Tesla's self-driving plan flips between sloppiness and stubbornness
Long before he became the new boss of Twitter, Musk was obsessed with making Tesla cars self-driving. The technology was quite expensive to develop, so when the supply chain began to collapse two years ago, Musk was determined to reduce costs. He targeted car radar sensors.
This sensor is designed to detect hazards at a distance and prevent the vehicle from hitting other cars while driving. There are now eight cameras mounted on the car, which can be used to see the road and spot hazards in every direction. Musk thinks that should be enough.
But according to multiple former employees, many Tesla engineers were shocked by this. They contacted a trusted former executive to try to persuade Musk to abandon this approach. Without radar, Tesla's vehicles would be prone to low-level perception errors when the camera is obscured by raindrops or even bright light, which could lead to a crash.
However, Musk did not seem convinced and he overruled the engineers' opinions. In May 2021, Tesla announced that it would remove radar from new cars. Soon after, the company began disabling radar in cars already on the road. Tesla cars that suddenly lose critical sensors are significantly more likely to crash and make other embarrassing mistakes, according to interviews with more than a dozen former employees, test drivers, safety officials and other experts.
Musk describes Tesla’s Full Self-Driving (FSD) technology as “the major difference between a Tesla being worth a lot of money or being worth essentially nothing,” but his self-driving car dream There were obviously obstacles.
Tesla has recalled and paused the rollout of the technology to eligible vehicles in recent weeks over concerns that its vehicles could violate speed limits and run through stop signs, according to U.S. officials. Customer complaints have piled up, including a lawsuit filed in court last month that said Musk exaggerated the technology's capabilities. Tesla's filings also show that regulators and government officials are scrutinizing Tesla's systems and its past statements as evidence of safety issues mounts.
In interviews, former employees who were involved in the development of Tesla’s driver-assist software attributed the company’s troubles to costs such as the speed of development and Musk’s decision to cancel the radar, which deviated from industry practice. cuts and other issues unique to Tesla. Additionally, Musk's erratic leadership style also played a role, forcing them to develop the technology at breakneck speed and push it to the public before it was ready. Some say even today they worry the software isn't safe enough for use on public roads.
John Bernal, a retired test operator who worked in Tesla's Autopilot department, said: "The system is progressing very slowly internally, but the public wants the company to release it as soon as possible." Bernal was fired in February 2022 when Tesla accused him of improperly using the technology after releasing a video of FSD.
Musk acquired the troubled social media platform Twitter last fall with great fanfare and mobilized dozens of Tesla engineers to help work on Twitter’s code, according to people familiar with the matter. Earlier this month, Tesla shares fell 6% after the company failed to announce major new products at its investor day.
Musk defended Tesla’s actions, saying it was a long-term bet that promised to unlock huge value. Tesla also said that vehicles with FSD software activated are at least five times less likely to be involved in a crash than vehicles driven normally. Musk and Tesla did not respond to repeated requests for comment.
But FSD’s story provides a vivid example of how the billionaire made it happen through rash decision-making, a stubborn insistence on doing things differently and an unwavering faith in an as-yet-unproven vision. One of the biggest bets gets complicated.
Patchwork solutions make it feel like technology is progressing
In April 2019, at a presentation called "Autonomous Investor Day", Musk made made perhaps his boldest prediction as Tesla CEO. He told investors at the time: "By the middle of next year, we will have more than 1 million Tesla vehicles equipped with fully autonomous driving hardware on the road. Our software will be automatically updated over the air, and FSD will be so reliable that drivers can even Sleeping in the car."
Investors were thrilled, and Tesla shares soared in 2020, making it the most valuable automaker and helping Musk become the world's richest man. After Autopilot, FDS was launched in 2014 and later allowed cars to drive autonomously on highways, steering, changing lanes and adjusting speed automatically. FSD aims to bring these features to city and residential streets, although this is a much more difficult task.
To achieve the above goals, automotive hardware and software need to be combined. Eight cameras are used to capture real-time footage of activity around the car, which allows the car to assess hazards such as pedestrians or cyclists and react accordingly. To deliver on his promise, Musk assembled a team of star engineers who were willing to work long hours and stay up late to solve problems. Musk is willing to test the latest software on his own cars and write "fix" requests for engineers with other executives.
Some ex-employees said the patchwork of solutions gave the illusion of continued technological progress but masked the lack of a coherent development strategy. While rivals such as Alphabet's self-driving car Waymo adopted strict testing protocols that limited the scope of its self-driving software, Tesla ultimately rolled out FSD to its 360,000 owners and left it to them to decide whether to activate it.
Tesla’s philosophy is simple: The more data the AI that guides the car is exposed to, the faster it learns. But this rough model also means that security is looser. Former Tesla employees say the company has chosen to let the software effectively learn on its own, developing brain-like agility through a rule-less technique called "neural networks." While this has the potential to speed up the training process, ultimately it is essentially a trial and error approach.
Competitors such as Waymo and Apple have adopted different autonomous approaches, setting the rules and addressing any violations if those restrictions are violated, according to Silicon Valley insiders familiar with the company's practices. Companies developing self-driving technology also often use sophisticated lidar and radar systems, which help software map their surroundings in detail.
Waymo spokesperson Julia Ilina said there are clear differences in the practices of the two companies. She said Waymo’s goal is to achieve complete autonomy and emphasize machine learning. Apple declined to comment.
Tesla’s approach has proven problematic many times. About two years ago, someone posted a video of the software struggling to navigate San Francisco's winding Lombard Street, and the video garnered tens of thousands of views. Bernal revealed that Tesla engineers built invisible barriers into the software, similar to bumpers in a bowling alley, to help the car stay on the road. A subsequent video shows the software running smoothly.
This made Bernal confused. As an in-house tester, it's part of his job to get behind the wheel on this stretch of road, and it's clear this is far from his typical experience on other public streets.
Radar originally played an important role in the design of Tesla vehicles and software, complementing cameras by providing a realistic view of the surrounding environment, especially in situations where vision may be obstructed. Tesla also uses ultrasonic sensors, which are short-range devices that can detect obstacles within a few centimeters around the car.
Even with radar, Tesla vehicles are not as sophisticated as other rival vehicles that use lidar. “One of the key advantages of lidar is that it can always spot a train or truck in advance, even if it’s No idea what that is. It knows there's something ahead and the vehicle can stop in time without knowing any more."
Cameras need to understand what they're seeing to be effective, relies on Tesla Workers label images recorded by vehicles, including stop signs and trains, to help the software know how to react.
Former Tesla employees said that at the end of 2020, Autopilot employees turned on their computers and discovered that workplace monitoring software had been installed within the company. The software monitors keystrokes and mouse clicks and tracks their image tags. If the mouse doesn't move for a period of time, a timer starts and the employee can be reprimanded until fired.
Last month, a group pushing for unionization at Tesla’s Buffalo plant raised concerns about workplace surveillance, and Tesla issued a response. The company said: "The reason for time monitoring of image tagging is to improve the ease of use of our tagging software. Its purpose is to calculate how long it takes to tag an image."
Musk once advocated A "visual only" navigation method because it's simpler, cheaper, and more intuitive. In February 2022, he wrote on Twitter: "Road systems are designed for cameras (eyes) and neural networks (brains)."
But many believe there are risks with this approach. A former Tesla Autopilot engineer said: "I just know that it is unsafe to use that software on the street. You can't predict what the car will do."
Remove Radar Leading to an increase in crashes
These former employees said the problems were noticed almost immediately after Tesla announced the removal of the radar in May 2021. During this time, the FSD testing program expanded from thousands to tens of thousands of drivers. Suddenly, Tesla vehicles were allegedly stopping for imagined dangers, misreading road signs and even failing to detect obstacles such as emergency vehicles, according to complaints filed with regulators.
Some people attribute the increase in "phantom braking" accidents in Tesla vehicles to a lack of radar. Data from the U.S. National Highway Traffic Safety Administration (NHTSA) shows that traffic accidents involving Tesla vehicles surged last year. Complaints about "phantom braking" have risen to 107 in the past three months, compared with 34 in the previous 22 months. NHTSA received about 250 complaints about the issue over a two-week period, and the agency launched an investigation after receiving 354 related complaints over a nine-month period.
Several months ago, NHTSA launched an investigation into Autopilot over about a dozen reports of Teslas crashing into stationary emergency vehicles. The latest example came to light this month, when the agency confirmed it was investigating a fatal crash in February involving a Tesla and a fire truck. Experts say radar can double-check what cameras see, since cameras are easily affected by bright light.
Missy Cummings, former NHTSA senior safety adviser, said: "This is not the only reason Tesla vehicles are in trouble, but it is an important reason. Radar can help detect The object in front. For computer vision with large errors, it can be used as a sensor to fuse it to check if there is a problem."
As the chief tester, Musk also requires frequent bug fixes for the software, Ask engineers to step in and adjust the code. One former executive recalled what an engineer who worked on the project told him: "No one can come up with a good idea when they're being chased by a tiger." An attitude of acceptance leads to a culture of conformity. Tesla fires employees who oppose Musk. The company has also pushed out so many software updates that in late 2021, NHTSA publicly warned Tesla not to release fixes without a formal recall notice.
Tesla and Twitter employees said Musk’s decision to acquire Twitter was a distraction. Many interviews with former employees and documents show that Musk asked dozens of Tesla engineers to help take over Twitter after the acquisition was completed last year. Software updates that were supposed to be released every two weeks are suddenly months apart as Tesla works to overcome bugs and chase more ambitious goals.
Some people lamented Musk's takeover of Twitter, saying he needed to refocus on Tesla to finish what he started. Tesla investor Ross Gerber said: "FSD bodes well for Tesla's bright future. We love Musk, he is an innovator of our time. We just want to see him come back wholeheartedly again To Tesla.”
Future full of uncertainty and facing multiple investigationsTesla engineers are exhausted and are resigning to look for opportunities elsewhere . Tesla AI director Andrej Karpathy took a month-long sabbatical last year and then chose to leave to join OpenAI, the company behind the chatbot ChatGPT. Meanwhile, Tesla Autopilot director Ashok Elluswamy has gone to work at Twitter.
As part of the ongoing investigation, the U.S. Department of Justice has requested documents related to FSD from Tesla. The U.S. Securities and Exchange Commission (SEC) is also looking into Musk's role in promoting Tesla's autonomous driving as part of a larger investigation.
In the lawsuit filed in February, Tesla was accused of making "false and misleading" statements that "significantly exaggerated" the safety and performance of Autopilot and FSD. That doesn’t include NHTSA’s two investigations into Autopilot, one into crashing emergency vehicles and another into “phantom braking.”
At this month’s Investor Day event, Musk appeared on stage with a dozen Tesla executives to tout the company’s extensive expertise. But the company didn't provide any major progress on FSD, despite having a section on the technology.
Many of Musk’s loyal customers have given up hope that his original promises will come true. Charles Cook, a commercial pilot and engineer from Jacksonville, Florida, owns a Model Y that he frequently drives with FSD activated.
While Cook was amazed by the technology’s capabilities, he was dissatisfied with its slow progress and the delay in delivering on Musk’s promises. He said: "Some people may have purchased the FSD software, thinking that they will now have a fully self-driving taxi, and then spent their hard-earned money on it. But now, Musk's engineers may be concerned about this Scoff. Some people probably spent $15,000 thinking they could have it next year and now they're disappointed." (小小)
The above is the detailed content of Tesla's self-driving plan flips between sloppiness and stubbornness. For more information, please follow other related articles on the PHP Chinese website!

Hot AI Tools

Undresser.AI Undress
AI-powered app for creating realistic nude photos

AI Clothes Remover
Online AI tool for removing clothes from photos.

Undress AI Tool
Undress images for free

Clothoff.io
AI clothes remover

AI Hentai Generator
Generate AI Hentai for free.

Hot Article

Hot Tools

Notepad++7.3.1
Easy-to-use and free code editor

SublimeText3 Chinese version
Chinese version, very easy to use

Zend Studio 13.0.1
Powerful PHP integrated development environment

Dreamweaver CS6
Visual web development tools

SublimeText3 Mac version
God-level code editing software (SublimeText3)

Hot Topics


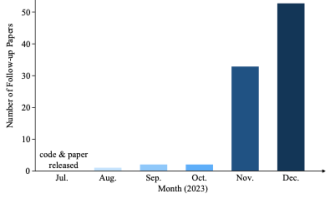
Written above & the author’s personal understanding Three-dimensional Gaussiansplatting (3DGS) is a transformative technology that has emerged in the fields of explicit radiation fields and computer graphics in recent years. This innovative method is characterized by the use of millions of 3D Gaussians, which is very different from the neural radiation field (NeRF) method, which mainly uses an implicit coordinate-based model to map spatial coordinates to pixel values. With its explicit scene representation and differentiable rendering algorithms, 3DGS not only guarantees real-time rendering capabilities, but also introduces an unprecedented level of control and scene editing. This positions 3DGS as a potential game-changer for next-generation 3D reconstruction and representation. To this end, we provide a systematic overview of the latest developments and concerns in the field of 3DGS for the first time.
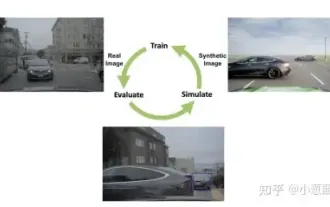
Yesterday during the interview, I was asked whether I had done any long-tail related questions, so I thought I would give a brief summary. The long-tail problem of autonomous driving refers to edge cases in autonomous vehicles, that is, possible scenarios with a low probability of occurrence. The perceived long-tail problem is one of the main reasons currently limiting the operational design domain of single-vehicle intelligent autonomous vehicles. The underlying architecture and most technical issues of autonomous driving have been solved, and the remaining 5% of long-tail problems have gradually become the key to restricting the development of autonomous driving. These problems include a variety of fragmented scenarios, extreme situations, and unpredictable human behavior. The "long tail" of edge scenarios in autonomous driving refers to edge cases in autonomous vehicles (AVs). Edge cases are possible scenarios with a low probability of occurrence. these rare events
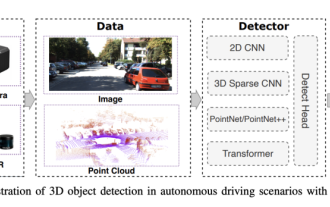
0.Written in front&& Personal understanding that autonomous driving systems rely on advanced perception, decision-making and control technologies, by using various sensors (such as cameras, lidar, radar, etc.) to perceive the surrounding environment, and using algorithms and models for real-time analysis and decision-making. This enables vehicles to recognize road signs, detect and track other vehicles, predict pedestrian behavior, etc., thereby safely operating and adapting to complex traffic environments. This technology is currently attracting widespread attention and is considered an important development area in the future of transportation. one. But what makes autonomous driving difficult is figuring out how to make the car understand what's going on around it. This requires that the three-dimensional object detection algorithm in the autonomous driving system can accurately perceive and describe objects in the surrounding environment, including their locations,
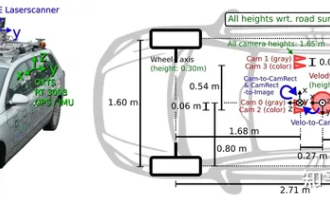
The first pilot and key article mainly introduces several commonly used coordinate systems in autonomous driving technology, and how to complete the correlation and conversion between them, and finally build a unified environment model. The focus here is to understand the conversion from vehicle to camera rigid body (external parameters), camera to image conversion (internal parameters), and image to pixel unit conversion. The conversion from 3D to 2D will have corresponding distortion, translation, etc. Key points: The vehicle coordinate system and the camera body coordinate system need to be rewritten: the plane coordinate system and the pixel coordinate system. Difficulty: image distortion must be considered. Both de-distortion and distortion addition are compensated on the image plane. 2. Introduction There are four vision systems in total. Coordinate system: pixel plane coordinate system (u, v), image coordinate system (x, y), camera coordinate system () and world coordinate system (). There is a relationship between each coordinate system,
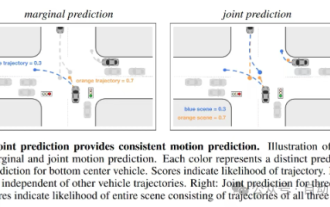
Trajectory prediction plays an important role in autonomous driving. Autonomous driving trajectory prediction refers to predicting the future driving trajectory of the vehicle by analyzing various data during the vehicle's driving process. As the core module of autonomous driving, the quality of trajectory prediction is crucial to downstream planning control. The trajectory prediction task has a rich technology stack and requires familiarity with autonomous driving dynamic/static perception, high-precision maps, lane lines, neural network architecture (CNN&GNN&Transformer) skills, etc. It is very difficult to get started! Many fans hope to get started with trajectory prediction as soon as possible and avoid pitfalls. Today I will take stock of some common problems and introductory learning methods for trajectory prediction! Introductory related knowledge 1. Are the preview papers in order? A: Look at the survey first, p

Original title: SIMPL: ASimpleandEfficientMulti-agentMotionPredictionBaselineforAutonomousDriving Paper link: https://arxiv.org/pdf/2402.02519.pdf Code link: https://github.com/HKUST-Aerial-Robotics/SIMPL Author unit: Hong Kong University of Science and Technology DJI Paper idea: This paper proposes a simple and efficient motion prediction baseline (SIMPL) for autonomous vehicles. Compared with traditional agent-cent
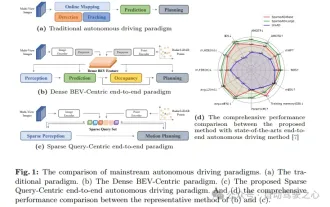
Written in front & starting point The end-to-end paradigm uses a unified framework to achieve multi-tasking in autonomous driving systems. Despite the simplicity and clarity of this paradigm, the performance of end-to-end autonomous driving methods on subtasks still lags far behind single-task methods. At the same time, the dense bird's-eye view (BEV) features widely used in previous end-to-end methods make it difficult to scale to more modalities or tasks. A sparse search-centric end-to-end autonomous driving paradigm (SparseAD) is proposed here, in which sparse search fully represents the entire driving scenario, including space, time, and tasks, without any dense BEV representation. Specifically, a unified sparse architecture is designed for task awareness including detection, tracking, and online mapping. In addition, heavy
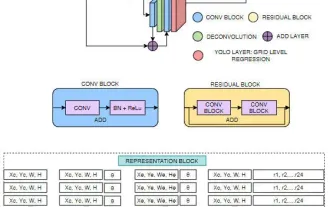
Target detection is a relatively mature problem in autonomous driving systems, among which pedestrian detection is one of the earliest algorithms to be deployed. Very comprehensive research has been carried out in most papers. However, distance perception using fisheye cameras for surround view is relatively less studied. Due to large radial distortion, standard bounding box representation is difficult to implement in fisheye cameras. To alleviate the above description, we explore extended bounding box, ellipse, and general polygon designs into polar/angular representations and define an instance segmentation mIOU metric to analyze these representations. The proposed model fisheyeDetNet with polygonal shape outperforms other models and simultaneously achieves 49.5% mAP on the Valeo fisheye camera dataset for autonomous driving
