How can technology support better, greener cities?
Urban population accounts for more than half of the world's population, and it is expected that by 2050, the urban population will increase by another 2.5 billion. They face growing environmental, social and financial pressures and crises (including the recent COVID-19 crisis), as well as infrastructure needs and increasing demands from residents for affordable services and improved quality of life.
However, local authorities and municipalities often lack well-prepared, high-quality and bankable infrastructure projects. In emerging markets around the world, which account for the majority of greenhouse gas emissions, they often exhibit poor infrastructure governance, economic regulation and policy, as well as limited fiscal autonomy and poor financial management.
The resilience of cities and infrastructure services is also critical to the economic recovery from the COVID pandemic, and to responding to past and current fiscal crises and recessions. Fiscal space for many local governments is shrinking. These impacts could limit the city's economic opportunities as revenues plummet along with the fiscal value of real estate.
At the same time, urban spending is increasing due to a slowdown in economic activity and a corresponding increase in job losses and safety net-related measures. Reduced revenues and increased expenditures will lead many cities to adopt prudent fiscal policies and significant adjustments in capital expenditures. Outside our European and UK cities, foreign investment to finance emerging market infrastructure has fallen; ongoing operations have been suspended and many projects have either been canceled or delayed.
So, in this economic climate, how can we contribute to the development of liveable, smart and green cities? At Wiia, we specialize in green cities and infrastructure tenders; low carbon and climate resilient Changing growth combined with smart technology can help cities address the above challenges. We need to green the recovery, build the resilience of cities and support economic transformation. We believe these three principles can help local governments develop effective bids, projects and spending to support the low carbon and climate resilient cities of our future:
Principle 1: Use data to create new businesses Model
Cities, regardless of their complexity and scope, generate oceans of data. Finding insights from all this data can help municipalities respond to emergencies, allocate resources wisely, and create new business models. Additionally, exposing real-time information helps individuals make better decisions and helps businesses create new revenue streams. As cities become smarter, they also become more livable and responsive.
Today, we can only see the role technology will eventually play in urban environments, such as real-time traffic data streaming, the use of drones and V2V connections. Smarter investments linked to spending, supported by data, mean strategically choosing to develop cost-effective projects that help address short-term difficulties while working towards long-term transport sustainability goals.
Principle 2: Integrate gender-based dimensions
The gender imbalance emerging from current patterns and trends in mobility and transportation reveals the disparities that exist in many cities around the world , which fundamentally affects three different aspects: the lack of knowledge about gender issues, the lack of gender mobility data and statistics, the need to plan gender-specific mobility services, and the need to better exploit synergies between urban and mobility planning.
Lessons learned from experience across Europe show that, in addition to the huge information gaps that need to be filled through improved gender-based statistics and research, measures implemented at local level are often pilot projects, due to a lack of Implementation and sustainability issues arise with the use of potentially scalable technologies.
Principle 3: Promote decarbonization through integrated technologies
Decarbonizing all modes of transport by 2050 is possible, but action is needed now. Cost-effective solutions for passenger and freight transport already exist and have been tested at scale – with electrified mobility solutions shared and integrated with walking, cycling and public transport to great effect.
Our analysis shows that to avoid unnecessary travel, shift to more efficient modes, and improve vehicles and fuels, policy and business actions by countries and cities need to be coordinated. Only by integrating the transport decarbonization model into the energy transition model can national policies be aligned behind compact, connected, clean cities.
For example, local authorities could support schemes and opportunities to deploy distributed renewable energy or improve building energy efficiency. Circular and systemic approaches are crucial if we want to create a living, human city where everything is used, reused and interconnected. Isolating or lumping specific solutions – such as transport versus energy – together will only have limited, and likely short-term, impact.
The above is the detailed content of How can technology support better, greener cities?. For more information, please follow other related articles on the PHP Chinese website!

Hot AI Tools

Undresser.AI Undress
AI-powered app for creating realistic nude photos

AI Clothes Remover
Online AI tool for removing clothes from photos.

Undress AI Tool
Undress images for free

Clothoff.io
AI clothes remover

AI Hentai Generator
Generate AI Hentai for free.

Hot Article

Hot Tools

Notepad++7.3.1
Easy-to-use and free code editor

SublimeText3 Chinese version
Chinese version, very easy to use

Zend Studio 13.0.1
Powerful PHP integrated development environment

Dreamweaver CS6
Visual web development tools

SublimeText3 Mac version
God-level code editing software (SublimeText3)

Hot Topics


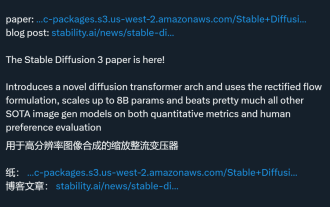
StableDiffusion3’s paper is finally here! This model was released two weeks ago and uses the same DiT (DiffusionTransformer) architecture as Sora. It caused quite a stir once it was released. Compared with the previous version, the quality of the images generated by StableDiffusion3 has been significantly improved. It now supports multi-theme prompts, and the text writing effect has also been improved, and garbled characters no longer appear. StabilityAI pointed out that StableDiffusion3 is a series of models with parameter sizes ranging from 800M to 8B. This parameter range means that the model can be run directly on many portable devices, significantly reducing the use of AI
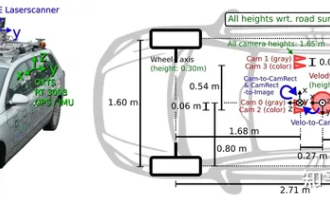
The first pilot and key article mainly introduces several commonly used coordinate systems in autonomous driving technology, and how to complete the correlation and conversion between them, and finally build a unified environment model. The focus here is to understand the conversion from vehicle to camera rigid body (external parameters), camera to image conversion (internal parameters), and image to pixel unit conversion. The conversion from 3D to 2D will have corresponding distortion, translation, etc. Key points: The vehicle coordinate system and the camera body coordinate system need to be rewritten: the plane coordinate system and the pixel coordinate system. Difficulty: image distortion must be considered. Both de-distortion and distortion addition are compensated on the image plane. 2. Introduction There are four vision systems in total. Coordinate system: pixel plane coordinate system (u, v), image coordinate system (x, y), camera coordinate system () and world coordinate system (). There is a relationship between each coordinate system,
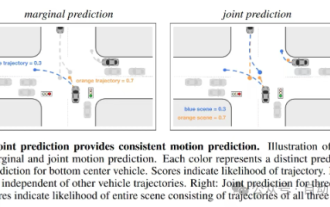
Trajectory prediction plays an important role in autonomous driving. Autonomous driving trajectory prediction refers to predicting the future driving trajectory of the vehicle by analyzing various data during the vehicle's driving process. As the core module of autonomous driving, the quality of trajectory prediction is crucial to downstream planning control. The trajectory prediction task has a rich technology stack and requires familiarity with autonomous driving dynamic/static perception, high-precision maps, lane lines, neural network architecture (CNN&GNN&Transformer) skills, etc. It is very difficult to get started! Many fans hope to get started with trajectory prediction as soon as possible and avoid pitfalls. Today I will take stock of some common problems and introductory learning methods for trajectory prediction! Introductory related knowledge 1. Are the preview papers in order? A: Look at the survey first, p
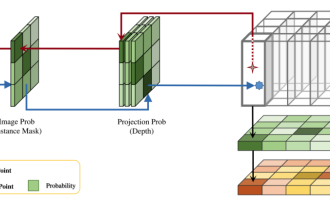
This paper explores the problem of accurately detecting objects from different viewing angles (such as perspective and bird's-eye view) in autonomous driving, especially how to effectively transform features from perspective (PV) to bird's-eye view (BEV) space. Transformation is implemented via the Visual Transformation (VT) module. Existing methods are broadly divided into two strategies: 2D to 3D and 3D to 2D conversion. 2D-to-3D methods improve dense 2D features by predicting depth probabilities, but the inherent uncertainty of depth predictions, especially in distant regions, may introduce inaccuracies. While 3D to 2D methods usually use 3D queries to sample 2D features and learn the attention weights of the correspondence between 3D and 2D features through a Transformer, which increases the computational and deployment time.
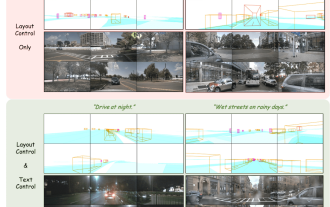
Some of the author’s personal thoughts In the field of autonomous driving, with the development of BEV-based sub-tasks/end-to-end solutions, high-quality multi-view training data and corresponding simulation scene construction have become increasingly important. In response to the pain points of current tasks, "high quality" can be decoupled into three aspects: long-tail scenarios in different dimensions: such as close-range vehicles in obstacle data and precise heading angles during car cutting, as well as lane line data. Scenes such as curves with different curvatures or ramps/mergings/mergings that are difficult to capture. These often rely on large amounts of data collection and complex data mining strategies, which are costly. 3D true value - highly consistent image: Current BEV data acquisition is often affected by errors in sensor installation/calibration, high-precision maps and the reconstruction algorithm itself. this led me to
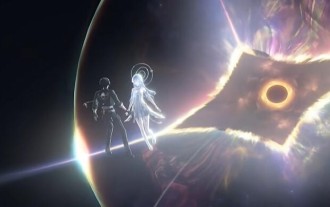
There are many treasure chests in Mingchao Jinzhou City. Many players want to know where the treasure chests are. The editor has marked the locations of all the treasure chests. Players can just follow the marked numbers to find them one by one. Let’s take a look at this Mingchao article for details. The latest collection of all treasure chests in Jinzhou City. Mingchao Strategy Collection Mingchao Jinzhou City Treasure Box Collection Location 1: Jinzhou City (1-25) Twenty-five treasure boxes, five voice boxes Location 2: Jinzhou City (26-41) Fifteen treasure boxes, three A voice box, two flying hunters
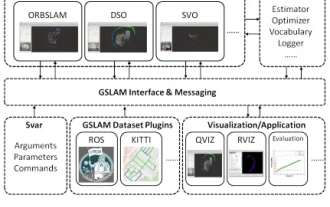
Suddenly discovered a 19-year-old paper GSLAM: A General SLAM Framework and Benchmark open source code: https://github.com/zdzhaoyong/GSLAM Go directly to the full text and feel the quality of this work ~ 1 Abstract SLAM technology has achieved many successes recently and attracted many attracted the attention of high-tech companies. However, how to effectively perform benchmarks on speed, robustness, and portability with interfaces to existing or emerging algorithms remains a problem. In this paper, a new SLAM platform called GSLAM is proposed, which not only provides evaluation capabilities but also provides researchers with a useful way to quickly develop their own SLAM systems.
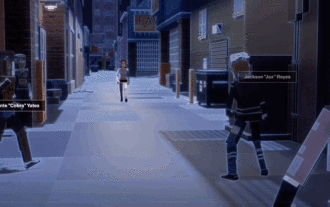
Please note that this square man is frowning, thinking about the identities of the "uninvited guests" in front of him. It turned out that she was in a dangerous situation, and once she realized this, she quickly began a mental search to find a strategy to solve the problem. Ultimately, she decided to flee the scene and then seek help as quickly as possible and take immediate action. At the same time, the person on the opposite side was thinking the same thing as her... There was such a scene in "Minecraft" where all the characters were controlled by artificial intelligence. Each of them has a unique identity setting. For example, the girl mentioned before is a 17-year-old but smart and brave courier. They have the ability to remember and think, and live like humans in this small town set in Minecraft. What drives them is a brand new,
