


Meta releases multi-purpose large model open source to help move one step closer to visual unification
After open source the SAM model that “divides everything”, Meta is going further and further on the road to “visual basic model”.
This time, they open sourced a set of models called DINOv2. These models can produce high-performance visual representations that can be used for downstream tasks such as classification, segmentation, image retrieval, and depth estimation without fine-tuning.
##This set of models has the following characteristics:
- Uses self-supervised training without requiring a large amount of labeled data;
- can be used as the backbone of almost all CV tasks, no fine-tuning is required, Such as image classification, segmentation, image retrieval and depth estimation;
- Learn features directly from images without relying on text descriptions, which allows the model to better understand local information;
- Can learn from any image collection;
- A pre-trained version of DINOv2 is already available and is comparable to CLIP and OpenCLIP on a range of tasks.
- Paper link: https://arxiv.org/pdf/2304.07193.pdf
- Project link: https://dinov2.metademolab.com/
Learning non-task-specific pre-trained representations has become a standard in natural language processing. You can use these features "as-is" (no fine-tuning required), and they perform significantly better on downstream tasks than task-specific models. This success is due to pre-training on large amounts of raw text using auxiliary objectives, such as language modeling or word vectors, which do not require supervision.
As this paradigm shift occurs in the field of NLP, it is expected that similar "base" models will emerge in computer vision. These models should generate visual features that work "out of the box" on any task, whether at the image level (e.g. image classification) or pixel level (e.g. segmentation).
These basic models have great hope to focus on text-guided pre-training, that is, using a form of text supervision to guide the training of features. This form of text-guided pre-training limits the information about the image that can be retained, as the caption only approximates the rich information in the image, and finer, complex pixel-level information may not be discovered with this supervision. Furthermore, these image encoders require already aligned text-image corpora and do not provide the flexibility of their text counterparts, i.e. cannot learn from raw data alone.
An alternative to text-guided pre-training is self-supervised learning, where features are learned from images only. These methods are conceptually closer to front-end tasks such as language modeling, and can capture information at the image and pixel level. However, despite their potential to learn general features, most of the improvements in self-supervised learning have been achieved in the context of pre-training on the small refined dataset ImageNet1k. There have been some efforts by some researchers to extend these methods beyond ImageNet-1k, but they focused on unfiltered datasets, which often resulted in significant degradation in performance quality. This is due to a lack of control over data quality and diversity, which are critical to producing good results.
In this work, researchers explore whether self-supervised learning is possible to learn general visual features if pre-trained on a large amount of refined data. They revisit existing discriminative self-supervised methods that learn features at the image and patch level, such as iBOT, and reconsider some of their design choices under larger datasets. Most of our technical contributions are tailored to stabilize and accelerate discriminative self-supervised learning when scaling model and data sizes. These improvements made their method approximately 2x faster and required 1/3 less memory than similar discriminative self-supervised methods, allowing them to take advantage of longer training and larger batch sizes.
Regarding the pre-training data, they built an automated pipeline for filtering and rebalancing the dataset from a large collection of unfiltered images. This is inspired by pipelines used in NLP, where data similarity is used instead of external metadata, and manual annotation is not required. A major difficulty when processing images is to rebalance concepts and avoid overfitting in some dominant modes. In this work, the naive clustering method can solve this problem well, and the researchers collected a small but diverse corpus consisting of 142M images to validate their method.
Finally, the researchers provide various pre-trained vision models, called DINOv2, trained on their data using different visual Transformer (ViT) architectures. They released all models and code to retrain DINOv2 on any data. When extended, they validated the quality of DINOv2 on a variety of computer vision benchmarks at the image and pixel levels, as shown in Figure 2. We conclude that self-supervised pre-training alone is a good candidate for learning transferable frozen features, comparable to the best publicly available weakly supervised models.
Data Processing
The researchers assembled their refined LVD by retrieving images from large amounts of unfiltered data that were close to images in multiple refined datasets -142M dataset. In their paper, they describe the main components in the data pipeline, including curated/unfiltered data sources, image deduplication steps, and retrieval systems. The entire pipeline does not require any metadata or text and processes images directly, as shown in Figure 3. The reader is referred to Appendix A for further details on the model methodology.
Figure 3: Overview of the data processing pipeline. Images from refined and non-refined data sources are first mapped to embeddings. The unrefined image is then deduplicated before being matched to the standard image. The resulting combination further enriches the initial data set through a self-supervised retrieval system.
Discriminative self-supervised pre-training
The researchers learned their features through a discriminative self-supervised method that can see The work is a combination of DINO and iBOT losses, centered on SwAV. They also added a regularizer to propagate features and a brief high-resolution training phase.
Efficient Implementation
They considered several improvements to train the model on a larger scale. The model is trained on an A100 GPU using PyTorch 2.0, and the code can also be used with a pretrained model for feature extraction. Details of the model are in Appendix Table 17. On the same hardware, the DINOv2 code uses only 1/3 of the memory and runs 2 times faster than the iBOT implementation.
Experimental results
In this section, the researcher will introduce the new model in many image understanding Empirical evaluation on tasks. They evaluated global and local image representations, including category and instance-level recognition, semantic segmentation, monocular depth prediction, and action recognition.
ImageNet Classification
#Other Image and Video Classification Benchmarks
##Instance identification
Dense recognition task
Qualitative results
The above is the detailed content of Meta releases multi-purpose large model open source to help move one step closer to visual unification. For more information, please follow other related articles on the PHP Chinese website!

Hot AI Tools

Undresser.AI Undress
AI-powered app for creating realistic nude photos

AI Clothes Remover
Online AI tool for removing clothes from photos.

Undress AI Tool
Undress images for free

Clothoff.io
AI clothes remover

AI Hentai Generator
Generate AI Hentai for free.

Hot Article

Hot Tools

Notepad++7.3.1
Easy-to-use and free code editor

SublimeText3 Chinese version
Chinese version, very easy to use

Zend Studio 13.0.1
Powerful PHP integrated development environment

Dreamweaver CS6
Visual web development tools

SublimeText3 Mac version
God-level code editing software (SublimeText3)

Hot Topics


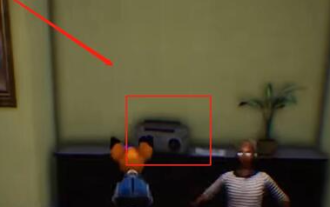
The Terror Corridor is a mission in Goat Simulator 3. How can you complete this mission? Master the detailed clearance methods and corresponding processes, and be able to complete the corresponding challenges of this mission. The following will bring you Goat Simulator. 3 Horror Corridor Guide to learn related information. Goat Simulator 3 Terror Corridor Guide 1. First, players need to go to Silent Hill in the upper left corner of the map. 2. Here you can see a house with RESTSTOP written on the roof. Players need to operate the goat to enter this house. 3. After entering the room, we first go straight forward, and then turn right. There is a door at the end here, and we go in directly from here. 4. After entering, we also need to walk forward first and then turn right. When we reach the door here, the door will be closed. We need to turn back and find it.
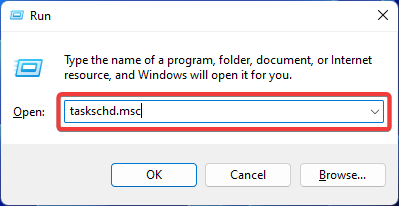
To automate tasks and manage multiple systems, mission planning software is a valuable tool in your arsenal, especially as a system administrator. Windows Task Scheduler does the job perfectly, but lately many people have reported operator rejected request errors. This problem exists in all iterations of the operating system, and even though it has been widely reported and covered, there is no effective solution. Keep reading to find out what might actually work for other people! What is the request in Task Scheduler 0x800710e0 that was denied by the operator or administrator? Task Scheduler allows automating various tasks and applications without user input. You can use it to schedule and organize specific applications, configure automatic notifications, help deliver messages, and more. it
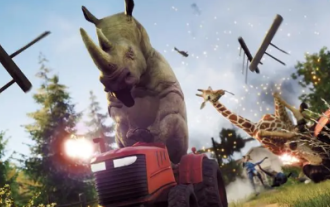
Goat Simulator 3 is a game with classic simulation gameplay, allowing players to fully experience the fun of casual action simulation. The game also has many exciting special tasks. Among them, the Goat Simulator 3 Imperial Tomb task requires players to find the bell tower. Some players are not sure how to operate the three clocks at the same time. Here is the guide to the Tomb of the Tomb mission in Goat Simulator 3! The guide to the Tomb of the Tomb mission in Goat Simulator 3 is to ring the bells in order. Detailed step expansion 1. First, players need to open the map and go to Wuqiu Cemetery. 2. Then go up to the bell tower. There will be three bells inside. 3. Then, in order from largest to smallest, follow the familiarity of 222312312. 4. After completing the knocking, you can complete the mission and open the door to get the lightsaber.
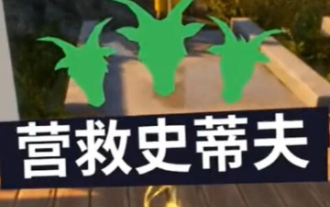
Rescue Steve is a unique task in Goat Simulator 3. What exactly needs to be done to complete it? This task is relatively simple, but we need to be careful not to misunderstand the meaning. Here we will bring you the rescue of Steve in Goat Simulator 3 Task strategies can help you better complete related tasks. Goat Simulator 3 Rescue Steve Mission Strategy 1. First come to the hot spring in the lower right corner of the map. 2. After arriving at the hot spring, you can trigger the task of rescuing Steve. 3. Note that there is a man in the hot spring. Although his name is Steve, he is not the target of this mission. 4. Find a fish named Steve in this hot spring and bring it ashore to complete this task.
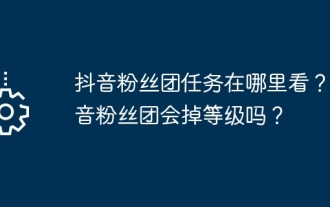
TikTok, as one of the most popular social media platforms at the moment, has attracted a large number of users to participate. On Douyin, there are many fan group tasks that users can complete to obtain certain rewards and benefits. So where can I find Douyin fan club tasks? 1. Where can I view Douyin fan club tasks? In order to find Douyin fan group tasks, you need to visit Douyin's personal homepage. On the homepage, you will see an option called "Fan Club." Click this option and you can browse the fan groups you have joined and related tasks. In the fan club task column, you will see various types of tasks, such as likes, comments, sharing, forwarding, etc. Each task has corresponding rewards and requirements. Generally speaking, after completing the task, you will receive a certain amount of gold coins or experience points.
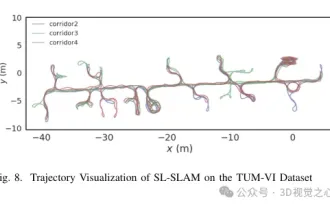
Written previously, today we discuss how deep learning technology can improve the performance of vision-based SLAM (simultaneous localization and mapping) in complex environments. By combining deep feature extraction and depth matching methods, here we introduce a versatile hybrid visual SLAM system designed to improve adaptation in challenging scenarios such as low-light conditions, dynamic lighting, weakly textured areas, and severe jitter. sex. Our system supports multiple modes, including extended monocular, stereo, monocular-inertial, and stereo-inertial configurations. In addition, it also analyzes how to combine visual SLAM with deep learning methods to inspire other research. Through extensive experiments on public datasets and self-sampled data, we demonstrate the superiority of SL-SLAM in terms of positioning accuracy and tracking robustness.
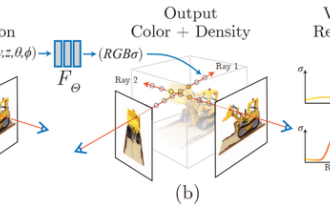
1 Introduction Neural Radiation Fields (NeRF) are a fairly new paradigm in the field of deep learning and computer vision. This technology was introduced in the ECCV2020 paper "NeRF: Representing Scenes as Neural Radiation Fields for View Synthesis" (which won the Best Paper Award) and has since become extremely popular, with nearly 800 citations to date [1 ]. The approach marks a sea change in the traditional way machine learning processes 3D data. Neural radiation field scene representation and differentiable rendering process: composite images by sampling 5D coordinates (position and viewing direction) along camera rays; feed these positions into an MLP to produce color and volumetric densities; and composite these values using volumetric rendering techniques image; the rendering function is differentiable, so it can be passed
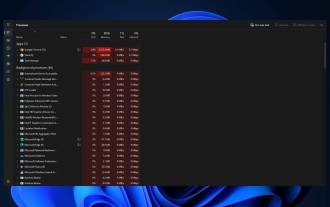
How to Pause Task Manager Process Updates in Windows 11 and Windows 10 Press CTRL+Window Key+Delete to open Task Manager. By default, Task Manager will open the Processes window. As you can see here, all the apps are endlessly moving around and it can be hard to point them down when you want to select them. So, press CTRL and hold it, this will pause the task manager. You can still select apps and even scroll down, but you must hold down the CTRL button at all times.
