ChatGPT has a dual impact on computing power
ChatGPT has been a hot topic in recent months. Behind its continued popularity is the rising demand for computing power for automatic generation of artificial intelligence content. On the one hand, ChatGPT has driven the growth in demand for computing power. On the other hand, ChatGPT has put forward higher requirements for computing power support.
Data shows that the total computing power consumption required for a ChatGPT model training is 3640PF-days, costing about 12 million U.S. dollars, and also requires considerable overhead during operation. . According to the Guosheng Securities report, based on the average number of unique visitors to ChatGPT in January of 13 million, the corresponding chip demand is more than 30,000 NVIDIA A100 GPUs, the approximate computing power cost is 800 million US dollars, and the daily electricity cost is 50,000 US dollars. Around USD. ChatGPT has received a US$13 billion investment from Microsoft, which is the confidence support behind its high computing power investment. It is precisely with the full support of the technology giant Microsoft from funds, future markets, to computing infrastructure (Azure cloud) that OpenAI can complete such a huge project as an independent entrepreneurial enterprise. Computing power requirements are closely related to parameter magnitude. Parameter magnitude is also an important reference for currently measuring the quality of large model training. The larger the number of parameters, the smarter the model, and the greater the overhead that comes with it. This forms the characteristic of high threshold for GPT type. The previous version of GPT-3 was open source, making it easier for other entrepreneurs to carry out new research and development work based on it. Starting from GPT-4, OpenAI open API charges based on byte traffic. Whether it is application development or secondary model development based on this, there is a high threshold.
The more interesting thing is that Meta’s LLaMA source code was leaked. As a result, the so-called "Llama Family" ("Llama" can be translated as "Alpaca") large model emerged. Developers who want to obtain open source resources can get a new option besides GPT-3.
The "large model"'s high dependence on computing power has at least two major impacts.
First, this is no longer a game of "grassroots" entrepreneurship, nor is it a game of "Copy To China" for Internet entrepreneurship in the past, but a competition of core capabilities of "real swords and real guns", from capital, technology to Comprehensive assessment of corporate strategic determination. In the traditional industrial era, China started from the processing industry and gradually moved up to the research and development of high-value core technologies. However, in fact, it is still a certain distance from the most advanced level in Europe and the United States. Especially in categories such as materials science that require long-term technical accumulation, the gap is still large.
Entering the digital era, the consensus among Chinese industry circles has become to start directly with core technology research and development.
It has become a trend for the United States and China to become less dependent on each other in science and technology. Although it is only the chip industry that is being constrained now, if "big models" develop into a factor of production, we will definitely encounter the same problem. Therefore, China must have its own "big model" core technology. Seeing this inevitability, Chinese technology companies have also become a trend to invest heavily in “big models”.
If small and medium-sized technology companies do not have the ability to develop native "big models", they can use the open source models of GPT-3 or LLaMA for development or secondary development to form their own "big models". Application development companies rarely develop native "big models". Unless they have extremely high confidence in the sales market of their applications, they will succumb to huge computing power cost pressures and instead pay API usage fees to develop related applications using ready-made models. .
Secondly, the construction of “Counting in the East and Counting in the West” comes at the right time and may usher in big business opportunities.
The western region is rich in "green power" resources. Under the overall plan of "Eastern Digital and Western Computing", the western hub is mainly responsible for backup and storage; the eastern region is responsible for real-time computing in smart cities, industrial Internet and Internet services. The work will be settled at the hub in the eastern region. The "big model" has huge requirements for computing power. Eastern IDC occupies computing power resources on a large scale, which has three major disadvantages: high cost (high electricity bills in the east), high emissions (the proportion of green electricity in the east is small), and squeezing out computing power for applications with low latency requirements. resources, which creates new business opportunities for computing power centers in the west.
On the one hand, placing the training calculations of "large models" in IDCs in the western region has higher requirements for improving the performance of local communication networks. The throughput of massive data is a problem that needs to be solved, but the cost of solving this problem is far less than the difference in IDC usage fees.
On the other hand, technology company giants have all put forward their own ESG (environmental social responsibility) goals, and carbon emissions are the most important indicator. The operation of "large models" and large computing power will produce a large amount of carbon emissions, and the demand for "green computing power" will become more urgent.
The above is the detailed content of ChatGPT has a dual impact on computing power. For more information, please follow other related articles on the PHP Chinese website!

Hot AI Tools

Undresser.AI Undress
AI-powered app for creating realistic nude photos

AI Clothes Remover
Online AI tool for removing clothes from photos.

Undress AI Tool
Undress images for free

Clothoff.io
AI clothes remover

AI Hentai Generator
Generate AI Hentai for free.

Hot Article

Hot Tools

Notepad++7.3.1
Easy-to-use and free code editor

SublimeText3 Chinese version
Chinese version, very easy to use

Zend Studio 13.0.1
Powerful PHP integrated development environment

Dreamweaver CS6
Visual web development tools

SublimeText3 Mac version
God-level code editing software (SublimeText3)

Hot Topics


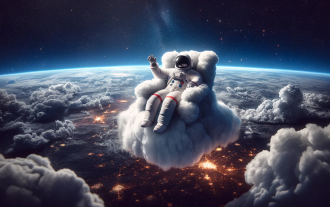
DALL-E 3 was officially introduced in September of 2023 as a vastly improved model than its predecessor. It is considered one of the best AI image generators to date, capable of creating images with intricate detail. However, at launch, it was exclus
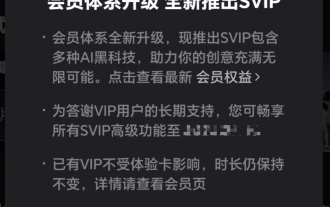
This site reported on June 27 that Jianying is a video editing software developed by FaceMeng Technology, a subsidiary of ByteDance. It relies on the Douyin platform and basically produces short video content for users of the platform. It is compatible with iOS, Android, and Windows. , MacOS and other operating systems. Jianying officially announced the upgrade of its membership system and launched a new SVIP, which includes a variety of AI black technologies, such as intelligent translation, intelligent highlighting, intelligent packaging, digital human synthesis, etc. In terms of price, the monthly fee for clipping SVIP is 79 yuan, the annual fee is 599 yuan (note on this site: equivalent to 49.9 yuan per month), the continuous monthly subscription is 59 yuan per month, and the continuous annual subscription is 499 yuan per year (equivalent to 41.6 yuan per month) . In addition, the cut official also stated that in order to improve the user experience, those who have subscribed to the original VIP
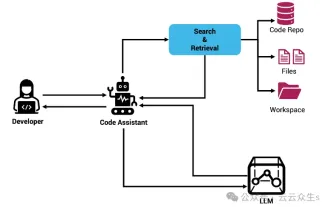
Improve developer productivity, efficiency, and accuracy by incorporating retrieval-enhanced generation and semantic memory into AI coding assistants. Translated from EnhancingAICodingAssistantswithContextUsingRAGandSEM-RAG, author JanakiramMSV. While basic AI programming assistants are naturally helpful, they often fail to provide the most relevant and correct code suggestions because they rely on a general understanding of the software language and the most common patterns of writing software. The code generated by these coding assistants is suitable for solving the problems they are responsible for solving, but often does not conform to the coding standards, conventions and styles of the individual teams. This often results in suggestions that need to be modified or refined in order for the code to be accepted into the application
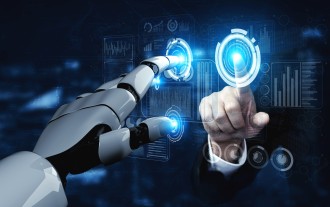
Large Language Models (LLMs) are trained on huge text databases, where they acquire large amounts of real-world knowledge. This knowledge is embedded into their parameters and can then be used when needed. The knowledge of these models is "reified" at the end of training. At the end of pre-training, the model actually stops learning. Align or fine-tune the model to learn how to leverage this knowledge and respond more naturally to user questions. But sometimes model knowledge is not enough, and although the model can access external content through RAG, it is considered beneficial to adapt the model to new domains through fine-tuning. This fine-tuning is performed using input from human annotators or other LLM creations, where the model encounters additional real-world knowledge and integrates it
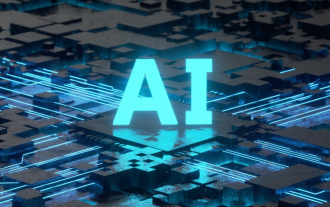
Editor |ScienceAI Question Answering (QA) data set plays a vital role in promoting natural language processing (NLP) research. High-quality QA data sets can not only be used to fine-tune models, but also effectively evaluate the capabilities of large language models (LLM), especially the ability to understand and reason about scientific knowledge. Although there are currently many scientific QA data sets covering medicine, chemistry, biology and other fields, these data sets still have some shortcomings. First, the data form is relatively simple, most of which are multiple-choice questions. They are easy to evaluate, but limit the model's answer selection range and cannot fully test the model's ability to answer scientific questions. In contrast, open-ended Q&A
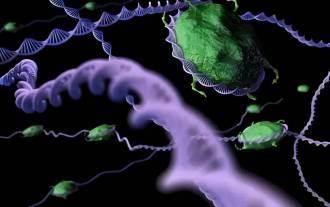
Editor | KX In the field of drug research and development, accurately and effectively predicting the binding affinity of proteins and ligands is crucial for drug screening and optimization. However, current studies do not take into account the important role of molecular surface information in protein-ligand interactions. Based on this, researchers from Xiamen University proposed a novel multi-modal feature extraction (MFE) framework, which for the first time combines information on protein surface, 3D structure and sequence, and uses a cross-attention mechanism to compare different modalities. feature alignment. Experimental results demonstrate that this method achieves state-of-the-art performance in predicting protein-ligand binding affinities. Furthermore, ablation studies demonstrate the effectiveness and necessity of protein surface information and multimodal feature alignment within this framework. Related research begins with "S
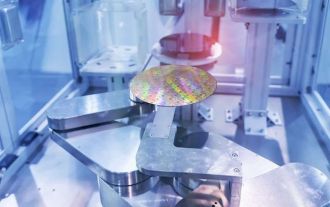
According to news from this website on July 5, GlobalFoundries issued a press release on July 1 this year, announcing the acquisition of Tagore Technology’s power gallium nitride (GaN) technology and intellectual property portfolio, hoping to expand its market share in automobiles and the Internet of Things. and artificial intelligence data center application areas to explore higher efficiency and better performance. As technologies such as generative AI continue to develop in the digital world, gallium nitride (GaN) has become a key solution for sustainable and efficient power management, especially in data centers. This website quoted the official announcement that during this acquisition, Tagore Technology’s engineering team will join GLOBALFOUNDRIES to further develop gallium nitride technology. G
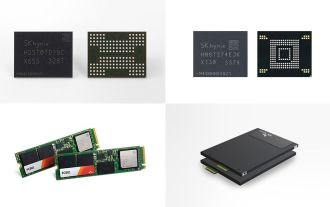
According to news from this site on August 1, SK Hynix released a blog post today (August 1), announcing that it will attend the Global Semiconductor Memory Summit FMS2024 to be held in Santa Clara, California, USA from August 6 to 8, showcasing many new technologies. generation product. Introduction to the Future Memory and Storage Summit (FutureMemoryandStorage), formerly the Flash Memory Summit (FlashMemorySummit) mainly for NAND suppliers, in the context of increasing attention to artificial intelligence technology, this year was renamed the Future Memory and Storage Summit (FutureMemoryandStorage) to invite DRAM and storage vendors and many more players. New product SK hynix launched last year
