


Artificial Intelligence, Machine Learning, and the Future of Software Development
Every successful interaction you have with your favorite apps is the result of a collaborative effort by the Quality Assurance (QA) team. These tireless problem hunters ensure that every aspect of the applications that mobile device users around the world rely on for their daily needs runs smoothly with every release and update.
When you wake up to the sound of your alarm in the morning, check the weather, or send a message to a loved one, we must give thanks to these often unsung heroes.
When the team's efforts failed, they were sure to hear the news: Many users didn't hesitate to provide negative feedback on popular (and very obvious) review sites.
The expectation of modern mobile app users - which is pretty much all of us these days - is perfection, and the primary goal of QA teams is to ensure every deployment of a bug-free product.
The presence of bugs and issues can quickly sink an application. Unfortunately, ensuring a bug-free experience is no easy task. And it's only getting more complicated. Today's software development world is becoming increasingly complex, and testing against the many potentials and scenarios that this complexity brings means that testing itself is becoming increasingly complex and resource-intensive.
Given the history of mobile application development, it is quite reasonable to expect that applications will only become more complex and require more advanced and frequent testing. But does it have to be this way? Are we destined to need more and more staff and bigger and bigger QA teams?
The 1980s: Manual Testing
Let’s take a moment to consider how we got here. Until recently - "Wow - I guess this was really the 1980s so long ago" - software QA teams relied heavily on manual testing of their equipment to ensure the products they brought to market performed well.
It was a simpler time when devices had far fewer features and active scenarios, so hand coding was an adequate way to test. Although tedious work takes a lot of time when performed thoroughly, manual testing works well for testers.
But technology, being an ever-evolving and improving beast, has ushered in changes in the form of automation, dramatically improving the testing process. Software continues to advance and become more complex.
1990s – 2010s: Coding Test Automation
Over the next few decades, advancements in testing freed QA testers from the requirement to physically walk through test cases. They no longer have to manually find bugs in spaghetti piles of code.
They have a new weapon in the war on software issues: manual testing at scale has become impractical, and if any QA team is going to thoroughly test a potential release in a reasonable amount of time, they need to The following is an automated tool for executing test scripts.
So, is the complexity war won? Not completely. It's best to think of automated testing less as a revolutionary innovation and more as another step in the arms race with the ever-evolving complexity of software.
The clock is ticking, but there is no clear victory on the horizon. As mobile apps gain popularity and become a core tool in many of our daily lives, automated testing is slowly losing ground. Fortunately, a long-awaited change is coming, a real revolution.
2020s: Codeless Test Automation
Until recently, the dilemma of QA testing teams had become quite dire indeed. To ensure high-quality product releases, automated testing requires increasingly sophisticated coding tools, which means QA teams need to dedicate more and more programmers to testing instead of other tasks, such as generating new features. Not only is this increasingly expensive, it also means pushing release dates further and further back. But the alternative, a catastrophic launch, can be much more expensive (as many high-profile failed launches have proven).
But the inevitable happened. Through the principle of abstraction—interface-based representation paves the way for extremely complex processes (think, for example, of the 1s and 0s hiding behind the article you are reading)—many experts have long heralded a new layer of abstraction, one that There’s a “no-code revolution” that has really come to fruition over the past few years.
Several platforms have emerged recently that allow for the use of no-code solutions in various industries. One of the more obvious examples of the no-code revolution is the popularity of true WYSIWYG website editors (think Squarespace or Wix), and in the less obvious area of software testing, the company I founded, Sofy, is a unique platform , which provides code-less testing for mobile applications.
The no-code revolution has brought about sea-changes, allowing non-experts to handle complex tasks and giving experts more time to handle other tasks. Therefore, we will undoubtedly see more and more no-code solutions for various industries in the near future.
2025? Truly Smart Self-Testing Software
That said, in the scheme of things, the no-code revolution is just another step forward, I believe the next step in software testing is to test itself software.
I'm not alone in this: Like the no-code revolution, self-testing software has been an expected reality for years. At the rate at which technology is changing and growing, it’s not absurd to imagine that by 2025, intelligent test automation (i.e., self-testing software) that can test AI operations without human intervention will be greatly expanded.
Currently, limited implementations of smart testing improve the speed and quality of software releases by relying on machine learning (ML) and artificial intelligence platforms. This allows for rapid and continuous testing (and therefore improved ROI). Additionally, AI can replicate human intelligence, while ML allows computers to learn without human intervention.
Artificial intelligence and machine learning employ deep learning-based algorithms to access data and learn from the data by extracting patterns for more efficient debugging and decision-making. Additionally, this technology allows QA teams to perform many tests across a variety of devices and different form factors.
Not days, but hours. Nowthis isa revolution.
No code still requires people; people are not machines: they make mistakes. Even without code—albeit greatly reduced—human error is still a factor that causes serious problems. Consider the overuse of resources, time, and effort caused by manual testing.
Smart Testing automatically generates and maintains test cases and generates valuable benefits that can be summarized as increased productivity and output quality. But to achieve intelligent test automation, you must first combine the following elements:
- Learning from human input: When a machine performs testing, it must act like a human. It must understand what humans need and want, and how humans use devices. As we discussed, this can be difficult to predict, and complex applications mean complex testing scenarios and patterns. However, the machine must be understood and operated from this vantage point.
- Learn from real-life product data: The machine must understand how the application is used in different production environments. This includes understanding the device that may be in use, the language it is set in and the flow of its use, including the use of menus, screens and actions.
- Training Data: Like self-driving cars (a nut that has yet to be cracked), machine learning requires training data to help outline software patterns.
These three items must be internalized and tested thoroughly for every code change. They must then be aggregated and prioritized in a seamless and intelligent manner. This is no small feat, but we will continue to work towards the next step.
We don’t have that yet. Each of these steps must be completed before we can move forward, but it's really just a matter of time.
Self-testing software is just the first step: I predict we can expect other no-code examples just hitting the market in the direction of machine learning. I believe it's only a matter of time before generating entire websites based on some user specified parameters becomes a reality. Today, the no-code revolution has finally arrived, but with it comes the beginning of another revolution.
The above is the detailed content of Artificial Intelligence, Machine Learning, and the Future of Software Development. For more information, please follow other related articles on the PHP Chinese website!

Hot AI Tools

Undresser.AI Undress
AI-powered app for creating realistic nude photos

AI Clothes Remover
Online AI tool for removing clothes from photos.

Undress AI Tool
Undress images for free

Clothoff.io
AI clothes remover

Video Face Swap
Swap faces in any video effortlessly with our completely free AI face swap tool!

Hot Article

Hot Tools

Notepad++7.3.1
Easy-to-use and free code editor

SublimeText3 Chinese version
Chinese version, very easy to use

Zend Studio 13.0.1
Powerful PHP integrated development environment

Dreamweaver CS6
Visual web development tools

SublimeText3 Mac version
God-level code editing software (SublimeText3)

Hot Topics




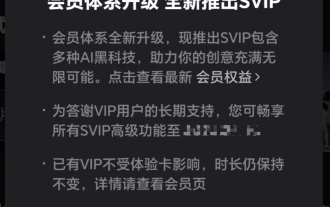
This site reported on June 27 that Jianying is a video editing software developed by FaceMeng Technology, a subsidiary of ByteDance. It relies on the Douyin platform and basically produces short video content for users of the platform. It is compatible with iOS, Android, and Windows. , MacOS and other operating systems. Jianying officially announced the upgrade of its membership system and launched a new SVIP, which includes a variety of AI black technologies, such as intelligent translation, intelligent highlighting, intelligent packaging, digital human synthesis, etc. In terms of price, the monthly fee for clipping SVIP is 79 yuan, the annual fee is 599 yuan (note on this site: equivalent to 49.9 yuan per month), the continuous monthly subscription is 59 yuan per month, and the continuous annual subscription is 499 yuan per year (equivalent to 41.6 yuan per month) . In addition, the cut official also stated that in order to improve the user experience, those who have subscribed to the original VIP
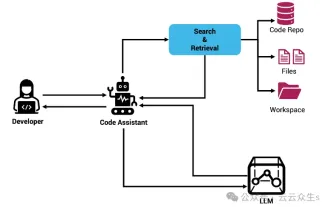
Improve developer productivity, efficiency, and accuracy by incorporating retrieval-enhanced generation and semantic memory into AI coding assistants. Translated from EnhancingAICodingAssistantswithContextUsingRAGandSEM-RAG, author JanakiramMSV. While basic AI programming assistants are naturally helpful, they often fail to provide the most relevant and correct code suggestions because they rely on a general understanding of the software language and the most common patterns of writing software. The code generated by these coding assistants is suitable for solving the problems they are responsible for solving, but often does not conform to the coding standards, conventions and styles of the individual teams. This often results in suggestions that need to be modified or refined in order for the code to be accepted into the application
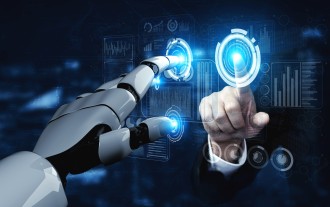
Large Language Models (LLMs) are trained on huge text databases, where they acquire large amounts of real-world knowledge. This knowledge is embedded into their parameters and can then be used when needed. The knowledge of these models is "reified" at the end of training. At the end of pre-training, the model actually stops learning. Align or fine-tune the model to learn how to leverage this knowledge and respond more naturally to user questions. But sometimes model knowledge is not enough, and although the model can access external content through RAG, it is considered beneficial to adapt the model to new domains through fine-tuning. This fine-tuning is performed using input from human annotators or other LLM creations, where the model encounters additional real-world knowledge and integrates it

To learn more about AIGC, please visit: 51CTOAI.x Community https://www.51cto.com/aigc/Translator|Jingyan Reviewer|Chonglou is different from the traditional question bank that can be seen everywhere on the Internet. These questions It requires thinking outside the box. Large Language Models (LLMs) are increasingly important in the fields of data science, generative artificial intelligence (GenAI), and artificial intelligence. These complex algorithms enhance human skills and drive efficiency and innovation in many industries, becoming the key for companies to remain competitive. LLM has a wide range of applications. It can be used in fields such as natural language processing, text generation, speech recognition and recommendation systems. By learning from large amounts of data, LLM is able to generate text
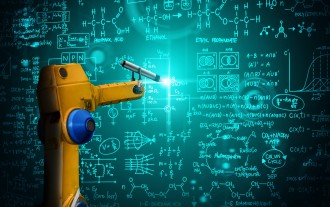
Machine learning is an important branch of artificial intelligence that gives computers the ability to learn from data and improve their capabilities without being explicitly programmed. Machine learning has a wide range of applications in various fields, from image recognition and natural language processing to recommendation systems and fraud detection, and it is changing the way we live. There are many different methods and theories in the field of machine learning, among which the five most influential methods are called the "Five Schools of Machine Learning". The five major schools are the symbolic school, the connectionist school, the evolutionary school, the Bayesian school and the analogy school. 1. Symbolism, also known as symbolism, emphasizes the use of symbols for logical reasoning and expression of knowledge. This school of thought believes that learning is a process of reverse deduction, through existing
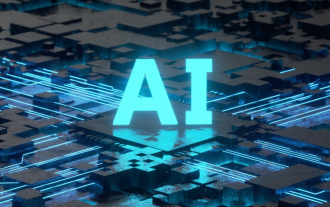
Editor |ScienceAI Question Answering (QA) data set plays a vital role in promoting natural language processing (NLP) research. High-quality QA data sets can not only be used to fine-tune models, but also effectively evaluate the capabilities of large language models (LLM), especially the ability to understand and reason about scientific knowledge. Although there are currently many scientific QA data sets covering medicine, chemistry, biology and other fields, these data sets still have some shortcomings. First, the data form is relatively simple, most of which are multiple-choice questions. They are easy to evaluate, but limit the model's answer selection range and cannot fully test the model's ability to answer scientific questions. In contrast, open-ended Q&A
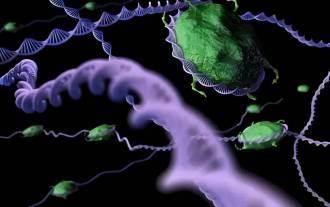
Editor | KX In the field of drug research and development, accurately and effectively predicting the binding affinity of proteins and ligands is crucial for drug screening and optimization. However, current studies do not take into account the important role of molecular surface information in protein-ligand interactions. Based on this, researchers from Xiamen University proposed a novel multi-modal feature extraction (MFE) framework, which for the first time combines information on protein surface, 3D structure and sequence, and uses a cross-attention mechanism to compare different modalities. feature alignment. Experimental results demonstrate that this method achieves state-of-the-art performance in predicting protein-ligand binding affinities. Furthermore, ablation studies demonstrate the effectiveness and necessity of protein surface information and multimodal feature alignment within this framework. Related research begins with "S
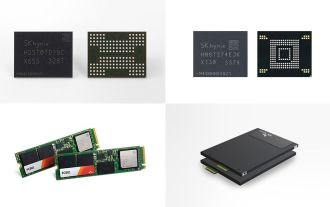
According to news from this site on August 1, SK Hynix released a blog post today (August 1), announcing that it will attend the Global Semiconductor Memory Summit FMS2024 to be held in Santa Clara, California, USA from August 6 to 8, showcasing many new technologies. generation product. Introduction to the Future Memory and Storage Summit (FutureMemoryandStorage), formerly the Flash Memory Summit (FlashMemorySummit) mainly for NAND suppliers, in the context of increasing attention to artificial intelligence technology, this year was renamed the Future Memory and Storage Summit (FutureMemoryandStorage) to invite DRAM and storage vendors and many more players. New product SK hynix launched last year
