


Machine learning is unlocking the mysteries of the universe in surprising ways
Space travel, exploration and observation often involve a series of the most complex and dangerous scientific and technological operations in human history. In these areas, artificial intelligence (AI) has proven itself to be a powerful assistant.
That’s why astronauts, scientists, and others whose mission is to explore and document the ultimate frontier are turning to machine learning (ML) to help deal with the challenges they face. Extraordinary challenge.
From guiding rockets through space to studying the surfaces of distant planets, to measuring the size of the universe and calculating the motion trajectories of celestial bodies, AI has many interesting and exciting application scenarios in space.
Space Voyage
During the take-off and landing process of a spacecraft, AI can automate engine operations and manage the actual deployment of functions such as landing gear, thereby optimizing the distribution and use of fuel.
SpaceX used the AI pilot system to achieve autonomous operation of its Falcon 9 spacecraft, and successfully docked with the International Space Station (ISS) in accordance with the cargo delivery contract signed with NASA. The system is able to calculate a rocket's trajectory through space, taking into account fuel use, atmospheric disturbances and liquid "sloshing" inside the engine.
CIMON 2 is a robot designed by Airbus, which is equivalent to a mobile Amazon Alexa virtual assistant next to astronauts. Built using the IBM Watson AI system, it uses an internal fan to propel itself forward and can act as a hands-free information database, computer and camera. It can even assess the mood and state of mind of astronauts by analyzing stress levels in their voices.
Mission planners at NASA’s Jet Propulsion Laboratory use AI to model and evaluate various mission parameters to understand the potential outcomes of different options and courses of action. These experiments can provide guidance information for future spacecraft design and engineering operations. The data collected can also be used for forward planning of a number of hypothetical future missions, including landings on Venus and Europa, the icy moon orbiting Jupiter.
SpaceX also uses AI algorithms to ensure that its Starlink satellites do not collide with other orbiting or transition vehicles in space. Their autonomous navigation system can detect nearby hazards in real time and adjust the satellite's speed and orbit to take evasive action.
The UK Space Agency has also developed autonomous systems that allow its spacecraft and satellites to avoid space debris through autonomous actions. By 2025, the British Space Agency plans to launch an autonomous spacecraft on this basis with the mission of capturing and cleaning up space debris. If not proactively controlled, space debris is likely to pose a threat to future spaceflight.
Planet Exploration
Mars rovers are robots designed to explore the surface of Mars. We can analyze and learn from the data they send back to Earth. Thanks to machine learning algorithms, these robots can navigate autonomously on the Martian surface, avoiding deep pits and steep walls that could damage or immobilize their hardware. The Spirit rover previously sent to Mars was stuck on the spot when its wheels got stuck in soft soil. NASA finally decided to give up rescue and contact in 2011. With the help of machine learning technology, NASA has successfully avoided the unexpected loss of another rover.
In recent years, NASA's Jet Propulsion Laboratory has used image recognition tools to study images taken by ground robots such as Mars rovers and classify terrain features. They even discovered a crater on the surface of Mars only four meters in diameter.
The Perseverance rover is equipped with a computer vision system called AEGIS that can detect and classify different rock types found on the surface of Mars, allowing us to learn more about the geological composition of the red planet.
You can even participate in training the AI algorithm used by the Mars rover at home. The AI4Mars project invites users to download tools to improve the autonomous navigation system on the Curiosity rover by marking terrain features on their personal computers.
While most surface exploration so far has been accomplished with wheeled robots, the European Space Agency is experimenting with the use of "hopping" robots. These robots can use their legs to move forward and jump. AI algorithms will coordinate the movement and balance of the robot's limbs to explore previously inaccessible locations on the moon, such as the Aristarchus Plateau on the moon, which was formed by a huge crater.
People have begun to use AI to detect the lunar surface and determine the best landing sites for future manned missions. This also helps astronauts fully understand the environment in which they will land in the future, and they do not have to face huge risks like the first generation of lunar lander such as Armstrong.
Charting the Universe
Astronomers are using AI to identify patterns in star clusters in distant nebulae, combined with other classified features detected in deep space to map the universe.
Take NASA's Kepler telescope as an example. It can determine the possible location of a planet by analyzing the attenuation of light radiation emitted by a star.
AI is also used to predict the activity of stars and galaxies, helping us understand the potential locations of cosmic events such as supernova explosions.
By analyzing the timing of the gravitational waves produced when these mysterious objects collide with neutron stars, researchers have detected the existence of dozens of black holes.
AI technology is also used to overlook the earth and the entire universe. The Autonomous Sciencecraft Experiment project, which began operation in 2004, is connected to the Earth Prediction 1 satellite, allowing it to automatically classify images captured by cameras, and then determine which images are more worthy of spending precious bandwidth transmission Return to Earth.
The SETI@Home project at the University of California, Berkeley, uses AI algorithms to process large amounts of data generated by radio telescopes, hoping to search for signs of extraterrestrial intelligence in space. Although the project has stopped sending new data to volunteers for inspection, there is still a large amount of data that has not been analyzed and retrieved, so the exciting truth may lie in this material!
AI was also used to create the most accurate image of a black hole to date. Roger Penrose, Reinhard Genzel and Andrea Ghez won the 2020 Nobel Prize for creating realistic images of the supermassive black hole at the center of the M87 galaxy.
The application scope of AI goes far beyond this. Researchers now hope to go beyond the event horizon and use AI technology to reveal what is going on inside a black hole. The work will also involve quantum computing and is expected to help physicists solve one of the most central problems in the field - unifying Einstein's general theory of relativity with the Standard Model of particle physics.
People even hope that AI can help measure the universe and better grasp its size and shape. Using an AI supercomputer to study astronomical data from Japan, we have successfully created a simulated star map that matches the known existence of the universe. This means we can predict the characteristics of the universe and move beyond the current boundaries of exploration that are hampered by the speed of light limit (i.e., the observable universe).
The above is the detailed content of Machine learning is unlocking the mysteries of the universe in surprising ways. For more information, please follow other related articles on the PHP Chinese website!

Hot AI Tools

Undresser.AI Undress
AI-powered app for creating realistic nude photos

AI Clothes Remover
Online AI tool for removing clothes from photos.

Undress AI Tool
Undress images for free

Clothoff.io
AI clothes remover

Video Face Swap
Swap faces in any video effortlessly with our completely free AI face swap tool!

Hot Article

Hot Tools

Notepad++7.3.1
Easy-to-use and free code editor

SublimeText3 Chinese version
Chinese version, very easy to use

Zend Studio 13.0.1
Powerful PHP integrated development environment

Dreamweaver CS6
Visual web development tools

SublimeText3 Mac version
God-level code editing software (SublimeText3)

Hot Topics


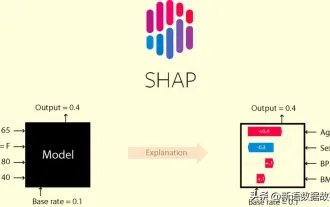
In the fields of machine learning and data science, model interpretability has always been a focus of researchers and practitioners. With the widespread application of complex models such as deep learning and ensemble methods, understanding the model's decision-making process has become particularly important. Explainable AI|XAI helps build trust and confidence in machine learning models by increasing the transparency of the model. Improving model transparency can be achieved through methods such as the widespread use of multiple complex models, as well as the decision-making processes used to explain the models. These methods include feature importance analysis, model prediction interval estimation, local interpretability algorithms, etc. Feature importance analysis can explain the decision-making process of a model by evaluating the degree of influence of the model on the input features. Model prediction interval estimate
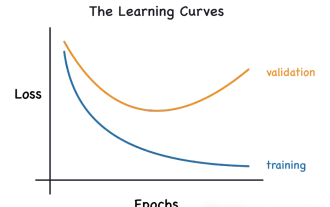
This article will introduce how to effectively identify overfitting and underfitting in machine learning models through learning curves. Underfitting and overfitting 1. Overfitting If a model is overtrained on the data so that it learns noise from it, then the model is said to be overfitting. An overfitted model learns every example so perfectly that it will misclassify an unseen/new example. For an overfitted model, we will get a perfect/near-perfect training set score and a terrible validation set/test score. Slightly modified: "Cause of overfitting: Use a complex model to solve a simple problem and extract noise from the data. Because a small data set as a training set may not represent the correct representation of all data." 2. Underfitting Heru
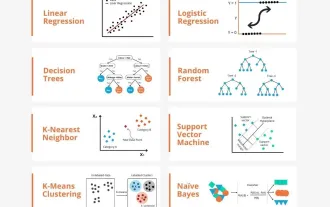
In layman’s terms, a machine learning model is a mathematical function that maps input data to a predicted output. More specifically, a machine learning model is a mathematical function that adjusts model parameters by learning from training data to minimize the error between the predicted output and the true label. There are many models in machine learning, such as logistic regression models, decision tree models, support vector machine models, etc. Each model has its applicable data types and problem types. At the same time, there are many commonalities between different models, or there is a hidden path for model evolution. Taking the connectionist perceptron as an example, by increasing the number of hidden layers of the perceptron, we can transform it into a deep neural network. If a kernel function is added to the perceptron, it can be converted into an SVM. this one
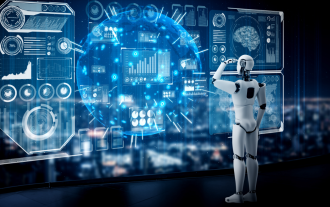
In the 1950s, artificial intelligence (AI) was born. That's when researchers discovered that machines could perform human-like tasks, such as thinking. Later, in the 1960s, the U.S. Department of Defense funded artificial intelligence and established laboratories for further development. Researchers are finding applications for artificial intelligence in many areas, such as space exploration and survival in extreme environments. Space exploration is the study of the universe, which covers the entire universe beyond the earth. Space is classified as an extreme environment because its conditions are different from those on Earth. To survive in space, many factors must be considered and precautions must be taken. Scientists and researchers believe that exploring space and understanding the current state of everything can help understand how the universe works and prepare for potential environmental crises
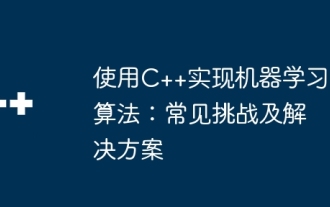
Common challenges faced by machine learning algorithms in C++ include memory management, multi-threading, performance optimization, and maintainability. Solutions include using smart pointers, modern threading libraries, SIMD instructions and third-party libraries, as well as following coding style guidelines and using automation tools. Practical cases show how to use the Eigen library to implement linear regression algorithms, effectively manage memory and use high-performance matrix operations.
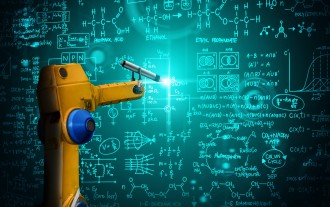
Machine learning is an important branch of artificial intelligence that gives computers the ability to learn from data and improve their capabilities without being explicitly programmed. Machine learning has a wide range of applications in various fields, from image recognition and natural language processing to recommendation systems and fraud detection, and it is changing the way we live. There are many different methods and theories in the field of machine learning, among which the five most influential methods are called the "Five Schools of Machine Learning". The five major schools are the symbolic school, the connectionist school, the evolutionary school, the Bayesian school and the analogy school. 1. Symbolism, also known as symbolism, emphasizes the use of symbols for logical reasoning and expression of knowledge. This school of thought believes that learning is a process of reverse deduction, through existing
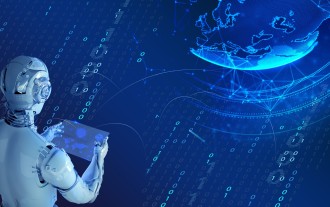
Translator | Reviewed by Li Rui | Chonglou Artificial intelligence (AI) and machine learning (ML) models are becoming increasingly complex today, and the output produced by these models is a black box – unable to be explained to stakeholders. Explainable AI (XAI) aims to solve this problem by enabling stakeholders to understand how these models work, ensuring they understand how these models actually make decisions, and ensuring transparency in AI systems, Trust and accountability to address this issue. This article explores various explainable artificial intelligence (XAI) techniques to illustrate their underlying principles. Several reasons why explainable AI is crucial Trust and transparency: For AI systems to be widely accepted and trusted, users need to understand how decisions are made
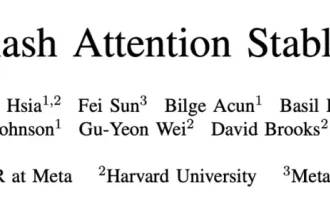
MetaFAIR teamed up with Harvard to provide a new research framework for optimizing the data bias generated when large-scale machine learning is performed. It is known that the training of large language models often takes months and uses hundreds or even thousands of GPUs. Taking the LLaMA270B model as an example, its training requires a total of 1,720,320 GPU hours. Training large models presents unique systemic challenges due to the scale and complexity of these workloads. Recently, many institutions have reported instability in the training process when training SOTA generative AI models. They usually appear in the form of loss spikes. For example, Google's PaLM model experienced up to 20 loss spikes during the training process. Numerical bias is the root cause of this training inaccuracy,
