


The big model can 'write' papers by itself, with formulas and references. The trial version is now online
In recent years, with the advancement of research in various subject areas, scientific literature and data have exploded, making it increasingly difficult for academic researchers to discover useful insights from large amounts of information. Usually, people use search engines to obtain scientific knowledge, but search engines cannot organize scientific knowledge autonomously.
Now, a research team from Meta AI has proposed Galactica, a new large-scale language model that can store, combine and reason about scientific knowledge.
- Paper address: https://galactica.org/static/paper.pdf
- Trial address: https://galactica.org/
How powerful is the Galactica model? It can do it by itself Summarize and summarize a review paper:
You can also generate an encyclopedia query for the entry:
Give knowledgeable answers to the questions asked:
These tasks are still necessary for anthropologists A challenging task, but one that Galactica accomplished very well. Turing Award winner Yann LeCun also tweeted his praise:
Let’s take a look at the specific details of the Galactica model.
Model Overview
The Galactica model is trained on a large scientific corpus of papers, reference materials, knowledge bases and many other sources, including more than 48 million articles Papers, textbooks and handouts, knowledge on millions of compounds and proteins, scientific websites, encyclopedias and more. Unlike existing language models that rely on uncurated, web-crawler-based text, the corpus used for Galactica training is high quality and highly curated. This study trained the model for multiple epochs without overfitting, where performance on upstream and downstream tasks was improved by using repeated tokens.
Galactica outperforms existing models on a range of scientific tasks. On technical knowledge exploration tasks such as LaTeX equations, the performance of Galactica and GPT-3 is 68.2% VS 49.0%. Galactica also excels at inference, significantly outperforming Chinchilla on the mathematical MMLU benchmark.
Galactica also outperforms BLOOM and OPT-175B on BIG-bench despite not being trained on a common corpus. Additionally, it achieved new performance highs of 77.6% and 52.9% on downstream tasks such as PubMedQA and MedMCQA development.
Simply put, the research encapsulates step-by-step reasoning in special tokens to mimic the inner workings. This allows researchers to interact with models using natural language, as shown below in Galactica’s trial interface.
It is worth mentioning that in addition to text generation, Galactica can also perform multi-modal tasks involving chemical formulas and protein sequences. This will contribute to the field of drug discovery.
Implementation details
The corpus of this article contains 106 billion tokens, which come from papers, references, encyclopedias, and other scientific materials. It can be said that this research includes both natural language resources (papers, reference books) and sequences in nature (protein sequences, chemical forms). Details of the corpus are shown in Tables 1 and 2 .
Now that we have the corpus, the next step is how to operate the data. Generally speaking, the design of tokenization is very important. For example, if protein sequences are written in terms of amino acid residues, then character-based tokenization is appropriate. In order to achieve tokenization, this study performed specialized tokenization on different modalities. Specific examples include (including but not limited to):
- Reference: Use special reference tokens [START_REF] and [END_REF] to wrap references;
- Stepwise reasoning: Use working memory tokens to encapsulate stepwise reasoning and simulate the internal working memory context;
- Numbers: Divide numbers into separate tokens. For example, 737612.62 → 7,3,7,6,1,2,.,6,2;
- SMILES formula: wrap the sequence with [START_SMILES] and [END_SMILES] and apply Character-based tokenization. Likewise, this study uses [START_I_SMILES] and [END_I_SMILES] to represent isomeric SMILES. For example: C(C(=O)O)N→C, (,C,(,=,O,),O,),N;
- DNA sequence: Apply one A character-based tokenization that treats each nucleotide base as a token, where the starting tokens are [START_DNA] and [END_DNA]. For example, CGGTACCCTC→C, G, G, T, A, C, C, C, T, C.
# Figure 4 below shows an example of processing references to a paper. When handling references use global identifiers and the special tokens [START_REF] and [END_REF] to represent the place of the reference.
#After the data set is processed, the next step is how to implement it. Galactica has made the following modifications based on the Transformer architecture:
- GeLU activation: Use GeLU activation for models of various sizes;
- Context window: For models of different sizes, use a context window of length 2048;
- No bias: Follow PaLM, no bias is used in dense kernel or layer specifications;
- Learning location embedding: Learning location embedding is used for the model;
- Vocabulary: Use BPE to build a vocabulary containing 50k tokens.
Table 5 lists models of different sizes and training hyperparameters.
Experiment
Duplicate tokens are considered harmless
As can be seen from Figure 6, after four epochs of training, the verification loss continues to decrease. The model with 120B parameters only starts to overfit at the beginning of the fifth epoch. This is unexpected because existing research shows that duplicate tokens can be harmful to performance. The study also found that the 30B and 120B models exhibited a double-decline effect epoch-wise, where the validation loss plateaued (or rose), followed by a decline. This effect becomes stronger after each epoch, most notably for the 120B model at the end of training.
The results of Figure 8 show that there is no sign of overfitting in the experiment, which shows that repeated tokens can improve the performance of downstream and upstream tasks.
Other results
It’s too slow to type the formula, now use the prompt LaTeX can be generated:
In a chemical reaction, Galactica is required to predict the product of the reaction in the chemical equation LaTeX. The model can be based only on the reactants. Making inferences, the results are as follows:
Some additional results are reported in Table 7:
Galactica's reasoning abilities. The study is first evaluated on the MMLU mathematics benchmark and the evaluation results are reported in Table 8. Galactica performs strongly compared to the larger base model, and using tokens appears to improve Chinchilla's performance, even for the smaller 30B Galactica model.
The study also evaluated the MATH dataset to further explore Galactica’s inference capabilities:
It can be concluded from the experimental results that Galactica is much better than the basic PaLM model in terms of thinking chain and prompts. This suggests that Galactica is a better choice for handling mathematical tasks.
The evaluation results on downstream tasks are shown in Table 10. Galactica significantly outperforms other language models and outperforms larger models on most tasks (Gopher 280B). The difference in performance was larger compared to Chinchilla, which appeared to be stronger on a subset of tasks: particularly high school subjects and less mathematical, memory-intensive tasks. In contrast, Galactica tends to perform better on math and graduate-level tasks.
The study also evaluated Chinchilla’s ability to predict citations given input context, an assessment of Chinchilla’s ability to organize scientific literature. Important test. The results are as follows:
For more experimental content, please refer to the original paper.
The above is the detailed content of The big model can 'write' papers by itself, with formulas and references. The trial version is now online. For more information, please follow other related articles on the PHP Chinese website!

Hot AI Tools

Undresser.AI Undress
AI-powered app for creating realistic nude photos

AI Clothes Remover
Online AI tool for removing clothes from photos.

Undress AI Tool
Undress images for free

Clothoff.io
AI clothes remover

AI Hentai Generator
Generate AI Hentai for free.

Hot Article

Hot Tools

Notepad++7.3.1
Easy-to-use and free code editor

SublimeText3 Chinese version
Chinese version, very easy to use

Zend Studio 13.0.1
Powerful PHP integrated development environment

Dreamweaver CS6
Visual web development tools

SublimeText3 Mac version
God-level code editing software (SublimeText3)

Hot Topics


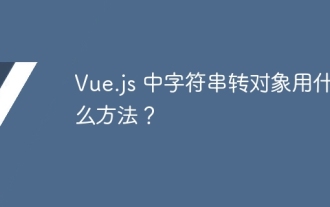
When converting strings to objects in Vue.js, JSON.parse() is preferred for standard JSON strings. For non-standard JSON strings, the string can be processed by using regular expressions and reduce methods according to the format or decoded URL-encoded. Select the appropriate method according to the string format and pay attention to security and encoding issues to avoid bugs.
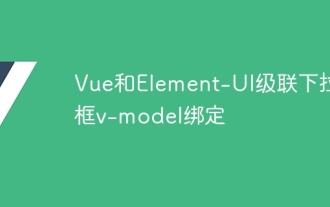
Vue and Element-UI cascaded drop-down boxes v-model binding common pit points: v-model binds an array representing the selected values at each level of the cascaded selection box, not a string; the initial value of selectedOptions must be an empty array, not null or undefined; dynamic loading of data requires the use of asynchronous programming skills to handle data updates in asynchronously; for huge data sets, performance optimization techniques such as virtual scrolling and lazy loading should be considered.
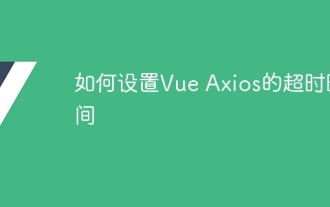
In order to set the timeout for Vue Axios, we can create an Axios instance and specify the timeout option: In global settings: Vue.prototype.$axios = axios.create({ timeout: 5000 }); in a single request: this.$axios.get('/api/users', { timeout: 10000 }).
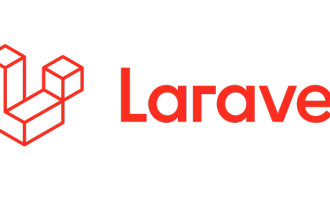
Efficiently process 7 million records and create interactive maps with geospatial technology. This article explores how to efficiently process over 7 million records using Laravel and MySQL and convert them into interactive map visualizations. Initial challenge project requirements: Extract valuable insights using 7 million records in MySQL database. Many people first consider programming languages, but ignore the database itself: Can it meet the needs? Is data migration or structural adjustment required? Can MySQL withstand such a large data load? Preliminary analysis: Key filters and properties need to be identified. After analysis, it was found that only a few attributes were related to the solution. We verified the feasibility of the filter and set some restrictions to optimize the search. Map search based on city
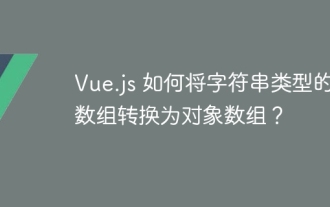
Summary: There are the following methods to convert Vue.js string arrays into object arrays: Basic method: Use map function to suit regular formatted data. Advanced gameplay: Using regular expressions can handle complex formats, but they need to be carefully written and considered. Performance optimization: Considering the large amount of data, asynchronous operations or efficient data processing libraries can be used. Best practice: Clear code style, use meaningful variable names and comments to keep the code concise.
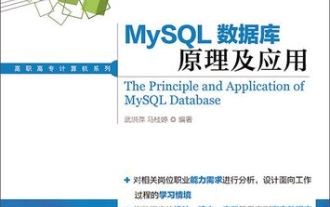
The article introduces the operation of MySQL database. First, you need to install a MySQL client, such as MySQLWorkbench or command line client. 1. Use the mysql-uroot-p command to connect to the server and log in with the root account password; 2. Use CREATEDATABASE to create a database, and USE select a database; 3. Use CREATETABLE to create a table, define fields and data types; 4. Use INSERTINTO to insert data, query data, update data by UPDATE, and delete data by DELETE. Only by mastering these steps, learning to deal with common problems and optimizing database performance can you use MySQL efficiently.
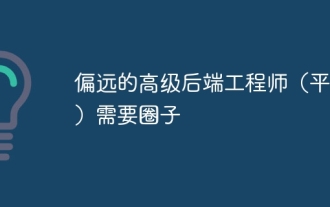
Remote Senior Backend Engineer Job Vacant Company: Circle Location: Remote Office Job Type: Full-time Salary: $130,000-$140,000 Job Description Participate in the research and development of Circle mobile applications and public API-related features covering the entire software development lifecycle. Main responsibilities independently complete development work based on RubyonRails and collaborate with the React/Redux/Relay front-end team. Build core functionality and improvements for web applications and work closely with designers and leadership throughout the functional design process. Promote positive development processes and prioritize iteration speed. Requires more than 6 years of complex web application backend
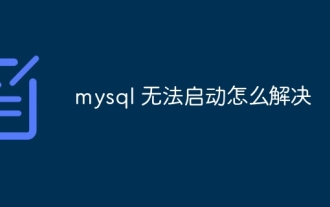
There are many reasons why MySQL startup fails, and it can be diagnosed by checking the error log. Common causes include port conflicts (check port occupancy and modify configuration), permission issues (check service running user permissions), configuration file errors (check parameter settings), data directory corruption (restore data or rebuild table space), InnoDB table space issues (check ibdata1 files), plug-in loading failure (check error log). When solving problems, you should analyze them based on the error log, find the root cause of the problem, and develop the habit of backing up data regularly to prevent and solve problems.
