


Beat OpenAI again! Google releases 2 billion parameter universal model to automatically recognize and translate more than 100 languages
Last week, OpenAI released the ChatGPT API and Whisper API, which just triggered a carnival among developers.
#On March 6, Google launched a benchmark model-USM. Not only can it support more than 100 languages, but the number of parameters has also reached 2 billion.
# Of course, the model is still not open to the public, "This is very Google"!
## Simply put, the USM model covers 12 million hours of speech and 28 billion sentences. It is pre-trained on an unlabeled dataset of 300 different languages and fine-tuned on a smaller labeled training set.
Google researchers said that although the annotation training set used for fine-tuning is only 1/7 of Whisper, USM has equivalent or even better results. performance, and also the ability to efficiently adapt to new languages and data.
##Paper address: https://arxiv.org/abs/2303.01037
The results show that USM not only achieves SOTA in multilingual automatic speech recognition and speech-text translation task evaluation, but can also be actually used in YouTube subtitle generation.
#Currently, the languages that support automatic detection and translation include mainstream English, Chinese, and small languages such as Assamese.
#The most important thing is that it can also be used for real-time translation of future AR glasses demonstrated by Google at last year’s IO conference.
##Jeff Dean personally announced: Let AI support 1,000 languages
In November last year, Google first announced a new project to "develop an artificial intelligence language model that supports the 1,000 most commonly used languages in the world."
#The release of the latest model is described by Google as a "critical step" towards its goal.
# When it comes to building language models, it can be said that there are many heroes competing.
According to rumors, Google plans to showcase more than 20 products powered by artificial intelligence at this year’s annual I/O conference.
Currently, automatic speech recognition faces many challenges:
- Traditional Supervised learning methods lack scalability
This requires algorithms that can use large amounts of data from different sources, enable model updates without the need for complete retraining, and be able to generalize to new languages and use cases . According to the paper, USM training uses three databases: unpaired audio data set, unpaired Text data set, paired ASR corpus. Includes YT-NTL-U (over 12 million hours of YouTube untagged audio data) and Pub-U (over 429,000 hours of speech content in 51 languages) Web-NTL (28 billion sentences in over 1140 different languages) YT-SUP and Pub-S corpora (over 10,000 hours of audio content and matching text)Fine-tuned self-supervised learning
USM uses a standard encoder-decoder structure, where the decoder can be CTC, RNN -T or LAS.
#For the encoder, USM uses Conformor, or convolution enhanced Transformer.
#The training process is divided into three stages.
#In the initial stage, unsupervised pre-training is performed using BEST-RQ (BERT-based random projection quantizer for speech pre-training). The goal is to optimize RQ.
#In the next stage, the speech representation learning model is further trained.
Use MOST (Multi-Object Supervised Pre-training) to integrate information from other text data.
The model introduces an additional encoder module that takes text as input and introduces additional layers to combine the speech encoder and text encoder output, and jointly train the model on unlabeled speech, labeled speech, and text data.
The last step is to fine-tune the ASR (automatic speech recognition) and AST (automatic speech translation) tasks. The pre-trained USM model only requires a small amount of Supervisory data can achieve good performance.
##USM overall training process
How does USM perform? Google tested it on YouTube subtitles, promotion of downstream ASR tasks, and automatic speech translation.
Performance on YouTube multi-language subtitles
Supervised YouTube data includes 73 languages, with an average of just under 3,000 hours of data per language. Despite limited supervision data, the model achieved an average word error rate (WER) of less than 30% across 73 languages, which is lower than state-of-the-art models within the United States.
In addition, Google compared it with the Whisper model (big-v2) trained with more than 400,000 hours of annotated data.
Among the 18 languages that Whisper can decode, its decoding error rate is less than 40%, while the average USM error rate is only 32.7%.
##Promotion of downstream ASR tasks
On publicly available datasets, USM shows lower performance on CORAAL (African American Dialect English), SpeechStew (English - United States), and FLEURS (102 languages) compared to Whisper WER, regardless of whether there is in-domain training data.
#The difference between the two models in FLEURS is particularly obvious.
##Performance on the AST task
Fine-tuning USM on the CoVoST dataset.
Divide the languages in the data set into three categories: high, medium, and low according to resource availability, and calculate the BLEU score on each category (the higher the better) , USM performs better than Whisper in every category.
Research has found that BEST-RQ pre-training is an effective way to extend speech representation learning to large data sets.
When combined with text injection in MOST, it improves the quality of downstream speech tasks, achieving state-of-the-art results on the FLEURS and CoVoST 2 benchmarks performance.
By training lightweight residual adapter modules, MOST represents the ability to quickly adapt to new domains. These remaining adapter modules only increase parameters by 2%.
Google said that currently, USM supports more than 100 languages and will expand to more than 100 languages in the future. More than 1000 languages. With this technology, it may be safe for everyone to travel around the world.
#Even, real-time translation of Google AR glasses products will attract many fans in the future.
#However, the application of this technology still has a long way to go.
#After all, in its speech at the IO conference facing the world, Google also wrote the Arabic text backwards, attracting many netizens to watch.
The above is the detailed content of Beat OpenAI again! Google releases 2 billion parameter universal model to automatically recognize and translate more than 100 languages. For more information, please follow other related articles on the PHP Chinese website!

Hot AI Tools

Undresser.AI Undress
AI-powered app for creating realistic nude photos

AI Clothes Remover
Online AI tool for removing clothes from photos.

Undress AI Tool
Undress images for free

Clothoff.io
AI clothes remover

Video Face Swap
Swap faces in any video effortlessly with our completely free AI face swap tool!

Hot Article

Hot Tools

Notepad++7.3.1
Easy-to-use and free code editor

SublimeText3 Chinese version
Chinese version, very easy to use

Zend Studio 13.0.1
Powerful PHP integrated development environment

Dreamweaver CS6
Visual web development tools

SublimeText3 Mac version
God-level code editing software (SublimeText3)

Hot Topics


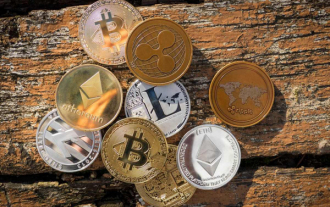
This article introduces the registration process of the Sesame Open Exchange (Gate.io) web version and the Gate trading app in detail. Whether it is web registration or app registration, you need to visit the official website or app store to download the genuine app, then fill in the user name, password, email, mobile phone number and other information, and complete email or mobile phone verification.
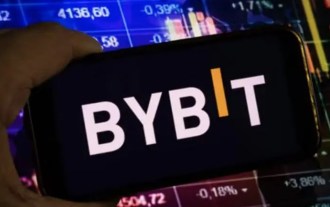
Why can’t the Bybit exchange link be directly downloaded and installed? Bybit is a cryptocurrency exchange that provides trading services to users. The exchange's mobile apps cannot be downloaded directly through AppStore or GooglePlay for the following reasons: 1. App Store policy restricts Apple and Google from having strict requirements on the types of applications allowed in the app store. Cryptocurrency exchange applications often do not meet these requirements because they involve financial services and require specific regulations and security standards. 2. Laws and regulations Compliance In many countries, activities related to cryptocurrency transactions are regulated or restricted. To comply with these regulations, Bybit Application can only be used through official websites or other authorized channels
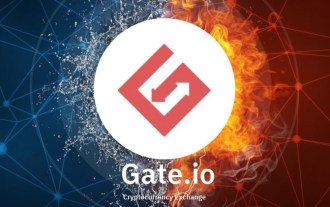
A detailed introduction to the login operation of the Sesame Open Exchange web version, including login steps and password recovery process. It also provides solutions to common problems such as login failure, unable to open the page, and unable to receive verification codes to help you log in to the platform smoothly.
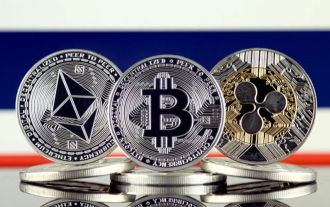
It is crucial to choose a formal channel to download the app and ensure the safety of your account.
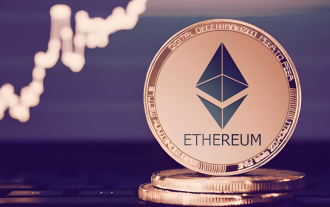
This article recommends the top ten cryptocurrency trading platforms worth paying attention to, including Binance, OKX, Gate.io, BitFlyer, KuCoin, Bybit, Coinbase Pro, Kraken, BYDFi and XBIT decentralized exchanges. These platforms have their own advantages in terms of transaction currency quantity, transaction type, security, compliance, and special features. For example, Binance is known for its largest transaction volume and abundant functions in the world, while BitFlyer attracts Asian users with its Japanese Financial Hall license and high security. Choosing a suitable platform requires comprehensive consideration based on your own trading experience, risk tolerance and investment preferences. Hope this article helps you find the best suit for yourself
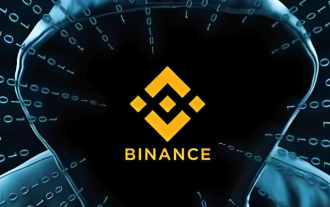
To access the latest version of Binance website login portal, just follow these simple steps. Go to the official website and click the "Login" button in the upper right corner. Select your existing login method. If you are a new user, please "Register". Enter your registered mobile number or email and password and complete authentication (such as mobile verification code or Google Authenticator). After successful verification, you can access the latest version of Binance official website login portal.
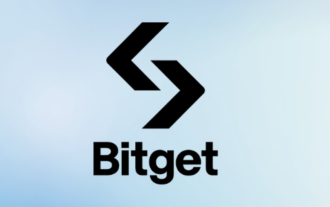
This guide provides detailed download and installation steps for the official Bitget Exchange app, suitable for Android and iOS systems. The guide integrates information from multiple authoritative sources, including the official website, the App Store, and Google Play, and emphasizes considerations during download and account management. Users can download the app from official channels, including app store, official website APK download and official website jump, and complete registration, identity verification and security settings. In addition, the guide covers frequently asked questions and considerations, such as
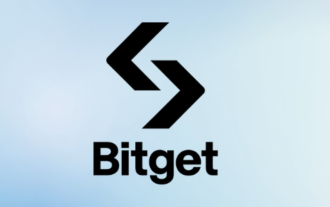
This guide provides detailed download and installation steps for the official Bitget Exchange app, suitable for Android and iOS systems. The guide integrates information from multiple authoritative sources, including the official website, the App Store, and Google Play, and emphasizes considerations during download and account management. Users can download the app from official channels, including app store, official website APK download and official website jump, and complete registration, identity verification and security settings. In addition, the guide covers frequently asked questions and considerations, such as
