The cost and sustainability of generative AI
AI is resource intensive for any platform, including public cloud. Most artificial intelligence technologies require a large amount of inference calculations, which collectively require higher processor, network, and storage requirements, and will also result in higher electricity bills, infrastructure costs, and carbon footprints.
The rise of generative artificial intelligence systems, taking ChatGPT as an example, has brought the above issues into focus again. Given the widespread adoption of this technology, and its likely large-scale adoption by companies, governments, and the general public, we can see the electricity consumption growth curve taking on a worrying arc.
Artificial intelligence technology has been around since the 1970s. Since the work of a mature artificial intelligence system requires a large amount of resources, it did not have much commercial impact initially. I remember that the artificial intelligence system I designed when I was in my 20s required more than $40 million in hardware, software, and data center space to run it. Spoiler alert: That project and many other AI projects never got off the ground. Not viable as a business case.
Cloud computing changes everything. Things that were once out of reach are now sufficiently cost-effective with the public cloud. In fact, as you might have guessed, the rise of the cloud has roughly coincided with the rise of artificial intelligence over the past 10 to 15 years. What I'm trying to say is that they are now tightly integrated.
Sustainability and Cost of Cloud Resources
You really don’t need to do much research to predict what will happen here. There will be a surge in demand for artificial intelligence services, such as generative AI systems that are now attracting attention, as well as other artificial intelligence and machine learning systems. This surge will be led by businesses looking for innovative advantages, such as smart supply chains, and even thousands of college students hoping to have a generative AI system to write their term papers.
More demand for artificial intelligence means more demand for the resources used by these artificial intelligence systems, such as public clouds and the services they provide. This demand is likely to be fulfilled through multiple data centers that house power-hungry servers and network equipment.
Public cloud providers, like any other utility resource provider, will increase prices as demand increases, just like we see seasonal increases in household electricity bills (also based on demand). Therefore, we often reduce usage, for example, turning the air conditioner to 24 degrees instead of 20 degrees in the summer.
However, higher cloud computing costs may not have the same impact on businesses. Enterprises may find that these artificial intelligence systems drive certain key business processes and are not dispensable. In many cases, they may try to save money within the business, perhaps by reducing headcount to offset the cost of AI systems. It’s no secret that generative AI systems will soon replace many information workers.
What can be done?
What can we do if the resource demands of running AI systems will result in higher computing costs and carbon output? The answer may lie in finding more efficient ways for AI to utilize resources such as processing, networking and storage.
For example, the sampling pipeline can speed up deep learning by reducing the amount of data processed. Research from MIT and IBM shows that you can use this approach to reduce the resources required to run neural networks on large data sets. However, it also limits accuracy, which may be acceptable for some business use cases, but not all.
Another approach already used in other technology areas is in-memory computing. This architecture can speed up AI processing by not moving data in and out of memory. Instead, AI calculations run directly within the memory module, which speeds things up significantly.
Other methods are also under development. For example, changing the physical processor, using co-processors for artificial intelligence calculations to increase computing speed, or adopting next-generation computing models such as quantum. You can expect a lot of technical announcements from the big public cloud vendors on how to solve many of these problems.
What should you do?
My advice is certainly not to avoid AI in order to get a lower cloud computing bill or save the planet. Artificial Intelligence is a foundational computing method that most businesses can leverage to gain significant value.
I recommend that you enter an AI-enabled or net new AI system development project with a clear understanding of the cost and impact on sustainability, which are directly related. You have to make a cost/benefit choice, and that really comes back to the value of cost and risk that you can bring to the business. After all, there is actually nothing new.
I do believe that most problems will be solved in innovative ways, whether it's memory or quantum computing or solutions we haven't seen yet. Both AI technology providers and cloud computing providers are keen to make AI more cost-effective and green. This is good news.
Source: www.cio.com
The above is the detailed content of The cost and sustainability of generative AI. For more information, please follow other related articles on the PHP Chinese website!

Hot AI Tools

Undresser.AI Undress
AI-powered app for creating realistic nude photos

AI Clothes Remover
Online AI tool for removing clothes from photos.

Undress AI Tool
Undress images for free

Clothoff.io
AI clothes remover

Video Face Swap
Swap faces in any video effortlessly with our completely free AI face swap tool!

Hot Article

Hot Tools

Notepad++7.3.1
Easy-to-use and free code editor

SublimeText3 Chinese version
Chinese version, very easy to use

Zend Studio 13.0.1
Powerful PHP integrated development environment

Dreamweaver CS6
Visual web development tools

SublimeText3 Mac version
God-level code editing software (SublimeText3)

Hot Topics




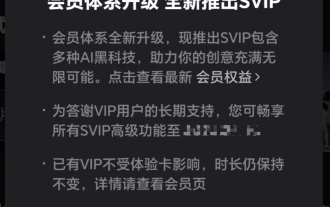
This site reported on June 27 that Jianying is a video editing software developed by FaceMeng Technology, a subsidiary of ByteDance. It relies on the Douyin platform and basically produces short video content for users of the platform. It is compatible with iOS, Android, and Windows. , MacOS and other operating systems. Jianying officially announced the upgrade of its membership system and launched a new SVIP, which includes a variety of AI black technologies, such as intelligent translation, intelligent highlighting, intelligent packaging, digital human synthesis, etc. In terms of price, the monthly fee for clipping SVIP is 79 yuan, the annual fee is 599 yuan (note on this site: equivalent to 49.9 yuan per month), the continuous monthly subscription is 59 yuan per month, and the continuous annual subscription is 499 yuan per year (equivalent to 41.6 yuan per month) . In addition, the cut official also stated that in order to improve the user experience, those who have subscribed to the original VIP
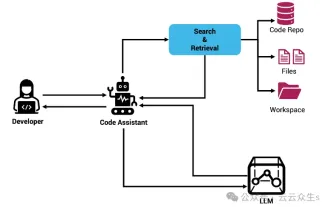
Improve developer productivity, efficiency, and accuracy by incorporating retrieval-enhanced generation and semantic memory into AI coding assistants. Translated from EnhancingAICodingAssistantswithContextUsingRAGandSEM-RAG, author JanakiramMSV. While basic AI programming assistants are naturally helpful, they often fail to provide the most relevant and correct code suggestions because they rely on a general understanding of the software language and the most common patterns of writing software. The code generated by these coding assistants is suitable for solving the problems they are responsible for solving, but often does not conform to the coding standards, conventions and styles of the individual teams. This often results in suggestions that need to be modified or refined in order for the code to be accepted into the application
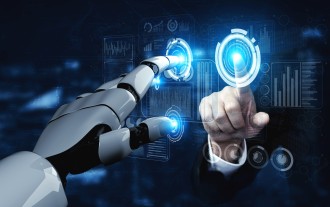
Large Language Models (LLMs) are trained on huge text databases, where they acquire large amounts of real-world knowledge. This knowledge is embedded into their parameters and can then be used when needed. The knowledge of these models is "reified" at the end of training. At the end of pre-training, the model actually stops learning. Align or fine-tune the model to learn how to leverage this knowledge and respond more naturally to user questions. But sometimes model knowledge is not enough, and although the model can access external content through RAG, it is considered beneficial to adapt the model to new domains through fine-tuning. This fine-tuning is performed using input from human annotators or other LLM creations, where the model encounters additional real-world knowledge and integrates it

To learn more about AIGC, please visit: 51CTOAI.x Community https://www.51cto.com/aigc/Translator|Jingyan Reviewer|Chonglou is different from the traditional question bank that can be seen everywhere on the Internet. These questions It requires thinking outside the box. Large Language Models (LLMs) are increasingly important in the fields of data science, generative artificial intelligence (GenAI), and artificial intelligence. These complex algorithms enhance human skills and drive efficiency and innovation in many industries, becoming the key for companies to remain competitive. LLM has a wide range of applications. It can be used in fields such as natural language processing, text generation, speech recognition and recommendation systems. By learning from large amounts of data, LLM is able to generate text
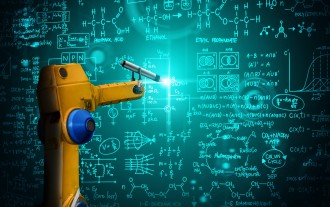
Machine learning is an important branch of artificial intelligence that gives computers the ability to learn from data and improve their capabilities without being explicitly programmed. Machine learning has a wide range of applications in various fields, from image recognition and natural language processing to recommendation systems and fraud detection, and it is changing the way we live. There are many different methods and theories in the field of machine learning, among which the five most influential methods are called the "Five Schools of Machine Learning". The five major schools are the symbolic school, the connectionist school, the evolutionary school, the Bayesian school and the analogy school. 1. Symbolism, also known as symbolism, emphasizes the use of symbols for logical reasoning and expression of knowledge. This school of thought believes that learning is a process of reverse deduction, through existing
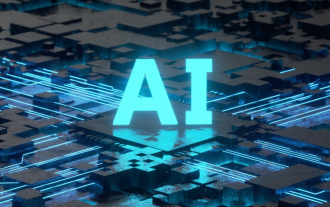
Editor |ScienceAI Question Answering (QA) data set plays a vital role in promoting natural language processing (NLP) research. High-quality QA data sets can not only be used to fine-tune models, but also effectively evaluate the capabilities of large language models (LLM), especially the ability to understand and reason about scientific knowledge. Although there are currently many scientific QA data sets covering medicine, chemistry, biology and other fields, these data sets still have some shortcomings. First, the data form is relatively simple, most of which are multiple-choice questions. They are easy to evaluate, but limit the model's answer selection range and cannot fully test the model's ability to answer scientific questions. In contrast, open-ended Q&A
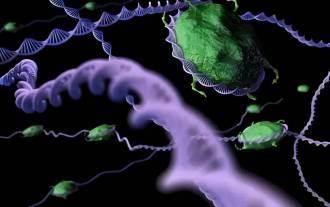
Editor | KX In the field of drug research and development, accurately and effectively predicting the binding affinity of proteins and ligands is crucial for drug screening and optimization. However, current studies do not take into account the important role of molecular surface information in protein-ligand interactions. Based on this, researchers from Xiamen University proposed a novel multi-modal feature extraction (MFE) framework, which for the first time combines information on protein surface, 3D structure and sequence, and uses a cross-attention mechanism to compare different modalities. feature alignment. Experimental results demonstrate that this method achieves state-of-the-art performance in predicting protein-ligand binding affinities. Furthermore, ablation studies demonstrate the effectiveness and necessity of protein surface information and multimodal feature alignment within this framework. Related research begins with "S
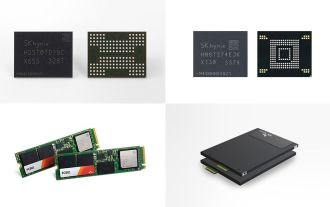
According to news from this site on August 1, SK Hynix released a blog post today (August 1), announcing that it will attend the Global Semiconductor Memory Summit FMS2024 to be held in Santa Clara, California, USA from August 6 to 8, showcasing many new technologies. generation product. Introduction to the Future Memory and Storage Summit (FutureMemoryandStorage), formerly the Flash Memory Summit (FlashMemorySummit) mainly for NAND suppliers, in the context of increasing attention to artificial intelligence technology, this year was renamed the Future Memory and Storage Summit (FutureMemoryandStorage) to invite DRAM and storage vendors and many more players. New product SK hynix launched last year
