they also have an incentive to make their software stacks more portable to other hardware, introducing competition into the machine learning space.
With the help of better APIs, PyTorch 2.0 can also support data parallelism, sharding, pipeline parallelism and tensor parallelism, bringing progress to distributed training. Additionally, it supports dynamic shapes natively across the stack, which among many other examples makes it easier to support different sequence lengths for LLMs. The picture below is the first time that a major compiler supports Dynamic Shapes from training to inference:
PrimTorch
Writing a high-performance backend for PyTorch that fully supports all 2000+ operators is no easy task for every machine learning ASIC except NVIDIA GPUs. PrimTorch reduces the number of operators to approximately 250 original operators while maintaining the same usability for PyTorch end users. PrimTorch makes implementation of different non-NVIDIA backends of PyTorch simpler and more accessible. Custom hardware and systems vendors can more easily roll out their software stacks.
TorchDynamo
Turning to graph patterns requires a reliable graph definition. Meta and PyTorch have been trying to make this shift for about 5 years, but every solution they came up with had significant shortcomings. Finally, they solved the problem using TorchDynamo. TorchDynamo will ingest any PyTorch user script, including scripts that call external third-party libraries, and generate FX graphs.
Dynamo reduces all complex operators to approximately 250 primitive operators in PrimTorch. Once the graph is formed, unused operators are discarded and the graph determines which intermediate operators need to be stored or written to memory, and which ones may be fused. This greatly reduces overhead within the model while being "seamless" to the user.
Of the 7000 PyTorch models tested, TorchDynamo has been applied to more than 99% of the models, including models from OpenAI, HuggingFace, Meta, NVIDIA, Stability.AI, etc., without the need for Make any changes to the original code. The 7000 models tested were randomly selected from the most popular projects using PyTorch on GitHub.
Google's TensorFlow/Jax and other graph mode execution pipelines often require users to ensure that their models fit the compiler architecture, So that the picture can be captured. Dynamo changes this by enabling partial graph capture, protected graph capture, and instant recapture.
Partial graph capture allows models to contain unsupported/non-python constructs. When a graph cannot be generated for a model part, a graph break will be inserted and unsupported construction will be performed in eager mode between part graphs.
Protected graph capture checks whether the captured graph is valid for execution. "Protection" means a change that requires recompilation. This is important because running the same code multiple times will not recompile multiple times. On-the-fly recapture allows the graph to be re-captured if the captured graph is not valid for execution.
The goal of PyTorch is to create a unified front-end with a smooth UX that leverages Dynamo Generate graph. The user experience of the solution does not change, but performance can be significantly improved. Capture graphs can be executed more efficiently in parallel on large amounts of computing resources.
Dynamo and AOT Autograd then pass the optimized FX graph to the PyTorch native compiler level TorchInductor. Hardware companies can also feed this graph into their own backend compilers.
TorchInductor
TorchInductor is a Python native deep learning compiler that can generate fast code for multiple accelerators and backends. Inductor will take FX graphs with about 250 operators and reduce them to about 50 operators. Next, the Inductor enters the scheduling phase, where operators are fused and memory planning is determined.
The Inductor then enters "Wrapper Codegen," which generates code that runs on a CPU, GPU, or other AI accelerator. The wrapper Codegen replaces the interpreter part of the compiler stack and can call the kernel and allocate memory. The backend code generation part leverages OpenAI Triton for GPUs and outputs PTX code. For CPUs, the Intel compiler generates C (also works on non-Intel CPUs).
They will support more hardware in the future, but the point is that Inductor greatly reduces the amount of work that compiler teams have to do when making compilers for their AI hardware accelerators. In addition, the code is more optimized for performance, and memory bandwidth and capacity requirements are significantly reduced.
What researchers need is not just a compiler that only supports GPUs, but also a compiler that supports various hardware backends.
OpenAI Triton
OpenAI Triton is a disruptive presence for Nvidia’s closed-source machine learning software. Triton takes data directly from Python or through the PyTorch Inductor stack, the latter being the most common usage. Triton is responsible for converting the input into an LLVM intermediate representation and generating code. NVIDIA GPUs will generate PTX code directly, skipping NVIDIA's closed-source CUDA libraries (such as cuBLAS) and instead using open-source libraries (such as cutlass).
CUDA is popular in the world of accelerated computing, but little known among machine learning researchers and data scientists. Using CUDA can present challenges and require a deep understanding of the hardware architecture, which can slow down the development process. As a result, machine learning experts may rely on CUDA experts to modify, optimize, and parallelize their code.
Triton makes up for this shortcoming, allowing high-level languages to achieve comparable performance to low-level languages. The Triton kernel itself is very clear to the typical ML researcher, which is very important for usability. Triton automates memory coalescing, shared memory management, and scheduling in SM. Triton is not particularly useful for element-wise matrix multiplication, but matrix multiplication can already be done very efficiently. Triton is useful for expensive point-by-point operations and reducing the overhead of complex operations.
OpenAI Triton currently only officially supports NVIDIA GPUs, but this will change in the near future to support multiple other hardware vendors. Other hardware accelerators can be integrated directly into Triton’s LLVM IR, which greatly reduces the time to build an AI compiler stack for new hardware.
Nvidia’s huge software system lacks foresight and cannot take advantage of its huge advantages in ML hardware and software, and it has failed to become the default compiler for machine learning. They lack the focus on usability that allows OpenAI and Meta to create software stacks that are portable to other hardware.
Original link: https://www.semianalysis.com/p/nvidiaopenaitritonpytorch
The above is the detailed content of Like TensorFlow, will NVIDIA's CUDA monopoly be broken?. For more information, please follow other related articles on the PHP Chinese website!

Hot AI Tools

Undresser.AI Undress
AI-powered app for creating realistic nude photos

AI Clothes Remover
Online AI tool for removing clothes from photos.

Undress AI Tool
Undress images for free

Clothoff.io
AI clothes remover

AI Hentai Generator
Generate AI Hentai for free.

Hot Article

Hot Tools

Notepad++7.3.1
Easy-to-use and free code editor

SublimeText3 Chinese version
Chinese version, very easy to use

Zend Studio 13.0.1
Powerful PHP integrated development environment

Dreamweaver CS6
Visual web development tools

SublimeText3 Mac version
God-level code editing software (SublimeText3)

Hot Topics
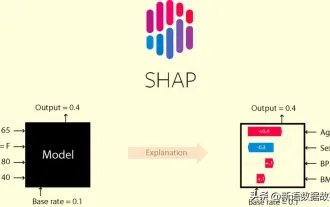
In the fields of machine learning and data science, model interpretability has always been a focus of researchers and practitioners. With the widespread application of complex models such as deep learning and ensemble methods, understanding the model's decision-making process has become particularly important. Explainable AI|XAI helps build trust and confidence in machine learning models by increasing the transparency of the model. Improving model transparency can be achieved through methods such as the widespread use of multiple complex models, as well as the decision-making processes used to explain the models. These methods include feature importance analysis, model prediction interval estimation, local interpretability algorithms, etc. Feature importance analysis can explain the decision-making process of a model by evaluating the degree of influence of the model on the input features. Model prediction interval estimate
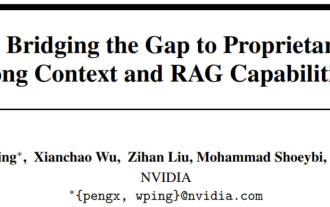
The open LLM community is an era when a hundred flowers bloom and compete. You can see Llama-3-70B-Instruct, QWen2-72B-Instruct, Nemotron-4-340B-Instruct, Mixtral-8x22BInstruct-v0.1 and many other excellent performers. Model. However, compared with proprietary large models represented by GPT-4-Turbo, open models still have significant gaps in many fields. In addition to general models, some open models that specialize in key areas have been developed, such as DeepSeek-Coder-V2 for programming and mathematics, and InternVL for visual-language tasks.
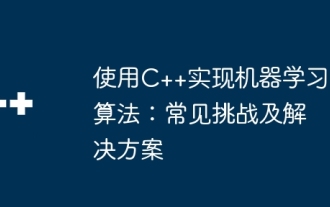
Common challenges faced by machine learning algorithms in C++ include memory management, multi-threading, performance optimization, and maintainability. Solutions include using smart pointers, modern threading libraries, SIMD instructions and third-party libraries, as well as following coding style guidelines and using automation tools. Practical cases show how to use the Eigen library to implement linear regression algorithms, effectively manage memory and use high-performance matrix operations.
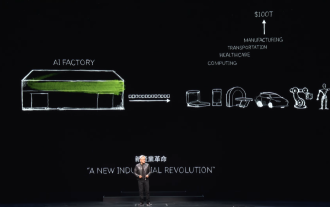
According to news from this site on June 2, at the ongoing Huang Renxun 2024 Taipei Computex keynote speech, Huang Renxun introduced that generative artificial intelligence will promote the reshaping of the full stack of software and demonstrated its NIM (Nvidia Inference Microservices) cloud-native microservices. Nvidia believes that the "AI factory" will set off a new industrial revolution: taking the software industry pioneered by Microsoft as an example, Huang Renxun believes that generative artificial intelligence will promote its full-stack reshaping. To facilitate the deployment of AI services by enterprises of all sizes, NVIDIA launched NIM (Nvidia Inference Microservices) cloud-native microservices in March this year. NIM+ is a suite of cloud-native microservices optimized to reduce time to market
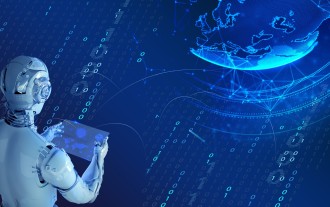
Translator | Reviewed by Li Rui | Chonglou Artificial intelligence (AI) and machine learning (ML) models are becoming increasingly complex today, and the output produced by these models is a black box – unable to be explained to stakeholders. Explainable AI (XAI) aims to solve this problem by enabling stakeholders to understand how these models work, ensuring they understand how these models actually make decisions, and ensuring transparency in AI systems, Trust and accountability to address this issue. This article explores various explainable artificial intelligence (XAI) techniques to illustrate their underlying principles. Several reasons why explainable AI is crucial Trust and transparency: For AI systems to be widely accepted and trusted, users need to understand how decisions are made
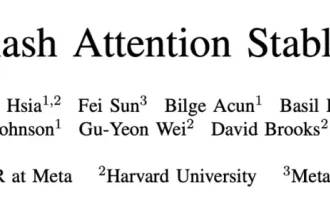
MetaFAIR teamed up with Harvard to provide a new research framework for optimizing the data bias generated when large-scale machine learning is performed. It is known that the training of large language models often takes months and uses hundreds or even thousands of GPUs. Taking the LLaMA270B model as an example, its training requires a total of 1,720,320 GPU hours. Training large models presents unique systemic challenges due to the scale and complexity of these workloads. Recently, many institutions have reported instability in the training process when training SOTA generative AI models. They usually appear in the form of loss spikes. For example, Google's PaLM model experienced up to 20 loss spikes during the training process. Numerical bias is the root cause of this training inaccuracy,
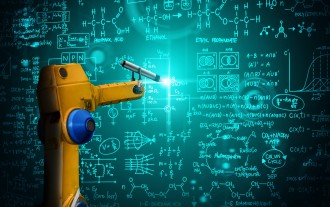
Machine learning is an important branch of artificial intelligence that gives computers the ability to learn from data and improve their capabilities without being explicitly programmed. Machine learning has a wide range of applications in various fields, from image recognition and natural language processing to recommendation systems and fraud detection, and it is changing the way we live. There are many different methods and theories in the field of machine learning, among which the five most influential methods are called the "Five Schools of Machine Learning". The five major schools are the symbolic school, the connectionist school, the evolutionary school, the Bayesian school and the analogy school. 1. Symbolism, also known as symbolism, emphasizes the use of symbols for logical reasoning and expression of knowledge. This school of thought believes that learning is a process of reverse deduction, through existing
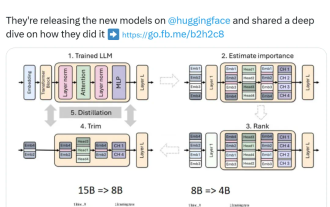
The rise of small models. Last month, Meta released the Llama3.1 series of models, which includes Meta’s largest model to date, the 405B model, and two smaller models with 70 billion and 8 billion parameters respectively. Llama3.1 is considered to usher in a new era of open source. However, although the new generation models are powerful in performance, they still require a large amount of computing resources when deployed. Therefore, another trend has emerged in the industry, which is to develop small language models (SLM) that perform well enough in many language tasks and are also very cheap to deploy. Recently, NVIDIA research has shown that structured weight pruning combined with knowledge distillation can gradually obtain smaller language models from an initially larger model. Turing Award Winner, Meta Chief A
