How nodejs interacts with big data
With the rapid development of the Internet and data technology, big data has gradually become one of the cores of corporate development strategies. In this data-driven era, how to efficiently process and manage massive data has become an important issue faced by enterprises. As a lightweight JavaScript running environment, Nodejs has also begun to be widely used in the field of big data, greatly improving the data processing efficiency and flexibility of enterprises.
How does Nodejs interact with big data?
Nodejs, as a JavaScript language running environment, can interact with various data storage systems through its powerful module mechanism. In the field of big data, distributed storage, distributed computing and other technologies are generally used, such as Hadoop, Spark, etc. Below, we will use Hadoop as an example to introduce how Nodejs interacts with big data.
- Using HDFS API for file operations
Hadoop Distributed File System (HDFS) is one of the core components of Hadoop, which can store large amounts of data in a distributed environment , and process them through the MapReduce computing model. Nodejs can directly interact with HDFS through the HDFS API to implement file upload, file download, file deletion and other operations.
The following is an example of using HDFS API to upload files in Nodejs:
const WebHDFS = require('webhdfs'); const fs = require('fs'); const hdfs = WebHDFS.createClient({ user: 'hadoop', host: 'hadoop-cluster', port: 50070, path: '/webhdfs/v1' }); const localFile = 'test.txt'; const remoteFile = '/user/hadoop/test.txt'; fs.createReadStream(localFile) .pipe(hdfs.createWriteStream(remoteFile)) .on('error', (err) => { console.error(`Error uploading file: ${err.message}`); }) .on('finish', () => { console.log('File uploaded successfully'); });
In this example, the webhdfs module is used to create an HDFS client through the HDFS URL and port number, and then use Nodejs The built-in fs module reads the file from the local and finally uploads it to HDFS.
- Using Hadoop Streaming for MapReduce calculations
MapReduce is a distributed computing model used to process large data sets in distributed storage. The MapReduce framework included in Hadoop can develop MapReduce tasks using Java language. However, using the MapReduce framework in Nodejs requires an adapter class library, which obviously reduces development efficiency. Therefore, using Hadoop Streaming can avoid this problem.
Hadoop Streaming is a tool for starting MapReduce tasks. It can interact with MapReduce tasks through standard input and standard output. Nodejs can use the child_process module to create a child process and pass the MapReduce program to be executed as a command line parameter into the child process. For specific implementation methods, please refer to the following sample code:
// mapper.js const readline = require('readline'); const rl = readline.createInterface({ input: process.stdin, output: process.stdout, terminal: false }); rl.on('line', (line) => { line .toLowerCase() .replace(/[.,?!]/g, '') .split(' ') .filter((word) => word.length > 0) .forEach((word) => console.log(`${word}\t1`)); }); // reducer.js let count = 0; process.stdin.resume(); process.stdin.setEncoding('utf-8'); process.stdin.on('data', (chunk) => { const lines = chunk.split('\n'); lines.forEach((line) => { if (line.trim().length) { const [word, num] = line.split('\t'); count += parseInt(num); } }); }); process.stdin.on('end', () => { console.log(`Total count: ${count}`); });
The above sample code is a simple MapReduce program. mapper.js cuts and filters the text in the input stream, and finally outputs the statistical results to the standard output stream. reducer.js reads data from the standard input stream, cumulatively counts the values of the same key, and finally outputs the result.
This MapReduce program can be executed through the following Nodejs code:
const { spawn } = require('child_process'); const mapper = spawn('/path/to/mapper.js'); const reducer = spawn('/path/to/reducer.js'); mapper.stdout.pipe(reducer.stdin); reducer.stdout.on('data', (data) => { console.log(`Result: ${data}`); }); mapper.stderr.on('data', (err) => { console.error(`Mapper error: ${err}`); }); reducer.stderr.on('data', (err) => { console.error(`Reducer error: ${err}`); }); reducer.on('exit', (code) => { console.log(`Reducer process exited with code ${code}`); });
In this example, the child_process module is used to create two child processes, one for executing mapper.js and one for executing reducer.js . The standard input and output of mapper and reducer are connected to form a MapReduce task, and the calculation results are finally output to the standard output stream.
In addition to using HDFS API and Hadoop Streaming, Nodejs can also interact with big data in various other ways, such as through RESTful API, using data collectors, etc. Of course, in practical applications, we need to choose the most suitable interaction method according to specific scenarios.
Summary
This article introduces how Nodejs interacts with big data. By using HDFS API and Hadoop Streaming, operations such as reading and writing big data and MapReduce calculations can be realized. Nodejs has the advantages of lightweight and high efficiency in the field of big data, and can help enterprises better manage and process massive data.
The above is the detailed content of How nodejs interacts with big data. For more information, please follow other related articles on the PHP Chinese website!

Hot AI Tools

Undresser.AI Undress
AI-powered app for creating realistic nude photos

AI Clothes Remover
Online AI tool for removing clothes from photos.

Undress AI Tool
Undress images for free

Clothoff.io
AI clothes remover

Video Face Swap
Swap faces in any video effortlessly with our completely free AI face swap tool!

Hot Article

Hot Tools

Notepad++7.3.1
Easy-to-use and free code editor

SublimeText3 Chinese version
Chinese version, very easy to use

Zend Studio 13.0.1
Powerful PHP integrated development environment

Dreamweaver CS6
Visual web development tools

SublimeText3 Mac version
God-level code editing software (SublimeText3)

Hot Topics



React combines JSX and HTML to improve user experience. 1) JSX embeds HTML to make development more intuitive. 2) The virtual DOM mechanism optimizes performance and reduces DOM operations. 3) Component-based management UI to improve maintainability. 4) State management and event processing enhance interactivity.
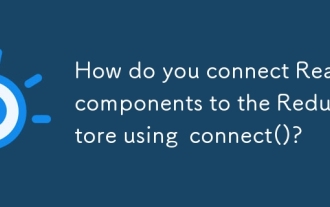
Article discusses connecting React components to Redux store using connect(), explaining mapStateToProps, mapDispatchToProps, and performance impacts.
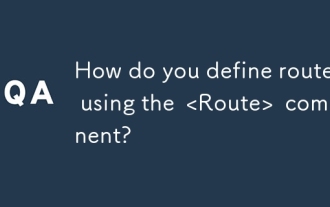
The article discusses defining routes in React Router using the <Route> component, covering props like path, component, render, children, exact, and nested routing.
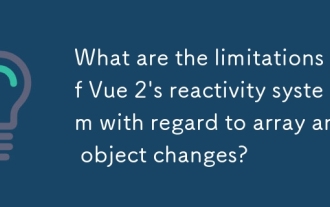
Vue 2's reactivity system struggles with direct array index setting, length modification, and object property addition/deletion. Developers can use Vue's mutation methods and Vue.set() to ensure reactivity.
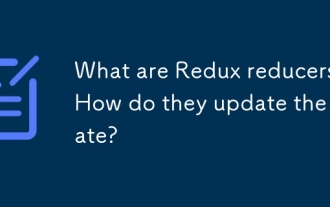
Redux reducers are pure functions that update the application's state based on actions, ensuring predictability and immutability.
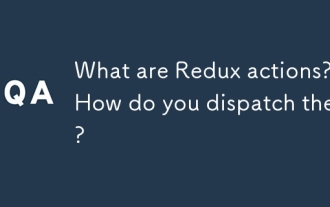
The article discusses Redux actions, their structure, and dispatching methods, including asynchronous actions using Redux Thunk. It emphasizes best practices for managing action types to maintain scalable and maintainable applications.
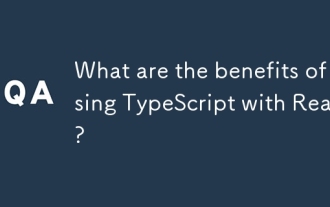
TypeScript enhances React development by providing type safety, improving code quality, and offering better IDE support, thus reducing errors and improving maintainability.

React components can be defined by functions or classes, encapsulating UI logic and accepting input data through props. 1) Define components: Use functions or classes to return React elements. 2) Rendering component: React calls render method or executes function component. 3) Multiplexing components: pass data through props to build a complex UI. The lifecycle approach of components allows logic to be executed at different stages, improving development efficiency and code maintainability.
