PwC: 11 security trends in ChatGPT and generative AI
Recently, PwC analysts shared their views with foreign technology media VentureBeat on how tools such as generative artificial intelligence and ChatGPT will affect the threat landscape and what use cases will emerge for defenders.
They believe that while AI’s ability to generate malicious code and phishing emails creates new challenges for enterprises, it also opens the door to a range of defensive use cases, such as threat detection. , remediation guidance, and securing Kubernetes and cloud environments, and more.
Overall, analysts are optimistic that defensive use cases will rise in the long term to combat malicious uses of AI.
Here are 11 predictions about how generative artificial intelligence will impact cybersecurity in the future.
1. Malicious Use of Artificial Intelligence
When it comes to what we can We are at an inflection point when it comes to leveraging artificial intelligence, a paradigm shift that affects everyone and everything. When AI is in the hands of citizens and consumers, great things can happen.
At the same time, it can be used by malicious threat actors for nefarious purposes, such as malware and sophisticated phishing emails.
Given the many unknowns about the future capabilities and potential of artificial intelligence, it is critical that organizations develop strong procedures to build resilience against malicious networks.
There also needs to be regulation based on social values, stipulating that the use of this technology must be ethical. At the same time, we need to be “smart” users of this tool and consider what safeguards are needed so that AI delivers maximum value while minimizing risk.
2. Need to protect the training and output of artificial intelligence
Now, generative artificial intelligence has the ability to The ability to help enterprises transform is important for leaders to partner with companies that have a deep understanding of how to navigate growing security and privacy considerations.
There are two reasons. First, companies must protect the training of AI because the unique knowledge they gain from fine-tuning models will be critical to how they run their businesses, deliver better products and services, and engage with employees, customers, and ecosystems.
Second, companies must also protect the cues and reactions they get from generative AI solutions because they reflect what the company’s customers and employees are doing with the technology.
3. Develop a generative AI usage policy
When you consider using your own content, files and When assets further train (fine-tune) a generative AI model so that it can operate on your business's unique capabilities within your (industry/professional) context, many interesting business use cases emerge. In this way, companies can use their unique intellectual property and knowledge to expand the way generative AI works.
This is where security and privacy become important. For a business, the way you enable generative AI to produce content should be your business’s privacy. Fortunately, most generative AI platforms have this in mind from the beginning, and are designed to prompt, output, and fine-tune content securely and privately.
However, now all users understand this. Therefore, any enterprise must establish policies for the use of generative AI to prevent confidential and private data from entering public systems and establish a safe and secure environment for generative AI within the enterprise.
4. Modern security auditing
Generative artificial intelligence is likely to lead to innovation in audit work. Sophisticated generative AI has the ability to create responses that take into account certain situations while being written in simple, understandable language.
What this technology provides is a single point for information and guidance, while also enabling document automation and analyzing data to respond to specific queries - all with great efficiency. This is a win-win result.
It’s easy to see that this capability can provide a better experience for our employees, which in turn provides a better experience for our customers.
5. Pay more attention to data hygiene and evaluation bias
Any data input into the artificial intelligence system may be risk of theft or misuse. First, determining the appropriate data to enter into the system will help reduce the risk of losing confidential and private information.
Additionally, it is important to do proper data collection to formulate detailed and targeted tips and feed them into the system so that you can get a more valuable output.
Once you have your output, you need to check if there are any inherent biases within the system. During this process, bring in a diverse team of professionals to help assess any bias.
Unlike coded or scripted solutions, generative AI is based on trained models, so the responses they give are not 100% predictable. For generative AI to give the most trustworthy output, it requires collaboration between the technology behind it and the people leveraging it.
6. Keep up with expanding risks and master the basics
Now that generative AI is being widely used Adopting and implementing strong security measures is necessary to protect against threat actors. The capabilities of this technology make it possible for cybercriminals to create deepfake images and more easily execute malware and ransomware strikes, and companies need to be prepared for these challenges.
The most effective cyber measures continue to receive the least attention: By maintaining basic cyber hygiene and compressing bulky legacy systems, companies can reduce cybercriminals.
Consolidating operating environments can reduce costs, allowing companies to maximize efficiency and focus on improving their cybersecurity measures.
7. Create new jobs and responsibilities
Overall, I recommend that companies consider embracing generative AI, rather than building firewalls and resistance - but with appropriate safeguards and risk mitigation in place. Generative AI has some very interesting potential in terms of how work gets done; it can actually help free up human time for analysis and creation.
The emergence of generative AI has the potential to lead to new jobs and responsibilities related to the technology itself - and create a responsibility to ensure that AI is used ethically and responsibly.
It will also require employees who use the information to develop a new skill - the ability to evaluate and identify whether the content created is accurate.
Just like calculators are used for simple math-related tasks, there are still many human skills that need to be applied in the daily use of generative AI, such as critical thinking and Customization with purpose—to unleash the full power of generative AI.
So while on the surface it may seem like a threat in terms of the ability to automate human tasks, it can also unleash creativity and help people excel at work.
8. Leveraging Artificial Intelligence to Optimize Network Investments
Even in times of economic uncertainty, companies There are no active efforts to reduce cybersecurity spending in 2023; however, CISOs must consider whether their investment decisions make more economical sense.
They are facing pressure to do more with less, leading them to invest in technology that replaces overly manual risk prevention and mitigation processes with automated alternatives.
Although generative AI is not perfect, it is very fast, efficient and coherent, and its skills are improving rapidly. By implementing the right risk technology – such as machine learning mechanisms designed for greater risk coverage and detection – organizations can save money, time and people and be better able to navigate and withstand any future uncertainty.
#9. Enhance Threat Intelligence
#While companies unleashing generative AI capabilities are focused on protection to prevent The creation and spread of malware, misinformation, or disinformation, but we need to assume that generative AI will be used by bad actors for these purposes and act in advance.
In 2023, we expect to see further enhancements in threat intelligence and other defense capabilities to leverage generative AI to do good for society. Generative AI will allow for fundamental advances in efficiency and real-time trust decision-making. For example, access to systems and information can be formed into real-time conclusions with a much higher level of confidence than currently deployed access and identity models.
What is certain is that generative AI will have a profound impact on every industry and the way companies within it operate; PwC believes these advancements will continue Human-led and technology-driven, 2023 will see the most rapid advances, setting the direction for the coming decades.
10. Threat Prevention and Managing Compliance Risks
As the threat landscape continues to evolve, health departments- - an industry awash in personal information - continues to find itself in the crosshairs of threat actors.
Health industry executives are increasing their cyber budgets and investing in automated technologies that can not only help prevent cyber strikes but also manage compliance risks, better Protect patient and staff data, reduce healthcare costs, eliminate inefficient processes, and more.
As generative artificial intelligence continues to advance, so do the risks and opportunities associated with securing healthcare systems, underscoring the ways in which the healthcare industry is building its capabilities as it embraces this new technology. The importance of cyber defense and resilience.
11. Implement a digital trust strategy
The speed of innovation in technologies like generative AI, coupled with the continued The “patchwork” of regulation and the erosion of trust in institutions require a more strategic approach.
By pursuing a digital trust strategy, enterprises can better coordinate traditionally siled functions such as cybersecurity, privacy and data governance, allowing them to anticipate risk while also freeing up the enterprise value.
At its core, the Digital Trust Framework identifies solutions that go beyond compliance – instead prioritizing trust and value exchange between organizations and customers.
The above is the detailed content of PwC: 11 security trends in ChatGPT and generative AI. For more information, please follow other related articles on the PHP Chinese website!

Hot AI Tools

Undresser.AI Undress
AI-powered app for creating realistic nude photos

AI Clothes Remover
Online AI tool for removing clothes from photos.

Undress AI Tool
Undress images for free

Clothoff.io
AI clothes remover

AI Hentai Generator
Generate AI Hentai for free.

Hot Article

Hot Tools

Notepad++7.3.1
Easy-to-use and free code editor

SublimeText3 Chinese version
Chinese version, very easy to use

Zend Studio 13.0.1
Powerful PHP integrated development environment

Dreamweaver CS6
Visual web development tools

SublimeText3 Mac version
God-level code editing software (SublimeText3)

Hot Topics
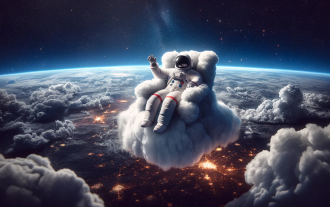
DALL-E 3 was officially introduced in September of 2023 as a vastly improved model than its predecessor. It is considered one of the best AI image generators to date, capable of creating images with intricate detail. However, at launch, it was exclus
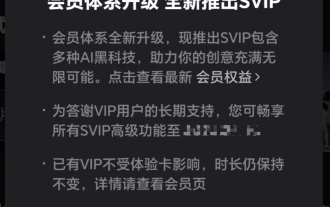
This site reported on June 27 that Jianying is a video editing software developed by FaceMeng Technology, a subsidiary of ByteDance. It relies on the Douyin platform and basically produces short video content for users of the platform. It is compatible with iOS, Android, and Windows. , MacOS and other operating systems. Jianying officially announced the upgrade of its membership system and launched a new SVIP, which includes a variety of AI black technologies, such as intelligent translation, intelligent highlighting, intelligent packaging, digital human synthesis, etc. In terms of price, the monthly fee for clipping SVIP is 79 yuan, the annual fee is 599 yuan (note on this site: equivalent to 49.9 yuan per month), the continuous monthly subscription is 59 yuan per month, and the continuous annual subscription is 499 yuan per year (equivalent to 41.6 yuan per month) . In addition, the cut official also stated that in order to improve the user experience, those who have subscribed to the original VIP
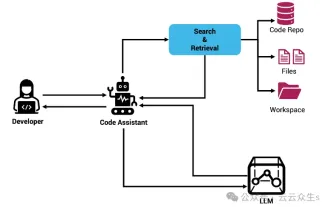
Improve developer productivity, efficiency, and accuracy by incorporating retrieval-enhanced generation and semantic memory into AI coding assistants. Translated from EnhancingAICodingAssistantswithContextUsingRAGandSEM-RAG, author JanakiramMSV. While basic AI programming assistants are naturally helpful, they often fail to provide the most relevant and correct code suggestions because they rely on a general understanding of the software language and the most common patterns of writing software. The code generated by these coding assistants is suitable for solving the problems they are responsible for solving, but often does not conform to the coding standards, conventions and styles of the individual teams. This often results in suggestions that need to be modified or refined in order for the code to be accepted into the application
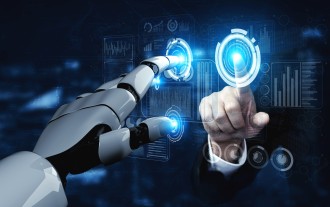
Large Language Models (LLMs) are trained on huge text databases, where they acquire large amounts of real-world knowledge. This knowledge is embedded into their parameters and can then be used when needed. The knowledge of these models is "reified" at the end of training. At the end of pre-training, the model actually stops learning. Align or fine-tune the model to learn how to leverage this knowledge and respond more naturally to user questions. But sometimes model knowledge is not enough, and although the model can access external content through RAG, it is considered beneficial to adapt the model to new domains through fine-tuning. This fine-tuning is performed using input from human annotators or other LLM creations, where the model encounters additional real-world knowledge and integrates it
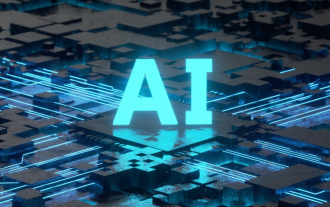
Editor |ScienceAI Question Answering (QA) data set plays a vital role in promoting natural language processing (NLP) research. High-quality QA data sets can not only be used to fine-tune models, but also effectively evaluate the capabilities of large language models (LLM), especially the ability to understand and reason about scientific knowledge. Although there are currently many scientific QA data sets covering medicine, chemistry, biology and other fields, these data sets still have some shortcomings. First, the data form is relatively simple, most of which are multiple-choice questions. They are easy to evaluate, but limit the model's answer selection range and cannot fully test the model's ability to answer scientific questions. In contrast, open-ended Q&A
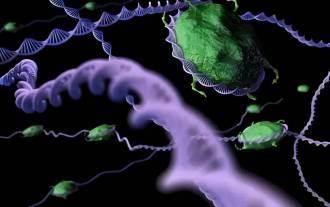
Editor | KX In the field of drug research and development, accurately and effectively predicting the binding affinity of proteins and ligands is crucial for drug screening and optimization. However, current studies do not take into account the important role of molecular surface information in protein-ligand interactions. Based on this, researchers from Xiamen University proposed a novel multi-modal feature extraction (MFE) framework, which for the first time combines information on protein surface, 3D structure and sequence, and uses a cross-attention mechanism to compare different modalities. feature alignment. Experimental results demonstrate that this method achieves state-of-the-art performance in predicting protein-ligand binding affinities. Furthermore, ablation studies demonstrate the effectiveness and necessity of protein surface information and multimodal feature alignment within this framework. Related research begins with "S
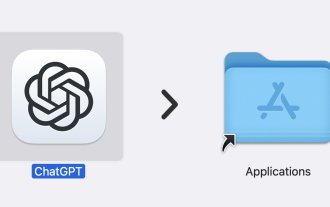
Open AI’s ChatGPT Mac application is now available to everyone, having been limited to only those with a ChatGPT Plus subscription for the last few months. The app installs just like any other native Mac app, as long as you have an up to date Apple S
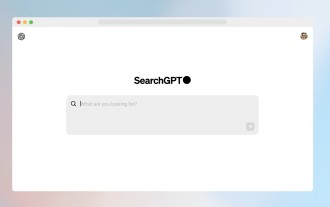
Open AI is finally making its foray into search. The San Francisco company has recently announced a new AI tool with search capabilities. First reported by The Information in February this year, the new tool is aptly called SearchGPT and features a c
