


USB: The first semi-supervised classification learning benchmark that unifies visual, language and audio classification tasks
Currently, the development of semi-supervised learning is in full swing. However, existing semi-supervised learning benchmarks are mostly limited to computer vision classification tasks, excluding consistent and diverse evaluation of classification tasks such as natural language processing and audio processing. In addition, most semi-supervised papers are published by large institutions, and it is often difficult for academic laboratories to participate in advancing the field due to limitations in computing resources.
To this end, researchers from Microsoft Research Asia and researchers from Westlake University, Tokyo Institute of Technology, Carnegie Mellon University, Max Planck Institute and other institutions proposed Unified SSL Benchmark (USB): the first semi-supervised classification learning benchmark that unifies visual, language and audio classification tasks.
This paper not only introduces more diverse application fields, but also uses a visual pre-training model for the first time to greatly reduce the verification time of semi-supervised algorithms, making semi-supervised research more convenient for researchers. Especially small research groups are more friendly. Relevant papers have been accepted by NeurIPS 2022, the top international academic conference in the field of artificial intelligence.
## Article link: https://arxiv.org/pdf/2208.07204.pdf
Code link: https://github.com/microsoft/Semi-supervised-learning
Supervised learning By building models to fit labeled data, neural network models produce competitive results when trained on large amounts of high-quality labeled data using supervised learning.
For example, according to statistics from the Paperswithcode website, on the million-level data set of ImageNet, traditional supervised learning methods can achieve an accuracy of more than 88%. However, obtaining large amounts of labeled data is often time-consuming and laborious.
In order to alleviate the dependence on labeled data, semi-supervised learning (SSL) is committed to utilizing a large amount of unlabeled data when there is only a small amount of labeled data. to improve the generalization of the model. Semi-supervised learning is also one of the important topics of machine learning. Before deep learning, researchers in this field proposed classic algorithms such as semi-supervised support vector machines, entropy regularization, and collaborative training.
Deep semi-supervised learningWith the rise of deep learning, deep semi-supervised learning algorithms have also made great progress. At the same time, technology companies including Microsoft, Google, and Meta have also recognized the huge potential of semi-supervised learning in practical scenarios.
For example, Google uses noisy student training, a semi-supervised algorithm, to improve its search performance [1]. The most representative semi-supervised algorithms currently use cross-entropy loss for training on labeled data, and consistency regularization on unlabeled data to encourage invariant predictions to input perturbations.
For example, the FixMatch[2] algorithm proposed by Google at NeurIPS 2020 uses augmentation anchoring and fixed thresholding technologies to enhance the model to enhance data with different strengths. Generalizability and reducing the impact of noisy pseudo labels. During training, FixMatch filters unlabeled data below a user-provided/pre-defined threshold.
FlexMatch[3], jointly proposed by Microsoft Research Asia and Tokyo Institute of Technology at NeurIPS 2021, takes into account the different learning difficulties between different categories, so it proposes course pseudo-labels ( curriculum pseudo labeling) technology, different thresholds should be used for different categories.
Specifically, for easy-to-learn categories, the model should set a high threshold to reduce the impact of noisy pseudo-labels; for difficult-to-learn categories, the model should set a low threshold to encourage this category fitting. The learning difficulty evaluation of each class depends on the number of unlabeled data samples falling into that class and above a fixed value.
At the same time, researchers from Microsoft Research Asia also collaborated to propose a unified Pytorch-based semi-supervised method code library TorchSSL[4], which provides deep methods and common data in the field. Sets and benchmark results are uniformly supported.
Figure 1: FlexMatch algorithm process
Problems and challenges in the current semi-supervised learning code library
Although the development of semi-supervised learning is in full swing, researchers have noticed that most of the current papers in the semi-supervised direction only focus on computer vision (CV) classification tasks. For other fields, such as natural language processing (NLP) and audio processing (audio), Researchers cannot know whether these algorithms that are effective in CV tasks are still effective in different fields.
In addition, most semi-supervised papers are published by large institutions, and it is often difficult for academic laboratories to participate in promoting the development of this field due to limitations in computing resources. . In general, semi-supervised learning benchmarks currently have the following two problems:
(1) Insufficient diversity. Most of the existing semi-supervised learning benchmarks are limited to CV classification tasks (i.e., CIFAR-10/100, SVHN, STL-10 and ImageNet classification), excluding consistent and diverse evaluation of classification tasks such as NLP, audio, etc., while in NLP The lack of sufficient labeled data in and audio is also a common problem.
(2) Time-consuming and unfriendly to academia. Existing semi-supervised learning benchmarks such as TorchSSL are often time-consuming and environmentally unfriendly as it often requires training deep neural network models from scratch. Specifically, evaluating FixMatch[1] using TorchSSL requires approximately 300 GPU days. Such high training costs make SSL-related research unaffordable for many research laboratories (especially those in academia or small research groups), thus hindering the progress of SSL.
USB: A new benchmark library with diverse tasks and more friendly to researchers
In order to solve the above problems, researchers from Microsoft Research Asia teamed up with Westlake University, Tokyo Researchers from TU, Carnegie Mellon University, Max Planck Institute and other institutions proposed Unified SSL Benchmark (USB), which is the first semi-supervised classification to unify visual, language and audio classification tasks Learning Benchmarks.
Compared with previous semi-supervised learning benchmarks (such as TorchSSL) that only focused on a small number of visual tasks, this benchmark not only introduces more diverse application fields, but also utilizes visual pre-training for the first time. The model (pretrained vision Transformer) greatly reduces the verification time of semi-supervised algorithms (from 7000 GPU hours to 900 GPU hours), making semi-supervised research more friendly to researchers, especially small research groups.
Relevant papers have been accepted by NeurIPS 2022, the top academic conference in the field of international artificial intelligence. (Click "Read the original text" to learn more)
Solution provided by USB
So, how can USB solve the problems of the current semi-supervised benchmarks in one go? ? The researchers mainly made the following improvements:
(1) To enhance task diversity, USB introduced 5 CV data sets, 5 NLP data sets and 5 audio data sets. and provides a diverse and challenging benchmark that enables consistent evaluation of multiple tasks from different domains. Table 1 provides a detailed comparison of tasks and training time between USB and TorchSSL.
Table 1: Task and training time comparison between USB and TorchSSL frameworks
(2) In order to improve training efficiency, researchers introduced pre-trained vision Transformer into SSL instead of training ResNets from scratch. Specifically, the researchers found that using pre-trained models can significantly reduce the number of training iterations without affecting performance (e.g., reducing the number of training iterations for a CV task from 1 million steps to 200,000 steps).
(3) In order to be more friendly to researchers, researchers have implemented 14 SSL algorithms as open source and open sourced a modular code library and related configuration files for researchers to easily reproduce the results in the USB report. To get started quickly, USB also provides detailed documentation and tutorials. In addition, USB also provides the pip package for users to directly call the SSL algorithm. The researchers promise to continue to add new algorithms (such as unbalanced semi-supervised algorithms, etc.) and more challenging data sets to USB in the future. Table 2 shows the algorithms and modules already supported in USB.
Table 2: Supported algorithms and modules in USB
Semi-supervised learning has important research and application value in the future by utilizing large amounts of unlabeled data to train more accurate and robust models. Researchers at Microsoft Research Asia expect that through this USB work, they can help academia and industry make greater progress in the field of semi-supervised learning.
The above is the detailed content of USB: The first semi-supervised classification learning benchmark that unifies visual, language and audio classification tasks. For more information, please follow other related articles on the PHP Chinese website!

Hot AI Tools

Undresser.AI Undress
AI-powered app for creating realistic nude photos

AI Clothes Remover
Online AI tool for removing clothes from photos.

Undress AI Tool
Undress images for free

Clothoff.io
AI clothes remover

AI Hentai Generator
Generate AI Hentai for free.

Hot Article

Hot Tools

Notepad++7.3.1
Easy-to-use and free code editor

SublimeText3 Chinese version
Chinese version, very easy to use

Zend Studio 13.0.1
Powerful PHP integrated development environment

Dreamweaver CS6
Visual web development tools

SublimeText3 Mac version
God-level code editing software (SublimeText3)

Hot Topics


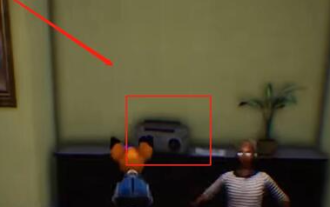
The Terror Corridor is a mission in Goat Simulator 3. How can you complete this mission? Master the detailed clearance methods and corresponding processes, and be able to complete the corresponding challenges of this mission. The following will bring you Goat Simulator. 3 Horror Corridor Guide to learn related information. Goat Simulator 3 Terror Corridor Guide 1. First, players need to go to Silent Hill in the upper left corner of the map. 2. Here you can see a house with RESTSTOP written on the roof. Players need to operate the goat to enter this house. 3. After entering the room, we first go straight forward, and then turn right. There is a door at the end here, and we go in directly from here. 4. After entering, we also need to walk forward first and then turn right. When we reach the door here, the door will be closed. We need to turn back and find it.
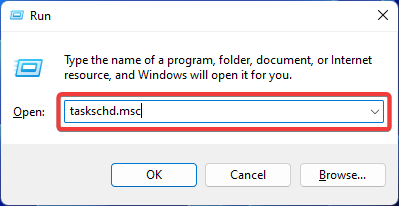
To automate tasks and manage multiple systems, mission planning software is a valuable tool in your arsenal, especially as a system administrator. Windows Task Scheduler does the job perfectly, but lately many people have reported operator rejected request errors. This problem exists in all iterations of the operating system, and even though it has been widely reported and covered, there is no effective solution. Keep reading to find out what might actually work for other people! What is the request in Task Scheduler 0x800710e0 that was denied by the operator or administrator? Task Scheduler allows automating various tasks and applications without user input. You can use it to schedule and organize specific applications, configure automatic notifications, help deliver messages, and more. it
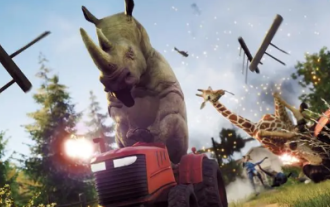
Goat Simulator 3 is a game with classic simulation gameplay, allowing players to fully experience the fun of casual action simulation. The game also has many exciting special tasks. Among them, the Goat Simulator 3 Imperial Tomb task requires players to find the bell tower. Some players are not sure how to operate the three clocks at the same time. Here is the guide to the Tomb of the Tomb mission in Goat Simulator 3! The guide to the Tomb of the Tomb mission in Goat Simulator 3 is to ring the bells in order. Detailed step expansion 1. First, players need to open the map and go to Wuqiu Cemetery. 2. Then go up to the bell tower. There will be three bells inside. 3. Then, in order from largest to smallest, follow the familiarity of 222312312. 4. After completing the knocking, you can complete the mission and open the door to get the lightsaber.
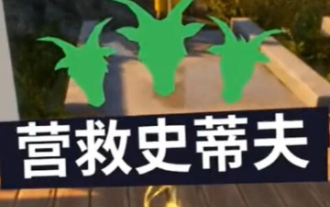
Rescue Steve is a unique task in Goat Simulator 3. What exactly needs to be done to complete it? This task is relatively simple, but we need to be careful not to misunderstand the meaning. Here we will bring you the rescue of Steve in Goat Simulator 3 Task strategies can help you better complete related tasks. Goat Simulator 3 Rescue Steve Mission Strategy 1. First come to the hot spring in the lower right corner of the map. 2. After arriving at the hot spring, you can trigger the task of rescuing Steve. 3. Note that there is a man in the hot spring. Although his name is Steve, he is not the target of this mission. 4. Find a fish named Steve in this hot spring and bring it ashore to complete this task.
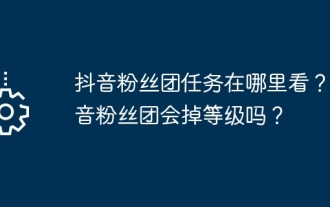
TikTok, as one of the most popular social media platforms at the moment, has attracted a large number of users to participate. On Douyin, there are many fan group tasks that users can complete to obtain certain rewards and benefits. So where can I find Douyin fan club tasks? 1. Where can I view Douyin fan club tasks? In order to find Douyin fan group tasks, you need to visit Douyin's personal homepage. On the homepage, you will see an option called "Fan Club." Click this option and you can browse the fan groups you have joined and related tasks. In the fan club task column, you will see various types of tasks, such as likes, comments, sharing, forwarding, etc. Each task has corresponding rewards and requirements. Generally speaking, after completing the task, you will receive a certain amount of gold coins or experience points.
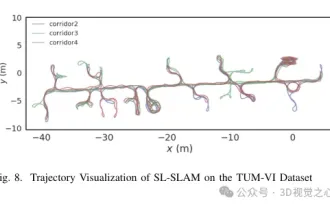
Written previously, today we discuss how deep learning technology can improve the performance of vision-based SLAM (simultaneous localization and mapping) in complex environments. By combining deep feature extraction and depth matching methods, here we introduce a versatile hybrid visual SLAM system designed to improve adaptation in challenging scenarios such as low-light conditions, dynamic lighting, weakly textured areas, and severe jitter. sex. Our system supports multiple modes, including extended monocular, stereo, monocular-inertial, and stereo-inertial configurations. In addition, it also analyzes how to combine visual SLAM with deep learning methods to inspire other research. Through extensive experiments on public datasets and self-sampled data, we demonstrate the superiority of SL-SLAM in terms of positioning accuracy and tracking robustness.
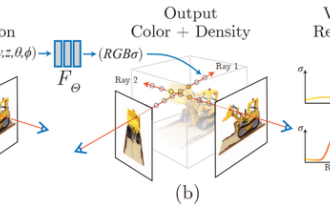
1 Introduction Neural Radiation Fields (NeRF) are a fairly new paradigm in the field of deep learning and computer vision. This technology was introduced in the ECCV2020 paper "NeRF: Representing Scenes as Neural Radiation Fields for View Synthesis" (which won the Best Paper Award) and has since become extremely popular, with nearly 800 citations to date [1 ]. The approach marks a sea change in the traditional way machine learning processes 3D data. Neural radiation field scene representation and differentiable rendering process: composite images by sampling 5D coordinates (position and viewing direction) along camera rays; feed these positions into an MLP to produce color and volumetric densities; and composite these values using volumetric rendering techniques image; the rendering function is differentiable, so it can be passed
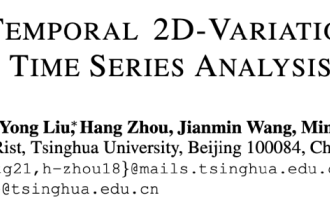
Achieving task universality is a core issue in the research of basic deep learning models, and is also one of the main focuses in the recent direction of large models. However, in the field of time series, various types of analysis tasks vary greatly. There are prediction tasks that require fine-grained modeling and classification tasks that require extracting high-level semantic information. How to build a unified deep basic model to efficiently complete various timing analysis tasks has not yet been established. To this end, a team from the School of Software of Tsinghua University conducted research on the basic issue of timing change modeling and proposed TimesNet, a task-universal timing basic model. The paper was accepted by ICLR 2023. Author list: Wu Haixu*, Hu Tengge*, Liu Yong*, Zhou Hang, Wang Jianmin, Long Mingsheng Link: https://ope
