14 common operations in Excel using Python
Hello everyone, I am a rookie!
The data is the sales data found online. It looks like this:
1. Association formula: Vlookup
vlookup is almost the most commonly used formula in Excel, and is generally used for related queries between two tables. So I first divided this table into two tables.
df1=sale[['订单明细号','单据日期','地区名称', '业务员名称','客户分类', '存货编码', '客户名称', '业务员编码', '存货名称', '订单号', '客户编码', '部门名称', '部门编码']] df2=sale[['订单明细号','存货分类', '税费', '不含税金额', '订单金额', '利润', '单价','数量']]
Demand: I want to know the profit corresponding to each order of df1.
The profit column exists in the table of df2, so I want to know the profit corresponding to each order of df1. When using excel, first confirm that the order detail number is a unique value, then add a new column in df1 and write: =vlookup(a2,df2!a:h,6,0), and then pull it down and it will be OK. (I won’t write excel for the remaining 13)
How to implement it using python?
#查看订单明细号是否重复,结果是没。 df1["订单明细号"].duplicated().value_counts() df2["订单明细号"].duplicated().value_counts() df_c=pd.merge(df1,df2,on="订单明细号",how="left")
2. Pivot table
Requirement: I want to know the total profit and average profit earned by salesmen in each region.
pd.pivot_table(sale,index="地区名称",columns="业务员名称",values="利润",aggfunc=[np.sum,np.mean])
3. Compare the differences between the two columns
Because the data dimensions in each column of this table are different, comparison is meaningless, so I first made an order detail The differences in numbers are then compared.
Requirement: Compare the difference between the order detail number and order detail number 2 and display it.
sale["订单明细号2"]=sale["订单明细号"] #在订单明细号2里前10个都+1. sale["订单明细号2"][1:10]=sale["订单明细号2"][1:10]+1 #差异输出 result=sale.loc[sale["订单明细号"].isin(sale["订单明细号2"])==False]
4. Remove duplicate values
Requirement: Remove duplicate values coded by the salesperson
sale.drop_duplicates("业务员编码",inplace=True)
5. Missing value processing
First check which columns of sales data have missing values.
#列的行数小于index的行数的说明有缺失值,这里客户名称329<335,说明有缺失值 sale.info()
Requirements: fill in missing values with 0 or delete rows with missing values in customer codes. In fact, the method of processing missing values is very complicated. Here we only introduce simple processing methods. If it is a numerical variable, the most commonly used method is the average, median or mode. For more complex ones, the random forest model can be used to predict based on other dimensions. The result is populated. If it is a categorical variable, it is more accurate to fill it in based on business logic. For example, the requirement here is to fill in the missing value of the customer name: it can be filled according to the customer name corresponding to the inventory with the highest frequency of occurrence in the inventory classification.
Here we use a simple solution: fill the missing values with 0 or delete the rows with missing values in the customer code.
#用0填充缺失值 sale["客户名称"]=sale["客户名称"].fillna(0) #删除有客户编码缺失值的行 sale.dropna(subset=["客户编码"])
6. Multi-condition filtering
Demand: I would like to know the information about salesman Zhang Ai, who sells goods in the Beijing area with an order amount greater than 6,000.
sale.loc[(sale["地区名称"]=="北京")&(sale["业务员名称"]=="张爱")&(sale["订单金额"]>5000)]
7. Fuzzy filtering data
Requirement: Filter information whose inventory name contains "Samsung" or "Sony".
sale.loc[sale["存货名称"].str.contains("三星|索尼")]
8. Classification and summary
Demand: The total profit of each salesperson in the Beijing area.
sale.groupby(["地区名称","业务员名称"])["利润"].sum()
9. Condition calculation
Demand: How many orders have the inventory name containing "Samsung" and the tax is higher than 1,000? What is the sum and average profit of these orders? (Or minimum value, maximum value, quartile, label difference)
sale.loc[sale["存货名称"].str.contains("三星")&(sale["税费"]>=1000)][["订单明细号","利润"]].describe()
10. Delete spaces between data
Requirement: Delete the spaces on both sides of the inventory name.
sale["Inventory name"].map(lambda s:s.strip(""))
11. Data separation
Requirement: Separate date and time.
sale=pd.merge(sale,pd.DataFrame(sale["单据日期"].str.split(" ",expand=True)),how="inner",left_index=True,right_index=True)
12. Replacement of outliers
First, use the describe() function to briefly check whether there are any outliers in the data.
#You can see that the output tax has a negative number. This is generally not the case and is regarded as an outlier.
sale.describe()
Requirement: Use 0 to replace outliers.
sale["订单金额"]=sale["订单金额"].replace(min(sale["订单金额"]),0)
13. Grouping
Requirements: Group regions according to profit data distribution: "Poor", "Medium", "Better", "Very Good" Okay"
First of all, of course, we need to look at the data distribution of profits. Here we use quartiles to judge.
sale.groupby("地区名称")["利润"].sum().describe()
Group the regional total profit in the [-9,7091] interval as "poor" according to the quartile, (7091,10952] interval The grouping is "moderate" (10952,17656] is grouped as good, (17656,37556] is grouped as very good.
#先建立一个Dataframe sale_area=pd.DataFrame(sale.groupby("地区名称")["利润"].sum()).reset_index() #设置bins,和分组名称 bins=[-10,7091,10952,17656,37556] groups=["较差","中等","较好","非常好"] #使用cut分组 #sale_area["分组"]=pd.cut(sale_area["利润"],bins,labels=groups)
14. Define tags according to business logic
Demand: Product information with a sales profit margin (i.e. profit/order amount) greater than 30% and marking it as a high-quality product, and less than 5% as an ordinary product.
sale.loc[(sale["利润"]/sale["订单金额"])>0.3,"label"]="优质商品" sale.loc[(sale["利润"]/sale["订单金额"])<0.05,"label"]="一般商品"
In fact, there are many commonly used operations in excel, I just I have listed 14 commonly used ones. If you want to implement any other operations, you can comment and discuss them together. In addition, I also know that my writing of Python is not streamlined enough, so I use loc inertly. (In fact, the query will be more streamlined). If you are familiar with these few If you have a better way to write the operation, please be sure to comment and let me know, thank you!
Finally, I would like to say that I think it is best not to compare excel and python to study which one is easier to use. In fact, they are both tools. As the most widespread data processing tool, excel has been monopolized for so many years and must be quite convenient in data processing. Excellent, some operations are indeed easier in python, but there are also many operations in excel that are easier than python.
For example, a very simple operation: sum each column and display it on the bottom line. Excel just adds a sum() function to a column, and then pulls it to the left to solve it, while python Then you need to define a function (because python needs to determine the format and will directly report an error if it is not a numeric data.)
The above is the detailed content of 14 common operations in Excel using Python. For more information, please follow other related articles on the PHP Chinese website!

Hot AI Tools

Undresser.AI Undress
AI-powered app for creating realistic nude photos

AI Clothes Remover
Online AI tool for removing clothes from photos.

Undress AI Tool
Undress images for free

Clothoff.io
AI clothes remover

AI Hentai Generator
Generate AI Hentai for free.

Hot Article

Hot Tools

Notepad++7.3.1
Easy-to-use and free code editor

SublimeText3 Chinese version
Chinese version, very easy to use

Zend Studio 13.0.1
Powerful PHP integrated development environment

Dreamweaver CS6
Visual web development tools

SublimeText3 Mac version
God-level code editing software (SublimeText3)

Hot Topics


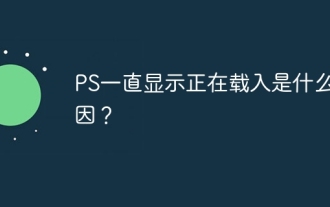
PS "Loading" problems are caused by resource access or processing problems: hard disk reading speed is slow or bad: Use CrystalDiskInfo to check the hard disk health and replace the problematic hard disk. Insufficient memory: Upgrade memory to meet PS's needs for high-resolution images and complex layer processing. Graphics card drivers are outdated or corrupted: Update the drivers to optimize communication between the PS and the graphics card. File paths are too long or file names have special characters: use short paths and avoid special characters. PS's own problem: Reinstall or repair the PS installer.
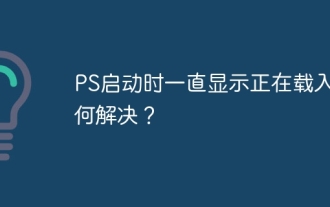
A PS stuck on "Loading" when booting can be caused by various reasons: Disable corrupt or conflicting plugins. Delete or rename a corrupted configuration file. Close unnecessary programs or upgrade memory to avoid insufficient memory. Upgrade to a solid-state drive to speed up hard drive reading. Reinstalling PS to repair corrupt system files or installation package issues. View error information during the startup process of error log analysis.
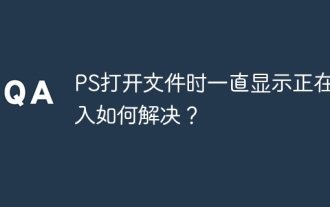
"Loading" stuttering occurs when opening a file on PS. The reasons may include: too large or corrupted file, insufficient memory, slow hard disk speed, graphics card driver problems, PS version or plug-in conflicts. The solutions are: check file size and integrity, increase memory, upgrade hard disk, update graphics card driver, uninstall or disable suspicious plug-ins, and reinstall PS. This problem can be effectively solved by gradually checking and making good use of PS performance settings and developing good file management habits.
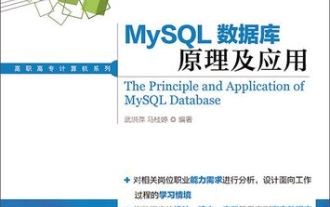
The article introduces the operation of MySQL database. First, you need to install a MySQL client, such as MySQLWorkbench or command line client. 1. Use the mysql-uroot-p command to connect to the server and log in with the root account password; 2. Use CREATEDATABASE to create a database, and USE select a database; 3. Use CREATETABLE to create a table, define fields and data types; 4. Use INSERTINTO to insert data, query data, update data by UPDATE, and delete data by DELETE. Only by mastering these steps, learning to deal with common problems and optimizing database performance can you use MySQL efficiently.
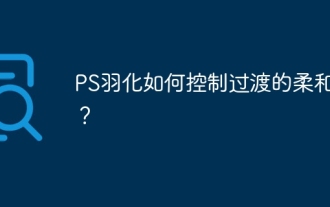
The key to feather control is to understand its gradual nature. PS itself does not provide the option to directly control the gradient curve, but you can flexibly adjust the radius and gradient softness by multiple feathering, matching masks, and fine selections to achieve a natural transition effect.
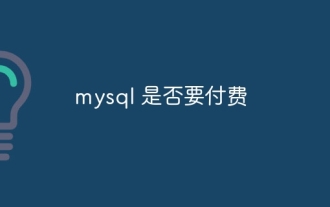
MySQL has a free community version and a paid enterprise version. The community version can be used and modified for free, but the support is limited and is suitable for applications with low stability requirements and strong technical capabilities. The Enterprise Edition provides comprehensive commercial support for applications that require a stable, reliable, high-performance database and willing to pay for support. Factors considered when choosing a version include application criticality, budgeting, and technical skills. There is no perfect option, only the most suitable option, and you need to choose carefully according to the specific situation.
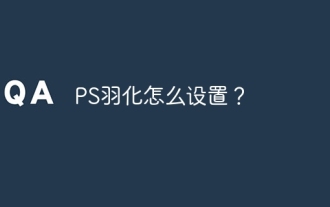
PS feathering is an image edge blur effect, which is achieved by weighted average of pixels in the edge area. Setting the feather radius can control the degree of blur, and the larger the value, the more blurred it is. Flexible adjustment of the radius can optimize the effect according to images and needs. For example, using a smaller radius to maintain details when processing character photos, and using a larger radius to create a hazy feeling when processing art works. However, it should be noted that too large the radius can easily lose edge details, and too small the effect will not be obvious. The feathering effect is affected by the image resolution and needs to be adjusted according to image understanding and effect grasp.
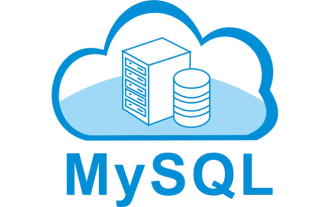
MySQL performance optimization needs to start from three aspects: installation configuration, indexing and query optimization, monitoring and tuning. 1. After installation, you need to adjust the my.cnf file according to the server configuration, such as the innodb_buffer_pool_size parameter, and close query_cache_size; 2. Create a suitable index to avoid excessive indexes, and optimize query statements, such as using the EXPLAIN command to analyze the execution plan; 3. Use MySQL's own monitoring tool (SHOWPROCESSLIST, SHOWSTATUS) to monitor the database health, and regularly back up and organize the database. Only by continuously optimizing these steps can the performance of MySQL database be improved.
