


Tired of image generation, Google turned to text → video generation, two powerful tools that challenge resolution and length at the same time
After converting text to image for more than half a year, technology giants such as Meta and Google have set their sights on a new battlefield: text to video.
Last week, Meta announced a tool that can generate high-quality short videos - Make-A-Video. The videos generated using this tool are very imaginative.
Of course, Google is not to be outdone. Just now, the company’s CEO Sundar Pichai personally announced their latest achievements in this field: two text-to-video tools – Imagen Video and Phenaki. The former focuses on video quality, while the latter mainly challenges video length. It can be said that each has its own merits.
#The teddy bear washing dishes below was generated using Imagen Video. You can see that the resolution and coherence of the picture are certain. protection.
Imagen Video: Given text prompts, generate high-definition videos
Generative modeling in recent text-to-image AI systems Significant progress has been made in DALL-E 2, Imagen, Parti, CogView and Latent Diffusion. In particular, diffusion models have achieved great success in a variety of generative modeling tasks such as density estimation, text-to-speech, image-to-image, text-to-image, and 3D synthesis.
What Google wants to do is generate videos from text. Previous work on video generation has focused on restricted datasets with autoregressive models, latent variable models with autoregressive priors, and more recently, non-autoregressive latent variable methods. Diffusion models have also demonstrated excellent medium-resolution video generation capabilities.
On this basis, Google launched Imagen Video, a text-conditional video generation system based on the cascade video diffusion model. Given a text prompt, Imagen Video can generate high-definition video through a system consisting of a frozen T5 text encoder, a basic video generation model, and a cascaded spatiotemporal video super-resolution model.
Paper address: https://imagen.research.google/video/paper.pdf
In the paper, Google describes in detail how to extend the system into a high-definition text-to-video model, including selecting a fully convolutional spatiotemporal super-resolution model at certain resolutions and selecting the v parameter of the diffusion model. ization and other design decisions. Google has also successfully migrated previous diffusion-based image generation research results to a video generation setting.
Google found that Imagen Video was able to upscale the 24fps 64 frames 128×128 video generated by previous work to 128 frames 1280×768 HD video. In addition, Imagen Video has a high degree of controllability and world knowledge, can generate video and text animations in diverse artistic styles, and has 3D object understanding capabilities.
Let us enjoy some more videos generated by Imagen Video, such as panda driving:
游游Wooden ship in space:
For more generated videos, please see: https://imagen.research.google/video/
Methods and Experiments
Overall, Google’s video generation framework is a cascade of seven sub-video diffusion models, which perform text-conditional video generation, spatial super-resolution, and temporal super-resolution accordingly. Using the entire cascade, Imagen Video is able to produce 128 frames of 1280×768 HD video (approximately 126 million pixels) at 24 frames per second.
Meanwhile, with the help of progressive distillation, Imagen Video generates high-quality videos using only eight diffusion steps in each sub-model. This speeds up video generation time by approximately 18x.
Figure 6 below shows the entire cascade pipeline of Imagen Video, including a frozen text encoder, a basic video diffusion model and 3 spatial super-resolution (SSR) and 3 temporal super-resolution (TSR) models. The seven video diffusion models have a total of 11.6 billion parameters.
During the generation process, the SSR model improves the spatial resolution of all input frames, while the TSR model improves the temporal resolution by filling in intermediate frames between input frames. All models generate a complete block of frames simultaneously so that the SSR model does not suffer from noticeable artifacts.
Imagen Video is built on the video U-Net architecture, as shown in Figure 7 below.
In experiments, Imagen Video used the publicly available LAION-400M image-text dataset, 14 million video-text pairs and 60 million images. Train on text pairs. As a result, as mentioned above, Imagen Video is not only able to generate high-definition videos, but also has some unique features that unstructured generative models that learn purely from data do not have.
Figure 8 below shows Imagen Video’s ability to generate videos with an artistic style learned from image information, such as a video in the style of a Van Gogh painting or a watercolor painting.
Figure 9 below shows Imagen Video’s ability to understand 3D structures. It can generate videos of rotating objects, and the general structure of the object can also be reserve.
Figure 10 below shows how Imagen Video can reliably generate text in a variety of animated styles, some of which are difficult to create using traditional tools.
For more experimental details, please refer to the original paper.
Phenaki: You tell the story and I'll draw it
We know that although video is essentially a series of images, generating a coherent long video does not So easy, because there is very little high-quality data available for this task, and the task itself is computationally demanding.
What’s more troublesome is that short text prompts like the ones used for image generation are usually not enough to provide a complete description of the video. What the video needs is a series of prompts or stories. Ideally, a video generation model must be able to generate videos of any length and adjust the generated video frames according to the prompt changes at a certain time t. Only with this ability can the works generated by the model be called "video" rather than "moving images", and open up the road to real-life creative applications in art, design and content creation.
Researchers from Google and other institutions said, "To our knowledge, story-based conditional video generation has never been explored before, and this is the first paper moving towards this goal. Early papers."
- Paper link: https://pub-bede3007802c4858abc6f742f405d4ef.r2.dev/paper.pdf
- Project link: https://phenaki.github.io/#interactive
Since there is no story-based data set available Learning, there is no way for researchers to accomplish these tasks simply by relying on traditional deep learning methods (simply learning from data). Therefore, they designed a model specifically for this task.
This new text-to-video model is called Phenaki, which uses "text-to-video" and "text-to-image" data to jointly train. The model has the following capabilities:
1. Generate temporally coherent diverse videos under the condition of an open domain prompt, even if the prompt is a new concept combination (see Figure 3 below ). The generated video can be several minutes long, even though the video used for training the model is only 1.4 seconds (8 frames/second)
2. According to a The story (that is, a series of prompts) generates a video, as shown in Figure 1 and Figure 5 below:
From In the following animation we can see the coherence and diversity of the videos generated by Phenaki:
To achieve For these capabilities, researchers cannot rely on existing video encoders, which either only decode fixed-size videos or encode frames independently. To solve this problem, they introduced a new encoder-decoder architecture - C-ViViT.
C-ViViT can:
- Use the temporal redundancy in the video to improve the reconstruction quality of the model in each frame, while Compress the number of video tokens by 40% or more;
- Allows encoding and decoding of variable-length videos given a causal structure.
PHENAKI model architecture
Inspired by previous research on autoregressive text-to-image and text-to-video , Phenaki's design mainly consists of two parts (see Figure 2 below): an encoder-decoder model that compresses video into discrete embeddings (ie tokens) and a transformer model that converts text embeddings into video tokens.
Obtaining a compressed representation of a video is one of the main challenges in generating videos from text. Previous work either used per-frame image encoders, such as VQ-GAN, or fixed-length video encoders, such as VideoVQVAE. The former allows the generation of videos of arbitrary length, but in practical use, the videos must be short because the encoder cannot compress the video in time and the tokens are highly redundant in consecutive frames. The latter is more efficient in terms of number of tokens, but it does not allow generating videos of arbitrary length.
In Phenaki, the researcher's goal is to generate variable-length videos while compressing the number of video tokens as much as possible, so that the Transformer model can be used within the current computing resource constraints. To this end, they introduce C-ViViT, a causal variant of ViViT with additional architectural changes for video generation, which can compress videos in both temporal and spatial dimensions while maintaining temporal autoregression. This feature allows the generation of autoregressive videos of arbitrary length.
In order to obtain text embeddings, Phenaki also uses a pre-trained language model-T5X.
Please refer to the original paper for specific details.
The above is the detailed content of Tired of image generation, Google turned to text → video generation, two powerful tools that challenge resolution and length at the same time. For more information, please follow other related articles on the PHP Chinese website!

Hot AI Tools

Undresser.AI Undress
AI-powered app for creating realistic nude photos

AI Clothes Remover
Online AI tool for removing clothes from photos.

Undress AI Tool
Undress images for free

Clothoff.io
AI clothes remover

AI Hentai Generator
Generate AI Hentai for free.

Hot Article

Hot Tools

Notepad++7.3.1
Easy-to-use and free code editor

SublimeText3 Chinese version
Chinese version, very easy to use

Zend Studio 13.0.1
Powerful PHP integrated development environment

Dreamweaver CS6
Visual web development tools

SublimeText3 Mac version
God-level code editing software (SublimeText3)

Hot Topics


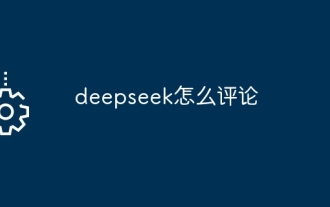
DeepSeek is a powerful information retrieval tool. Its advantage is that it can deeply mine information, but its disadvantages are that it is slow, the result presentation method is simple, and the database coverage is limited. It needs to be weighed according to specific needs.
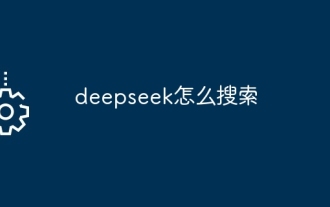
DeepSeek is a proprietary search engine that only searches in a specific database or system, faster and more accurate. When using it, users are advised to read the document, try different search strategies, seek help and feedback on the user experience in order to make the most of their advantages.
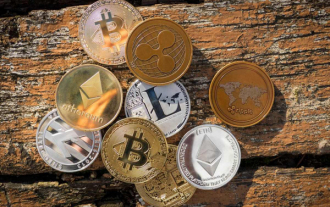
This article introduces the registration process of the Sesame Open Exchange (Gate.io) web version and the Gate trading app in detail. Whether it is web registration or app registration, you need to visit the official website or app store to download the genuine app, then fill in the user name, password, email, mobile phone number and other information, and complete email or mobile phone verification.
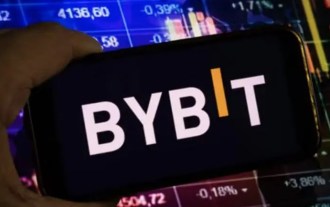
Why can’t the Bybit exchange link be directly downloaded and installed? Bybit is a cryptocurrency exchange that provides trading services to users. The exchange's mobile apps cannot be downloaded directly through AppStore or GooglePlay for the following reasons: 1. App Store policy restricts Apple and Google from having strict requirements on the types of applications allowed in the app store. Cryptocurrency exchange applications often do not meet these requirements because they involve financial services and require specific regulations and security standards. 2. Laws and regulations Compliance In many countries, activities related to cryptocurrency transactions are regulated or restricted. To comply with these regulations, Bybit Application can only be used through official websites or other authorized channels
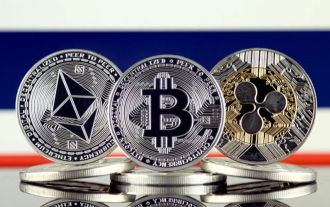
It is crucial to choose a formal channel to download the app and ensure the safety of your account.
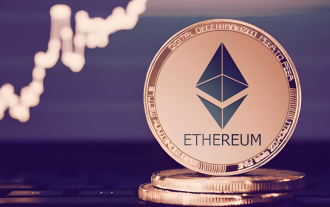
This article recommends the top ten cryptocurrency trading platforms worth paying attention to, including Binance, OKX, Gate.io, BitFlyer, KuCoin, Bybit, Coinbase Pro, Kraken, BYDFi and XBIT decentralized exchanges. These platforms have their own advantages in terms of transaction currency quantity, transaction type, security, compliance, and special features. For example, Binance is known for its largest transaction volume and abundant functions in the world, while BitFlyer attracts Asian users with its Japanese Financial Hall license and high security. Choosing a suitable platform requires comprehensive consideration based on your own trading experience, risk tolerance and investment preferences. Hope this article helps you find the best suit for yourself
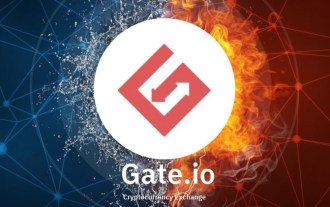
A detailed introduction to the login operation of the Sesame Open Exchange web version, including login steps and password recovery process. It also provides solutions to common problems such as login failure, unable to open the page, and unable to receive verification codes to help you log in to the platform smoothly.
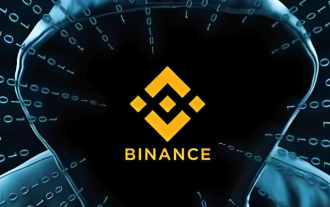
To access the latest version of Binance website login portal, just follow these simple steps. Go to the official website and click the "Login" button in the upper right corner. Select your existing login method. If you are a new user, please "Register". Enter your registered mobile number or email and password and complete authentication (such as mobile verification code or Google Authenticator). After successful verification, you can access the latest version of Binance official website login portal.
